Abstract
Cerchar abrasion index (CAI) value is one of the most important test parameters for determining the abrasion value of a cutter or drilling bit. This study aims to present the relation between CAI and various geomechanical properties of building stones. Herein, single and multiple regression analyses were performed to determine the best measure of the relation between CAI and three geomechanical properties. The CAI value is strongly related to the Shore hardness. However, the correlation coefficient increases when we jointly consider the Shore hardness, porosity, and uniaxial compression strength of rock. On the contrary, the analyses results show that the significance level of the CAI value is lower when the CAI value is less than 1. The correlation between CAI and Shore hardness is higher when the CAI values greater than 1 are considered. Also, the R2 value increases from 0.843 to 0.946 in multiple regression analysis.
Similar content being viewed by others
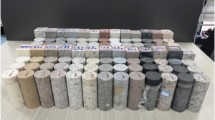
Avoid common mistakes on your manuscript.
Introduction
Natural stones are commonly used building materials, and the cutting operation is very important in the processing and production of natural stones. The Cerchar abrasivity index (CAI) value is well known as a crucial factor for determining the cutting process performance of natural building stones, particularly the selection of cutting materials. Additionally, to determine rock abrasiveness, the CAI value is commonly used.
Abrasion influences the wear life of cutting tools in any rock excavation operation, where small holes are drilled for blasting large-diameter tunnels, which are bored by tunnel boring machines (TBMs). Abrasion and wear are vital parameters for determining the lifetime of the drilling rods/bits or cutter in mining and industrial applications. Various rock abrasion measures have been introduced over the years to help engineers estimate tool life. As the wear life of rock cutting tools often has a linear relation with the degree of rock abrasion, any variation in these measurements will have a direct and proportional impact on the estimated tool life, operational duration, and related costs (Rostami et al. 2014). The factors affecting rock abrasivity are as follows: mineral composition, grain shape and size, hardness of rock minerals, type of matrix material, and the physical and mechanical properties of rocks, such as strength, hardness, and toughness (Singh and Ghose 2016). CAI is one of the easiest, most convenient, and most reliable tests for determining the abrasiveness of rocks (Tripathy et al. 2015), and the performance of excavators and cutting machines can be predicted using the CAI value.
CAI was developed in the 1970s by the Centre d’Etudes et Recherches des Charbonages (CERCHAR) de France, and the test procedure was first published in 1986 (Cerchar 1986). Currently, two types of testing devices are used to determine CAI values: CERCHAR apparatus and the West apparatus. In both devices, a rock specimen is held in a vise and a stylus with a 90° conical tip loaded with a static force of 70 N moves over the rock surface at a velocity of 10 mm/s while scratching it. This procedure is repeated for at least five times on each surface. The length of the wear flat is measured with a microscope, and the CAI value is calculated using Eq. 1 as follows:
where di is the diameter of the abraded flat area measured in 0.1-mm units (ASTM D7625 2010; Alber et al. 2014). Table 1 shows the CAI-based classification of abrasivity established by the International Society for Rock Mechanics (ISRM) 2015 (Alber et al. 2014).
CAI continues to be studied by many researchers. One of the earliest CAI studies was conducted by Suana and Peters (1982), where the CAI values were predicted on the basis of the equivalent quartz content, as determined in thin-section analyses. West (1989) studied the relation between the quartz content and CAI value of rocks and reported that the quartz content is the main parameter influencing the CAI value. Al-Ameen and Waller (1994) reported the CAI value to be broadly related to rock strength. Plinninger et al. (2004) studied the correlation between the CAI and other wear-relevant parameters. The authors determined that although CAI is a quick and simple testing method for rock abrasivity classification, the Cerchar test has some weaknesses with respect to predicting wear. Alber (2008) demonstrated the effect of stress on CAI and proposed a new approach for estimating tool wear life based on the determination of the stress-dependent CAI value. Lassnig et al. (2008) reported the CAI value to be independent of grain size. Yaralı et al. (2008) investigated the relations between the CAI and petrographic properties of coal and found a linear relation between the CAI values of rocks and their quartz content, degree of cementing, equivalent quartz content, and quartz grain size. Kahraman et al. (2010) used a number of indirect methods to predict the uniaxial compression strength and elastic modulus of Misis Fault Breccia, as well as the CAI, using regression and artificial neural networks analysis. Deliormanli (2012) revealed the relation between the CAI value, strength, and wear properties of marble stones. Moradizadeh et al. (2013) studied the relation between the CAI value and the equivalent quartz content, grain content, and several geomechanical properties, such as the point load strength index, slake durability index, and moisture content in sandstone rocks in Iran. Kahraman et al. (2015) used the CAI and other non-destructive methods as well as regression analysis to investigate the predictability of differential stress values (Δσ) in Misis Fault Breccia. The authors determined that applying a multiple regression model that included the CAI value increases the model reliability for predicting Δσ in Misis Fault Breccia. Er and Tuğrul (2016) used a simple regression method to examine the relation between the CAI and the physico-mechanical properties of different granitic rocks. A strong relation between the CAI and the mean quartz size and content was found. Further, positive correlations between the CAI and the Shore hardness, Schmidt hardness, uniaxial compression strength, and tensile strength and a negative correlation between the CAI and the Bohme abrasion strength in granitic rocks were also found. Ko et al. (2016) noted the inadequacy of a single parameter in predicting the CAI value and highlighted the positive influence of the uniaxial compression strength (UCS) and the brittleness index in the CAI prediction model for igneous rocks and the UCS and the Brazilian tensile strength on the CAI prediction model for metamorphic rocks. Capik and Yilmaz (2017) investigated the relation between CAI and uniaxial compressive strength (UCS), point load strength, Brazilian tensile strength, Schmidt rebound hardness, and equivalent quartz content (EQC). Also, the relation between drill bit lifetime and CAI was examined. They revealed positive relation between CAI and uniaxial compressive strength (UCS), point load strength, Brazilian tensile strength, Schmidt rebound hardness, and equivalent quartz content.
In this study, the correlation between three geomechanical properties and the CAI value of building stones, as well as the reliability of the CAI values, was investigated. Abrasivity is strongly related to the hardness, strength, and porosity of rocks. UCS, porosity, and Shore hardness tests were conducted to build a predictive model for the CAI index. The correlation between these geomechanical properties and the CAI was determined using simple and multiple regression analyses.
Materials and methods
Experimental works
Tests were conducted on samples that were primarily taken from the western region of Turkey. In this study, 30 different types of building stone specimens were used and all tests, related to the UCS, porosity, Shore hardness, and CAI, were conducted according to ISRM and ASTM standards (ASTM D7625 2010; Alber et al. 2014; ISRM 1979a, b; Altindag and Guney 2007).
The UCS tests on cylindrical core specimens were conducted with a height-to-diameter ratio between 2.5 and 3.0. A loading rate of 150 kg/s was chosen to ensure that the stress rate ranged between 0.5 and 1.0 MPa/s. The tests were repeated on five samples for each rock type.
The porosity of the rocks was determined using saturation and caliper techniques. Tests on cubic samples were performed and the dimensions of the samples were measured using a digital Vernier caliper with an accuracy of 0.01-mm. Caliper measurements were measured several times on each side. The bulk volume (V) of the specimens was calculated by multiplying the dimensions of three sides. Then, the samples were immersed in water and they saturated in a day. After removing the saturated samples from the water, the surfaces were dried and the saturated-surface dry mass (Msat) was determined. Next, the samples to were dried to a constant mass at 105 °C, cooled in a desiccator, and the dry mass (Ms) of the samples was then determined. The porosity of the rocks was calculated as follows:
where ρw is the density of water.
Next, the Shore hardness of the specimens was determined, which is a widely used scale for measuring rock hardness, using a standard C-2 type Shore scleroscope (Fig. 1a). One surface of the cubic samples was polished and a 1-cm margin from was taken the sample edges. On each sample, 30 measurements were performed and the average values were taken as the Shore hardness of the rock.
CAI tests were performed using a modified West apparatus (Fig. 1b) with styluses (HRC 55 ± 1) loaded with 70 N moving over a distance of 10 mm in 10 s. The tip of each stylus has a conical angle of 90°. These tests were performed on the cubic samples, and five styluses were used for each sample. For the test result, the average value of five measurements was used. The styluses were inspected before each test to ensure the accuracy of the wear flat. A side-view wear-flat measurement method was used to measure the wear surface of the styluses. The wear surface of each stylus was measured using a Nikon SMZ 1500 stereoscopic zoom microscope with 0.01-mm accuracy. Table 2 lists the results of the above tests.
Data analysis
Next, regression modeling techniques were used to evaluate the relation between the CAI and other parameters, using both simple and multiple regression models to establish the relations.
Simple linear regression is a statistical method that summarizes and examines the relation between two continuous variables. One variable, denoted as x, is regarded as the predictor, (independent) and the other, denoted as y, is the response (dependent) variable. A simple regression is defined as follows:
where
A is the constant and B1 is the x coefficient representing the slope of the equation.
These parameters in the equation were calculated using the least squares method. The analysis, which is supported with the correlation coefficient (r), is a parameter showing the degree of the linear relation between two variables, usually labeled as X and Y. The value of r can range between 1 and − 1. A correlation coefficient value close to 1 indicates a strong positive linear relation. A value close to − 1 indicates a strong negative linear relation. A value close to 0 indicates the absence of any linear relation, although there could be a nonlinear relation between the variables.
Multiple linear regression is the most commonly used form of linear regression analysis. As a predictive analysis method, multiple linear regression is used to explain the relation between one continuous dependent variable and two or more independent variables.
The multiple linear regression model relating a y variable to x variables is written as follows:
The result indicates the relation between a dependent or criterion variable of interest (Y) and a set of k independent variables or potential predictor variables (X1, X2, X3, …, XN), whereby the scores of all the variables are measured for N cases.
These calculations are reasonably complicated. Therefore, in this study, the statistical software program Minitab v13 was used for multiple regression analysis. Then, the analysis results were evaluated by determining the correlation coefficients and performing F tests (Afifi and Azen 1979; Natrella 1963; Neter and Wasserman 1974).
Analysis of variance (ANOVA) is a well-known statistical method for analyzing quantitative data. It computes the probability that differs among the observed means could simply be induced by chance. ANOVA is indeed a more specific and constrained example of the general approach employed in multiple regression analysis. ANOVA results are depicted in an ANOVA table, which contains columns labeled “Source,” “SS or sum of squares,” “df for degrees of freedom,” “MS for the mean square,” “F or F ratio,” and “P, prob., probability, sig., or sig. of F.” F ratio (Fc) is computed by dividing the mean squares between by the mean squares within.
The proposed hypotheses were tested using an F test in each ANOVA, as follows:
A null (H0) hypothesis means that no relation exists between the dependent variable Y and the independent variable Xi. The other hypothesis, H1, is the opposite of null and the tested hypothesis is always denoted by H0. This concept can be described in this way: if the Ftable value is smaller than Fc (Ft < Fc), null (H0) is declined, or in other words, H1 is accepted instead (Gunst and Mason 1980).
Results and discussions
All the statistical evaluations were performed using Minitab V13 statistical software. First, a simple linear regression analyses was conducted for each parameter and a correlation matrix was constructed, as shown in Table 3. Figure 2 shows a scatterplot of the related parameters.
During the statistical analysis of CAI and porosity (%), the functional relation between the two groups was determined. This function was derived using the least squares method, as shown in Fig. 2a, where it can be seen that there is no linear relation between the CAI and porosity (R = − 0.234).
During the statistical analysis of CAI and UCS, a function that defines the relation between the CAI and UCS values was obtained. This function was also derived using the least squares method, as shown in Fig. 2b. As can be seen from the plot, a low value of R = 0.388 is consistent with the absence of any linear relation between the CAI and UCS.
During the statistical analysis of the CAI and Shore hardness, a functional relation was obtained, as shown in Fig. 2c, which suggests that there is a strong positive relation between the CAI and Shore hardness values (R = 0.901).
After evaluating the porosity, UCS, and Shore hardness with respect to CAI, a multiple linear regression analyses was performed to estimate the CAI. UCS, porosity, and Shore hardness were used as independent variables to predict the CAI value and multiple regression analyses were performed using two and three variables.
In the first multiple regression analysis, the predictability of the CAI was examined on the basis of UCS and porosity values. The analysis results for UCS versus CAI and porosity versus CAI indicate that there is no linear relation in either case (R = 0.432 and R2 = 0.187). The adjusted R2 value indicates that this model accounts for 18.7% of variance in the CAI–no significance model (Fig. 3).
Second multiple regression analysis was performed for Shore hardness and UCS versus CAI; the results are listed in Table 4. The significance of this model was noted by citing the R (0.911) and adjusted R2 (0.831) values, which show the strength of the model. For the final results, we can say that the Fc ratio (in the ANOVA results shown in Table 4) is 66.60 and is significant at P < 0.001. The Ft ratio from the F distribution table (for α = 0.05) is 3.35. The Ft < Fc and P < 0.05 result shows that the null (H0) hypothesis is rejected. This yields a strong proof for a linear relation between the response (CAI) and two explanatory variables (Shore hardness and UCS; Fig. 4). The proposed model for estimating the CAI based on Shore hardness and UCS is given below:
Third multiple regression analysis was conducted for the predictability of the CAI based on the Shore hardness and porosity values; the results are listed in Table 5. According to the multiple regression analysis, the Shore hardness versus CAI and the porosity versus CAI results indicate that there may be a linear relation (R = 0.906, R2 = 0.822). The adjusted R2 value shows that this model accounts for 82.2% of the variance in the CAI, which is significant. In Table 5, when df1 = 2 and df2 = 27, the Ft value from the F distribution table (for α = 0.05) decreases to 3.35, whereas the calculated Fc value is 62.30. In other words, Ft is significantly smaller than Fc. Additionally, the P value is smaller than 0.05, which supports proof for a linear relation between the CAI and the two explanatory variables (reject the H0). It can be concluded that the multiple regression equation used to determine the results, as listed in Table 5, can be used for predicting the CAI (Fig. 5). The model is as follows:
It was found that the three-parameter multiple linear regression was the best model for CAI estimation. Table 6 shows the ANOVA results for the model with three parameters. The sum of squares values reflects the partitioning of the total variance in the criterion by the regression effect due to intelligence and residual. To compute the F ratio, the sum of squares regression and sum of squares residual are divided by their respective degrees of freedom, which result in mean square values. Then, the mean square regression value is divided by the mean square residual value. The resulting F ratio is then compared to those in an F table of critical values to determine whether the observed F ratio is greater than would be expected on the basis of chance. The critical value of the F ratio with (3, 26) degrees of freedom and an alpha level of.05 is 2.97. Here, the F ratio in Table 6 is 46.55, which is greater than the critical value of 2.97. The P column in Table 8 also reflects the fact that our observed critical F value is less than the F ratio (P < 0.05). Therefore, we can conclude from this analysis that the regression effect for intelligence is greater than zero and thus intelligence alone is a good predictor of CAI (Fig. 6). The best model developed for estimating the CAI with three parameters is as follows:
CAI values range from less than 0.5 for soft rocks to more than 5.0 for hard rocks. Soft rocks are known to generate a little wear on the CAI testing pin, which makes determining the CAI value very hard (Deliormanli 2012). For this reason, the samples were classified according to CAI value and simple regression analysis was carried out separately for the CAI value higher and lower than 1. Figure 7a–c shows scatterplot of the related parameters for the CAI value lower than 1. According to the results, no significant linear relation was found between CAI and porosity and UCS or Shore hardness for the samples with CAI value lower than 1. When the samples with a CAI value higher than 1 were considered (Fig. 7d–f), a high correlation between Shore hardness and CAI (R = 0.946, R2 = 0.895) was found. After simple regression analysis, multiple regression analysis for samples having a CAI value higher than 1 was performed.
For CAI values higher than 1, a multiple regression analysis was performed to determine the predictability of the CAI using UCS and porosity values; the results are listed in Table 7. According to these results for CAI versus UCS and porosity, there is no linear relation (R = 0.17, R2 = 0.031). The adjusted R2 value indicates that this model accounts for 0.05% of variance in the CAI–no significance model (Fig. 8).
Second multiple regression analysis was performed to determine the predictability of CAI using Shore hardness and UCS; the results are given in Table 8. According to these results, the Shore hardness versus CAI and UCS versus CAI could have a strong linear relation (R = 0.955, R2 = 0.913). The adjusted R2 value indicates that this model accounts for 91.3% of variance in the CAI–a significance model (Fig. 9) In Table 8, we can see that when df1 = 2 and df2 = 11, the Ft value from the F distribution table (for α = 0.05) decreases to 3.98 and the calculated Fc value is 57.99. In other words, Ft is smaller than Fc (Ft < Fc). Also, when the P value is smaller than 0.05, it supports a proof for a linear relation between the CAI and the two explanatory variables (reject the H0). Based on these results, we can conclude that the results for the multiple regression analysis, as listed in Table 8, can be used to predict the CAI. The model for this CAI prediction is as follows:
We performed a third multiregression analysis for Shore hardness and porosity versus CAI, the results of which are shown in Table 9. The significance of the model was noted by citing the multiple R (0.957) and adjusted R2 (0.916) values, which display the strength of the model. The results of the model prediction are given in Table 9. For the final results, it can be seen that the Fc ratio (in the ANOVA; Table 11) is 60.21 and is significant at P < 0.001. The Ft ratio from the F distribution table (for α = 0.05) is 3.98. Therefore, Ft < Fc and P < 0.05 indicate that the null (H0) hypothesis is rejected. This situation supplies proof for a linear relation between the response (CAI) and the two explanatory variables (Shore hardness and porosity; Fig. 10). The equation for this model is as follows:
Next, correlation and multiple regression analyses were conducted to examine the relation between the CAI and the potential predictors Shore hardness, porosity, and UCS. Table 10 summarizes the descriptive statistical and analysis results, where it can be seen that each of the predictors is positively and significantly correlated with the CAI. The multiple regression model using all three predictors produced R = 0.972, R2 = 0.946, Ft < Fc (3.70 < 57.90), and P < 0.05. These results indicate that Shore hardness, porosity, and UCS have significance in predicting the CAI (Fig. 11). The best model developed for estimating the CAI with three parameters for the samples having CAI values higher than 1 is as follows:
Models developed from multiple regression analyses and regression coefficients are listed in Table 11. It is obviously seen that samples with CAI value is higher than 1 have higher relation between CAI and other tested geomechanical properties.
Previous studies related to CAI have been carried out as three main groups. Some researchers have focused on CAI values predicted on the basis of petrographic properties such as equivalent quartz content, average grain size, and grade and type of cement (Suana and Peters 1982; West 1989; Lassnig et al. 2008; Moradizadeh et al. 2013), while many studies have investigated equipment used and test conditions (Plinninger et al. 2003; Rostami 2005; Rostami et al. 2005; Käsling and Thuro 2010; Hamzaban et al. 2012; Aydın et al. 2016; Hamzaban et al. 2018). Others have studied correlations between CAI and geomechanical properties of the rock (Al-Ameen and Waller 1994; Plinninger et al. 2004; Yaralı et al. 2008; Kahraman et al. 2010; Deliormanli 2012; Ko et al. 2016; Er and Tugrul 2016).
In this study, relations between three geomechanical properties and CAI values of building stones were investigated. Unlike previous studies, the results of the study showed that CAI is strongly related to the Shore hardness of the rock. Moreover, the relation can be determined more strongly with UCS and porosity values using multiple regression analyses.
Another difference with previous studies is that, for CAI values higher than 1, the results obtained from multiple regression analysis are positively and significantly correlated with CAI, but not with UCS and porosity versus CAI.
Conclusion
Correlations between the CAI and three geomechanical properties of sedimentary, igneous, and metamorphic rocks were investigated in this study. Using simple and multiple regression analyses, the degree to which CAI values can be predicted was determined based on results obtained from rock test measurements. The results are profoundly discussed in the following paragraphs.
Based on simple regression analysis, it was found that Shore hardness has a strong relation with the CAI index, whereas the porosity and uniaxial strength of rocks have no positive correlation. We determined the relation between the geomechanical properties and CAI using multiple regression analysis. Strong multiple correlation values were obtained from these analyses, except for UCS and porosity versus CAI.
For rocks with CAI values higher than 1, the obtained multiple regression analysis results indicate that each of the three parameters is positively and significantly correlated with the CAI but not UCS and porosity versus CAI.
The simple regression analysis results show that there is a positive correlation between CAI and UCS and negative correlation between CAI and porosity. These correlations are not strong, but there is a strong positive relation between the CAI and Shore hardness values. For these reasons, multiple regression analysis results in Tables 4, 5, 6, 7, 8, 9, and 10; F values of UCS and porosity are quite low. At the same time, their effects are very limited in Eqs. 6, 7, 8, 9, 10, and 11.
The CAI is a major factor used in evaluating natural stones to be used as building materials. The results indicate strong correlations between the CAI and geomechanical properties of rocks, which can be used to predict the CAI.
References
Afifi AA, Azen SP (1979) Statistical analysis, computer oriented approach. Academic Press, New York
Al-Ameen SI, Waller MD (1994) The influence of rock strength and abrasive mineral content on the Cerchar abrasivity index. Eng Geol 36:293–301
Alber M (2008) Stress dependency of the Cerchar abrasivity index (CAI) and its effect on wear of selected rock cutting tools. Tunn Undergr Space Technol 23:351–359. https://doi.org/10.1016/j.tust.2007.05.008
Alber M, Yarali O, Dahl F, Bruland A, Kasling H, Michalakopoulos TN, Cardu M, Hagan P, Aydın H, Ozarslan A (2014) ISRM suggested method for determining the abrasivity of rock by the CERCHAR abrasivity test. Rock Mech Rock Eng 47:261–266
Altindag R, Guney (2007) A ISRM suggested method for determining the shore hardness value. In: Ulusay R, Hudson JA (eds) The complete ISRM suggested methods for rock characterization, testing and monitoring: 1974–2006, pp 109–112
ASTM D7625 (2010) Standard test method for laboratory determination of abrasiveness of rock using the CERCHAR method, American Society for Testing and Materials, West Conshohocken, United States, p. 1–6 10–1520/D7625-10
Aydın H, Yaralı O, Duru H (2016) The effects of specimen surface conditions and type of test apparatus on Cerchar abrasivity index. Karaelmas J Sci Eng 6(2):293–298
Capik M, Yilmaz AO (2017) Correlation between Cerchar abrasivity index, rock properties, and drill bit lifetime. Arab J Geosci 10(15). https://doi.org/10.1007/s12517-016-2798-7
Cerchar (1986) The Cerchar abrasiveness index Centre d’Etudes et Recherches de Charbonnages de France, Verneuil
Deliormanli AH (2012) Cerchar abrasivity index (CAI) and its relation to strength and abrasion test methods for marble stones. Constr Build Mater 30:16–21. https://doi.org/10.1016/j.conbuildmat.2011.11.023
Er S, Tugrul A (2016) Correlation of physico-mechanical properties of granitic rocks with Cerchar abrasivity index in Turkey. Measurement 91:114–123. https://doi.org/10.1016/j.measurement.2016.05.034
Gunst RF, Mason RL (1980) Regression analysis and its application. Marcel Dekker Inc, New York
Hamzaban M-T, Memarian H, Rostami J, Ghasemi-Monfared H (2012) Study of rock–pin interaction in Cerchar abrasivity test. Int J Rock Mech Min Sci 72:100–108. https://doi.org/10.1016/j.ijrmms.2014.09.007
Hamzaban M-T, Memarian H, Rostami J (2018) Determination of scratching energy index for Cerchar abrasion test. J Min Environ 9(1):73–89. https://doi.org/10.22044/jme.2017.5738.1389
ISRM (1979a) Suggested methods for determining water content, porosity, density, absorption and related properties and swelling and slake-durability index properties. Int J Rocks Mech Min Sci Geomech 16:141–156
ISRM (1979b) Suggested methods for determining the uniaxial compressive strength and deformability of rock materials. Int J Rocks Mech Min Sci Geomech 16:135–140
Kahraman S, Alber M, Fener M, Gunaydin O (2010) The usability of Cerchar abrasivity index for the prediction of UCS and E of Misis Fault Breccia: regression and artificial neural networks analysis. Expert Syst Appl 37:8750–8756. https://doi.org/10.1016/j.eswa.2010.06.039
Kahraman S, Alber M, Gunaydin O, Fener M (2015) The usability of the Cerchar abrasivity index for the evaluation of the triaxial strength of Misis Fault Breccia. Bull Eng Geol Environ 74:163–170. https://doi.org/10.1007/s10064-014-0618-4
Käsling H, Thuro K (2010) Determining abrasivity of rock and soil in the laboratory. In: Williams et al (eds) Geologically active. Taylor & Francis Group, London, pp 1973–1980
Ko TY, Kim KT, Son Y, Jeon S (2016) Effect of geomechanical properties on Cerchar abrasivity index (CAI) and its application to TBM tunnelling. Tunn Undergr Space Technol 57:99–111. https://doi.org/10.1016/j.tust.2016.02.006
Lassnig K, Latal C, Klima K (2008) Impact of grain size on the Cerchar abrasiveness test. Geomech Tunn 1(1):71–76. https://doi.org/10.1002/geot.200800008
Moradizadeh M, Ghafoori M, Lashkaripour G, Azali TS (2013) Utilizing geological properties for predicting Cerchar abrasiveness index (CAI) in sandstones. Int J Emerg Technol Adv Eng 3(9):99–109
Natrella MG (1963) Experimental statistics. National Bureau of Standards, Washington DC
Neter J, Wasserman W (1974) Applied linear statistical models. R.D. Irwin Inc., Homewood
Plinninger R, Kasling H, Thuro K, Spaun G (2003) Testing conditions and geomechanical properties influencing the CERCHAR abrasiveness index (CAI) value. Int J Rock Mech Min Sci 40:259–263. https://doi.org/10.1016/S1365-1609(02)00140-5
Plinninger R, Kasling H, Thuro K (2004) Wear prediction in hardrock excavation using the CERCHAR abrasiveness index (CAI). In: Proceedings of the Eurock 2004 and 53rd Geomechanics Colloquium, p 599–604
Rostami J (2005) CAI testing and its implications. Tunnels Tunn Int 37(10):43–46
Rostami J, Ozdemir L, Bruland A, Dahl F (2005) Review of issues related to Cerchar abrasivity testing and their implications on geotechnical investigations and cutter cost estimates. In: Hutton JD, Rogstad WD (eds) Proceedings of the 2005 Rapid Excavation and Tunnelling Conference (RETC) in Seattle. Society for Mining, Metallurgy and Exploration, Littleton
Rostami J, Ghasemi A, Gharahbagh AE, Dogruoz C, Dahl F (2014) Study of dominant factors affecting cerchar abrasivity index. Rock Mech Rock Eng 47:1905–1919. https://doi.org/10.1007/s00603-013-0487-3
Singh RN, Ghose AK (2016) Engineered rock structures in mining and civil construction. Taylor & Francis/Balkema, Netherlands
Suana M, Peters TJ (1982) The Cerchar abrasivity index and its relation to rock mineralogy and petrography. Rock Mech 15(1):1–8. https://doi.org/10.1007/BF01239473
Tripathy A, Singh TN, Kundu J (2015) Prediction of abrasiveness index of some Indian rocks using soft computing methods. Measurement 68:302–309. https://doi.org/10.1016/j.measurement.2015.03.009
West G (1989) Rock abrasiveness testing for tunnelling. Int J Rock Mech Min Sci Geomech Abstr 26(2):151–160
Yaralı O, Yasar E, Bacak G, Ranjith PG (2008) A study of rock abrasivity and tool wear in coal measures rocks. Int J Coal Geol 74:53–66. https://doi.org/10.1016/j.coal.2007.09.007
Yenice H, Ozdogan MV, Ozfirat MK (2018) A sampling study on rock properties affecting drilling rate index (DRI). J Afr Earth Sci 141:1–8. https://doi.org/10.1016/j.jafrearsci.2018.01.015
Author information
Authors and Affiliations
Corresponding author
Rights and permissions
About this article
Cite this article
Ozdogan, M.V., Deliormanli, A.H. & Yenice, H. The correlations between the Cerchar abrasivity index and the geomechanical properties of building stones. Arab J Geosci 11, 604 (2018). https://doi.org/10.1007/s12517-018-3958-8
Received:
Accepted:
Published:
DOI: https://doi.org/10.1007/s12517-018-3958-8