Abstract
Bloom date is an important production trait in sour cherry (Prunus cerasus L.) as the risk of crop loss to floral freeze injury increases with early bloom time. Knowledge of the major loci controlling bloom date would enable breeders to design crosses and select seedlings with late bloom date. As sour cherry is a segmental allotetraploid, quantitative trait locus (QTL) analysis for bloom date was performed based on haplotype reconstruction by identifying the parental origins of marker alleles in sour cherry. A total of 338 sour cherry individuals from five F1 populations were genotyped using the cherry 6K Illumina Infinium® SNP array and phenotyped for bloom date in 3 years. A total of four QTLs were identified on linkage group (G)1, G2, G4, and G5, respectively. For these QTLs, 14 haplotypes constructed for the QTL regions were significantly associated with bloom date, accounting for 10.1–27.9% of the bloom date variation within individual populations. The three most significant haplotypes, which were identified for the G4 (G4-k), G2 (G2-j), and G1 (G1-c) QTLs, were associated with 2.8, 1.8, and 1.0 days bloom delay, respectively. These three haplotypes were also demonstrated to have additive effects on delaying bloom date for both individual and multiple QTLs. These results demonstrate that bloom date is under polygenic control in sour cherry; yet, pyramiding late blooming haplotypes for single and multiple QTLs would be an effective strategy to obtain later blooming offspring.
Similar content being viewed by others
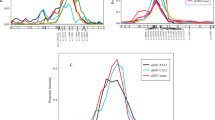
Avoid common mistakes on your manuscript.
Introduction
Breeding for late bloom date is an important objective for many Prunus breeding programs due to the increased risk of crop loss for early blooming cultivars. In sour cherry (Prunus cerasus), low-temperature damage to flower buds is the most important factor limiting yields. For example, Michigan produces around 70% of the nation’s total crop each year; however, in 2012, more than 90% of the sour cherry crop was lost due to multiple freezes resulting in huge economic losses (NASS 2012). Therefore, late bloom date to reduce the likelihood of flower bud loss from spring freeze damage is a major breeding priority for sour cherry (Iezzoni 1996). Sour cherry exhibits more extensive variation for late bloom date than sweet cherry (Prunus avium), one of its two progenitor species. This is likely due to variation contributed by the second progenitor species, the very late blooming ground cherry (Prunus fruticosa) that is native to Russia (Dirlewanger et al. 2009). Therefore, the identification of bloom date QTL is of particular interest in sour cherry. Mining alleles conferring late bloom date and development of new cultivars with late bloom would significantly reduce the probability of floral damage caused by low spring temperatures.
Bloom date is a quantitative trait with high broad sense heritability in most fruit tree species (Anderson and Seeley 1993; Wang et al. 2000; Dirlewanger et al. 2012; Sánchez-Pérez et al. 2014). QTL for bloom date has been detected in numerous genomic regions in Prunus species; however, a major and consistent QTL was identified within the same region on G4 in almond, sweet cherry, peach, and apricot (Dirlewanger et al. 1999; Verde et al. 2002; Quilot et al. 2004; Fan et al. 2010; Campoy et al. 2011; Dirlewanger et al. 2012). For example, in almond, late bloom was found to be controlled primarily by one major gene located on G4 responsible for at least 79% of the phenotypic variation (Ballester et al. 2001). In sweet cherry, QTL with the largest effect was detected on G4 using two F1 progeny populations from the crosses ‘Regina’ × ‘Lapins’ and ‘Regina’ × ‘Garnet’ (Castède et al. 2014). Dirlewanger et al. (2012) and Castède et al. (2014) identified QTL linked to bloom date on G1 and G2 using the same populations in sweet cherry as well. Wang et al. (2000) also identified bloom date QTLs on G1 and G2 in the sour cherry ‘Rheinische Schattenmorelle’ × ‘Erdi Botermo’ F1 population using restriction fragment length polymorphism markers. The genomic synteny of Prunus species (peach, almond, apricot, and cherry) has been known to be highly conserved, and therefore, the discovery of QTL in one species often leads to the discovery of that QTL in the same region in another species (Dirlewanger et al. 2004).
Sour cherry is a segmental allotetraploid, meaning that while there is preferential pairing of chromosomes within the sub-genomes of sour cherry, non-bivalent pairing between sub-genomes is also common (Beaver and Iezzoni 1993; Schuster and Wolfram 2005). Because of the complexity of chromosome pairing, the understanding of the genetic control of bloom date in sour cherry has lagged behind that of other Prunus species. With the availability of the cherry 6K Illumina Infinium® II array as part of the RosBREED project (www.rosbreed.org) (Peace et al. 2012), genome-wide single nucleotide polymorphism (SNP) markers can be assayed. Additionally, advanced statistical approaches and software such as TetraploidMap (Hackett et al. 2007) and TetraOrigin (Zheng et al. 2016) allow researchers to phase parental linkage with the high-density SNP markers and use this information to construct offspring haplotypes for each of the four homologous chromosomes.
In this study, QTL detection was performed in five sour cherry F1 populations and QTL haplotype-trait associations were investigated. The objectives of this study were to (1) identify QTLs controlling bloom date in sour cherry and (2) determine the late-blooming alleles (haplotypes) for these QTL that would have potential for marker-assisted breeding.
Materials and methods
Plant materials
A total of 338 parents, ancestors, and offspring from five bi-parental F1 populations including eight parents were used in this study (Fig. 1a). Populations were as follows: M172 × 25-02-29 (Pop1, N = 111), ‘Montmorency’ × 25-02-29 (Pop2, N = 53), 25-14-20 × 25-02-29 (Pop3, N = 67), ‘Újfehértói Fürtös’ (UF) × ‘Surefire’ (Pop4, N = 76), and ‘Rheinische Schattenmorelle’ (RS) × ‘Englaise Timpurii’ (ET) (Pop5, N = 23). All of these individuals are maintained at the Michigan State University Clarksville Research Station, Clarksville, Michigan.
a Pedigrees of the five bi-parental populations used in this study where the eight parents of the bi-parental populations are in blue boxes. The F1 populations are in white boxes with the number of progeny individuals below. Grandparents of the populations are also included when available (gray) to show the relatedness of populations. Parental qP-BD2.1m haplotypes across different generations are illustrated using letters to identify the different haplotypes. Those haplotypes with the same letter and color are identical by descent. For example, haplotype “j” segregating in populations 3 and 4 are identical by descent, originating from ‘UF’. b Marker allele composition of each haplotype for qP-BD2.1m is illustrated using the smallest number of markers needed to differentiate the haplotypes. The complete marker composition is in Supplementary Fig. S4
Phenotypic data
Bloom date was recorded in 2011, 2012, and 2014 when 50% of the flowers were open on a tree. Bloom date data were converted to growing degree days (GDD) with a base temperature of 4.4 °C (40 °F) as described by Stegmeir et al. (2014a). The phenotypic data were analyzed using SAS version 9.13 (SAS Institute Inc.), and the model PROC MIXED was used to obtain the best linear unbiased prediction (BLUP) value among different years. PROC CORR was performed to calculate the correlation coefficients of bloom date among different years.
Broad-sense heritability (H2) was calculated using estimates for the offspring based on the following random linear model:
where Y ij is the phenotypic value of the jth offspring in ith year, μ is the mean bloom date, y i is the random effect of the ith year on the bloom date, g j is the random genotypic effect of jth progeny, and e ij is the model residual. H2 was calculated using the following equation: H2 = σ2 g / (σ2 g + σ2 e / n), where σ2 g is the genetic variance, σ2 e is the residual error, and n is the number of years.
Genotypic data
All individuals were genotyped using the RosBREED Illumina Infinium® cherry SNP array of 5696 SNP markers (Peace et al. 2012), and SNP genotypes were scored using the Genotyping Module of GenomeStudio Data Analysis software (Illumina Inc. 2010). For sour cherry, five genotypes were possible for each SNP when considering allele dosage: AAAA, AAAB, AABB, ABBB, and BBBB. SNP data from a diverse range of sweet cherry selections and seedlings (105 individuals) were included to aid in the determination of dosage as the two homozygous (AAAA and BBBB for sour cherry corresponding to AA and BB in sweet cherry) and balanced heterozygous (AABB for sour cherry corresponding to AB in sweet cherry) classes co-located. All samples that had GenCall Scores greater than 0.15 were used for clustering following the built-in algorithm “Gentrain2” (Teo et al. 2007). Manual editing was done to check and adjust clusters to the expected genotypic classes. Following the procedure outlined in Peace et al. (2012), SNP markers were classified into polymorphic (at least two clusters identified), monomorphic, unresolved polymorphic (ambiguous clusters), and failed markers when considering all individuals from the five populations. For each F1 population, only those SNP markers which were polymorphic between the two parents and segregated in the progeny were used in the QTL analysis. The genetic positions of all markers were determined by aligning and integrating their physical positions based on the peach genome v2.0 (Verde et al. 2017) with the sweet cherry ‘Regina’ × ‘Lapins’ SNP consensus linkage map (Klagges et al. 2013).
Genome-wide haplotype reconstruction (marker phase determination)
Genome-wide haplotype reconstruction within each full-sib tetraploid F1 population was performed using TetraOrigin software (Zheng et al. 2016). This process included two stages. The first step was parental linkage phasing to reconstruct the most probable parental haplotypes for each of the four homologous chromosomes for each of the eight Prunus chromosomes. The second step was ancestral inference to probabilistically reconstruct the offspring haplotypes conditional on the parental haplotypes. For each offspring, this resulted in an estimation of probability for each parental haplotype (n = 8), thereby indicating which four haplotypes the offspring had inherited from each of its parents.
QTL analysis
QTL analysis was performed for each SNP segregating within each population using the haplotype probability linear regression model of Bourke (2014) as illustrated by Rak et al. (2017). For each offspring, this model includes a variable for the probability of parental origin for each marker that was obtained from the genome-wide haplotype reconstruction. This variable had a value of either “0.25” or “0” (output from TetraOrigin) if the allele is present or absent in the offspring, respectively. Intermediate values between 0 and 0.25 were assigned where parental homolog origin was uncertain. The linear model was estimated using R version 3.1.3 (R Core Team 2015). One thousand permutations were used to determine the LOD thresholds for marker significance (Churchill and Doerge 1994). The QTL nomenclature used follows the guideline available at Genome Database for Rosaceae (GDR, www.rosaceae.org).
QTL haplotype effects
For all the QTL identified in this study, QTL haplotypes (alleles) were obtained from the results of genome-wide haplotype reconstruction. QTL haplotypes were identified that spanned a 5 Mbp region centered on the QTL peak and named using different letters. When a crossover took place within a QTL region, the haplotype was represented as missing data since it was unclear which parental haplotype contributed to the trait. To determine if QTL haplotypes had a positive or negative effect on bloom date, a two-tailed Student’s t test was performed to compare the trait means of the two groups: individuals with the haplotype versus individuals without the haplotype. The threshold for significance of the t tests was P < 0.05. A QTL haplotype was considered to be stably associated with bloom date if it was significant for at least two out of the 3 years in this study.
Results
Phenotype variation of bloom date
A wide range of variation for bloom date was observed for individuals of all populations (Table 1, Supplementary Fig. S1). Bloom date ranged from 279 to 430 GDD with a mean value of 340 [standard deviation (SD) = 28] when considering all 3 years. Bloom date for individuals was significantly correlated (r = 0.84–0.90) across years (Table 1). Of the parents, ‘Montmorency’, ‘UF’, and ‘RS’ exhibited later blooming than M172, 25-14-20, and 25-02-29 (Supplementary Fig. S1). Individuals which bloomed late (more than 370 GDD) were primarily found in Pop2, Pop4, and Pop5 (Table 1, Supplementary Fig. S1). Within populations, the ANOVA analysis revealed highly significant effects for the different genotypes (Table 1). In all five populations, the broad-sense heritabilities were high (0.89–0.96), indicating that much of the phenotypic variation in these populations is genetically controlled (Table 1).
Identification of QTLs for bloom date
A total of 2058 out of 5696 SNPs were used to construct the genetic map resulting in an average coverage of one marker every 0.3 cM, and the largest gap was 7.4 cM on G6 (Supplementary Table S1, Supplementary Fig. S2). The numbers of SNPs that were polymorphic between the parents for each population and therefore used to inform the QTL analyses for Pop1 to Pop5 were 1795, 1795, 1807, 1787, and 1856 SNPs, respectively.
Genome-wide QTL analysis using the haplotype probability linear regression model identified four bloom date QTLs on four different linkage groups: G1, G2, G4, and G5 (Table 2, Fig. 2, Supplementary Table S2). These four QTLs were only identified in three of the five populations, Pop1, Pop2, and Pop4, where the threshold LOD values of marker significance in each population were 3.5, 3.5, and 3.2, respectively. The lack of QTL identification in Pop3 and Pop5 is presumably due to the low progeny number for Pop5 and the concentration of bloom time exhibited by offspring in Pop3 (Supplementary Fig. S1). QTL qP-BD1.2m was identified at the same G1 region in two populations (Pop1 and Pop2). The peak positions of this QTL were not equivalent in the two populations and were ~ 2 Mb apart; however, as the intervals for the QTLs overlapped, the G1 QTLs were considered the same QTL (Fig. 2a, b). However, qP-BD1.2m was considered to be different from the G1 QTL reported by Castède et al. (2014, 2015) because the confidence intervals did not overlap (Supplementary Table S2), and both these QTLs were different from the weak QTL identified at ~ 44 cM in Pop4 (Fig. 2c). Therefore, we named the QTL identified in Pop1 and Pop2 as qP-BD1.2m rather than qP-BD1.1m. QTL qP-BD2.1m was identified in Pop4 with the peak SNP marker ss490549295 (Table 2, Fig. 2c), a position which was consistent with that identified in sweet cherry (Castède et al. 2014). QTL qP-BD4.1m was also identified in Pop4. The peak position for qP-BD4.1m was just outside the 95% confidence interval for the G4 QTL reported by Castède et al. (2014); however, the QTL confidence intervals overlapped, and therefore, they were considered the same QTL (Supplementary Table S2). QTL qP-BD5.1m was only identified in Pop1 (Table 2, Fig. 2a), and its physical location overlapped with the G5 bloom date QTL previously reported in sweet cherry (Castède et al. 2014) (Supplementary Table S2).
QTL positions along the eight Prunus linkage groups for bloom date identified in three F1 populations. a Pop1: M172 × 25-14-20 progeny. b Pop2: ‘Montmorency’ × 25-14-20 progeny. c Pop4: ‘Újfehértói Fürtös’ × ‘Surefire’ progeny. Permutation test was conducted 1000 times to determine the threshold (red lines) of significance. The resulting threshold values were 3.5, 3.5, and 3.2 for Pop1, Pop2, and Pop4, respectively
Haplotype identification for the bloom date QTLs
The target regions which were used to construct QTL haplotypes for the four bloom date QTLs identified ranged from 4.873 to 5.269 Mbp (Supplementary Table S2). Haplotypes constructed for these four QTL regions on G1, G2, G4, and G5 consisted of 50, 55, 39, and 50 SNPs, respectively. For qP-BD1.2m, 13 haplotypes were built for the eight parents and designated as haplotypes “a” through “m” (Table 3, Supplementary Fig. S3). For qP-BD2.1m, 17 haplotypes were identified for the eight parents and designated as haplotypes “a” through “q” (Table 3, Supplementary Fig. S4). For qP-BD4.1m, 16 haplotypes were identified for eight parents and designated as “a” through “p” (Table 3, Supplementary Fig. S5). Lastly, for qP-BD5.1m, 15 haplotypes were built for the eight parents and were designated as haplotypes “a” through “o” (Table 3, Supplementary Fig. S6). In total, 32 genotypes were deduced for the four QTLs for the eight parents (Table 3). Of these 32 genotypes, 29 were tetra-allelic and 3 were tri-allelic. As an example, SNP marker composition for the haplotypes spanning qP-BD2.1m is illustrated in Fig. 1b using the smallest number of markers needed to differentiate the haplotypes.
Due to the pedigree relationships among the parents, some of the haplotypes were known to be identical by descent (IBD). This is illustrated using the haplotypes for qP-BD2.1m, where haplotype G2-d in ‘RS’ is IBD to haplotype G2-d in 25-02-29 (Fig. 1a). Haplotype G2-d is also present in ‘Montmorency’ and ‘Surefire’, but despite having the same SNP genotype, it is not possible to conclude that their haplotype G2-d is IBD with that present in 25-02-29 and ‘RS’.
Genetic effects of individual QTL haplotypes on bloom date
A total of 14 haplotypes constructed for the four QTLs were significantly associated with bloom date within individual populations (Table 4, Fig. 3). These 14 QTL haplotypes explained 10.1–27.9% of bloom date variation within individual populations, corresponding to an effect on bloom date ranging from − 1.4 to 2.8 days (Table 4).
Four of the 13 qP-BD1.2m haplotypes identified (G1-a, -c, -d, and -e) were significantly associated with bloom date within individual populations (Table 4, Fig. 3a). The presence of G1-c derived from 25-02-29, ‘Montmorency’, and M172 was associated with late bloom date in both Pop1 and Pop2. In Pop1 and Pop2, the bloom date delays were 15 and 24 GDD, respectively, and the percentages of phenotypic variation explained were 16.8 and 20.9%, respectively. In contrast, the presence of G1-d also derived from 25-02-29 and ‘Montmorency’ was associated with early bloom date in Pop1 and Pop2, with the maximum effect in Pop2. In this population, G1-d had an early blooming effect of 30 GDD and the percent phenotypic variation explained was 26.1%. G1-a and G1-e derived from 25-02-29 and M172, respectively, were also associated with earlier bloom date, but their effects were less than that of G1-d. Haplotypes G1-c and G1-e that were associated with late and early blooming, respectively, were also segregating in Pops 3, 4, and 5; however, the haplotype effects were not significant in these populations (Supplementary Table S3).
Three of the 17 qP-BD2.1m haplotypes (G2-c, -d, and -j) were significantly associated with bloom date within individual populations (Table 4, Fig. 3b). The presence of G2-c derived from 20-02-29 in Pop1 was significantly associated with late bloom date; however, G2-c was not significantly associated with bloom date in the other two populations for which 25-02-29 was a parent (Pop2 and 3). The presence of G2-d was associated with early bloom date in both Pop1 and Pop4 (Fig. 3b), and for these two populations, three of the four parents each had one copy of G2-d. G2-j derived from ‘UF’ was significantly associated with late blooming in Pop4, and the bloom delay associated with the presence/absence of G2-j was a mean delay of 25 GDD (Table 4, Fig. 3b). G2-j was also present in 25-14-20, and it is IBD since ‘UF’ is one of the parents of 25-14-20 (Fig. 1a); however, G2-j contributed by 25-14-20 in Pop3 was not significantly associated with bloom date (Supplementary Table S3).
Four of the 16 qP-BD4.1m haplotypes (G4-c, -e, -i, and -k) were significantly associated with bloom date within individual populations (Table 4). The presence of G4-e, G4-i, and G4-k was significantly associated with late blooming in Pop1, Pop2, and Pop4, respectively (Fig. 3c). G4-k had the largest effect on bloom date delay (+ 38 GDD, 2.8 days) compared to the other late blooming haplotypes across all the other QTLs. Both parents of Pop4 (‘UF’ and ‘Surefire’) carried this very late G4-k haplotype, which was consistent with their late bloom dates, and with Pop4 having a high proportion of later blooming individuals (Table 3). Despite qP-BD4.1m not being significant in Pop3 using the haplotype probability linear regression model, G4-c derived from 25-02-29 and segregating in Pop3 was significantly associated with early bloom time.
Three of the 15 haplotypes identified for qP-BD5.1m (G5-c, -e, and -g) were significantly associated with bloom time in only one population, Pop1 (Table 1). Only G5-g was associated with late bloom (Table 4, Fig. 3d). Additional significant differences were not found for G5-c and G5-g despite these haplotypes segregate in three and two other populations, respectively (Supplementary Table S3).
Additive genetic effects of QTL haplotypes on bloom date
Among all the significant haplotypes, the presence of G1-c, G2-j, and G4-k had the largest effects associated with late bloom date. None of the parents had more than one dose of any of these late bloom haplotypes (Table 3); however, in two populations, both the female and male parent shared the same late bloom date haplotype; therefore, a dosage effect could be investigated in the offspring. In Pop1, both parents had one G1-c haplotype, and therefore, it was possible for progeny to inherit zero, one, or two G1-c haplotypes. The mean bloom date was significantly later for Pop1 progeny with two G1-c haplotypes compared to progeny with just one G1-c (Fig. 4a). Most of the latest blooming progeny (80%) had at least one G1-c; however, some also had no G1-c (Fig. 4b). Dosage effects of haplotypes on bloom date were also observed in Pop4 as the same haplotype, G4-k, was shared between the two parents ‘Újfehértói Fürtös’ and ‘Surefire’. Offspring with two doses of G4-k had a later mean bloom date compared to individuals with zero or one dose (Fig. 4c). Moreover, almost all the offspring with two doses of G4-k had very late bloom dates, exceeding more than 350 GDD (Fig. 4d).
Dosage effects of the G1 QTL (qP-BD1.2m) haplotype c within Pop1 and the G4 QTL (qP-BD4.1m) haplotype k within Pop4. Mean comparisons of bloom date (BD, heat unit accumulation) among individuals with different dosages of haplotype (a, c). Frequency distribution of bloom date among individuals with different dosages of haplotype (b, d). Bars indicate standard errors
Significant additive effects were also identified when two late blooming haplotypes from different QTL were combined in offspring within Pop1, Pop2, and Pop4 (Fig. 5). In Pop1, those offspring with late bloom haplotypes from qP-BD1.2m and qP-BD4.1m (G1-c and G4-e) had a significantly later mean bloom date compared to those offspring with none or one of the late bloom haplotypes. A similar effect was observed in Pop4 for two different late bloom haplotypes, one each from qP-BD2.1m and qP-BD4.1m. For Pop2, those offspring that had G4-i had a significantly later bloom date if they also had G1-c.
Within population mean comparisons for bloom date (presented as growing degree days) based on the presence or absence of two different QTL haplotypes in each progeny individual. Comparisons include the late bloom date haplotypes for qP-BD4.1m (G4-k, -e, and -i) with the late bloom date haplotypes from qP-BD2.1m (G2-j) and qP-BD1.2m (G1-c). Within each population, means that were significantly different (P < 0.05) are indicated by different letters
Discussion
QTL detection in tetraploid sour cherry
Compared to diploid or auto-tetraploid species, QTL mapping studies in sour cherry have been hampered due to its lack of strict bivalent pairing and unbalanced sub-genome chromosome segregation during meiosis. Fortunately, the probabilistic approach of TetraOrigin software facilitates the deduction of the linkage phase of high-density SNPs for both parents and offspring. TetraOrigin software proved robust for complex chromosome pairing, various marker segregation types, flaws of genetic map positions, offspring dosage errors, and missing data (Zheng et al. 2016). In addition, a QTL was detected in Pop2 which only consisted of 53 offspring, and two QTLs were detected in Pop4 which consisted of 76 offspring; an important consideration given the space and cost requirements for maintaining tree plantings. The use of the multiple populations also allowed QTL validation concurrent with QTL discovery as qP-BD1.2m was significant in two populations and haplotypes for qP-BD2.1m and qP-BD4.1m were significant in two and four populations, respectively.
Of the four QTL identified in this study, all were previously identified in cherry and other Prunus species (Dirlewanger et al. 1999; Wang et al. 2000; Ballester et al. 2001; Verde et al. 2002; Quilot et al. 2004; Fan et al. 2010; Campoy et al. 2011; Dirlewanger et al. 2012; Castède et al. 2014). Among these QTLs, the one with the largest effect was on G4 (qP-BD4.1m) which is consistent with previous findings, including findings from two bi-parental F1 populations in sweet cherry. In a ‘Regina’ × ‘Lapins’ sweet cherry population, the QTL on G4 explained 24–47% of the bloom date variance (Dirlewanger et al. 2012), and in a second population (‘Regina’ × ‘Garnet’), the G4 QTL was significantly associated with a 3.8-day bloom date difference (Castède et al. 2014). The second largest effect was observed for qP-BD1.2m. This QTL is likely the same as that identified in sour cherry on G1 by Wang et al. (2000) based on pedigree relationships among the parents. The source of the G1 bloom date QTL identified by Wang et al. (2000) was ‘Erdi Botermo’, and this cultivar is the parent and half-sib of 25-02-29 and M172, respectively, two parents from whom significant qP-BD1.2m haplotypes were identified. Although 25-02-29 was a parent for three populations (Pop1, Pop2, and Pop3), this QTL was only identified in two populations (Pop1 and Pop2). This suggests that the other parent of Pop3 may have had an impact on the ability to detect this QTL. QTLs qP-BD2.1m and qP-BD5.1m are likely the same QTLs identified by Castède et al. (2014) due to overlapping QTL intervals on G2 and G5. However, our study did not identify minor bloom time QTL that were also identified in sweet cherry by Castède et al. (2014) on G1, G3, G6, G7, and G8. Taken together, although there are some major effect QTL for bloom date in cherry, the presence of at least one QTL per linkage group indicates that bloom date is under polygenic control.
Haplotype effects on bloom date and their breeding application
One limitation of this study was that the QTL haplotypes were based on SNP marker composition spanning the QTL region and not variation in underlying genes. Therefore, it is possible that haplotypes assigned different letters over-represent the number of functional alleles. The finding that an overwhelming number of the deduced QTL genotypes (91%) were tetra-allelic suggests that this is the case. However, if haplotypes which were known to have identical functional genes could have been condensed, it may have resulted in the identification of more significant differences. In contrast, it is also possible that haplotypes with the same SNP marker composition and therefore assigned the same letter are not IBD and do not have identical functional genes. For example, G1-d which is associated with late bloom time when derived from ‘Montmorency’ and 25-02-29 is also present in ‘UF’ and its grandchild 25-14-20 but not significantly associated with bloom time in ‘UF’ or 25-14-20 offspring. In this example, either G1-d represents different functional alleles in these plant materials or other functional alleles segregating in these populations mask the effect of this haplotype. This illustrates the value of defining haplotype effects using multiple parents and progeny populations, as the significance of bloom time haplotypes is highly dependent on genetic background.
The three most important late bloom date haplotypes (G1-c, G2-j, and G4-k) were demonstrated to have additive effects on delaying bloom time in the populations studied, both within and across QTLs. Gene dosage (additive effects for multiple copies of the same allele) has been shown to affect structural gene expression and the related phenotype in diploid maize and tetraploid potato (Birchler and Newton 1981; Guo et al. 1996; Iovene et al. 2013). In sour cherry, despite the lack of knowledge of the underlying genes, the evidence of dosage suggests that it may be possible to further delay bloom time with the presence of three or even four doses of the late bloom date alleles. Combining late blooming haplotypes across QTL also resulted in increased bloom delay. Collectively, these results illustrate that pyramiding late blooming haplotypes both within and across QTLs would be an effective strategy to obtain later blooming offspring.
To breed late blooming sour cherry cultivars, it would be most beneficial to initially focus on qP-BD4.1m, as G4-k has the largest effect on bloom date. Development of SSR markers located within the favorable haplotype could be used for marker-assisted selection. This technique has been successfully used to develop DNA markers in tetraploid sour cherry for cherry leaf spot resistant (Stegmeir et al. 2014b) and red fruit color (Stegmeir et al. 2015). However, decisions to use DNA information for seedling selection would need to be informed by the effect of the QTL regions on other important target traits. For example, qP-BD4.1m is likely linked with a major QTL for maturity date identified in other Prunus species (Sánchez-Pérez et al. 2007; Dirlewanger et al. 2012; Pirona et al. 2013). Likewise, qP-BD2.1m is located in a QTL “hotspot” that includes QTLs for fruit size and firmness (Zhang et al. 2010; De Franceschi et al. 2013; Cai et al. 2017). Given that the desired cultivar would be a late blooming tree with firm large fruit, studies are underway to genetically dissect these chromosome regions, including the assignment of trait effects to functional alleles, and search for the underlying genes.
References
Andersen RL, Brown SK, Way RD, Livermore KG, Terry DE (1999) Sour cherry cultivar named ‘Surefire’. U.S. plant patent 11108P
Anderson JL, Seeley SD (1993) Bloom delay in deciduous fruits. Hortic Rev 15:97–144
Ballester J, Socias I, Company R, Arús P, deVicente MC (2001) Genetic mapping of a major gene delaying blooming time in almond. Plant Breed 120(3):268–270. https://doi.org/10.1046/j.1439-0523.2001.00604.x
Beaver JA, Iezzoni AF (1993) Allozyme inheritance in tetraploid sour cherry (Prunus cerasus L.) J Am Soc Hortic Sci 118:873–877
Birchler JA, Newton KJ (1981) Modulation of protein levels in chromosomal dosage series of maize: the biochemical basis of aneuploid syndromes. Genetics 99(2):247–266
Bourke PM (2014) QTL analysis in polyploids: model testing and power calculations for an autotetraploid. In: Minor thesis (MSc) plant breeding, University and Research Centre, Wageningen
Cai L, Voorrips RE, van de Weg E, Peace P, Iezzoni A (2017) Genetic structure of a QTL hotspot on chromosome 2 in sweet cherry indicates positive selection for favorable haplotypes. Mol Breed 37(7):85. https://doi.org/10.1007/s11032-017-0689-6
Campoy JA, Ruiz D, Egea J, Rees DJG, Celton JM, Martinez-Gomez P (2011) Inheritance of flowering time in apricot (Prunus armeniaca L.) and analysis of linked quantitative trait loci (QTLs) using simple sequence repeat (SSR) markers. Plant Mol Biol Rep 29(2):404–410. https://doi.org/10.1007/s11105-010-0242-9
Castède S, Campoy JA, García JQ, Le Dantec L, Lafargue M, Barreneche T, Wenden B, Dirlewanger E (2014) Genetic determinism of phenological traits highly affected by climate change in Prunus avium: flowering data dissected into chilling and heat requirements. New Phytol 202(2):703–715. https://doi.org/10.1111/nph.12658
Castède S, Campoy JA, Le Dantec L, Quero-García J, Barreneche T, Wenden B, Dirlewanger E (2015) Mapping of candidate genes involved in bud dormancy and flowering time in sweet cherry (Prunus avium). PLoS One 10(11):e0143250. https://doi.org/10.1371/journal.pone.0143250
Churchill GA, Doerge RW (1994) Empirical threshold values for quantitative trait mapping. Genetics 138(3):963–971
De Franceschi P, Stegmeir T, Cabrera A, van der Knaap E, Rosyara UR, Sebolt AM, Dondini L, Dirlewanger E, Quero-Garcia J, Campoy JA, Iezzoni AF (2013) Cell number regulator genes in Prunus provide candidate genes for the control of fruit size in sweet and sour cherry. Mol Breed 32(2):311–326. https://doi.org/10.1007/s11032-013-9872-6
Dirlewanger E, Moing A, Rothan C, Svanella L, Pronier V, Guye A, Plomion C, Monet R (1999) Mapping QTLs controlling fruit quality in peach (Prunus persica (L.) Batsch). Theor Appl Genet 98(1):18–31. https://doi.org/10.1007/s001220051035
Dirlewanger E, Graziano E, Joobeur T, Garriga-Caldere F, Cosson P, Howard W, Arus P (2004) Comparative mapping and marker-assisted selection in Rosaceae fruit crops. Proc Natl Acad Sci U S A 101(26):9891–9896. https://doi.org/10.1073/pnas.0307937101
Dirlewanger E, Claverie J, Iezzoni AF, Wunsch A (2009) Sweet and sour cherry: linkage maps, QTL detection and marker assisted selection. In: Folta KM, Gardiner SE (eds) Genetics and Genomics of Rosaceae. Springer, New York, pp 291–313. https://doi.org/10.1007/978-0-387-77491-6_14
Dirlewanger E, Quero-García J, Le Dantec L, Lambert P, Ruiz D, Dondini L, Illa E, Quilot-Turion B, Audergon J-M, Tartarini S, Letourmy P, Arús P (2012) Comparison of the genetic determinism of two key phenological traits, flowering and maturity dates, in tree Prunus species: peach, apricot and sweet cherry. Heredity 109(5):280–292. https://doi.org/10.1038/hdy.2012.38
Fan S, Bielenberg DG, Zhebentyayeva TN, Reighard GL, Okie WR, Holland D, Abbott AG (2010) Mapping quantitative trait loci associated with chilling requirement, heat requirement and bloom date in peach (Prunus persica). New Phytol 185(4):917–930. https://doi.org/10.1111/j.1469-8137.2009.03119.x
Guo M, Davis D, Birchler JA (1996) Dosage effects on gene expression in a maize ploidy series. Genetics 142(4):1349–1355
Hackett CA, Milne I, Bradshaw JE, Luo Z (2007) TetraploidMap for windows: linkage map construction and QTL mapping in autotetraploid species. J Hered 98(7):727–729. https://doi.org/10.1093/jhered/esm086
Iezzoni AF (1996) Sour cherry cultivars: objectives and methods of fruit breeding and characteristics of principal commercial cultivars. In: Webster AD, Looney NE (eds) Cherries: Crop physiology, production and uses. University Press, Cambridge, pp 223–241
Illumina Inc. (2010) GenomeStudio genotyping module v1.0, User Guide. Illumina Inc., San Diego
Iovene M, Zhang T, Lou Q, Buell CR, Jiang J (2013) Copy number variation in potato—an asexually propagated autotetraploid species. Plant J 75(1):80–89. https://doi.org/10.1111/tpj.12200
Klagges C, Campoy JA, Quero-Garcia J, Guzman A, Mansur L, Gratacos E, Silva H, Rosyara UR, Iezzoni A, Meisel LA, Dirlewanger E (2013) Construction and comparative analyses of highly dense linkage maps of two sweet cherry intra-specific progenies of commercial cultivars. PLoS One 8(1):e54743. https://doi.org/10.1371/journal.pone.0054743
NASS (2012) National Agricultural Statistics Service. http://www.nass.usda.org
Peace C, Bassil N, Main D, Ficklin S, Rosyara UR, Stegmeir T, Sebolt A, Gilmore B, Lawley C, Mockler TC, Bryant DW, Wilhelm L, Iezzoni A (2012) Development and evaluation of a genome-wide 6K SNP array for diploid sweet cherry and tetraploid sour cherry. PLoS One 7(12):e48305. https://doi.org/10.1371/journal.pone.0048305
Pirona R, Eduardo I, Pacheco I, Da Silva Linge C, Miculan M, Verde I, Tartarini S, Dondini L, Pea G, Bassi D, Rossini L (2013) Fine mapping and identification of a candidate gene for a major locus controlling maturity date in peach. BMC Plant Biol 13(1):166. https://doi.org/10.1186/1471-2229-13-166
Quilot B, Wu BH, Kervella J, Genard M, Foulongne M, Moreau K (2004) QTL analysis of quality traits in an advanced backcross between Prunus persica cultivars and the wild relative species P. davidiana. Theor Appl Genet 109(4):884–897. https://doi.org/10.1007/s00122-004-1703-z
R Core Team (2015) R: a language and environment for statistical computing. R Foundation for Statistical Computing, Vienna URL http://www.R-project.org/
Rak K, Bethke PC, Palta JP (2017) QTL mapping of potato chip color and tuber traits within an autotetraploid family. Mol Breed 37(2):15. https://doi.org/10.1007/s11032-017-0619-7
Sánchez-Pérez W, Howad F, Dicenta P, Arús, P, Martínez-Gómez P (2007) Mapping major genes and quantitative trait loci controlling agronomic traits in almond. Plant Breed 126(3):310–318. https://doi.org/10.1111/j.1439-0523.2007.01329.x
Sánchez-Pérez R, Del Cueto J, Dicenta F, Martínez-Gómez P (2014) Recent advancements to study flowering time in almond and other Prunus species. Front Plant Sci 5:334
Schuster M, Wolfram B (2005) Sour cherry breeding at Dresden-Pillnitz. Acta Hortic (667):127–130. https://doi.org/10.17660/ActaHortic.2005.667.17
Stegmeir T, Sebolt A, Iezzoni A (2014a) Phenotyping protocol for sour cherry (Prunus cerasus L.) to enable a better understanding of trait inheritance. J Am Pomol Soc 68:40–47
Stegmeir T, Schuster M, Sebolt A, Rosyara U, Sundin GW, Iezzoni A (2014b) Cherry leaf spot resistance in cherry (Prunus) is associated with a quantitative trait locus on linkage group 4 inherited from P. canescens. Mol Breed 34(3):927–935. https://doi.org/10.1007/s11032-014-0086-3
Stegmeir T, Cai L, Basundari FRA, Sebolt AM, Iezzoni AF (2015) A DNA test for fruit color in tetraploid sour cherry (Prunus cerasus L.) Mol Breed 35(7):149. https://doi.org/10.1007/s11032-015-0337-y
Teo YY, Inouye M, Small KS, Gwilliam R, Deloukas P, Kwiatkowski DP, Clark TG (2007) A genotype calling algorithm for the Illumina Bead Array platform. Bioinformatics 23(20):2741–2746. https://doi.org/10.1093/bioinformatics/btm443
Verde I, Quarta R, Cedrola C, Dettori MT (2002) QTL analysis of agronomic traits in a BC1 peach population. Acta Hortic (592):291–297. https://doi.org/10.17660/ActaHortic.2002.592.41
Verde I, Jenkins J, Dondini L, Micali S, Pagliarani G, Vendramin E, Paris R, Aramini V, Gazza L, Rossini L, Bassi D, Troggio M, Shu S, Grimwood J, Tartarini S, Dettori MT, Schmutz J (2017) The Peach v2.0 release: high-resolution linkage mapping and deep resequencing improve chromosome-scale assembly and contiguity. BMC Genomics 18(1):225. https://doi.org/10.1186/s12864-017-3606-9
Wang D, Karle R, Iezzoni AF (2000) QTL analysis of flower and fruit traits in sour cherry. Theor Appl Genet 100(3-4):535–544. https://doi.org/10.1007/s001220050070
Zhang G, Sebolt A, Sooriyapathirana S, Wang D, Bink M, Olmstead J, Iezzoni A (2010) Fruit size QTL analysis of an F1 population derived from a cross between a domesticated sweet cherry cultivar and a wild forest sweet cherry. Tree Genet Genomes 6(1):25–36. https://doi.org/10.1007/s11295-009-0225-x
Zheng C, Voorrips RE, Jansen J, Hackett CA, Ho J, Bink MC (2016) Probabilistic multilocus haplotype reconstruction in outcrossing tetraploids. Genetics 203(1):119–131. https://doi.org/10.1534/genetics.115.185579
Acknowledgements
This project was supported in part by the USDA-NIFA-Specialty Crop Research Initiative project, RosBREED: Enabling marker-assisted breeding in Rosaceae (2009-51181-05808), and RosBREED 2: Combining disease resistance with horticultural quality in new rosaceous cultivars (2014-51181-22378).
Author information
Authors and Affiliations
Corresponding author
Ethics declarations
Conflict of interest
The authors declare that they have no conflict of interest.
Data Archiving Statement
The linkage map constructed and the QTL regions identified will be made available at the Genome Database for Rosaceae (www.rosaceae.org/publication_datasets) under accession number tfGDR 1033.
Additional information
Communicated by E. Dirlewanger
Electronic supplementary material
Supplementary Table S1
(DOCX 15 kb)
Supplementary Table S2
(DOCX 17 kb)
Supplementary Table S3
(DOCX 46 kb)
Supplementary Fig. S1
(DOCX 165 kb)
Supplementary Fig. S2
(DOCX 61 kb)
Supplementary Fig. S3
(XLSX 20 kb)
Supplementary Fig. S4
(XLSX 22 kb)
Supplementary Fig. S5
(XLSX 17 kb)
Supplementary Fig. S6
(XLSX 23 kb)
Rights and permissions
About this article
Cite this article
Cai, L., Stegmeir, T., Sebolt, A. et al. Identification of bloom date QTLs and haplotype analysis in tetraploid sour cherry (Prunus cerasus). Tree Genetics & Genomes 14, 22 (2018). https://doi.org/10.1007/s11295-018-1236-2
Received:
Revised:
Accepted:
Published:
DOI: https://doi.org/10.1007/s11295-018-1236-2