Abstract
Soybean (Glycine max L. Merr.) is a major crop and a leading source of protein meal and edible oil worldwide. Plant height (PHT), lodging (LDG), and days to maturity (MAT) are three important agronomic traits that influence the seed yield of soybean. The objective of this study was to map quantitative trait loci (QTL) for PHT, LDG and MAT using a high density SNP map of a recombinant inbred line soybean mapping population. With single factor analysis of variance six, four, and three QTL were identified across environments for PHT, LDG, and MAT, respectively. Several QTL for each trait were also detected by composite interval mapping with high confidence. Two and one QTL for PHT and LDG, respectively were novel QTL identified in this study. Additionally, most QTL identified in the present study are flanked by two or more SNP markers that are closely linked to each QTL. The SNP markers identified to be closely linked to each QTL in this study are valuable for marker assisted selection (MAS) of the QTL by interested soybean breeding programs. Thus, this study clearly advances the knowledge on genetic controls of plant height, lodging and maturity in soybean, and identifies more efficient and reliable markers for MAS for these traits than those currently exist.
Similar content being viewed by others
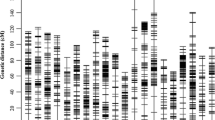
Avoid common mistakes on your manuscript.
Introduction
Soybean (Glycine max L. Merr.) is a major crop and a leading source of protein meal and edible oil worldwide (Wilson 2004; Zhang et al. 2004). Plant height (PHT), lodging (LDG), and days to maturity (MAT) are three important agronomic traits that influence the seed yield of soybean (Lee et al. 1996a, b; Chapman et al. 2003; Panthee et al. 2007). All three traits are quantitative traits, implying that each trait is controlled by multiple genes or quantitative trait loci (QTL) (Lee et al. 1996a, b; Orf et al. 1999; Panthee et al. 2007; Liu et al. 2013). In most previously published studies on mapping QTL conditioning PHT, LDG and MAT in soybean, low to moderate resolution genetic maps of RFLP, AFLP and/or SSR markers were used. Lee et al. (1996a, b) used maps with 137 to 153 RFLP makers to map PHT, LDG and MAT in two soybean populations. Mansur et al. (1996), Orf et al. (1999), Chapman et al. (2003), and Zhang et al. (2004) used combinations of RFLP, AFLP and/or SSR markers for mapping these traits. The genetic maps used in all these studies had incomplete coverage of the soybean genome with some large gaps in the maps. Liu et al. (2013) has recently reported using 313 single nucleotide polymorphism (SNP) markers along with 167 SSR markers to map QTL controlling PHT in soybean.
Following the completion of Williams82 soybean genome sequence (Schmutz et al. 2010), millions of SNPs and insertion/deletions were identified through resequencing of tens of wild and cultivated soybean genotypes (Kim et al. 2010; Lam et al. 2010; Li et al. 2013). Hyten et al. (2010) published a high density consensus map of soybean where a total of 5,500 markers were integrated, including 3,792 SNPs. They also developed a “Universal Soy Linkage Panel” (USLP 1.0) of 1,536 robust bi-allelic SNP array for automated high-throughput assay on Illumina GoldenGate Platform (Hyten et al. 2010). More recently, Illumina Infinium BeadChip containing over 50 K SNPs was developed and evaluated for hundreds of wild and cultivated soybean genotypes (Song et al. 2013). Due to high abundance and automatable high-throughput genotyping, SNPs are now widely used in plants for construction of genetic map and linkage analysis (Huo et al. 2011; Jun et al. 2012a; Nguyen et al. 2012), comparative genomics (Hufford et al. 2012), genome-wide association mapping (Hwang et al. 2014), and genomic predictions (Crossa et al. 2013) for many crops.
Soybean SNP markers have been recently used to map QTL for a number of traits, including resistance to biotic or abiotic stresses (Kim et al. 2011; Jun et al. 2012a, b; Nguyen et al. 2012; Ellis et al. 2012; Lee et al. 2013a, b, 2014), seed yield (Kim et al. 2012; Liu et al. 2013), seed protein/oil (Hwang et al. 2014), and leaf traits (Jun et al. 2014). However, soybean SNP markers have so far been underutilized for mapping many important agronomic traits in soybean, including PHT, LDG and MAT. Using the USLP 1.0 of 1,536 SNP markers, a dense genetic map of 516 SNP markers were developed for a F7-derived recombinant inbred line (RIL) mapping population from a cross of ‘Wyandot’ x PI 567301B, and was used to map QTL for resistance to soybean aphid (Jun et al. 2012a) and several leaf traits (leaf area, leaf shape, and specific leaf weight) in soybean (Jun et al. 2014). The objective of our study was to map QTL for PHT, LDG and MAT in the Wyandot x PI 567301B population in two different environments and across environments using the map of SNP markers.
Materials and methods
Plant materials
A recombinant inbred line (RIL) population developed from a cross between Wyandot and PI 567301B has been used in previous studies for mapping QTL for soybean aphid resistance, powdery mildew resistance, and leaf traits (Jun et al. 2012a, b, 2014). Wyandot is a maturity group (MG) II (2.9) soybean variety developed in Ohio. PI 567301B is a Chinese germplasm in MG IV, which was initially identified as a source of soybean aphid resistance. Both parents have the indeterminate growth habit. Wyandot is a high-yielding cultivar adapted to the growing conditions in Ohio, but PI 567301B is not. During the development of the mapping population, it was noticed that PI 567301B had taller plants with greater tendency to lodging and had more secondary and tertiary branches compared to Wyandot (data not shown).
Experimental design and collection of phenotypic data
Field experiments were conducted as described in Jun et al. (2014). Briefly, a randomized complete block design was used for each year with two replications. Only 91 of the 94 F7-derived RILs used for generating the SNP map were available for phenotypic evaluation. In 2011 and 2012, field evaluations of agronomic traits were conducted by planting three seeds in a hole and then thinning to one plant per hole by hand at the V1 growth stage (Fehr et al. 1971). A 0.9-m single row plot of nine plants per RIL was grown with a distance of 10-cm between plants with 0.9-m row spacing. Pre-emergence and post-emergence herbicides were applied both years for weed control and no fertilizers were added to the field in either year. Due to drought condition during initial growth stages in 2012, the field was irrigated at the V2 and V8 stages of growth, but no irrigation water was applied during 2011 with normal growing conditions. Data were collected for PHT, LDG and MAT in both years. The average length from ground to the terminal bud of three plants in the middle of each row at maturity was measured for PHT. LDG was rated on a scale of one (all plants erect) to five (all plants prostrate on ground) at maturity. MAT was the number of days from date of planting to the R8 growth stage, at which 95 % of pods turned to mature pod colors (Fehr et al. 1971).
SNP genotyping and construction of genetic linkage map
DNA extraction and SNP marker genotyping was conducted as described in Jun et al. (2012a). Briefly, 94 RILs of Wyandot x PI 567301B population and the two parents were genotyped with the USLP 1.0 containing 1,536 SNP evenly distributed over the twenty chromosomes of soybean. A framework genetic map was constructed using the Kosambi mapping function with JoinMap® 4 (Van Ooijen 2006). Linkage groups were determined at the logarithm of odd (LOD) of 3.0 and a maximum marker distance of 40 centiMorgan (cM).
Statistical analyses
Single marker-trait association was initially analyzed for each trait and year using PROC GLM in SAS9.3 (SAS Institute, Cary, NC). Subsequently, composite interval mapping was also used to identify QTL associated with PHT, LDG, and MAT via MapQTL®5 (Van Ooijen 2004). LOD threshold was determined at 95 % of significance level by a 1000-permutation test (Churchill and Doerge 1994). QTL with a LOD score greater than the empirical LOD threshold were declared significantly associated with the traits. The averaged data over two replications within each year was used for detection of QTL for each year, and 2-year averaged values of each trait were used for QTL analyses across years. Broad-sense heritability (H 2) on a line-mean basis for PHT, LDG, and MAT was estimated by the equation \(H^{2} = {{\sigma_{\text{g}}^{2} } \mathord{\left/ {\vphantom {{\sigma_{\text{g}}^{2} } {\left( {\sigma_{\text{g}}^{2} + \sigma_{\text{ge}}^{2} /{\text{n}} + \sigma_{\text{e}}^{2} /{\text{nr}}} \right)}}} \right. \kern-0pt} {\left( {\sigma_{\text{g}}^{2} + \sigma_{\text{ge}}^{2} /{\text{n}} + \sigma_{\text{e}}^{2} /{\text{nr}}} \right)}}\) where \(\sigma_{\text{g}}^{2}\) is genotypic variance, \(\sigma_{\text{ge}}^{2}\) is variance due to genotype × environment, \(\sigma_{\text{e}}^{2}\) is error variance, n is the number of environments, and r is the number of replications within environment (Nyquist and Barker 1991). PROC VARICOMP was used to obtain the variance components in SAS9.3. Pearson’s correlation among the three traits was calculated using PROC CORR in SAS9.3.
Results
Construction of a genetic map
A total of 516 SNP loci were integrated into 32 linkage groups with a total length of 2,005.3 cM (Jun et al. 2012a). From the 516 SNP, 399 unique loci were generated in the map, 15 to 30 loci of which were anchored per chromosome (Chrm.) with average marker density of approximately 5 cM (Jun et al. 2012a).
Phenotypic variation of PHT, LDG, and MAT
Wyandot and PI 567301B showed significant variation in PHT, LDG, and MAT for both years and averaged across years (P < 0.0001), and 91 F7-derived RILs segregated normally (Fig. 1). Averaged over years, PHT of PI 567301B was almost double than Wyandot and the RILs segregated widely ranging between 69 and 164 cm (Fig. 1). Wyandot and PI 567301B across years differed by a score of 3.3 in LDG and the LDG scores of RILs ranged from 1.0 to 5.0 (Fig. 1). MAT of PI 567301B (146) was delayed by 15 days compared to Wyandot (131), and the range of MAT among the RILs was approximately 45 days. Eleven RILs had significant transgressive segregation for earlier or later maturity than the early parent Wyandot or the late parent PI 567301B, respectively by Fisher’s least significant difference (LSD) test (LSD = 6.5, P < 0.05). Four RILs were delayed 6.5 days or longer than the late maturity parent PI 567301B, while seven RILs matured 6.5 or more days earlier than Wyandot.
Frequency distribution of plant height, lodging, and maturity in 2011, 2012, and the 2-year averaged in the 91 RILs of Wyandot × PI 567301B population. Mean values of the two parents were indicated by arrows with the initials W and P, corresponding Wyandot and PI 567301B. The same color-code was used to present years in arrows and bars. Least significant difference (P < 0.05) for each trait and each year was presented by bars above each histogram. A Plant height; B Lodging; C Maturity
For parental checks, PHT and LDG scores were similar between the 2 years, whereas MAT was significantly longer in 2012 compared to 2011. Among the 91 RILs, PHT were moderately correlated with LDG and MAT in both years, with the correlation coefficients (r) of 0.36 and 0.47 in 2011, and 0.38 and 0.53 in 2012, respectively (P < 0.001). LDG was moderately correlated with MAT in 2012 (r = 0.45, P < 0.001), but not significant in 2011. Broad-sense heritability (H 2) of the traits was high for PHT (0.83) and MAT (0.94), while H 2 of LDG was moderate (0.54).
QTL identified using analysis of variance (ANOVA) and composite interval mapping (CIM)
QTL were initially identified via single-factor ANOVA using the trait data of each year and averaged over the 2 years, followed by CIM. Table 1 lists the SNP markers significantly (P < 0.01) linked with the trait averaged across years and these markers were also significant at P < 0.05 in each year. Thus the loci reported in Table 1 can be considered stable across environments. Table 2 presents the QTL identified by CIM at above threshold LOD values using two-year averages of the traits. Additional SNP markers significant at P < 0.05 for the ANOVA are listed in Supplementary Table 1. This threshold may be considered relatively low to declare QTL in this study, however, it is still important to report these markers because the QTL in Supplementary Table 1 are located in genomic regions reported for the respective traits in previous studies.
Six QTL were identified for PHT, explaining 7 to 18 % of phenotypic variance (PV) across years. PHT-13 (named by the combination of trait and Chrm.) was the largest effect QTL associated with PHT and significant in both years and averaged across years at P < 0.01. PHT-11 also had high level of significance (P < 0.01) and accounted for more than 10 % of phenotypic variance in all three analyses (Table 1). These two QTL have been previously reported by other researchers (Reinprecht et al. 2006; Chen et al. 2007). In addition, two QTL on Chrm.12 are first reported in this study, which are highly significant (P < 0.01) for two-year average, but their significance was slightly below the P < 0.01 threshold in one of the 2 years. By CIM, the large-effect QTL on Chrm. 13 was consistently above LOD threshold regardless of the year, explaining 18 to 33 % of PV (Table 2; Fig. 2a). Another QTL on Chrm. 6 was also significant in 2012 and in the 2-year average, explaining 12 to 15 % of PV on PHT, although this QTL was not significant in 2011 (Table 2; Fig. 2a).
Graphical presentation of position of QTL associated with plant height, lodging, and maturity with LOD plots for 2011, 2012, and the averaged across 2 years. The hatched lines on the LOD plots indicate the LOD thresholds. The 1- and 2-LOD intervals are displayed as bars and solid lines, respectively. A Plant height; B Lodging; C Maturity
A total of four QTL were detected for LDG averaged across the years by single-factor ANOVA (P < 0.01). Two loci were located in Chrm. 4 (designated as LDG-4-5 and LDG-4-32, adding cM distance following the chromosome number), but the SNP markers were separated by a distance of more than 20 cM (Table 1). The LDG-4-32 QTL has not been published earlier, but the other three QTL have been published in the previous studies (Kim et al. 2012; Li et al. 2008a; Mansur et al. 1996; Zhang et al. 2004). In CIM, two QTL were identified on Chrm. 4 and 19 for LDG averaged across the years, which individually explained over 11 % of PV (Table 2). However, these two QTL were not significant in individual year (Table 2).
Three QTL were identified for MAT averaged across years on Chrm. 4, 11, and 12, which had been reported earlier (Lee et al. 1996b; Li et al. 2008b; Zhang et al. 2004) (Table 1). The largest effect QTL for MAT was on Chrm. 12 (MAT-12), which explained 29–30 % of PV. Another QTL on Chrm. 11 (MAT-11) was also highly significant in all three analyses with major effects accounting for up to 17 % of PV. The two MAT QTL on Chrm. 11 and 12 were also highly significant (LOD = 5.8–10.0) in CIM regardless of years accounting for 13–23 % of PV (Table 2; Fig. 2c). Depending on their estimated additive effects, Wyandot allele at each QTL advanced pod maturity up to 8 days (Table 2).
Discussion
The main purpose of this study was to identify QTL for PHT, LDG, and MAT in the Wyandot × PI 567301B population. A number of studies have been conducted to dissect genetic variation for these traits over last two decades (Mansur et al. 1996; Lee et al. 1996a, b; Orf et al. 1999; Panthee et al. 2007). Most of these published studies utilized low resolution genetic maps, where the numbers of RFLP, AFLP, or SSR markers used were not high enough for determination of precise genomic locations of QTL, and the molecular marker and QTL were not tightly linked for MAS with high level of confidence. Also, AFLP and RFLP are not efficient markers for MAS. SNPs are currently the most efficient and favored molecular markers for MAS in most plant species, including Glycine max. Therefore, the present study was undertaken to use a high resolution molecular map of more than 500 SNP markers to map QTL for three important agronomic traits of soybean. The SNPs used in this study are all publicly available (USLP1.0) and thus are free to be used in MAS or otherwise.
The two parents, Wyandot and PI 567301B, of the mapping population had large variation between them for all three traits of interest, and the RILs were expected to significantly segregate among them for each trait. Indeed, large variation was observed among the 91 RILs for each of the traits (Fig. 1). Heritability of PHT (0.83), LDG (0.54), and MAT (0.94) in this study was moderate to high, and in agreement with Lee et al. (1996a), who reported H 2 of 0.78, 0.41 and 0.87 for PHT, LDG, and MAT, respectively. These values were relatively higher than another previous study (Panthee et al. 2007), where a large genotype × environment interaction contributed to low heritability estimates. In soybean, heritability for LDG was generally lower than PHT and MAT (Hanson 1963). There was no transgressive segregation among RILs for PHT and LDG, while significant transgressive segregation was observed for MAT towards earlier or later maturity. It is possible because these RILs have the corresponding QTL alleles for early or late maturity from both parents at several loci conditioning MAT (Table 3). Early maturity was conditioned by alleles of both parents in this population. The seven RILs with earlier maturity also have early-maturity alleles from PI 567301B (late maturity parent), in addition to the early maturity alleles from Wyandot (early maturity parent) (Table 3).
The present study identified QTL for each trait by single-factor ANOVA and CIM, including a few novel QTL and some large effect ones (Tables 1, 2). This was possible due to the large differences among the RILs for each trait and the use of a high resolution genetic map. Since many QTL associated with PHT, LDG, and MAT have been previously reported, there is less chance to find novel QTL associated with these traits. Based on our online literature search and QTL listed in SoyBase (http://www.soybase.org/, accessed August 2014), a few novel QTL were identified in this study (Table 1). A QTL was declared novel in the present study, in case it was more than 20 cM away from previously reported QTL. Previously many of the QTL for these traits were determined using coarse genetic maps. In this study, two novel QTL for PHT and one for LDG were significant in single-factor ANOVA for two-year averages. Particularly, a new QTL for LDG (LDG-4-32) was significant in ANOVA for each year and averaged across years. This QTL was also detected by CIM with above threshold LOD (3.4) in 2011 and had a LOD of 2.5 in average of the 2 years (Fig. 2b). The novel QTL would add to the growing knowledge on the genetic control of these traits.
This study showed that quantitative traits can be strongly affected by environmental factors. In 2012, a severe drought occurred at the beginning of the growing season. There was significant (P < 0.05) genotype × environment interaction for LDG and this unusual condition would affect QTL detection. As a result, less number of QTL were detected across environments by single-factor ANOVA and CIM (Tables 1, 2). The genotype x environment interaction emphasizes the importance of validation or confirmation of QTL associated with quantitative traits via experiments over multiple years and/or locations. In addition to multi-environment tests, QTL can be considered validated when the same QTL are mapped in multiple populations developed using a different set of parents. Validation of a QTL is important, particularly for traits that are environmentally sensitive, because a validated QTL indicates stable expression across environments. The present study validated a number of QTL previously reported in different genetic sources. Especially, PHT-6, PHT-13, LDG-4-5, MAT-11 and MAT-12 were validated with high levels of confidence by ANOVA and CIM. These QTL can be considered robust and environmentally stable.
Though the pattern of relationship between yield and other agronomic traits may vary in soybean, it was shown that late maturity and tall plant height were associated with high yield (Ablett et al. 1989; Mansur et al. 1996; Cober and Morrison 2010). Thus, PHT, LDG, and MAT need to be considered when selecting lines with other desirable traits, such as insect and disease resistance. The validated QTL and three novel QTL in the present study are flanked by two or more SNP markers that are closely linked (within 1–5 cM) to each QTL. The SNP markers identified as closely linked to each QTL in this study are valuable for MAS of the QTL by interested soybean breeding programs. Thus, this study clearly advances the knowledge on the genetic control of plant height, lodging and maturity in soybean, and identifies more efficient and reliable markers for MAS for these traits than those currently exist.
References
Ablett GR, Beversdorf WD, Dirks VA (1989) Performance and stability of indeterminate and determinate soybean in short-season environments. Crop Sci 29:1428–1433
Chapman A, Pantalone VR, Ustun A, Allen FL, Landau-Ellis D, Trigiano RN, Gresshoff PM (2003) Quantitative trait loci for agronomic and seed quality traits in an F2 and F4:6 soybean population. Euphytica 129:387–393
Chen Q, Zhang Z, Liu C, Xin D, Qiu H, Shan D, Shan C, Hu D (2007) QTL analysis of major agronomic traits in soybean. Agric Sci China 6:399–405
Churchill GA, Doerge RW (1994) Empirical threshold values for quantitative trait mapping. Genetics 138:963–971
Cober ER, Morrison MJ (2010) Regulation of seed yield and agronomic characters by photoperiod sensitivity and growth habit genes in soybean. Theor Appl Genet 120:1005–1012
Crossa J, Beyene Y, Kassa S, Perez P, Hickey JM, Chen C, de los Campos G, Burgueno J, Windhausen VS, Buckler E, Jannink J, Lopez Cruz MA, Babu R (2013) Genomic prediction in maize breeding populations with genotyping-by-sequencing. G3-Genes Genomes Genet 3:1903–1926
Ellis ML, Wang H, Paul PA, St Martin SK, McHale LK, Dorrance AE (2012) Identification of soybean genotypes resistant to Fusarium graminearum and genetic mapping of resistance quantitative trait loci in the cultivar Conrad. Crop Sci 52:2224–2233
Fehr WR, Caviness CE, Burmood DT, Pennington JS (1971) Stage of development descriptions for soybeans, Glycine max (L.) Merr. Crop Sci 11:929–931
Hanson WD (1963) Heritability. In: Hanson WD, Robinson HF (eds) Statistical genetics and plant breeding. National Academy of Sciences-National Research Council, Washington, D.C., pp 125–140
Hufford MB, Xu X, van Heerwaarden J, Pyhaejaervi T, Chia J, Cartwright RA, Elshire RJ, Glaubitz JC, Guill KE, Kaeppler SM, Lai J, Morrell PL, Shannon LM, Song C, Springer NM, Swanson-Wagner RA, Tiffin P, Wang J, Zhang G, Doebley J, McMullen MD, Ware D, Buckler ES, Yang S, Ross-Ibarra J (2012) Comparative population genomics of maize domestication and improvement. Nat Genet 44:808–811
Huo N, Garvin DF, You FM, McMahon S, Luo MC, Gu YQ, Lazo GR, Vogel JP (2011) Comparison of a high-density genetic linkage map to genome features in the model grass Brachypodium distachyon. Theor Appl Genet 123:455–464
Hwang EY, Song Q, Jia G, Specht JE, Hyten DL, Costa J, Cregan PB (2014) A genome-wide association study of seed protein and oil content in soybean. BMC Genomics 15:1. doi:10.1186/1471-2164-15-1
Hyten DL, Choi I, Song Q, Specht JE, Carter TE Jr, Shoemaker RC, Hwang E, Matukumalli LK, Cregan PB (2010) A high density integrated genetic linkage map of soybean and the development of a 1536 universal soy linkage panel for quantitative trait locus mapping. Crop Sci 50:960–968
Jun T, Mian MAR, Michel AP (2012a) Genetic mapping revealed two loci for soybean aphid resistance in PI 567301B. Theor Appl Genet 124:13–22
Jun T, Mian MAR, Kang S, Michel AP (2012b) Genetic mapping of the powdery mildew resistance gene in soybean PI 567301B. Theor Appl Genet 125:1159–1168
Jun T, Freewalt K, Michel AP, Mian MAR (2014) Identification of novel QTL for leaf traits in soybean. Plant Breed 133:61–66
Kim MY, Lee S, Van K, Kim T, Jeong S, Choi I, Kim D, Lee Y, Park D, Ma J, Kim W, Kim B, Park S, Lee K, Kim DH, Kim KH, Shin JH, Jang YE, Do Kim K, Liu WX, Chaisan T, Kang YJ, Lee Y, Kim K, Moon J, Schmutz J, Jackson SA, Bhak J, Lee S (2010) Whole-genome sequencing and intensive analysis of the undomesticated soybean (Glycine soja Sieb. and Zucc.) genome. Proc Natl Acad Sci USA 107:22032–22037
Kim M, Hyten DL, Niblack TL, Diers BW (2011) Stacking resistance alleles from wild and domestic soybean sources improves soybean cyst nematode resistance. Crop Sci 51:934–943
Kim KS, Diers BW, Hyten DL, Mian MAR, Shannon JG, Nelson RL (2012) Identification of positive yield QTL alleles from exotic soybean germplasm in two backcross populations. Theor Appl Genet 125:1353–1369
Lam H, Xu X, Liu X, Chen W, Yang G, Wong F, Li M, He W, Qin N, Wang B, Li J, Jian M, Wang J, Shao G, Wang J, Sun SS, Zhang G (2010) Resequencing of 31 wild and cultivated soybean genomes identifies patterns of genetic diversity and selection. Nat Genet 42:1053–1059
Lee SH, Bailey MA, Mian MAR, Shipe ER, Ashley DA, Parrott WA, Hussey RS, Boerma HR (1996a) Identification of quantitative trait loci for plant height, lodging, and maturity in a soybean population segregating for growth habit. Theor Appl Genet 92:516–523
Lee SH, Bailey MA, Mian MAR, Carter TE, Ashley DA, Hussey RS, Parrott WA, Boerma HR (1996b) Molecular markers associated with soybean plant height, lodging, and maturity across locations. Crop Sci 36:728–735
Lee S, Mian MAR, McHale LK, Wang H, Wijeratne AJ, Sneller CH, Dorrance AE (2013a) Novel quantitative trait loci for partial resistance to Phytophthora sojae in soybean PI 398841. Theor Appl Genet 126:1121–1132
Lee S, Mian MAR, McHale LK, Sneller CH, Dorrance AE (2013b) Identification of quantitative trait loci conditioning partial resistance to Phytophthora sojae in soybean PI 407861A. Crop Sci 53:1022–1031
Lee S, Mian MA, Sneller CH, Wang H, Dorrance AE, McHale LK (2014) Joint linkage QTL analyses for partial resistance to Phytophthora sojae in soybean using six nested inbred populations with heterogeneous conditions. Theor Appl Genet 127:429–444
Li D, Pfeiffer TW, Cornelius PL (2008a) Soybean QTL for yield and yield components associated with Glycine soja alleles. Crop Sci 48:571–581
Li W, Zheng DH, Van K, Lee SH (2008b) QTL mapping for major agronomic traits across two years in soybean (Glycine max L. Merr.). J Crop Sci Biotechnol 11:171–190
Li Y, Zhao S, Ma J, Li D, Yan L, Li J, Qi X, Guo X, Zhang L, He W, Chang R, Liang Q, Guo Y, Ye C, Wang X, Tao Y, Guan R, Wang J, Liu Y, Jin L, Zhang X, Liu Z, Zhang L, Chen J, Wang K, Nielsen R, Li R, Chen P, Li W, Reif JC, Purugganan M, Wang J, Zhang M, Wang J, Qiu L (2013) Molecular footprints of domestication and improvement in soybean revealed by whole genome re-sequencing. BMC Genomics 14:579
Liu W, Kim M, Van K, Lee Y, Li H, Liu X, Lee S (2011) QTL identification of yield-related traits and their association with flowering and maturity in soybean. J Crop Sci Biotechnol 14:65–70
Liu Y, Li Y, Reif JC, Mette MF, Liu Z, Liu B, Zhang S, Yan L, Chang R, Qiu L (2013) Identification of quantitative trait loci underlying plant height and seed weight in soybean. Plant Genome 6. doi:10.3835/plantgenome2013.03.0006
Mansur LM, Orf JH, Chase K, Jarvik T, Cregan PB, Lark KG (1996) Genetic mapping of agronomic traits using recombinant inbred lines of soybean. Crop Sci 36:1327–1336
Nguyen VT, Vuong TD, VanToai T, Lee JD, Wu X, Mian MAR, Dorrance AE, Shannon JG, Nguyen HT (2012) Mapping of quantitative trait loci associated with resistance to Phytophthora sojae and flooding tolerance in soybean. Crop Sci 52:2481–2493
Nyquist WE, Barker RJ (1991) Estimation of heritability and prediction of selection response in plant populations. Crit Rev Plant Sci 10:235–322
Orf JH, Chase K, Jarvik T, Mansur LM, Cregan PB, Adler FR, Lark KG (1999) Genetics of soybean agronomic traits: I. Comparison of three related recombinant inbred populations. Crop Sci 39:1642–1651
Panthee DR, Pantalone VR, Saxton AM, West DR, Sams CE (2007) Quantitative trait loci for agronomic traits in soybean. Plant Breed 126:51–57
Reinprecht Y, Poysa VW, Yu K, Rajcan I, Ablett GR, Pauls P (2006) Seed and agronomic QTL in low linolenic acid, lipoxygenase-free soybean (Glycine max (L.) Merrill) germplasm. Genome 49:1510–1527
Schmutz J, Cannon SB, Schlueter J, Ma J, Mitros T, Nelson W, Hyten DL, Song Q, Thelen JJ, Cheng J, Xu D, Hellsten U, May GD, Yu Y, Sakurai T, Umezawa T, Bhattacharyya MK, Sandhu D, Valliyodan B, Lindquist E, Peto M, Grant D, Shu S, Goodstein D, Barry K, Futrell-Griggs M, Abernathy B, Du J, Tian Z, Zhu L, Gill N, Joshi T, Libault M, Sethuraman A, Zhang X, Shinozaki K, Nguyen HT, Wing RA, Cregan P, Specht J, Grimwood J, Rokhsar D, Stacey G, Shoemaker RC, Jackson SA (2010) Genome sequence of the palaeopolyploid soybean. Nature 463:178–183
Song Q, Hyten DL, Jia G, Quigley CV, Fickus EW, Nelson RL, Cregan PB (2013) Development and evaluation of SoySNP50K, a high-density genotyping array for soybean. PLoS ONE 8:e54985
Van Ooijen JW (2004) MapQTL 5, Software for the mapping of quantitative trait loci in experimental population. B. V. Kyazma, Wageningen, Netherlands
Van Ooijen JW (2006) JoinMap 4. software for the calculation of genetic linkage maps in experimental populations. B. V. Kyazma, Wageningen, Netherlands
Wilson RF (2004) Seed composition. In: Boerma HR, Specht JE (eds) Soybeans: improvement, production, and uses. ASA-CSSA-SSSA, Madison, pp 621–677
Zhang WK, Wang YJ, Luo GZ, Zhang JS, He CY, Wu XL, Gai JY, Chen SY (2004) QTL mapping of ten agronomic traits on the soybean (Glycine max L. Merr.) genetic map and their association with EST markers. Theor Appl Genet 108:1131–1139
Acknowledgments
We thank Tim Mendiola, Keith Freewalt, and Ronald Fioritto for their technical help in this study. We also thank members of the Soybean Genomics and Improvement Laboratory, USDA-ARS, Beltsville, MD for development and distribution of the Universal Soy Linkage Panel (USLP 1.0) of 1,536 SNP markers used in this study. This study was supported by UDSA-ARS, Ohio State University/Ohio Agricultural Research and Development Center and a Grant from the Ohio Soybean Council.
Disclaimer
Trade and manufacturers' names are necessary to report factually on available data, however, the USDA neither guarantees nor warrants the standard of the product and the use of the name by the USDA implies no approval of the product to the exclusion of others that may also be suitable.
Author information
Authors and Affiliations
Corresponding author
Electronic supplementary material
Below is the link to the electronic supplementary material.
Rights and permissions
About this article
Cite this article
Lee, S., Jun, T.H., Michel, A.P. et al. SNP markers linked to QTL conditioning plant height, lodging, and maturity in soybean. Euphytica 203, 521–532 (2015). https://doi.org/10.1007/s10681-014-1252-8
Received:
Accepted:
Published:
Issue Date:
DOI: https://doi.org/10.1007/s10681-014-1252-8