Abstract
Purpose
Despite advances in personalizing the efficacy of cancer therapy, our ability to identify patients at risk of severe treatment side effects and provide individualized supportive care is limited. This is particularly the case for mucositis (oral and gastrointestinal), with no comprehensive risk evaluation strategies to identify high-risk patients. We, the Multinational Association for Supportive Care in Cancer/International Society for Oral Oncology (MASCC/ISOO) Mucositis Study Group, therefore aimed to systematically review current evidence on that factors that influence mucositis risk to provide a foundation upon which future risk prediction studies can be based.
Methods
We identified 11,018 papers from PubMed and Web of Science, with 197 records extracted for full review and 113 meeting final eligibility criteria. Data were then synthesized into tables to highlight the level of evidence for each risk predictor.
Results
The strongest level of evidence supported dosimetric parameters as key predictors of mucositis risk. Genetic variants in drug-metabolizing pathways, immune signaling, and cell injury/repair mechanisms were also identified to impact mucositis risk. Factors relating to the individual were variably linked to mucositis outcomes, although female sex and smoking status showed some association with mucositis risk.
Conclusion
Mucositis risk reflects the complex interplay between the host, tumor microenvironment, and treatment specifications, yet the large majority of studies rely on hypothesis-driven, single-candidate approaches. For significant advances in the provision of personalized supportive care, coordinated research efforts with robust multiplexed approaches are strongly advised.
Similar content being viewed by others
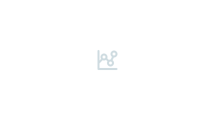
Avoid common mistakes on your manuscript.
Introduction
Individualized care is a key strategic focus in precision medicine with relevance across almost all facets of care. In particular, methods of enhancing anti-cancer treatment efficacy have advanced rapidly, enabling patients to receive treatments specifically tailored to their genetic traits and tumor microenvironment [1, 2]. Unfortunately, the importance of personalized supportive cancer care is less well recognized, with limited appreciation for the complex interactions that account for the significant heterogeneity seen in toxicity profiles of various anti-cancer agents.
Mucositis is a ubiquitous complication of almost all cancer therapies, with treatment-specific manifestations reflecting core pharmacological and biophysical actions of various agents and treatments [3]. Common to all therapies is the high degree of heterogeneity in the clinical manifestations of mucositis, with patients presenting along a spectrum—from mild mucosal irritation to severe, ulcerative lesions—despite equivalent tumor burden, demographic profile, and treatment specifications [4].
In their mild forms, both oral (OM) and gastrointestinal mucositis (GI-M) can be adequately managed with existing supportive care measures [5,6,7,8,9,10]. When severe (NCI CTCAE III–V), OM and GI-M are catalysts for potentially lethal complications including infection, renal insufficiency, and graft versus host disease [11,12,13]. In the setting of pelvic radiation, acute GI-M is by far the largest predictor of late GI injury [14, 15]. When neutropenia is controlled, severe mucositis is the single largest factor defining the maximal tolerated dose of anti-cancer therapy and a significant driver of dose reductions, interruptions/delays, and treatment discontinuation [16]. Severe mucositis requires intensive in-patient supportive care such as the provision of intravenous fluids and electrolytes, parenteral nutrition, and opioid analgesics and thus greatly impacts quality of life for weeks to months [17]. Early intervention is considered critical in managing the clinical burden of severe mucositis; however, it remains unclear who is at risk of developing severe symptoms. As such, there is a clear and currently unmet need to identify patients at risk of severe mucositis to enable methods of risk minimization and the provision of targeted supportive care.
To date, methods of predicting mucositis risk have focused on the individual and their treatment [2]. These approaches are limited as they are largely unmodifiable and highly dichotomous. Similarly, of the studies that have begun to illustrate the genetic nature of mucositis risk [18,19,20], the translation of these findings are plagued by substantial inconsistencies and disparities between the proportion of people that carry distinct mutations and those that develop severe mucositis. Given our understanding of mucositis pathobiology has become increasingly sophisticated [3], new methods of risk prediction must acknowledge the variety of factors that determine mucositis risk and our siloed approach to personalized supportive care must be revised.
This systematic review, coordinated by the Multinational Association for Supportive Care in Cancer/International Society of Oral Oncology (MASCC/ISOO) Mucositis Study Group, represents an in-depth evaluation of the factors that contribute to mucositis risk. We aim to provide a clear overview of the factors identified to contribute to mucositis risk, discuss new approaches to risk prediction, and provide a practical framework for future studies aiming to develop the next generation of risk prediction tools.
Methods
Search strategy, study selection, and data retrieval
PubMed and Web of Sciences were searched for papers published using keywords listed in Table S1. Papers were screened for eligibility (HRW/JB) based on title and abstract. Clinical studies conducted between January 2000 and July 2019, in which the objective was the identification of (1) predictors of OM/GI-M or (2) associations between baseline characteristics and mucositis outcomes, were included. Reviews, preclinical research, strictly interventional studies, and case studies were excluded. Full details of the search strategy can be found in the supplementary material.
Eligible papers were delegated to independent reviewers who (in a single-fashion) extracted data using a standard electronic template to define study design, cancer type and stage, treatment specifications, patient demographics, mucositis outcome measure (OM or GI-M) and assessment scale/study endpoint, blinding, participant recruitment (consecutive/non-consecutive), statistical analyses, and key findings related to mucositis prediction. Irrelevant papers were excluded before all data were synthesized by study leads (HRW/JMB).
Studies were stratified based on the following: (1) patient cohort (children (0–18 years), adults), (2) treatment modality (high-dose chemotherapy/total body irradiation for hematopoietic stem cell transplantation, chemotherapy, radiotherapy, chemoradiation), (2) mucositis type (OM, GI-M), and (3) category of risk predictor (genetic, individual, tumor, treatment, comorbidities/medication use, and miscellaneous). Information to assist with the interpretation of results was additionally extracted including oncology cohort, tumor type, treatment specifications, sample size, drop-out rate, statistical approach(es), and mucositis assessment scale. Data were synthesized and organized into tables indicating evidence for each mucositis risk predictor. Factors were assessed for their association of OM and GI-M and assigned level 1 evidence when ≤ 3 high-quality studies identified an association with mucositis risk, level 2 evidence when 3–7 studies were identified, and level 3 evidence when there were > 7 studies. Inclusion of systematic review/meta-analysis data elevated the level of evidence. Importantly, these tables are intended to provide a simple and high-level overview of the factors identified to influence mucositis risk. They are intended to guide future research, not clinical practice.
Results
A total of 11,018 papers were identified from our initial search strategy, 197 eligible for inclusion (Fig. 1). After a full-text review, further 85 papers were excluded largely due to mucositis not being a primary endpoint, no attempt of risk prediction, or case reports, leaving 113 papers for final inclusion. Studies included in this review were prospective observational, retrospective observational, and secondary analyses of previously conducted randomized control studies. Most studies were conducted in adult patients, with only ten predicting mucositis in children.
The majority of studies evaluated a single risk prediction category (89/113) with cancer regimen-related factors the most common category. Studies evaluating genetic predictors were also common, and closely followed by those assessing factors related to the individual. The remaining studies evaluated the impact of tumor-related factors, biological parameters, medication and supportive care use, comorbidities, hygiene, and the microbiota on mucositis outcomes.
Mucositis assessment
Almost all studies evaluated OM or GI-M in isolation, with only 24 of the 113 studies included evaluating both. There was a significant diversity in type and quality of scales used to define mucositis presence and severity, with both clinical and patient-reported outcomes used. OM was assessed using the National Cancer Institute’s Common Terminology Criteria for Adverse Events (NCI CTCAE; N = 34, including studies that assessed OM and GI-M concurrently), Radiation Oncology Research Group (RTOG; N = 23), the World Health Organization OM assessment scale (WHO; N = 13), the Head and Neck Radiotherapy Questionnaire (HNRQ; N = 1), the Oral Mucositis Assessment Scale (OMAS; N = 1), the Oral Mucositis Index (OMI; N = 4), the Oral Assessment Guide (OAG; N = 1), and the Bearman’s Oral Mucositis Scale (N = 1). GI-M was assessed primarily using the NCI CTCAE (N = 40, including studies that assessed OM and GI-M concurrently); however, other methods were also used including RTOG (N = 4), WHO (N = 3), the Inflammatory Bowel Disease Questionnaire (IBDQ; N = 1), endoscopic evaluation (N = 1), the bowel problem scale (N = 1) [21], and intestinal wall thickness (N = 1) [22]. In N = 8 cases, mucositis severity was reported but the assessment scale used was not specified.
Treatment specifications define mucositis risk
The impact of treatment specifications, particularly dosimetric parameters, on OM and GI-M risk was evaluated in a variety of cohorts undergoing chemotherapy, radiotherapy, and chemoradiotherapy for a variety of solid and hematological tumors (summarized in Table 1). In all cases, “risk” is defined as an increased risk of mucositis unless specified. Clear dose-response relationships were identified for both OM and GI-M, with increasing doses of melphalan [23, 24], methotrexate (MTX) [25], and radiation [26,27,28,29,30] predictive of OM, GI-M, and late rectal morbidity (radiation only). Unsurprisingly, the volume of bowel exposed to radiation was a strong predictor of acute and late GI toxicity reported across several studies [21, 31,32,33,34,35,36,37,38,39,40,41].
Treatment modality was also identified as an important mucositis predictor, with concurrent chemotherapy (in particular, cisplatin, and cetuximab) [42, 43] increasing the risk of OM and conventional 2D/3D radiotherapy associated with more severe mucositis compared with intensity-modulated radiotherapy (IMRT) [41, 44, 45]. One study also reported higher risk of OM in only daily fractionated radiotherapy compared with altered fractionated schedules [46]. Fully ablative/myeloablative conditioning (FAC/MAC) regimens were consistently reported as predictors of mucositis in people with hematological malignancies, particularly when containing etoposide [47], high-dose melphalan [23, 48, 49], busulfan [50], doxorubicin [22], or total body irradiation [51]. Of interest, three studies also reported the potential effect of circadian rhythm on mucositis risk, with radiotherapy delivered in the evening associated with a higher degree of OM [52] and associated weight loss [53] in patients with HNC. This is in contrast to GI-M in women with cervical cancer, with overall diarrhea and grade III–IV diarrhea both significantly increased when radiation was delivered in the morning compared with the evening [54].
Genetic predictors of mucositis risk
Pharmacogenetic variants
Polymorphism affecting a variety of gene clusters was evaluated for their ability to predict mucositis risk (summarized in Table 2). Mutations in drug-metabolizing pathways have been widely described for their influence on mucositis risk including methylenetetrahydrofolate reductase (MTHFR), cytochrome P450 (CYP), dihydropyrimidine dehydrogenase (DYPD), thymidylate synthase (TSMS), ATP-binding cassette (ABC) transporters, and glucoronosyltransferase 1A (UGTA1) (for detailed description of these genes and their pathways, please see [55]). Umbrella reviews support strong predictive power for UGT1A1*6 and *28 for irinotecan-induced GI-M, MTHFR C677 for MTX-induced OM and GI-M, and DPYD- and TYMS-related SNPs for mucositis caused by fluoropyridimine and platinum-based therapies [56,57,58]. The impact of MTHFR mutations on MTX-induced OM was also confirmed in two large cohorts of allo-SCT recipients with the C677 TT genotype associated with OM risk [51, 59], as well as a cohort of pediatric patients undergoing chemotherapy for ALL [60].
Cell signaling variants
Genes related to cellular growth and DNA repair mechanisms were also evaluated for their impact on mucositis risk (Table 2). The NBN rs1805794 CC genotype, high RPM1 gene expression, MDM2 (309T>G), and RB1 rs2227311 were all associated with a high OM risk in HNC patients [55, 61,62,63]. TGFBR25P and RAD51 G315C were associated with increased risk of GI-M in patients undergoing chemoradiotherapy for rectal and anal malignancies [64, 65]. The largest study in this cohort also reported strong GI-M prediction based on the VEGFR2 H472Q Q/Q rs1870377 genotype [66]; however, this was not consistent across both cohorts included in the study. The ERCC1 rs3212986 AA genotype was associated with a greater risk of late-onset GI-M in women with cervical cancer undergoing chemoradiotherapy [67]. Systematic review showed XRCC1 rs25487 was strongly predictive of OM and GI-M risk in HSCT recipients [68]; however, this was not upheld in a smaller cohort of HNC patients [69]. XRCC1 polymorphic variants were also associated with OM risk in HNC patients treated with chemoradiation [70].
Immunogenetic variants
The third category of genetic factors evaluated for their impact on OM and GI-M were those related to inflammatory/immune pathways (Table 2). Immunogenomic studies report variants in pattern recognition receptor pathways, with TLR2/TNFA associated with heightened GI-M risk in people with gastric/colorectal cancers undergoing 5-FU-based chemotherapy [71]. TNF-related variants were also identified as predictors of GI-M, with the 1031 TT genotype (and IL-1B-511 TC/TT) and baseline TNF mRNA expression associated with increased GI-M in patients undergoing chemoradiotherapy for GI [72] and esophageal cancers [73], respectively. Similarly, TNFRSR1A-610T>G, TNFA-1211T>C (CC genotype) and GHLR-2531C>T were strongly associated with OM risk in HNC patients undergoing radiotherapy [74,75,76]. Radiation-induced esophagitis was also linked with mutations in a variety of inflammatory pathways, including PSTG, TNF, IL6, IL4, and IL10 [43].
Uncategorized genetic predictors of OM risk also identified included the GT genotype in EDN1 rs1800541 [77], ZNF24 rs11081899-A [78], APEH c.1521G>C (rs4855883 CC genotype) [75], and miR-1206 rs2114358 (homozygous GG) [79].
Demographic, lifestyle and performance indicators linked with mucositis risk
Evidence from included studies for demographic and lifestyle predictors of mucositis was variable (summarized in Table 3). A strong and consistent outcome was female sex, which was linked to more severe chemotherapy-induced OM and GI-M across several studies [23, 41, 80,81,82,83]. Nonetheless, negative findings were also reported, with particularly robust evidence supporting no correlation between sex and OM in a study of 381 multiple myeloma patients [48]. Similarly, contradictory evidence supported male sex as a predictor of OM in HNC patients undergoing chemoradiotherapy [42].
Age was commonly reported as a predictor of mucositis; however, there was no uniformity with both decreasing and increasing age reported as predictive of mucositis severity [25, 41, 84,85,86,87]. Similarly, the evidence was unclear regarding the impact of BMI on mucositis risk, with evidence indicating that low BMI [23, 88] and a BMI > 25 [51] were predictive of OM risk. In contrast, while the evidence may be limited, there are consistent reports detailing baseline performance status, with low KPS [34] and ECOG performance status [24] predictive of OM and GI-M risk. Smoking was also identified as a predictor of OM in HNC patients and transplant recipients [69, 88, 89]. In contrast, one study also reported that non-smokers had a 2.7-fold increase in the risk of grade II–IV OM [90].
Several biological/laboratory parameters (assessed prior to therapy) were linked with mucositis risk (summarized in Table 3), including low colonic thymidylate synthase (TS) expression [91], ALT (>16Ul) [81], low urea [81], neutropenia [22], lymphopenia [42], low platelet counts [42], low hemoglobin levels [42], renal dysfunction [12], and serum creatinine [48]; however, the evidence was sparse and limited to a small number of stand-alone studies.
Comorbidities, medication use and previous therapy
There are limited data on the impact of comorbidities on mucositis outcomes (summarized in Table 3), with only one study reporting inflammatory bowel disease (IBD) as a predictor of late-onset bowel injury caused by radiotherapy [28]. Similarly, the number of bowel movements at baseline was reported to predict GI-M risk [92]. Herpes simplex virus (HPV) was also reported to increase the risk of OM, with HPV+ patients reported to have a 6.8-fold increased risk of grade III–IV OM following chemoradiotherapy [90].
Few studies investigated the impact of medication use. One study reported multivitamin use prior to therapy as a predictor of lower OM scores [51]. Another study reported that antibiotic therapy for > 10 days in the month preceding treatment was a predictor of GI-M (neutropenic colitis) in children with ALL [22]. This was also supported in a cohort of adult patients undergoing conditioning chemotherapy for HSCT, with antibiotic use and GvHD prophylaxis with MTX predictive of OM [88].
Exposure to previous radiotherapy was reported as a predictor of OM in auto-SCT recipients [47], supporting reports of residual DNA damage as an independent predictor of OM risk [93]. Similarly, OM in previous chemotherapy treatment was reported to predict OM in HN patients undergoing adjunctive radiotherapy [85].
Tumor-related variables linked with mucositis risk
Both tumor location [42, 84], size [92], and stage [69] were reported as predictors of OM and GI-M, respectively, in stand-alone studies (summarized in Table 4). A single study also reported a diagnosis of NHL (versus other hematological malignancies) as a predictor of OM [47]. In children, germinal tumors (versus non-germinal) and hematological malignancies versus CNS tumors (specifically Hodgkin’s lymphoma) were reported to increase the risk of HSCT-induced OM [50, 94].
Areas requiring further investigation
While there were a large number of positive associations identified in our systematic review, we also identified negative studies or those that required further validation. For example, two studies reported no significant association between GI-M and MTHFR 677T mutations in patients undergoing chemoradiotherapy for rectal/anal cancer [95, 96]. Similarly, two large studies (N = 322, N = 113) failed to identify any significant association between MTX transporter mutations and TYMS SNPs for OM risk in children with ALL [19, 97] despite strong evidence supporting its role in adult cohorts [57]. While a systematic review of over 6500 patients supported a strong association between XRCC1 (rs25487) and OM and GI-M risk in HSCT recipients [68], another reported no significant association in XRCC1 mutations and OM risk in HNC patients [69]. Similarly, Lunberg et al. [98] and Goutham et al. [99] reported no significant effect of TGFB on OM despite a positive association identified for GI-M. Several studies, including a large prospective trial in 381 participants [100], also failed to identify any demographic influence on mucositis risk, including age, sex, BMI, and race [24, 48, 101,100,103].
Discussion
This paper is the first to collect and synthesize factors identified to contribute to mucositis risk and symptom severity. The purpose of which is to provide a foundation for exploration of promising research pathways to optimize risk stratification tools. The panel reviewed 197 publications, with 113 eligible for assessment. The study inclusion period of 2000–2019 was chosen to reflect the rapidly changing face of cancer treatment and toxicity management with the advent of new technologies and supportive agents. Despite this, mucositis continues to be a major challenge in the delivery of effective care. It is critical that research efforts and resources are dedicated to identifying patients at risk of severe mucositis to mitigate the constellation of clinical, psychosocial, and economic burdens with which it is associated, and potentially prevent long-term sequelae.
The most common factors investigated for their influence on mucositis were treatment-related factors, with dosimetric parameters evaluated across several oncology cohorts. Unsurprisingly, the strongest level of evidence was achieved for the volume of target organ (gastrointestinal tract and oral cavity, to lesser extent) exposed to irradiation [31, 32, 35, 36, 38,39,40, 100]. Similarly, increasing doses of melphalan [23, 24] and methotrexate [25], myeloablative conditioning therapy [88, 104, 105], and conventional RT (versus IMRT) [45] were all robustly demonstrated to increase an individual’s risk of mucositis. However, these parameters provide little benefit in terms of risk as they are largely unmodifiable, and the concept that increasing exposure of the alimentary mucosa to cytotoxic therapy increases mucositis risk reflects what is already well understood from a pathobiological perspective. It is also important to note that while female sex was consistently linked with increased mucositis risk, this may not be a direct effect and is likely mediated through altered dosimetric parameters. Of the studies included that identified female sex as a risk factor, dosimetric parameters were not considered, and in one case, females were reported to receive higher doses due to the way in which chemotherapeutic drugs are administered unadjusted for sex-dependent anthropometry [24].
Multiparameter risk prediction modeling was uncommon in the studies included for review, with most hypothesis-driven focusing on a single parameter in a strictly defined cohort, and a compartmentalized/siloed viewpoint when it comes to treatment outcomes. While this provides focus to many studies, single-candidate gene studies fail to address the complexities of mucosal injury and are limited by the overriding risk of false negatives, given the large number of participants required to obtain sufficient power [106]. Similarly, they are impacted with greater ease by confounding factors such as cohort heterogeneity, and gene-drug dose interactions need to be considered. As such, it is unsurprising to see such inconsistency in the literature. Pathway analyses and genome-wide association studies (GWAS) have the potential to offer greater insight both pathobiologically and predictively [4]; however, they are rare, presumably due to the high logistical demand of patient recruitment, biospecimen collection, and mucositis assessment, as well as cost. It was for this reason that we decided to include both association and risk prediction studies to provide a comprehensive overview of the current landscape regarding mucositis prediction/risk factors. So, while association studies provide a solid foundational knowledge of the factor that are likely to govern risk, they fail to provide tangible benefits in the provision of personalized supportive care. Future studies must therefore aim to prospectively develop multiplexed predictive strategies that are developed in training cohorts and validated in an independent manner. There has already been promising early work identifying clustered predictors and algorithms that predict high-risk patients and their response to intervention [107,106,109]. Moving forward, coordinated efforts, appropriate infrastructure, and consistent/comprehensive data collection across multiple locations with access to biobanks would drastically improve our ability to identify meaningful risk predictors, which will lay the foundation for personalized supportive care in which toxicity, efficacy, and cost can be prioritized based on the individual’s needs (Fig. 2).
Conceptual framework for implementing personalized cancer therapy that optimizes efficacy, toxicity, and economic outcomes based on comprehensive risk evaluation and individual needs of the patient. Multiplexed risk evaluation tools should provide risk stratification for all toxicities/side effects, providing the option to preferentially avoid toxicities considered most impactful for the individual while optimizing efficacy and reducing cost. Proactive and tailored supportive care can be directed to high-risk patients, while low-risk patients can avoid unnecessary interventions and be treated in an out-patient setting with confidence. Clear risk evaluation also provides patients with greater clarity regarding treatment outcomes which can be used to guide decision-making particularly in end-of-life care when quality of life may be prioritized over quantity
Critical to such efforts is the recognition of non-genomic risk predictors, which are likely to offer great predictive power when combined with treatment and genomic factors. This has been adopted in gerioncology, with a risk stratification tool developed using geriatric assessment variables; laboratory tests; and patient, tumor and treatment variables [110, 111]. While authors used a multiplexed approach, the variables included were primarily related to the performance status of the individual and treatment specifications, and thus could be improved by inclusion of modifiable biological and genetic parameters. An emerging area of personalized risk prediction, with relevance to geriatric oncology and the broader field, is the microbiota. While this has primarily focused on immunotherapy-induced colitis, evidence suggests pre-therapy microbial composition dictates mucositis caused by both chemotherapy and radiotherapy, with early microbial changes predictive of ulcerative OM development [112, 113]. While an area of interest is increasing due to the modifiable nature of the microbiota, this is a relatively new area of risk prediction, and thus, few studies were included in this systematic review. Given the profound influence the microbiota has on both local mucosal and immune function, the influence of the gastrointestinal and oral microbiota is likely to be significant. Future biobanking efforts to evaluate pre-therapy microbial predictors and clinically feasible methods of characterizing the microbiota (e.g., FAIMS) are therefore warranted.
With the development of multiparameter risk prediction models and appropriate recognition of systems medicine approaches to analysis, risk prediction serves to not only identify high-risk patients but also guide the development of next-generation interventions. These are likely to be unique to specific oncology cohorts and will almost certainly differ between adults and children. Disappointingly, in the 113 papers included in our analysis, only 10 addressed the topic of risk prediction in children. Developing risk prediction tools for children undergoing cancer therapy is of particular relevance as mucositis (and its associated manifestations) not only poses an acute impact on their quality of life but is also increasingly recognized for its influence on late effects in survivors of childhood cancer [114, 115].
Risk prediction in children is also critical as many factors recognized for their influence on mucositis development in adults are likely to be poorly extrapolated to children (e.g., comorbidities, smoking status, medication use). Similarly, microbial communities are well recognized to differ between adults and children [116], hindering efforts to predict pediatric mucositis based on microbial enterotypes identified in adults. A growing body of evidence also suggests that mucosal barrier injury and microbial injury are catalysts for other late-onset conditions (seen in both adults and children), including cardiac toxicity [117], graft versus host disease [13], fatigue [118], and neurocognitive impairment [119]. As such, a new frontier in risk prediction will be the interaction between acute and chronic toxicities, and the ability to mitigate chronic morbidity via the personalized prevention of acute injury.
In addition to its influence on late-onset toxicities, mucositis is well established to cluster with other significant toxicities, including fatigue, pain, and blood stream infection, suggesting common molecular pathways [118, 120,119,122]. It is therefore intuitive to suggest that symptom clusters also have common, or at least complementary, risk predictors. This has already been highlighted with the microbiota now recognized to predict multiple toxicities. Similarly, overlap exists in predictors of mucosal and hematological toxicities, reiterating the importance of targeting ubiquitous mechanisms of injury and inflammation with relevance to the broader field of regimen-related toxicities. These approaches will likely be based on multiplexed approaches, with weight given to certain parameters with particular relevance to organ-specific toxicities.
Despite a large number of positive studies included in this review, we also identified several negative studies that require further investigation or validation. While the number of negative studies is likely to be higher than those identified, biased by the pressure to produce positive findings, these studies also emphasize the challenges faced in conducting rigorous risk prediction studies. We identified a number of studies that had very low patient numbers, used non-validated assessment scales, and poorly defined patient populations that were undermined by high heterogeneity. Future studies must be appropriately powered and be guided by validated principles for mucositis assessment. The increased utilization and appropriate use of biobanking and shared data may help overcome the difficulties of recruitment to predictive supportive care studies and increase power. The involvement of bioinformatic and statistical experts to develop uniform methods of risk prediction analysis in the field of supportive care is also critical to success.
While this paper represents an important step in laying a foundation of knowledge regarding mucositis risk, it is not without its flaws. Firstly, we cast a large net to capture the breadth and variability of studies addressing mucositis risk prediction. This resulted in a large number of studies or varying relevance that were identified in our initial search strategy. With no uniform terminology relating to mucositis, particularly GI-M (i.e. diarrhea, gut toxicity, colitis), there is also the possibility that papers were not identified in our search strategy when they were not indexed using our defined terminology criteria. This highlights the need to standardize reporting of mucositis in clinical trials. Similarly, variable assessment scales and outcome measures prevented meta-analyses being performed and severely compromised our ability to draw parallels between studies.
In conclusion, mucositis risk prediction is becoming increasingly recognized for its role in the provision of individualized cancer care. Despite identification of distinct factors associated with mucositis risk, we remain limited in our ability to identify high-risk patients with current evidence undermined by inconsistencies, siloed approaches, and unpowered studies. For significant advances in our ability to provide personalized supportive care, large and coordinated research efforts with innovative data sharing and input by statistical support personnel are required. These approaches should focus on multiplexed approaches that address related toxicities to provide a comprehensive risk evaluation of an individual with specific weighting reflecting their unique oncological scenario and personal requirements.
Take home messages and call to action
-
1.
Personalizing supportive cancer care is critical and requires a solid foundational understanding of the factors that govern individual risk.
-
2.
Multiparameter risk prediction efforts with appropriate statistical approaches must be prioritized over restrictive, hypothesis-driven studies.
-
3.
Risk prediction strategies identified in adults are not easily translated to children, and as such, specific risk stratification methods must be identified for pediatric cohorts.
-
4.
Oral and gastrointestinal mucositis must be approached more holistically with risk prediction methods developed in parallel given the commonalities in pathobiology.
-
5.
Validated assessment scales and outcome measures should be prioritized and used consistently.
-
6.
Coordinated, international biobanking of patient biospecimens coupled with comprehensive data collection related to patient demographics, treatment specifications, and outcomes are desperately required to enable more robust risk prediction efforts with greater power and clinical influence.
-
7.
Future efforts in risk prediction should focus on multiplexed approaches that transcend a single toxicity and aim to predict clusters of related symptoms
References
Bode AM, Dong Z (2018) Recent advances in precision oncology research. NPJ Precis Oncol 2:11. https://doi.org/10.1038/s41698-018-0055-0
Bode AM, Dong Z (2017) Precision oncology-the future of personalized cancer medicine? NPJ Precis Oncol 1(1):2. https://doi.org/10.1038/s41698-017-0010-5
Bowen J, Al-Dasooqi N, Bossi P, Wardill H, Van Sebille Y, Al-Azri A, Bateman E, Correa ME, Raber-Durlacher J, Kandwal A, Mayo B, Nair RG, Stringer A, Ten Bohmer K, Thorpe D, Lalla RV, Sonis S, Cheng K, Elad S, Mucositis Study Group of the Multinational Association of Supportive Care in Cancer/International Society of Oral O (2019) The pathogenesis of mucositis: updated perspectives and emerging targets. Supportive care in cancer: official journal of the Multinational Association of Supportive Care in Cancer 27(10):4023–4033. https://doi.org/10.1007/s00520-019-04893-z
Bachour PC, Sonis ST (2018) Predicting mucositis risk associated with cytotoxic cancer treatment regimens: rationale, complexity, and challenges. Current opinion in supportive and palliative care 12(2):198–210. https://doi.org/10.1097/SPC.0000000000000339
Ariyawardana A, Cheng KKF, Kandwal A, Tilly V, Al-Azri AR, Galiti D, Chiang K, Vaddi A, Ranna V, Nicolatou-Galitis O, Lalla RV, Bossi P, Elad S, Mucositis Study Group of the Multinational Association of Supportive Care in Cancer/International Society for Oral O (2019) Systematic review of anti-inflammatory agents for the management of oral mucositis in cancer patients and clinical practice guidelines. Supportive care in cancer: official journal of the Multinational Association of Supportive Care in Cancer 27(10):3985–3995. https://doi.org/10.1007/s00520-019-04888-w
Correa MEP, Cheng KKF, Chiang K, Kandwal A, Loprinzi CL, Mori T, Potting C, Rouleau T, Toro JJ, Ranna V, Vaddi A, Peterson DE, Bossi P, Lalla RV, Elad S (2019) Systematic review of oral cryotherapy for the management of oral mucositis in cancer patients and clinical practice guidelines. Supportive care in cancer: official journal of the Multinational Association of Supportive Care in Cancer. 28:2449–2456. https://doi.org/10.1007/s00520-019-05217-x
Hong CHL, Gueiros LA, Fulton JS, Cheng KKF, Kandwal A, Galiti D, Fall-Dickson JM, Johansen J, Ameringer S, Kataoka T, Weikel D, Eilers J, Ranna V, Vaddi A, Lalla RV, Bossi P, Elad S, Mucositis Study Group of the Multinational Association of Supportive Care in Cancer/International Society for Oral O (2019) Systematic review of basic oral care for the management of oral mucositis in cancer patients and clinical practice guidelines. Supportive care in cancer: official journal of the Multinational Association of Supportive Care in Cancer 27(10):3949–3967. https://doi.org/10.1007/s00520-019-04848-4
Yarom N, Hovan A, Bossi P, Ariyawardana A, Jensen SB, Gobbo M, Saca-Hazboun H, Kandwal A, Majorana A, Ottaviani G, Pentenero M, Nasr NM, Rouleau T, Lucas AS, Treister NS, Zur E, Ranna V, Vaddi A, Cheng KKF, Barasch A, Lalla RV, Elad S, Mucositis Study Group of the Multinational Association of Supportive Care in Cancer/International Society of Oral O (2019) Systematic review of natural and miscellaneous agents for the management of oral mucositis in cancer patients and clinical practice guidelines-part 1: vitamins, minerals, and nutritional supplements. Supportive care in cancer: official journal of the Multinational Association of Supportive Care in Cancer 27(10):3997–4010. https://doi.org/10.1007/s00520-019-04887-x
Zadik Y, Arany PR, Fregnani ER, Bossi P, Antunes HS, Bensadoun RJ, Gueiros LA, Majorana A, Nair RG, Ranna V, Tissing WJE, Vaddi A, Lubart R, Migliorati CA, Lalla RV, Cheng KKF, Elad S, Mucositis Study Group of the Multinational Association of Supportive Care in Cancer/International Society of Oral O (2019) Systematic review of photobiomodulation for the management of oral mucositis in cancer patients and clinical practice guidelines. Supportive care in cancer: official journal of the Multinational Association of Supportive Care in Cancer 27(10):3969–3983. https://doi.org/10.1007/s00520-019-04890-2
Bowen JM, Gibson RJ, Coller JK, Blijlevens N, Bossi P, Al-Dasooqi N, Bateman EH, Chiang K, de Mooij C, Mayo B, Stringer AM, Tissing W, Wardill HR, van Sebille YZA, Ranna V, Vaddi A, Keefe DM, Lalla RV, Cheng KKF, Elad S, Mucositis Study Group of the Multinational Association of Supportive Care in Cancer/International Society of Oral O (2019) Systematic review of agents for the management of cancer treatment-related gastrointestinal mucositis and clinical practice guidelines. Supportive care in cancer: official journal of the Multinational Association of Supportive Care in Cancer 27(10):4011–4022. https://doi.org/10.1007/s00520-019-04892-0
Rashidi A, Shanley R, Holtan SG, MacMillan ML, Blazar BR, Khoruts A, Weisdorf DJ (2018) Pretransplant serum citrulline predicts acute graft-versus-host disease. Biology of blood and marrow transplantation: journal of the American Society for Blood and Marrow Transplantation 24(11):2190–2196. https://doi.org/10.1016/j.bbmt.2018.06.036
Mizuno H, Miyai H, Yokoi A, Kobayashi T, Inabu C, Maruyama T, Ekuni D, Mizukawa N, Kariya S, Nishizaki K, Kimata Y, Morita M (2019) Relationship between renal dysfunction and oral mucositis in patients undergoing concurrent chemoradiotherapy for pharyngeal cancer: a retrospective cohort study. In vivo (Athens, Greece) 33 (1):183-189. Doi:https://doi.org/10.21873/invivo.11457
Jenq RR, Taur Y, Devlin SM, Ponce DM, Goldberg JD, Ahr KF, Littmann ER, Ling L, Gobourne AC, Miller LC, Docampo MD, Peled JU, Arpaia N, Cross JR, Peets TK, Lumish MA, Shono Y, Dudakov JA, Poeck H, Hanash AM, Barker JN, Perales MA, Giralt SA, Pamer EG, van den Brink MR (2015) Intestinal Blautia is associated with reduced death from graft-versus-host disease. Biology of blood and marrow transplantation: journal of the American Society for Blood and Marrow Transplantation 21(8):1373–1383. https://doi.org/10.1016/j.bbmt.2015.04.016
Ippolito E, Guido A, Macchia G, Deodato F, Giaccherini L, Farioli A, Arcelli A, Cuicchi D, Frazzoni L, Cilla S, Buwenge M, Mantini G, Alitto AR, Nuzzo M, Valentini V, Ingrosso M, Morganti AG, Fuccio L (2017) Predictive factors of late-onset rectal mucosal changes after radiotherapy of prostate cancer. In vivo (Athens, Greece) 31 (5):961-966. Doi:https://doi.org/10.21873/invivo.11154
Peach MS, Showalter TN, Ohri N (2015) Systematic review of the relationship between acute and late gastrointestinal toxicity after radiotherapy for prostate cancer. Prostate Cancer 2015:624736. doi:https://doi.org/10.1155/2015/624736
Shaw PJ, Nath CE, Lazarus HM (2014) Not too little, not too much-just right! (Better ways to give high dose melphalan). Bone Marrow Transplant 49(12):1457–1465. https://doi.org/10.1038/bmt.2014.186
McCullough RW (2017) US oncology-wide incidence, duration, costs and deaths from chemoradiation mucositis and antimucositis therapy benefits. Future oncology (London, England) 13(30):2823–2852. https://doi.org/10.2217/fon-2017-0418
Heil SG (2019) Genetics of high-dose methotrexate-induced oral mucositis: current perspectives. Pharmacogenomics 20(9):621–623. https://doi.org/10.2217/pgs-2019-0062
Oosterom N, Berrevoets M, den Hoed MAH, Zolk O, Hoerning S, Pluijm SMF, Pieters R, de Jonge R, Tissing WJE, van den Heuvel-Eibrink MM, Heil SG (2018) The role of genetic polymorphisms in the thymidylate synthase (TYMS) gene in methotrexate-induced oral mucositis in children with acute lymphoblastic leukemia. Pharmacogenet Genomics 28(10):223–229. https://doi.org/10.1097/fpc.0000000000000352
van Erp NP, Eechoute K, van der Veldt AA, Haanen JB, Reyners AK, Mathijssen RH, Boven E, van der Straaten T, Baak-Pablo RF, Wessels JA, Guchelaar HJ, Gelderblom H (2009) Pharmacogenetic pathway analysis for determination of sunitinib-induced toxicity. Journal of clinical oncology: official journal of the American Society of Clinical Oncology 27(26):4406–4412. https://doi.org/10.1200/jco.2008.21.7679
Chen RC, Mamon HJ, Ancukiewicz M, Killoran JH, Crowley EM, Blaszkowsky LS, Wo JY, Ryan DP, Hong TS (2012) Dose--volume effects on patient-reported acute gastrointestinal symptoms during chemoradiation therapy for rectal cancer. Int J Radiat Oncol Biol Phys 83(4):e513–e517. https://doi.org/10.1016/j.ijrobp.2012.01.013
Reyna-Figueroa J, Garcia-Beristain JC, Galindo-Delgado P, Limon-Rojas AE, Madrid-Marina V (2015) Antibiotic use before chemotherapy: a risk factor for developing neutropenic colitis in children with leukemia. J Pediatr Hematol Oncol 37(2):121–127. https://doi.org/10.1097/mph.0000000000000227
Vokurka S, Steinerova K, Karas M, Koza V (2009) Characteristics and risk factors of oral mucositis after allogeneic stem cell transplantation with FLU/MEL conditioning regimen in context with BU/CY2. Bone Marrow Transplant 44(9):601–605. https://doi.org/10.1038/bmt.2009.66
Blijlevens N, Schwenkglenks M, Bacon P, D’Addio A, Einsele H, Maertens J, Niederwieser D, Rabitsch W, Roosaar A, Ruutu T, Schouten H, Stone R, Vokurka S, Quinn B, McCann S (2008) Prospective oral mucositis audit: oral mucositis in patients receiving high-dose melphalan or BEAM conditioning chemotherapy--European Blood and Marrow Transplantation Mucositis Advisory Group. Journal of clinical oncology : official journal of the American Society of Clinical Oncology 26(9):1519–1525. https://doi.org/10.1200/jco.2007.13.6028
Kashiwazaki H, Matsushita T, Sugita J, Shigematsu A, Kasashi K, Yamazaki Y, Kanehira T, Kondo T, Endo T, Tanaka J, Hashino S, Nishio M, Imamura M, Kitagawa Y, Inoue N (2012) A comparison of oral mucositis in allogeneic hematopoietic stem cell transplantation between conventional and reduced-intensity regimens. Support Care Cancer 20(5):933–939. https://doi.org/10.1007/s00520-011-1164-2
Bhide SA, Gulliford S, Schick U, Miah A, Zaidi S, Newbold K, Nutting CM, Harrington KJ (2012) Dose-response analysis of acute oral mucositis and pharyngeal dysphagia in patients receiving induction chemotherapy followed by concomitant chemo-IMRT for head and neck cancer. Radiother Oncol 103(1):88–91. https://doi.org/10.1016/j.radonc.2011.12.027
Werbrouck J, De Ruyck K, Duprez F, Veldeman L, Claes K, Van Eijkeren M, Boterberg T, Willems P, Vral A, De Neve W, Thierens H (2009) Acute normal tissue reactions in head-and-neck cancer patients treated with IMRT: influence of dose and association with genetic polymorphisms in DNA DSB repair genes. Int J Radiat Oncol Biol Phys 73(4):1187–1195. https://doi.org/10.1016/j.ijrobp.2008.08.073
Barnett GC, De Meerleer G, Gulliford SL, Sydes MR, Elliott RM, Dearnaley DP (2011) The impact of clinical factors on the development of late radiation toxicity: results from the Medical Research Council RT01 trial (ISRCTN47772397). Clinical oncology (Royal College of Radiologists (Great Britain)) 23(9):613–624. https://doi.org/10.1016/j.clon.2011.03.001
Orlandi E, Iacovelli NA, Rancati T, Cicchetti A, Bossi P, Pignoli E, Bergamini C, Licitra L, Fallai C, Valdagni R, Cavallo A (2018) Multivariable model for predicting acute oral mucositis during combined IMRT and chemotherapy for locally advanced nasopharyngeal cancer patients. Oral Oncol 86:266–272. https://doi.org/10.1016/j.oraloncology.2018.10.006
Mazeron R, Fokdal LU, Kirchheiner K, Georg P, Jastaniyah N, Segedin B, Mahantshetty U, Hoskin P, Jurgenliemk-Schulz I, Kirisits C, Lindegaard JC, Dorr W, Haie-Meder C, Tanderup K, Potter R (2016) Dose-volume effect relationships for late rectal morbidity in patients treated with chemoradiation and MRI-guided adaptive brachytherapy for locally advanced cervical cancer: results from the prospective multicenter EMBRACE study. Radiother Oncol 120(3):412–419. https://doi.org/10.1016/j.radonc.2016.06.006
Simpson DR, Song WY, Moiseenko V, Rose BS, Yashar CM, Mundt AJ, Mell LK (2012) Normal tissue complication probability analysis of acute gastrointestinal toxicity in cervical cancer patients undergoing intensity modulated radiation therapy and concurrent cisplatin. Int J Radiat Oncol Biol Phys 83(1):e81–e86. https://doi.org/10.1016/j.ijrobp.2011.12.012
Nakamura A, Shibuya K, Matsuo Y, Nakamura M, Shiinoki T, Mizowaki T, Hiraoka M (2012) Analysis of dosimetric parameters associated with acute gastrointestinal toxicity and upper gastrointestinal bleeding in locally advanced pancreatic cancer patients treated with gemcitabine-based concurrent chemoradiotherapy. Int J Radiat Oncol Biol Phys 84(2):369–375. https://doi.org/10.1016/j.ijrobp.2011.12.026
Arcadipane F, Olimpio E, Ragona R, Martini S, Iorio GC, Gallio E, Di Muzio J, Franco P, Ricardi U (2019) Clinical and dosimetric predictors of oral mucositis in the treatment of head and neck cancer. Radiother Oncol 132:71–71. https://doi.org/10.1016/s0167-8140(19)30300-7
Cattaneo GM, Passoni P, Longobardi B, Slim N, Reni M, Cereda S, di Muzio N, Calandrino R (2013) Dosimetric and clinical predictors of toxicity following combined chemotherapy and moderately hypofractionated rotational radiotherapy of locally advanced pancreatic adenocarcinoma. Radiother Oncol 108(1):66–71. https://doi.org/10.1016/j.radonc.2013.05.011
Sanguineti G, Endres EJ, Sormani MP, Parker BC (2009) Dosimetric predictors of diarrhea during radiotherapy for prostate cancer. Strahlentherapie und Onkologie: Organ der Deutschen Rontgengesellschaft [et al] 185(6):390–396. https://doi.org/10.1007/s00066-009-1953-4
Han K, Cummings BJ, Lindsay P, Skliarenko J, Craig T, Le LW, Brierley J, Wong R, Dinniwell R, Bayley AJ, Dawson LA, Ringash J, Krzyzanowska MK, Moore MJ, Chen EX, Easson AM, Kassam Z, Cho C, Kim J (2014) Prospective evaluation of acute toxicity and quality of life after IMRT and concurrent chemotherapy for anal canal and perianal cancer. Int J Radiat Oncol Biol Phys 90(3):587–594. https://doi.org/10.1016/j.ijrobp.2014.06.061
Gunnlaugsson A, Nilsson P, Kjellen E, Johnsson A (2009) The effect on the small bowel of 5-FU and oxaliplatin in combination with radiation using a microcolony survival assay. Radiation oncology (London, England) 4:61. https://doi.org/10.1186/1748-717X-4-61
Julie DA, Oh JH, Apte AP, Deasy JO, Tom A, Wu AJ, Goodman KA (2016) Predictors of acute toxicities during definitive chemoradiation using intensity-modulated radiotherapy for anal squamous cell carcinoma. Acta oncologica (Stockholm, Sweden) 55(2):208–216. https://doi.org/10.3109/0284186x.2015.1043396
Tho LM, Glegg M, Paterson J, Yap C, MacLeod A, McCabe M, McDonald AC (2006) Acute small bowel toxicity and preoperative chemoradiotherapy for rectal cancer: investigating dose-volume relationships and role for inverse planning. Int J Radiat Oncol Biol Phys 66(2):505–513. https://doi.org/10.1016/j.ijrobp.2006.05.005
Olsen JR, Moughan J, Myerson R, Abitbol A, Doncals DE, Johnson D, Schefter TE, Chen Y, Fisher B, Michalski J, Narayan S, Chang A, Crane CH, Kachnic L (2017) Predictors of radiation therapy-related gastrointestinal toxicity from anal cancer dose-painted intensity modulated radiation therapy: secondary analysis of NRG oncology RTOG 0529. Int J Radiat Oncol Biol Phys 98(2):400–408. https://doi.org/10.1016/j.ijrobp.2017.02.005
Yang TJ, Oh JH, Son CH, Apte A, Deasy JO, Wu A, Goodman KA (2013) Predictors of acute gastrointestinal toxicity during pelvic chemoradiotherapy in patients with rectal cancer. Gastrointestinal cancer research : GCR 6(5–6):129–136
Nishii M, Soutome S, Kawakita A, Yutori H, Iwata E, Akashi M, Hasegawa T, Kojima Y, Funahara M, Umeda M, Komori T (2019) Factors associated with severe oral mucositis and candidiasis in patients undergoing radiotherapy for oral and oropharyngeal carcinomas: a retrospective multicenter study of 326 patients. Supportive care in cancer: official journal of the Multinational Association of Supportive Care in Cancer 28:1069–1075. https://doi.org/10.1007/s00520-019-04885-z
Hildebrandt MA, Komaki R, Liao Z, Gu J, Chang JY, Ye Y, Lu C, Stewart DJ, Minna JD, Roth JA, Lippman SM, Cox JD, Hong WK, Spitz MR, Wu X (2010) Genetic variants in inflammation-related genes are associated with radiation-induced toxicity following treatment for non-small cell lung cancer. PLoS One 5(8):e12402. https://doi.org/10.1371/journal.pone.0012402
Huang CM, Huang MY, Tsai HL, Huang CW, Ma CJ, Lin CH, Huang CJ, Wang JY (2017) A retrospective comparison of outcome and toxicity of preoperative image-guided intensity-modulated radiotherapy versus conventional pelvic radiotherapy for locally advanced rectal carcinoma. J Radiat Res 58(2):247–259. https://doi.org/10.1093/jrr/rrw098
Prasad S, Cambridge L, Huguet F, Chou JF, Zhang Z, Wu AJ, O’Reilly EM, Allen PJ, Goodman KA (2016) Intensity modulated radiation therapy reduces gastrointestinal toxicity in locally advanced pancreas cancer. Practical radiation oncology 6(2):78–85. https://doi.org/10.1016/j.prro.2015.09.006
Bonner J, Giralt J, Harari P, Spencer S, Schulten J, Hossain A, Chang SC, Chin S, Baselga J (2016) Cetuximab and radiotherapy in laryngeal preservation for cancers of the larynx and hypopharynx: a secondary analysis of a randomized clinical trial. JAMA Otolaryngol Head Neck Surg 142(9):842–849. https://doi.org/10.1001/jamaoto.2016.1228
Bolwell BJ, Kalaycio M, Sobecks R, Andresen S, Kuczkowski E, Bernhard L, Lomax R, Kohuth J, Mendiola S, Rybicki L, Pohlman B (2002) A multivariable analysis of factors influencing mucositis after autologous progenitor cell transplantation. Bone Marrow Transplant 30(9):587–591. https://doi.org/10.1038/sj.bmt.1703694
Grazziutti ML, Dong L, Miceli MH, Krishna SG, Kiwan E, Syed N, Fassas A, van Rhee F, Klaus H, Barlogie B, Anaissie EJ (2006) Oral mucositis in myeloma patients undergoing melphalan-based autologous stem cell transplantation: incidence, risk factors and a severity predictive model. Bone Marrow Transplant 38(7):501–506. https://doi.org/10.1038/sj.bmt.1705471
Wardley AM, Jayson GC, Swindell R, Morgenstern GR, Chang J, Bloor R, Fraser CJ, Scarffe JH (2000) Prospective evaluation of oral mucositis in patients receiving myeloablative conditioning regimens and haemopoietic progenitor rescue. Br J Haematol 110(2):292–299. https://doi.org/10.1046/j.1365-2141.2000.02202.x
Fadda G, Campus G, Luglie P (2006) Risk factors for oral mucositis in paediatric oncology patients receiving alkylant chemotherapy. BMC oral health 6:13. https://doi.org/10.1186/1472-6831-6-13
Robien K, Schubert MM, Bruemmer B, Lloid ME, Potter JD, Ulrich CM (2004) Predictors of oral mucositis in patients receiving hematopoietic cell transplants for chronic myelogenous leukemia. J Clin Oncol 22(7):1268–1275. https://doi.org/10.1200/jco.2004.05.147
Goyal M, Shukla P, Gupta D, Bisht SS, Dhawan A, Gupta S, Pant MC, Verma NS (2009) Oral mucositis in morning vs. evening irradiated patients: a randomised prospective study. Int J Radiat Biol 85(6):504–509. https://doi.org/10.1080/09553000902883802
Bjarnason GA, Mackenzie RG, Nabid A, Hodson ID, El-Sayed S, Grimard L, Brundage M, Wright J, Hay J, Ganguly P, Leong C, Wilson J, Jordan RC, Walker M, Tu D, Parulekar W (2009) Comparison of toxicity associated with early morning versus late afternoon radiotherapy in patients with head-and-neck cancer: a prospective randomized trial of the National Cancer Institute of Canada Clinical Trials Group (HN3). International journal of radiation oncology, biology, physics 73(1):166–172. https://doi.org/10.1016/j.ijrobp.2008.07.009
Shukla P, Gupta D, Bisht SS, Pant MC, Bhatt ML, Gupta R, Srivastava K, Gupta S, Dhawan A, Mishra D, Negi MP (2010) Circadian variation in radiation-induced intestinal mucositis in patients with cervical carcinoma. Cancer 116(8):2031–2035. https://doi.org/10.1002/cncr.24867
Reyes-Gibby CC, Melkonian SC, Wang J, Yu RK, Shelburne SA, Lu C, Gunn GB, Chambers MS, Hanna EY, Yeung S-CJ, Shete S (2017) Identifying novel genes and biological processes relevant to the development of cancer therapy-induced mucositis: an informative gene network analysis. Figshare
Campbell JM, Bateman E, Peters M, Bowen JM, Keefe DM, Stephenson MD (2016) Fluoropyrimidine and platinum toxicity pharmacogenetics: an umbrella review of systematic reviews and meta-analyses. Pharmacogenomics 17(4):435–451. https://doi.org/10.2217/pgs.15.180
Campbell JM, Bateman E, Stephenson MD, Bowen JM, Keefe DM, Peters MD (2016) Methotrexate-induced toxicity pharmacogenetics: an umbrella review of systematic reviews and meta-analyses. Cancer Chemother Pharmacol 78(1):27–39. https://doi.org/10.1007/s00280-016-3043-5
Campbell JM, Stephenson MD, Bateman E, Peters MD, Keefe DM, Bowen JM (2017) Irinotecan-induced toxicity pharmacogenetics: an umbrella review of systematic reviews and meta-analyses. Pharmacogenomics J 17(1):21–28. https://doi.org/10.1038/tpj.2016.58
Ulrich CM, Yasui Y, Storb R, Schubert MM, Wagner JL, Bigler J, Ariail KS, Keener CL, Li S, Liu H, Farin FM, Potter JD (2001) Pharmacogenetics of methotrexate: toxicity among marrow transplantation patients varies with the methylenetetrahydrofolate reductase C677T polymorphism. Blood 98(1):231–234
Tantawy AA, El-Bostany EA, Adly AA, Abou El Asrar M, El-Ghouroury EA, Abdulghaffar EE (2010) Methylene tetrahydrofolate reductase gene polymorphism in Egyptian children with acute lymphoblastic leukemia. Blood coagulation & fibrinolysis: an international journal in haemostasis and thrombosis 21(1):28–34. https://doi.org/10.1097/MBC.0b013e32833135e9
Venkatesh GH, Manjunath VB, Mumbrekar KD, Negi H, Fernandes DJ, Sharan K, Banerjee S, Bola Sadashiva SR (2014) Polymorphisms in radio-responsive genes and its association with acute toxicity among head and neck cancer patients. PLoS One 9(3):e89079. https://doi.org/10.1371/journal.pone.0089079
Mlak R, Powrozek T, Brzozowska A, Homa-Mlak I, Mazurek M, Malecka-Massalska T (2018) RRM1 gene expression evaluated in the liquid biopsy (blood cfRNA) as a non-invasive, predictive factor for radiotherapy-induced oral mucositis and potential prognostic biomarker in head and neck cancer patients. Cancer biomarkers: section A of Disease markers 22(4):657–667. https://doi.org/10.3233/cbm-171082
Borchiellini D, Etienne-Grimaldi MC, Bensadoun RJ, Benezery K, Dassonville O, Poissonnet G, Llorca L, Ebran N, Formento P, Chateau Y, Thariat J, Milano G (2017) Candidate apoptotic and DNA repair gene approach confirms involvement of ERCC1, ERCC5, TP53 and MDM2 in radiation-induced toxicity in head and neck cancer. Oral Oncol 67:70–76. https://doi.org/10.1016/j.oraloncology.2017.02.003
Smith JJ, Wasserman I, Milgrom SA, Chow OS, Chen CT, Patil S, Goodman KA, Garcia-Aguilar J (2017) Single nucleotide polymorphism TGFbeta1 R25P correlates with acute toxicity during neoadjuvant chemoradiotherapy in rectal cancer patients. Int J Radiat Oncol Biol Phys 97(5):924–930. https://doi.org/10.1016/j.ijrobp.2016.12.015
Osti MF, Nicosia L, Agolli L, Gentile G, Falco T, Bracci S, Di Nardo F, Minniti G, De Sanctis V, Valeriani M, Maglio M, Borro M, Simmaco M, Enrici RM (2017) Potential role of single nucleotide polymorphisms of XRCC1, XRCC3, and RAD51 in predicting acute toxicity in rectal cancer patients treated with preoperative radiochemotherapy. Am J Clin Oncol 40(6):535–542. https://doi.org/10.1097/coc.0000000000000182
Bohanes P, Rankin CJ, Blanke CD, Winder T, Ulrich CM, Smalley SR, Rich TA, Martensen JA, Benson AB 3rd, Mayer RJ, Cripps CM, Danenberg K, Makar KW, Zhang W, Benedetti JK, Lenz HJ (2015) Pharmacogenetic analysis of INT 0144 trial: association of polymorphisms with survival and toxicity in rectal cancer patients treated with 5-FU and radiation. Clin Cancer Res 21(7):1583–1590. https://doi.org/10.1158/1078-0432.Ccr-14-0857
Soares S, Nogueira A, Coelho A, Assis J, Pereira D, Bravo I, Medeiros R (2018) Relationship between clinical toxicities and ERCC1 rs3212986 and XRCC3 rs861539 polymorphisms in cervical cancer patients. Int J Biol Markers 33(1):116–123. https://doi.org/10.5301/ijbm.5000279
Zhao J, Zhi Z, Zhang M, Li Q, Li J, Wang X, Ma C (2018) Predictive value of single nucleotide polymorphisms in XRCC1 for radiation-induced normal tissue toxicity. OncoTargets and therapy 11:3901–3918. https://doi.org/10.2147/ott.S156175
Chen H, Wu M, Li G, Hua L, Chen S, Huang H (2017) Association between XRCC1 single-nucleotide polymorphism and acute radiation reaction in patients with nasopharyngeal carcinoma: a cohort study. Medicine 96(44):e8202. https://doi.org/10.1097/md.0000000000008202
Nanda SS, Gandhi AK, Rastogi M, Khurana R, Hadi R, Sahni K, Mishra SP, Srivastava AK, Bhatt MLB, Parmar D (2018) Evaluation of XRCC1 gene polymorphism as a biomarker in head and neck cancer patients undergoing chemoradiation therapy. Int J Radiat Oncol Biol Phys 101(3):593–601. https://doi.org/10.1016/j.ijrobp.2018.03.039
Coller JK, White IA, Logan RM, Tuke J, Richards AM, Mead KR, Karapetis CS, Bowen JM (2015) Predictive model for risk of severe gastrointestinal toxicity following chemotherapy using patient immune genetics and type of cancer: a pilot study. Supportive care in cancer: official journal of the Multinational Association of Supportive Care in Cancer 23(5):1233–1236. https://doi.org/10.1007/s00520-014-2481-z
Sakamoto K, Oka M, Yoshino S, Hazama S, Abe T, Okayama N, Hinoda Y (2006) Relation between cytokine promoter gene polymorphism and toxicity of 5-fluorouracil plus cisplatin chemotherapy. Oncol Rep 16(2):381–387
Bowen JM, White I, Smith L, Tsykin A, Kristaly K, Thompson SK, Karapetis CS, Tan H, Game PA, Irvine T, Hussey DJ, Watson DI, Keefe DM (2015) Pre-therapy mRNA expression of TNF is associated with regimen-related gastrointestinal toxicity in patients with esophageal cancer: a pilot study. Support Care Cancer 23(11):3165–3172. https://doi.org/10.1007/s00520-015-2696-7
Brzozowska A, Powrozek T, Homa-Mlak I, Mlak R, Ciesielka M, Golebiowski P, Malecka-Massalska T (2018) Polymorphism of promoter region of TNFRSF1A gene (-610 T > G) as a novel predictive factor for radiotherapy induced oral mucositis in HNC patients. Pathology oncology research : POR 24(1):135–143. https://doi.org/10.1007/s12253-017-0227-1
Brzozowska A, Mlak R, Homa-Mlak I, Golebiowski P, Mazurek M, Ciesielka M, Malecka-Massalska T (2018) Polymorphism of regulatory region of APEH gene (c.-521G>C, rs4855883) as a relevant predictive factor for radiotherapy induced oral mucositis and overall survival in head neck cancer patients. Oncotarget 9(51):29644–29653. https://doi.org/10.18632/oncotarget.25662
Brzozowska A, Homa-Mlak I, Mlak R, Golebiowski P, Mazurek M, Ciesielka M, Malecka-Massalska T (2018) Polymorphism of regulatory region of GHRL gene (-2531C>T) as a promising predictive factor for radiotherapy-induced oral mucositis in patients with head neck cancer. Head & neck 40(8):1799–1811. https://doi.org/10.1002/hed.25154
Ma WL, Liu R, Huang LH, Zou C, Huang J, Wang J, Chen SJ, Meng XG, Yang JK, Li H, Yang GP, Guo CX (2017) Impact of polymorphisms in angiogenesis-related genes on clinical outcomes of radiotherapy in patients with nasopharyngeal carcinoma. Clin Exp Pharmacol Physiol 44(5):539–548. https://doi.org/10.1111/1440-1681.12738
Le Z, Niu X, Chen Y, Ou X, Zhao G, Liu Q, Tu W, Hu C, Kong L, Liu Y (2017) Predictive single nucleotide polymorphism markers for acute oral mucositis in patients with nasopharyngeal carcinoma treated with radiotherapy. Oncotarget 8(38):63026–63037. https://doi.org/10.18632/oncotarget.18450
Gutierrez-Camino A, Oosterom N, den Hoed MAH, Lopez-Lopez E, Martin-Guerrero I, Pluijm SMF, Pieters R, de Jonge R, Tissing WJE, Heil SG, Garcia-Orad A, van den Heuvel-Eibrink MM (2017) The miR-1206 microRNA variant is associated with methotrexate-induced oral mucositis in pediatric acute lymphoblastic leukemia. Pharmacogenet Genomics 27(8):303–306. https://doi.org/10.1097/fpc.0000000000000291
Vokurka S, Bystricka E, Koza V, Scudlova J, Pavlicova V, Valentova D, Visokaiova M, Misaniova L (2006) Higher incidence of chemotherapy induced oral mucositis in females: a supplement of multivariate analysis to a randomized multicentre study. Supportive care in cancer: official journal of the Multinational Association of Supportive Care in Cancer 14(9):974–976. https://doi.org/10.1007/s00520-006-0031-z
Tebbutt NC, Norman AR, Cunningham D, Allen M, Chau I, Oates J, Hill M (2003) Analysis of the time course and prognostic factors determining toxicity due to infused fluorouracil. Br J Cancer 88(10):1510–1515. https://doi.org/10.1038/sj.bjc.6600917
Schwab M, Zanger UM, Marx C, Schaeffeler E, Klein K, Dippon J, Kerb R, Blievernicht J, Fischer J, Hofmann U, Bokemeyer C, Eichelbaum M, German FUTSG (2008) Role of genetic and nongenetic factors for fluorouracil treatment-related severe toxicity: a prospective clinical trial by the German 5-FU Toxicity Study Group. Journal of clinical oncology: official journal of the American Society of Clinical Oncology 26(13):2131–2138. https://doi.org/10.1200/JCO.2006.10.4182
Valeh M, Kargar M, Mansouri A, Kamranzadeh H, Gholami K, Heidari K, Hajibabaei M (2018) Factors affecting the incidence and severity of oral mucositis following hematopoietic stem cell transplantation. International journal of hematology-oncology and stem cell research 12(2):142–152
van den Broek GB, Balm AJ, van den Brekel MW, Hauptmann M, Schornagel JH, Rasch CR (2006) Relationship between clinical factors and the incidence of toxicity after intra-arterial chemoradiation for head and neck cancer. Radiother Oncol 81(2):143–150. https://doi.org/10.1016/j.radonc.2006.09.002
Porock D, Nikoletti S, Cameron F (2004) The relationship between factors that impair wound healing and the severity of acute radiation skin and mucosal toxicities in head and neck cancer. Cancer Nurs 27(1):71–78
Cakmak S, Nural N (2019) Incidence of and risk factors for development of oral mucositis in outpatients undergoing cancer chemotherapy. Int J Nurs Pract 25(1):e12710. https://doi.org/10.1111/ijn.12710
Carreon-Burciaga RG, Castaneda-Castaneira E, Gonzalez-Gonzalez R, Molina-Frechero N, Gaona E, Bologna-Molina R (2018) Severity of oral mucositis in children following chemotherapy and radiotherapy and its implications at a single oncology centre in Durango State, Mexico. Int J Pediatr 2018:3252765–3252765. https://doi.org/10.1155/2018/3252765
Shouval R, Kouniavski E, Fein J, Danylesko I, Shem-Tov N, Geva M, Yerushalmi R, Shimoni A, Nagler A (2019) Risk factors and implications of oral mucositis in recipients of allogeneic hematopoietic stem cell transplantation. Eur J Haematol 103:402–409. https://doi.org/10.1111/ejh.13299
Tao Z, Gao J, Qian L, Huang Y, Zhou Y, Yang L, He J, Yang J, Wang R, Zhang Y (2017) Factors associated with acute oral mucosal reaction induced by radiotherapy in head and neck squamous cell carcinoma: a retrospective single-center experience. Medicine 96(50):e8446. https://doi.org/10.1097/md.0000000000008446
Vatca M, Lucas JT Jr, Laudadio J, D’Agostino RB, Waltonen JD, Sullivan CA, Rouchard-Plasser R, Matsangou M, Browne JD, Greven KM, Porosnicu M (2014) Retrospective analysis of the impact of HPV status and smoking on mucositis in patients with oropharyngeal squamous cell carcinoma treated with concurrent chemotherapy and radiotherapy. Oral Oncol 50(9):869–876. https://doi.org/10.1016/j.oraloncology.2014.06.010
Santini D, Vincenzi B, Perrone G, Rabitti C, Borzomati D, Caricato M, La Cesa A, Grilli C, Verzi A, Coppola R, Tonini G (2004) Thymidylate synthase expression in normal colonic mucosa: a predictive marker of toxicity in colorectal cancer patients receiving 5-fluorouracil-based adjuvant chemotherapy. Oncology 67(2):135–142. https://doi.org/10.1159/000081000
Xu B, Guo Y, Chen Y, Lu H, Tang T, Yue Z, Guan G, Chi P, Lin C (2015) Is the irradiated small bowel volume still a predictor for acute lower gastrointestinal toxicity during preoperative concurrent chemo-radiotherapy for rectal cancer when using intensity-modulated radiation therapy? Radiat Oncol 10:257. https://doi.org/10.1186/s13014-015-0566-6
Goutham HV, Mumbrekar KD, Vadhiraja BM, Fernandes DJ, Sharan K, Kanive Parashiva G, Kapaettu S, Bola Sadashiva SR (2012) DNA double-strand break analysis by gamma-H2AX foci: a useful method for determining the overreactors to radiation-induced acute reactions among head-and-neck cancer patients. Int J Radiat Oncol Biol Phys 84(5):e607–e612. https://doi.org/10.1016/j.ijrobp.2012.06.041
Allen G, Logan R, Revesz T, Keefe D, Gue S (2018) The prevalence and investigation of risk factors of oral mucositis in a pediatric oncology inpatient population; a prospective study. J Pediatr Hematol Oncol 40(1):15–21. https://doi.org/10.1097/mph.0000000000000970
Ruiz-Arguelles GJ, Coconi-Linares LN, Garces-Eisele J, Reyes-Nunez V (2007) Methotrexate-induced mucositis in acute leukemia patients is not associated with the MTHFR 677T allele in Mexico. Hematology (Amsterdam, Netherlands) 12(5):387–391. https://doi.org/10.1080/10245330701448479
Agostini M, Pasetto LM, Pucciarelli S, Terrazzino S, Ambrosi A, Bedin C, Galdi F, Friso ML, Mescoli C, Urso E, Leon A, Lise M, Nitti D (2008) Glutathione S-transferase P1 Ile105Val polymorphism is associated with haematological toxicity in elderly rectal cancer patients receiving preoperative chemoradiotherapy. Drugs Aging 25(6):531–539. https://doi.org/10.2165/00002512-200825060-00006
Liu SG, Gao C, Zhang RD, Zhao XX, Cui L, Li WJ, Chen ZP, Yue ZX, Zhang YY, Wu MY, Wang JX, Li ZG, Zheng HY (2017) Polymorphisms in methotrexate transporters and their relationship to plasma methotrexate levels, toxicity of high-dose methotrexate, and outcome of pediatric acute lymphoblastic leukemia. Oncotarget 8(23):37761–37772. https://doi.org/10.18632/oncotarget.17781
Lundberg M, Saarilahti K, Mäkitie AA, Mattila PS (2010) TGFbeta1 genetic polymorphism is associated with survival in head and neck squamous cell carcinoma independent of the severity of chemoradiotherapy induced mucositis. Oral Oncol 46(5):369–72. https://doi.org/10.1016/j.oraloncology.2010.02.012
Goutham HV, Mumbrekar KD, Hitendra N, Vadhiraja BM, Fernandes DJ, Satish Rao Dr BS (2017) Genotype-phenotype association of TGF-β1 and GST with chemo-radiotherapy induced toxicity. Int J Radiat Res 15(1):15–23 https://doi.org/10.18869/acadpub.ijrr.15.1.15
Gunnlaugsson A, Kjellen E, Nilsson P, Bendahl PO, Willner J, Johnsson A (2007) Dose-volume relationships between enteritis and irradiated bowel volumes during 5-fluorouracil and oxaliplatin based chemoradiotherapy in locally advanced rectal cancer. Acta oncologica (Stockholm, Sweden) 46(7):937–944. https://doi.org/10.1080/02841860701317873
Dodd MJ, Miaskowski C, Dibble SL, Paul SM, MacPhail L, Greenspan D, Shiba G (2000) Factors influencing oral mucositis in patients receiving chemotherapy. Cancer Pract 8(6):291–297
van Kuilenburg AB, Klumpen HJ, Westermann AM, Zoetekouw L, Van Lenthe H, Bakker PJ, Richel DJ, Guchelaar HJ (2007) Increased dihydropyrimidine dehydrogenase activity associated with mild toxicity in patients treated with 5-fluorouracil and leucovorin. European journal of cancer (Oxford, England : 1990) 43(2):459–465. https://doi.org/10.1016/j.ejca.2006.09.017
Figliolia SL, Oliveira DT, Pereira MC, Lauris JR, Mauricio AR, Oliveira DT, Mello de Andrea ML (2008) Oral mucositis in acute lymphoblastic leukaemia: analysis of 169 paediatric patients. Oral Dis 14(8):761–766. https://doi.org/10.1111/j.1601-0825.2008.01468.x
Diaconescu R, Flowers CR, Storer B, Sorror ML, Maris MB, Maloney DG, Sandmaier BM, Storb R (2004) Morbidity and mortality with nonmyeloablative compared with myeloablative conditioning before hematopoietic cell transplantation from HLA-matched related donors. Blood 104(5):1550–1558. https://doi.org/10.1182/blood-2004-03-0804
Anand A, Anandi P, Jain NA, Lu K, Dunavin N, Hourigan CS, Le RQ, Chokshi PD, Ito S, Stroncek DF, Sabatino M, Barrett AJ, Battiwalla M (2016) CD34+ selection and the severity of oropharyngeal mucositis in total body irradiation-based allogeneic stem cell transplantation. Supportive care in cancer: official journal of the Multinational Association of Supportive Care in Cancer 24(2):815–822. https://doi.org/10.1007/s00520-015-2848-9
Reinbolt RE, Sonis S, Timmers CD, Fernandez-Martinez JL, Cernea A, de Andres-Galiana EJ, Hashemi S, Miller K, Pilarski R, Lustberg MB (2018) Genomic risk prediction of aromatase inhibitor-related arthralgia in patients with breast cancer using a novel machine-learning algorithm. Cancer Med 7(1):240–253. https://doi.org/10.1002/cam4.1256
Alterovitz G, Tuthill C, Rios I, Modelska K, Sonis S (2011) Personalized medicine for mucositis: Bayesian networks identify unique gene clusters which predict the response to gamma-D-glutamyl-L-tryptophan (SCV-07) for the attenuation of chemoradiation-induced oral mucositis. Oral Oncol 47(10):951–955. https://doi.org/10.1016/j.oraloncology.2011.07.006
Sonis S, Antin J, Tedaldi M, Alterovitz G (2013) SNP-based Bayesian networks can predict oral mucositis risk in autologous stem cell transplant recipients. Oral Dis 19(7):721–727. https://doi.org/10.1111/odi.12146
Suresh AV, Varma PP, Sinha S, Deepika S, Raman R, Srinivasan M, Mandapal T, Reddy CO, Anand BB (2010) Risk-scoring system for predicting mucositis in patients of head and neck cancer receiving concurrent chemoradiotherapy [rssm-hn]. J Cancer Res Ther 6(4):448–451. https://doi.org/10.4103/0973-1482.77100
Hurria A, Cirrincione CT, Muss HB, Kornblith AB, Barry W, Artz AS, Schmieder L, Ansari R, Tew WP, Weckstein D, Kirshner J, Togawa K, Hansen K, Katheria V, Stone R, Galinsky I, Postiglione J, Cohen HJ (2011) Implementing a geriatric assessment in cooperative group clinical cancer trials: CALGB 360401. Journal of clinical oncology: official journal of the American Society of Clinical Oncology 29(10):1290–1296. https://doi.org/10.1200/JCO.2010.30.6985
Hurria A, Togawa K, Mohile SG, Owusu C, Klepin HD, Gross CP, Lichtman SM, Gajra A, Bhatia S, Katheria V, Klapper S, Hansen K, Ramani R, Lachs M, Wong FL, Tew WP (2011) Predicting chemotherapy toxicity in older adults with cancer: a prospective multicenter study. Journal of clinical oncology: official journal of the American Society of Clinical Oncology 29(25):3457–3465. https://doi.org/10.1200/JCO.2011.34.7625
Covington JA, Wedlake L, Andreyev J, Ouaret N, Thomas MG, Nwokolo CU, Bardhan KD, Arasaradnam RP (2012) The detection of patients at risk of gastrointestinal toxicity during pelvic radiotherapy by electronic nose and FAIMS: a pilot study. Sensors (Basel) 12(10):13002–13018. https://doi.org/10.3390/s121013002
Orlandi E, Iacovelli NA, De Cecco L, Rancati T, Cavallo A, Ferella L, Mancinelli E, Serafini MS, Devecchi A, Giandini T, Cicchetti A, Bossi P, Alfieri S, Locati L, Licitra L, Fallai C, Valdagni R (2019) Role of microbiota in predicting oral mucositis in head and neck cancer patients treated with IMRT. Radiother Oncol 132:57–57. https://doi.org/10.1016/s0167-8140(19)30278-6
Chua LL, Rajasuriar R, Azanan MS, Abdullah NK, Tang MS, Lee SC, Woo YL, Lim YA, Ariffin H, Loke P (2017) Reduced microbial diversity in adult survivors of childhood acute lymphoblastic leukemia and microbial associations with increased immune activation. Microbiome 5(1):35. https://doi.org/10.1186/s40168-017-0250-1
Friend AJ, Feltbower RG, Newton HL, Picton HM, Glaser AW (2018) Late effects of childhood cancer. Lancet (London, England) 391 (10132):1772. doi:https://doi.org/10.1016/S0140-6736(18)30572-5
Derrien M, Alvarez AS, de Vos WM (2019) The gut microbiota in the first decade of life. Trends Microbiol 27(12):997–1010. https://doi.org/10.1016/j.tim.2019.08.001
Huang K, Liu Y, Tang H, Qiu M, Li C, Duan C, Wang C, Yang J, Zhou X (2019) Glabridin prevents doxorubicin-induced cardiotoxicity through gut microbiota modulation and colonic macrophage polarization in mice. Front Pharmacol 10:107. https://doi.org/10.3389/fphar.2019.00107
Wang A, Ling Z, Yang Z, Kiela PR, Wang T, Wang C, Cao L, Geng F, Shen M, Ran X, Su Y, Cheng T, Wang J (2015) Gut microbial dysbiosis may predict diarrhea and fatigue in patients undergoing pelvic cancer radiotherapy: a pilot study. PLoS One 10(5):e0126312. https://doi.org/10.1371/journal.pone.0126312
Wen Y, Jin R, Chen H (2019) Interactions between gut microbiota and acute childhood leukemia. Front Microbiol 10:1300. https://doi.org/10.3389/fmicb.2019.01300
Dong ST, Butow PN, Costa DS, Lovell MR, Agar M (2014) Symptom clusters in patients with advanced cancer: a systematic review of observational studies. J Pain Symptom Manag 48(3):411–450. https://doi.org/10.1016/j.jpainsymman.2013.10.027
Montassier E, Al-Ghalith GA, Ward T, Corvec S, Gastinne T, Potel G, Moreau P, De La Cochetiere MF, Batard E, Knights D (2016) Erratum to: pretreatment gut microbiome predicts chemotherapy-related bloodstream infection. Genome Med 8(1):61. https://doi.org/10.1186/s13073-016-0321-0
Wardill HR, Van Sebille YZ, Mander KA, Gibson RJ, Logan RM, Bowen JM, Sonis ST (2015) Toll-like receptor 4 signaling: a common biological mechanism of regimen-related toxicities: an emerging hypothesis for neuropathy and gastrointestinal toxicity. Cancer Treat Rev 41(2):122–128. https://doi.org/10.1016/j.ctrv.2014.11.005
Acknowledgments
Thanks are given to members of the Multinational Association of Supportive Care in Cancer and International Society for Oral Oncology Mucositis Study Group for supporting this initiative.
Funding
Hannah R Wardill is supported by an NHMRC CJ Martin Biomedical Research Fellowship.
Author information
Authors and Affiliations
Consortia
Contributions
HRW/JMB/SE/PB/KKFC/STS: conceptualization and project initiation, DAC/LP/SE/HRW/JMB: search strategy and record retrieval, HRW/JMB: record triaging, HRW/JMB/NMAB/YZAVS/EAHL/MAC: record review and data extraction, HRW/JMB: data synthesis/writing, All authors: review and editing of final manuscript.
Corresponding author
Ethics declarations
Conflict of interest
Dr. Sonis reports personal fees from Biomodels, LLC, personal fees from Primary Endpoint Solutions, LLC, outside of the submitted work. As an employee of Biomodels and PES, he is involved in assisting industry, government and academics in the study and enablement of drugs, biologicals and devices to treat patients for a broad range of indications including cancer and oral toxicities of cancer therapy. He does not have equity or receive payment from any of the companies’ clients.
Code availability
N/A
Ethics approval
N/A
Consent to participate
N/A
Consent for publication
N/A
Additional information
Publisher’s note
Springer Nature remains neutral with regard to jurisdictional claims in published maps and institutional affiliations.
Electronic supplementary material
ESM 1
(DOCX 22 kb)
Rights and permissions
About this article
Cite this article
Wardill, H.R., Sonis, S.T., Blijlevens, N.M.A. et al. Prediction of mucositis risk secondary to cancer therapy: a systematic review of current evidence and call to action. Support Care Cancer 28, 5059–5073 (2020). https://doi.org/10.1007/s00520-020-05579-7
Received:
Accepted:
Published:
Issue Date:
DOI: https://doi.org/10.1007/s00520-020-05579-7