Abstract
Massively parallel sequencing (MPS) of identity informative single-nucleotide polymorphisms (IISNPs) enables hundreds of forensically relevant markers to be analysed simultaneously. Generating DNA sequence data enables more detailed analysis including identification of sequence variations between individuals. The GeneRead DNAseq 140 IISNP MPS panel (QIAGEN) has been evaluated on both the MiSeq (Illumina) and Ion PGM™ (Applied Biosystems) MPS platforms using the GeneRead DNAseq Targeted Panels V2 library preparation workflow (QIAGEN). The aims of this study were to (1) determine if the GeneRead DNAseq panel is effective for identity testing by assessing deviation from Hardy-Weinberg (HWE) and pairwise linkage equilibrium (LE); (2) sequence samples with the GeneRead DNAseq panel on the Ion PGM™ using the QIAGEN workflow and assess specificity, sensitivity and accuracy; (3) assess the efficacy of adding biological samples directly to the GeneRead DNAseq PCR, without prior DNA extraction; and (4) assess the effect of varying coverage and allele frequency thresholds on genotype concordance. Analyses of the 140 SNPs for HWE and LE using Fisher’s exact tests and the sequential Bonferroni correction revealed that one SNP was out of HWE in the Japanese population and five SNP combinations were commonly out of LE in 13 of 14 populations. The panel was sensitive down to 0.3125 ng of DNA input. A direct-to-PCR approach (without DNA extraction) produced highly concordant genotypes. The setting of appropriate allele frequency thresholds is more effective for reducing erroneous genotypes than coverage thresholds.
Similar content being viewed by others
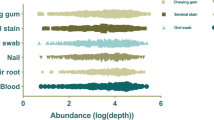
Avoid common mistakes on your manuscript.
Introduction
Massively parallel sequencing (MPS), or next-generation sequencing (NGS), enables the analysis of hundreds of markers in multiple samples simultaneously. Forensic markers including short tandem repeats (STRs), single-nucleotide polymorphisms (SNPs), insertion/deletions (indels) and microhaplotypes, for both identification and intelligence, can be analysed in a single analysis, thereby reducing the consumption of (often limited) evidential material [1]. Of these, the small amplicon size of SNPs compared to STRs confers suitability to the analysis of degraded DNA [2]. SNPs can also be utilised for DNA intelligence purposes by providing phenotype information, when STR profiling has not identified the DNA donor [3]. The application of MPS to SNP analysis has allowed for larger, more sensitive multiplexes with a higher throughput, which has widened the forensic applications of SNPs.
Numerous SNP panels have been developed for forensic purposes [1, 4,5,6,7], and whilst some have been applied using MPS [3, 8], they were limited in the number of SNP loci which could be multiplexed as a result of the single base extension (SBE) chemistry originally employed. The high-throughput afforded by MPS enables large numbers of SNP loci to be sequenced simultaneously, and more recently, MPS panels consisting of hundreds of SNP loci have been developed for identity and intelligence purposes [9,10,11,12,13]. Whilst nucleotide barcoding enables multiple samples to be sequenced using a single MPS run, thus increasing efficiency, further work is required to reduce the costs and times associated with the library preparation process and further enable the automation of this step.
The GeneRead Custom DNAseq 140-SNP panel (GeneRead DNAseq) for human identification (QIAGEN) consists of 88 SNPs from the Kidd lab [14] as well as those from the SNPforID 52plex [7]. This panel has been assessed by Grandell et al. [9] on the MiSeq (Illumina) and de la Puente et al. [10] on the Ion PGM™ (Applied Biosystems). Whilst the panel demonstrated potential for application to forensic casework due to its high discrimination power, sensitivity and robustness, its performance on highly degraded samples, routinely encountered in a forensic context, has had limited testing.
The process of DNA extraction can be lengthy with the potential for contamination, depending on the number of tube transfers. Therefore, the addition of DNA samples directly to PCR (direct-to-PCR) can reduce sample handling time and overall cost to create a more streamlined workflow. Additionally, by omitting extraction, loss of DNA can be minimised [15]. Alternatively, FTA paper is embedded with preservatives that prevent the degradation of DNA in biological samples that are applied to them, including blood, saliva and semen. FTA cards have been previously used in direct-to-PCR, prior to MPS analysis [9].
Limited data have been reported on species specificity and the potential effects of donor or extraction method on DNA yields and subsequent sequence results for GeneRead DNAseq. In addition, it is important to assess the effect of coverage threshold (below which reads are ignored) and allele frequency threshold (below which alleles are not called), as these will influence the genotype concordance depending on any sequencing errors and alignment problems. It should be noted that allele frequency threshold (AFT) determines the minimum homozygote threshold. An AFT of 5%, for example, implies that homozygotes will be called for any allele with frequency in the range of 95–100%.
The objectives of this study were to evaluate the GeneRead DNAseq panel for potential application to forensic identity testing. We tested for Hardy-Weinberg equilibrium (HWE) and linkage equilibrium (LE) in major global populations, required for calculating random match probabilities (RMPs). Forensic validation criteria included sensitivity, specificity and accuracy. We also investigated application of biological samples ‘direct-to-PCR’ and established coverage and allele frequency thresholds for accurate allele calling for single source samples (determined by varying the AFT and coverage thresholds). Four different DNA extraction methods were compared for genotype concordance to test for compatibility with the GeneRead DNAseq panel. A fast library preparation procedure (the QIAseq cfDNA All-In-One Library T Kit: QIAGEN) was employed for all samples, followed by sequencing on an Ion PGM™.
Methods
DNA samples
Biological samples from two donors (A and B) were collected with informed consent. The samples were allocated to a number of forensic assessment studies as outlined in Tables 1 and 2.
Forensic assessment criteria
Forensic assessment criteria included differentiation between donors, effect of sample source, effect of extraction method, species specificity, effect of PCR inhibition, effect of UV degradation and efficacy of direct-to-PCR (Tables 1 and 2). Two different donors (A and B), three different sample types (saliva, blood, semen) and four different extraction methods were applied to assess effects on genotyping accuracy. A chimpanzee blood sample was analysed to see if the panel amplified DNA from a closely related non-human species. Three samples were spiked with increasing amounts of humic acid (HA) (50 ng, 75 ng and 100 ng) to investigate the effect of PCR inhibition on the performance of the GeneRead DNAseq panel. Three extracted DNA samples were exposed to UV light for increasing amounts of time (20, 40 and 60 min). The light was generated from a 30-W source (Sankyo Denki, 253.7 nm, UV-C), with a distance of 32 cm from the samples. Lastly, samples were added direct-to-PCR so that their genotypes could be compared with those from samples that underwent DNA extraction.
DNA extraction
DNA was extracted using four different methods: organic extraction (phenol/chloroform with ethanol precipitation) [16], QIAamp Mini-Spin Column (QIAGEN), ChargeSwitch Forensic DNA Purification kit (Thermo Fisher Scientific: TFS) and QIAsymphony DNA Investigator kit (QIAGEN). Extraction protocols were followed according to the published methodology or the manufacturer’s instructions, as appropriate, with the following alterations: For the organic extraction, the 56 °C incubation time was reduced to 90 min and dithiothreitol (DTT) was omitted from the extraction buffer, as proteinase K and the lysis buffer (Tris-HCl/EDTA/NaCl/SDS) were sufficient for extraction of DNA; for the QIAamp Mini-Spin Column protocol, 500 μL of buffer AL was added to the collected samples instead of 400 μL; for the ChargeSwitch protocol, the incubation time was reduced from 1 h to 35 min with the inclusion of vortexing every 5 min. DNA quantitation was carried out using the Investigator Quantiplex HYres Kit according to the manufacturer’s protocol [17] on a 7500 Real-Time PCR System (Applied Biosystems).
Target enrichment
Target enrichment was performed according to the GeneRead DNAseq Targeted Panels V2 Handbook in 8-well PCR tubes (Axygen Scientific). The recommended 20-ng template input amount was reduced for most samples as described in Tables 1 and 2. In order to account for lower template input amounts, the recommended 20 PCR cycles was also altered for most samples, as detailed in Tables 1 and 2.
Direct-to-PCR
Some samples were added directly to PCRs. A 1.20-mm disc of the three samples on FTA paper was obtained using a Harris ID Uni-Core punch and added to 8 μL of water and 32 μL of mastermix. Five samples collected on cotton swabs were added by removing the tip of the cotton swab and placing it in 500 μL STR GO! Lysis Buffer (QIAGEN). The samples were then incubated at 95 °C for 10 min on a heat block and vortexed every 2 min. Two microlitres of lysate was then added directly to each PCR reaction with 6 μL of nuclease-free water.
Library and template preparation
Libraries were created and amplified using the QIAseq cfDNA All-In-One T Kit (QIAGEN) according to the manufacturer’s standard protocol as well as the optional extra protocol (Amplification of cfDNA library). The 48 samples were barcoded using two All-In-One T Kit adapter plates, each containing 24 barcodes. AMPure XP beads (Beckman Coulter) were used to purify the libraries and remove any residual adapter-dimers according to the manufacturer’s protocol. Twelve representative samples were electrophoresed on a 2100 Bioanalyzer (Agilent) to check the quality and quantity of the libraries using a DNA 1000 chip (Agilent) according to the manufacturer’s recommended protocol.
The amplified DNA libraries were quantified using the QIAseq Library Quant Assay (Protocol 1: Real-Time PCR for GeneRead Library Quant Kit for Ion Torrent: QIAGEN). Following quantification, each sample was diluted to 30 pM and combined into two pools of 24 samples. Pool A consisted of samples 1–24 and Pool B consisted of samples 25–48. The libraries were templated and loaded onto two Ion 316™ v2 BC Chips (Chip 1 and Chip 2) using the Ion PGM™ Hi-Q™ View Chef Kit on the Ion Chef™ (Applied Biosystems) according to the Precision ID Panels with Ion PGM™ System Application Guide protocol.
Sequencing
Sequencing of chips 1 and 2 was performed on an Ion PGM™ using an Ion PGM™ Hi-Q Sequencing Kit according to the Precision ID Panels with Ion PGM™ System Application Guide protocol. The human reference genome was hg19, and the number of flows was set to 500. The target and hotspot regions were supplied by QIAGEN (Electronic Supplementary Materials (ESM) 1 and 2). The barcodes employed by the QIAseq cfDNA Library T Kit are the IonXpress barcodes (Applied Biosystems).
Data analysis
The coverage data were harvested as a variant call file (VCF) from the variantCaller (v5.0.4.0) plugin on the Torrent Suite™ Server (Applied Biosystems). Optimal coverage and allele frequency thresholds (AFT) were investigated by varying the coverage threshold for each allele (below which reads were ignored) from 0 to 200× (in steps of 20×) and the AFT (below which alleles were not called) from 0 to 50% (1% steps between 0 and 5% and 5% steps between 5% and 50%). The genotype concordance for each sample with 1 ng of template was then calculated at each combination of coverage threshold and AFT. Genotype concordance was calculated by comparing each sample genotype to the consensus genotype and reported as a percentage of loci with matching genotypes.
HWE and LE analysis
HWE and LE for the 140 SNPs in the GeneRead DNAseq panel were assessed by harvesting genotypes from the SPSmart online database [18] (http://spsmart.cesga.es/) accessing 1000 Genomes Phase 1 (1093 individuals). Three of the 140 SNPs in the GeneRead DNAseq panel were missing from the database (rs1029047, rs2920816 and rs938283) and were therefore excluded from HWE and LE analysis. Descriptive population genetic statistics and Wright’s F statistics were calculated using Genetic Data Analysis (GDA) software [19]. Fisher’s exact tests [20] for HWE and pairwise LE were performed and p values generated for the multiple hypotheses of no difference. The sequential Bonferroni procedure [21] was applied to the p values to correct for the multiple comparison problem where significant p values are expected to occur by chance.
Results
HWE and LE deviations
Only one SNP, rs7520386 in the Japanese population, was found to deviate from HWE (Table 3). The population with the most SNP combinations out of LE was the African-American population with 14 significant departures from LE (Table 3). The five SNP combinations most commonly exhibiting significant departures from LE for 13 of the 14 subpopulations were rs10768550-rs10500617, rs2175957-rs8070085, rs2291395-rs4789798, rs9606186-rs5746846 and rs689512-rs374163-rs2292972. The three SNPs in the last combination consistently departed from LE, due to their close proximity on the same chromosome.
Coverage distribution
Coverage distribution was assessed for all samples (Fig. 1). The mean coverage of all samples ranged from 19× (rs1058083) to 1387× (SNP rs521861). The mean coverage across all samples and all SNPs was 607×, and the median coverage across all samples and all SNPs was 584×. Consistently low coverage was observed for rs1058083 which was nearly always below 100× (Fig. 1).
Frequency distribution
Allele frequencies were assessed and compared across all samples (Fig. 2). The minimum non-zero allele frequency observed for all nucleotide bases for all samples was 0.01. The maximum allele frequency observed for all nucleotide bases for all samples was one. One SNP (rs1029047) had consistently poor allele balance (means of 24% and 76%) which made genotype calling (homozygote versus heterozygote) ambiguous (Fig. 2).
Mean frequencies for each allele (A, C, G & T) for each SNP across 24 samples with 1-ng DNA template from donor A. These form clusters for sequencing error (lower left), heterozygotes (middle), and homozygotes (upper right). The rs numbers are denoted for outlier SNPs including those with high error rates (rs2399332, rs1031825), low coverage (rs1058083), and poor heterozygote allele balance (rs1029047)
Effect of altering coverage and allele frequency thresholds
The effects of altering coverage threshold and AFT were investigated to determine an optimal combination of thresholds for highest genotype concordance and reproducible allele calls. The consensus genotypes for donors A and B are included as Electronic Supplementary Material (ESM) 3. The optimal AFT at which 99% genotype concordance was reached for the 11 saliva samples from donor A (with 1 ng of DNA template input) ranged from 4 to 35% (Table 4). However, to achieve 99% genotype concordance for all samples, the common AFT range is 15–25%. The highest coverage threshold at which 99% genotype concordance achieved was 120× (sample 11), and there was no lower limit. Genotype concordance declined when the coverage threshold was greater than 20% of the mean coverage of each sample (Electronic Supplementary Material (ESM) 4).
Addition of samples directly to PCR
The genotypes for the eight samples that were added directly to the enrichment PCR (blood semen and saliva on FTA and saliva and blood swabs: Tables 1 and 2) were consistent with the corresponding samples that had undergone DNA extraction. DNA from saliva and blood on FTA paper and cotton swabs, added directly to PCR, resulted in at least 138/140 consensus genotypes (99% genotype concordance) (Fig. 3). Semen on FTA paper resulted in lower genotype concordance, with only 122/140 consensus genotypes (86% genotype concordance). The non-concordant SNPs are listed in Electronic Supplementary Material (ESM) 5.
Effects of environmental insults
Resilience to environmental insults was assessed by artificially degrading DNA samples via exposure to ultraviolet (UV) light for increasing amounts of time (20, 40 and 60 min) and by inducing PCR inhibition with the addition of HA to the enrichment PCR at three amounts (50, 75 and 100 ng). The number of consensus genotypes for the 20-min UV degraded sample was 138 of 140 resulting in 98.5% genotype concordance (Fig. 4). The samples exposed to UV light for 40 and 60 min both returned 140 consensus genotypes (100% genotype concordance) (Fig. 4). The PCR-inhibited samples returned 139 consensus genotypes (for 50 ng and 75 ng HA) and 140 consensus genotypes for 100 ng HA (Fig. 4). The two non-concordant SNPs for PCR inhibited samples were rs1029047 and rs1478829. The two non-concordant SNPs for the UV degraded samples were rs1029047 and rs993934. SNP rs1029047 was observed to be non-concordant for both environmental insults.
Species specificity
Species specificity was assessed by including two replicates of the same chimpanzee sample in the analysis, one on each of the Ion 316™ v2 BC chips. For each chimpanzee sample, 138 of the 140 loci mapped to the human genome reference (h19). The two chimpanzee samples were 96% concordant with each other (but not with the human donors), and both were homozygous at 99% of the loci (Electronic Supplementary Material (ESM) 6).
Effect of different extraction methods
Four different DNA extraction methods were used during this study. The number of consensus genotypes for all extraction methods and all samples was at least 139 (of 140) (Fig. 5). The SNPs that were not concordant between the different extraction methods were rs7704770 (semen on QIAsymphony), rs1029047 (donor A saliva on ChargeSwitch), rs2272998 (donor A saliva on QIAsymphony) and rs9951171 (donor B saliva on QIAsymphony).
Sensitivity
The sensitivity of the GeneRead DNAseq panel was assessed by applying a range (20–0.2 ng) of template input amounts at the enrichment PCR stage, in conjunction with altered PCR cycle numbers (Tables 1 and 2). Figure 6 shows the genotype concordance of the sensitivity series which was found to be between 99 and 100% down to a DNA input amount of 0.3125 ng DNA input. The genotype concordance was reduced to 96–98% at 0.2 ng DNA input. The negative controls (water) indicated homozygous genotypes for 16–17% of loci with 4–8% concordance with donor A or B (Electronic Supplementary Material (ESM) 7). More consensus genotypes were reported overall for chip 1 than chip 2. Chip 1 consistently had 140 consensus genotypes (down to 0.3125 ng). Chip 2 had 139 consensus genotypes for three amounts above 0.3125 ng (Fig. 6).
Discussion
Tests for HWE determine whether or not allele frequencies can be used to calculate genotype frequencies according to Hardy-Weinberg proportions (or deviations using a θ correction factor as defined by NRC II) [22]. Only one SNP (rs7520386) was out of HWE in the Japanese population (Table 3), indicating that the genotype frequencies of the SNPs contained in the GeneRead DNAseq panel can be used to calculate RMP for forensic identity purposes in all other populations. However, for use in Japanese populations, SNP rs7520386 may have to be removed from the panel.
The five SNP combinations that most commonly exhibited significant departures from LE in this study were also reported in previous studies by Pakstis et al. [14], Grandell et al. [9] and de la Puente et al. [10]. It was the recommendation of both Grandell et al. [9] and de la Puente et al. [10] to use haplotype frequencies instead of allele frequencies for the SNP combinations that were consistently out of LE when this panel is applied in forensic casework. According to this logic, the following SNP combinations could be reported as haplotypes: rs10768550-rs10500617, rs2175957-rs8070085, rs2291395-rs4789798, rs9606186-rs5746846 and rs689512-rs374163-rs2292972. This strategy is also suggested by Tillmar and Phillips (2017) in a study which assessed the impact of genetic linkage on forensic marker sets through the development of a biostatistical tool, ILIR (Impact of Linkage on forensic markers for Identity and Relationship testing) [23]. In addition to applying ILIR to assess linkage in forensic marker sets on a case-by-case basis, ILIR can also be applied when developing new forensic marker sets in the future to avoid the selection of markers which are not in linkage equilibrium.
Coverage distribution was assessed for all samples with coverage defined as the number of times an allele was sequenced (or read). SNP rs1058083 had consistently low coverage (minimum 1× and maximum 104×) which is consistent with studies by Grandell et al. [9] and de la Puente et al. [10]. However, rs1058083 was never non-concordant, suggesting that it may only limit the sensitivity of the panel and not the accuracy (Electronic Supplementary Material (ESM) 8). Based on the consistently poor coverage for this SNP, it is recommended that it be removed in order to increase the sensitivity of the GeneRead DNAseq panel. It should be noted that complete dropout was never observed for any locus: We only noted drop out of one of two alleles.
The two negative controls (one on each chip) received zero coverage for over 80% of loci, but the coverage for 16–17% of SNPs ranged from tens to hundreds (Electronic Supplementary Material (ESM) 7). These were consistent between the two controls, always homozygous and mostly not concordant with donors A and B, suggesting that the contaminating alleles were derived from some other source consisting of random alleles.
The allele frequency distribution for all samples was also assessed, and this revealed a SNP (rs1029047) with frequently poor allele frequency balance. This SNP is easily recognised as an outlier from the homozygote and heterozygote clusters in Fig. 2. Our finding is consistent with de la Puente et al. [10] who also reported that SNP rs1029047 was unbalanced because it was prone to sequence misalignments due to homopolymeric stretches immediately flanking each side of the SNP position.
Grandell et al. [9] also noted the consistently poor allelic balance for SNP rs1029047 and concluded that the use of marker specific inclusion thresholds should be applied for genotype calls instead of excluding the SNP from the panel entirely. rs1029047 is also in the Ion Torrent™ HID SNP 169-plex panel (TFS) and was reported by Børsting et al. [24] and Eduardoff et al. [25] to have consistently poor allelic balance. Børsting et al. [24] recommended the removal of the SNP from the panel as a result. MPS is subject to sequencing error, and the panel employed will also contribute to this, depending on the choice of SNPs and the sequences flanking these SNPs, some of which may cause bioinformatics sequence alignment problems. rs1029047 has been found to be non-concordant with control genotypes when sequenced on the Ion PGM™ as a result of homopolymer stretches in flanking regions [3, 13]. It is therefore recommended that SNP rs1029047 be removed from the GeneRead DNAseq panel because it exhibits poor allelic balance.
This study is limited by the inclusion of a maximum of only two replicates for any particular combination of conditions (donor, dilution, extraction method, species, tissue). However, it should be noted that the coverage distribution (Fig. 1) and allele frequency distribution (Fig. 2) were derived from all samples from donor A with a 1-ng template amount. This comprises 24 samples in total. The fact that these samples are from the same donor does have the disadvantage that not all possible genotypes (homozygotes and heterozygotes) for every SNP were observed, but it has the advantage that variation in coverage and allele frequencies for the same genotypes across multiple DNA extraction procedures and tissues were observed. While de la Puente et al. (2017) have already examined replicates from 16 different donors for the same panel on an Ion PGM, we examined reproducibility for the same donor.
Further, this study demonstrated the successful implementation of both the rapid and streamlined workflow of the QIAseq cfDNA All-In-One T Kit (QIAGEN) and the direct addition of biological samples to enrichment PCR. Saliva from donors A and B that were added directly to PCR had high numbers of concordant genotypes, which were consistent with the number of concordant genotypes from samples that went through DNA extraction prior to their addition to PCR (Fig. 3). The cause of the non-concordance that was observed for the direct-to-PCR samples was attributed to a combination of allele drop in and allele drop out due to poor allele balance and low coverage. Grandell et al. [9] reported consistent coverage and genotype calls for all 140 SNP loci for samples derived from blood on FTA paper. This conclusion is consistent with what we observed for blood on cotton swabs and FTA paper. These results indicate that adding blood and saliva samples directly to PCR (via FTA paper or cotton swab), without prior DNA extraction, is sufficient to yield successful sequencing results and reproducible genotypes. However, the types of biological samples that are appropriate for the direct-to-PCR approach will need to be assessed further. Semen on FTA paper added directly to PCR produced 10–12% less concordant genotypes than the saliva or blood samples. This lower performance could be attributed to the need for an additional step in DNA extraction for semen samples to degrade the proteins in the capsule surrounding the spermatozoa head (acrosome). This is the case for differential extraction as commonly applied in forensic laboratories.
The allele frequency window found to be universally ambiguous for separating homozygotes and heterozygotes was 15–25% for all 11 samples, when 99% genotype concordance was achieved (Table 4). This indicates that any alleles with a frequency less than 15% should be regarded as potential sequencing error and removed from analysis. Alleles with frequencies between 15–25% and 75–85% should be treated as ambiguous genotypes. Alleles with frequencies between 25 and 75% should be treated as heterozygotes, and loci with allele frequencies larger than 85% should be treated as homozygotes. Previous studies of the GeneRead DNAseq panel have set thresholds for genotype calls for both heterozygotes and homozygotes. Grandell et al. [9] and de la Puente et al. [10] applied a threshold of 0.4–0.6 for heterozygotes and 0.9–1 [9] and 0.95–1 [10] for homozygotes. However, these choices were not justified and may be conservative as we found that larger homozygote and heterozygote allele frequency windows are possible.
There appeared to be a reduction in genotype concordance when coverage threshold was greater than 20% of the mean coverage per sample (Electronic supplementary data 4). This can be attributed to the increased probability of allele dropout at higher coverage thresholds. It also indicates that when applying a coverage threshold, the mean coverage should be five times greater than the coverage threshold. Nevertheless, there does not appear to be a lower limit on coverage threshold below which genotype concordance drops, which means that a coverage threshold may not need to be applied to achieve highly concordant results. This could be beneficial in situations where biological samples have low starting concentrations, which result in low coverage. The AFT will account for any ambiguous or erroneous genotypes. However, the choice not to apply a coverage threshold could lead to the inclusion of sequencing errors and noise.
Effects of environmental insult were assessed by comparing the genotypes of the degraded or inhibited samples to the consensus genotypes. The enrichment PCR appears to be very resistant to inhibition and able to tolerate DNA degradation from UV light. The samples exposed to UV for the longest amount of time were expected to produce a lower number of consensus genotypes, but they yielded 100% genotype concordance. The panel appears able to successfully genotype DNA that has been exposed to UV light for up to an hour. de la Puente et al. [10] examined a replicate of degraded bone sample and reported 100% genotype concordance; however, the age and type of exposure of the bone sample used were not reported. Samples with HA added to PCR (samples 21–23) to initiate PCR inhibition indicated high levels of genotype concordance (Fig. 4). FTA paper, used in this study for direct-to-PCR samples, can contain PCR inhibitors [26]. Future studies may need to further assess PCR-inhibited samples on the GeneRead DNAseq panel to determine the efficacy of the direct-to-PCR method.
Four different extraction methods were employed to examine the effect of differing extraction methods on downstream sequencing results. These were QIAsymphony, QIAamp Mini- Spin column, ChargeSwitch and organic extraction. No extraction method produced less than 139 consensus genotypes (99% genotype concordance) (Fig. 5). This result indicates that these extraction methods and their corresponding extraction protocols generate reproducible genotypes using the GeneRead DNAseq panel. Non-concordance was attributed to allele dropout due to low allele coverage and poor allele balance for rs1029047. No extraction method performed significantly better than the others. However, the organic method was time consuming and required toxic reagents. de la Puente et al. [10] reported a similar result with an average genotype concordance of 99.52% for samples sequenced with the same GeneRead DNAseq panel.
The sensitivity study indicated high sensitivity with 99–100% genotype concordance for samples with input amount between 20 and 0.3125 ng (Fig. 6). de la Puente et al. [10] reported 100% genotype concordance for 0.5 ng, 0.25 ng and 0.125 ng of input DNA that was prepared from a standard. Grandell et al. [9] applied 2.5-ng, 0.625-ng, 0.2-ng and 0.025-ng input concentrations to a dilution series that reported a larger variation in allele read frequency (ARF) for 0.2-ng input.
Conclusions
The performance of the GeneRead DNAseq panel (QIAGEN) in this study indicates that the panel has great potential for application in forensic identity testing. The QIAseq cfDNA All-In-One T Kit half hour library preparation allowed for a time-efficient library preparation procedure that produced high-quality DNA libraries. The addition of biological samples directly to PCR produced highly concordant results, except for semen on FTA paper, suggesting that this adaptation has the potential to decrease sample handling and processing time. However, the compatibility of biological samples will need to be further evaluated by including more sample types, such as bone, in future direct-to-PCR studies.
It is recommended that two SNPs be removed from the panel; SNP rs1058083 (due to consistently low coverage) and SNP rs1029047 (due to consistently poor allelic balance). To accommodate the five SNP combinations that were consistently out of LE for 13 of the 14 subpopulations, haplotype frequencies could be used instead of allele frequencies in RMP calculations. Alternatively, all but one SNP from each combination could be removed from the panel. One SNP (rs7520386) may need to be removed when the panel is used in Japanese populations because of significant deviation from HWE.
For optimised genotype concordance, allele frequency windows of 25–75% should be applied for heterozygotes and 85% and above for homozygotes. Alleles with frequencies in the range 15–25% and 75–85% are ambiguous and could result in erroneous genotypes.
References
Budowle B, Van Daal A (2008) Forensically relevant SNP classes. BioTechniques 44(5):603–610
Butler JM, Coble MD, Vallone PM (2007) STRs vs. SNPs: thoughts on the future of forensic DNA testing. Forensic Sci Med Pathol 3(3):200–205
Daniel R, Santos C, Phillips C, Fondevila M, van Oorschot RAH, Carracedo Á, Lareu MV, McNevin D (2015) A SNaPshot of next generation sequencing for forensic SNP analysis. Forensic Sci Int Genet 14:50–60
Kidd KK et al (2015) Genetic markers for massively parallel sequencing in forensics. Forensic Sci Int 5:e677–e679
Lou C, Cong B, Li S, Fu L, Zhang X, Feng T, Su S, Ma C, Yu F, Ye J, Pei L (2011) A SNaPshot assay for genotyping 44 individual identification single nucleotide polymorphisms. Electrophoresis 32(3–4):368–378
Phillips C, Salas A, Sánchez JJ, Fondevila M, Gómez-Tato A, Alvarez-Dios J, Calaza M, de Cal MC, Ballard D, Lareu MV, Carracedo A, SNPforID Consortium (2007) Inferring ancestral origin using a single multiplex assay of ancestry-informative marker SNPs. Forensic Sci Int Genet 1(3):273–280
Sanchez JJ, Phillips C, Børsting C, Balogh K, Bogus M, Fondevila M, Harrison CD, Musgrave-Brown E, Salas A, Syndercombe-Court D, Schneider PM, Carracedo A, Morling N (2006) A multiplex assay with 52 single nucleotide polymorphisms for human identification. Electrophoresis 27(9):1713–1724
Mehta B, Daniel R, Phillips C, Doyle S, Elvidge G, McNevin D (2016) Massively parallel sequencing of customised forensically informative SNP panels on the MiSeq. ELECTROPHORESIS 37(21):2832–2840
Grandell I, Samara R, Tillmar AO (2016) A SNP panel for identity and kinship testing using massive parallel sequencing. Int J Legal Med 130(4):905–914
de la Puente M, Phillips C, Santos C, Fondevila M, Carracedo Á, Lareu MV (2017) Evaluation of the Qiagen 140-SNP forensic identification multiplex for massively parallel sequencing. Forensic Sci Int Genet 28:35–43
Phillips C, Parson W, Lundsberg B, Santos C, Freire-Aradas A, Torres M, Eduardoff M, Børsting C, Johansen P, Fondevila M, Morling N, Schneider P, EUROFORGEN-NoE Consortium, Carracedo A, Lareu MV (2014) Building a forensic ancestry panel from the ground up: the EUROFORGEN global AIM-SNP set. Forensic Sci Int Genet 11:13–25
Al-Asfi M, McNevin D, Mehta B, Power D, Gahan ME, Daniel R (2018) Assessment of the Precision ID Ancestry panel. Int J Legal Med 132(6):1581–1594
Seo SB, King JL, Warshauer DH, Davis CP, Ge J, Budowle B (2013) Single nucleotide polymorphism typing with massively parallel sequencing for human identification. Int J Legal Med 127(6):1079–1086
Pakstis AJ, Speed WC, Fang R, Hyland FCL, Furtado MR, Kidd JR, Kidd KK (2010) SNPs for a universal individual identification panel. Hum Genet 127(3):315–324
Ottens R et al (2013) Application of direct PCR in forensic casework. Forensic Sci Int 4(1):e47–e48
McNevin D (2016) Preservation of and DNA Extraction from Muscle Tissue. Methods Mol Biol 1420:43–53
QIAGEN(2014) Investigator Quantiplex HYres Handbook. Version 1:1–16
Amigo J, Salas A, Phillips C, Carracedo Á (2008) SPSmart: adapting population based SNP genotype databases for fast and comprehensive web access. BMC Bioinformatics 9(1):428
Weir BS, Cockerham C (1996) Genetic data analysis II: methods for discrete population genetic data. Sinauer Assoc. Inc., Sunderland
Fisher RA (1922) On the interpretation of χ 2 from contingency tables, and the calculation of P. J R Stat Soc 85(1):87–94
Benjamini Y, Hochberg Y (1995) Controlling the false discovery rate: a practical and powerful approach to multiple testing. J R Stat Soc Ser B Methodol 57(1):289–300
Council, N.R (1996) The evaluation of forensic DNA evidence. The National Academies Press, Washington, DC, p 272
Tillmar AO, Phillips C (2017) Evaluation of the impact of genetic linkage in forensic identity and relationship testing for expanded DNA marker sets. Forensic Sci Int Genet 26:58–65
Børsting C, Fordyce SL, Olofsson J, Mogensen HS, Morling N (2014) Evaluation of the ion torrent™ HID SNP 169-plex: a SNP typing assay developed for human identification by second generation sequencing. Forensic Sci Int Genet 12:144–154
Eduardoff M, Santos C, de la Puente M, Gross TE, Fondevila M, Strobl C, Sobrino B, Ballard D, Schneider PM, Carracedo Á, Lareu MV, Parson W, Phillips C (2015) Inter-laboratory evaluation of SNP-based forensic identification by massively parallel sequencing using the ion PGM™. Forensic Sci Int Genet 17:110–121
Sorensen A, Berry C, Bruce D, Gahan ME, Hughes-Stamm S, McNevin D (2016) Direct-to-PCR tissue preservation for DNA profiling. Int J Legal Med 130(3):607–613
Funding
The authors were supported by QIAGEN through their financial and technical contributions to this project.
Author information
Authors and Affiliations
Corresponding author
Ethics declarations
Conflict of interest
This study was partially funded by QIAGEN. N. Jones and I. Petermann are employed by QIAGEN.
Research involving human participants
Ethics approval was obtained from the University of Canberra Human Research Ethics Committee (Project Number CEHR 14-70), in accordance with the Helsinki Declaration.
Research involving animal participants
All applicable international, national, and/or institutional guidelines for the care and use of animals were followed. The Chimpanzee blood sample was donated from the sample collection of the Australian Federal Police (AFP).
Informed consent
Informed consent was obtained from all individual participants included in the study.
Additional information
Publisher’s Note
Springer Nature remains neutral with regard to jurisdictional claims in published maps and institutional affiliations.
Rights and permissions
About this article
Cite this article
Avent, I., Kinnane, A.G., Jones, N. et al. The QIAGEN 140-locus single-nucleotide polymorphism (SNP) panel for forensic identification using massively parallel sequencing (MPS): an evaluation and a direct-to-PCR trial. Int J Legal Med 133, 677–688 (2019). https://doi.org/10.1007/s00414-018-1975-5
Received:
Accepted:
Published:
Issue Date:
DOI: https://doi.org/10.1007/s00414-018-1975-5