Abstract
Multi-hesitant fuzzy sets (MHFSs) are hesitant fuzzy sets (HFSs) with membership function, permitting the same evaluation value to be repeated several times. MHFSs can depict uncertain information more effectively than HFSs. This study defined three outranking relations of multi-hesitant fuzzy numbers (MHFNs), namely strong dominant, weak dominant and indifferent relationships, based on the elimination and choice translating reality (ELECTRE) I method. Thereafter, we discussed the corresponding properties of the three outranking relations. We also presented an extended ELECTRE I method, in which the criteria are correlated to select the optimal alternatives, by combination of outranking relations and Choquet integral. Eventually, an application example of MHFNs was presented, and a comparative analysis was performed based on the same example.
Similar content being viewed by others
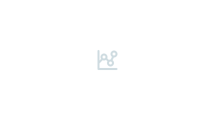
Explore related subjects
Discover the latest articles, news and stories from top researchers in related subjects.Avoid common mistakes on your manuscript.
1 Introduction
The elimination and choice translating reality (ELECTRE) is a family of multi-criteria decision analysis methods that originated in Europe and proposed by Benayoun et al. [1] and Roy [2]. It is typically classified as an “outranking method” of decision-making. To date, the ELECTRE method and its extensions showed playing an important role in the field of multi-criteria decision-making (MCDM) and have greatly attracted scholars’ attention [1,2,3,4,5,6,7,8]. For instance, Hatami-Marbini and Tavana [9] developed an extended ELECTRE I method with fuzzy information. Subsequently, Chen et al. [10] and Chen and Xu [11] developed the extended ELECTRE I and ELECTRE II methods using hesitant fuzzy information. Then, Peng et al. [12] presented an extension of the ELECTRE approach with multi-valued neutrosophic information. They were defined a number of outranking relations for multi-valued neutrosophic numbers, which are based on traditional ELECTRE methods.
In practice, the correlations (e.g. redundancy or complementarity) of criteria exist in real-life decision-making problems. For example, a number of scholars [13,14,15] supported three courses, namely literature, mathematics and physics, aiming to assess a group of students. Science-related courses are generally more important than literature ones; however, a student, who excels in literature and any science-related courses, is superior to others. Thus, a redundancy exists between mathematics and physics, where the weight of the combination of mathematics and physics is less than their total weight. Although there is a complementarity between literature and mathematics or physics, the weight of the combination of literature and mathematics or physics is higher than their total weight. The Choquet integral [16] is advantageous and valuable in handling MCDM problems with correlated criteria. In recent years, this integral has been extensively utilised for the aforementioned purpose [17,18,19,20,21,22]. For instance, Yu et al. [19] studied a few hesitant fuzzy Choquet integral operators. Peng et al. [22] presented single-valued neutrosophic hesitant Choquet integral operators.
In some cases, the membership degree of an element is neither a single value nor an interval, while a set of possible values. To manage such situations where decision-makers (DMs) are hesitant in expressing their preferences over alternatives, hesitant fuzzy sets (HFSs) [23, 24], another extension of traditional fuzzy sets (FSs) [25], provide a useful reference. HFSs were first introduced by Torra [23, 24], permitting the membership degree of an element to be a set of several possible values between 0 and 1. Recently, HFSs have received a remarkable attention in various scientific fields due to their capability to tackle uncertainty. In particular, the aggregation operators [26,27,28,29,30,31,32,33] and measures [34,35,36,37,38] of HFSs and their extensions [39, 40] have been investigated. Zhang and Xu [41] developed a novel method based on technique for order preference by similarity to ideal solution (TOPSIS) and the maximising deviation method for solving MCDM problems, in which the evaluation information provided by the DM was expressed in hesitant fuzzy elements and the information about attribute weights was incomplete. Lin et al. [42] defined a hesitant fuzzy MCDM method based on regret theory. Hu et al. [43] and Liao et al. [44] defined similarity, entropy and several correlation coefficients for HFSs. Li et al. [45] and Farhadinia [46] proposed a number of distance measures for HFSs. Khalid and Beg [47] and Zhang [48] discussed hesitant fuzzy preference relations.
However, in actual decision-making applications, some special cases may consistently emerge, where more than one DM provide the same evaluation values during their assessments. Compared with HFSs, multi-HFSs (MHFSs), which were also developed by Torra [23, 24], are notably consistent with practical decision-making conditions in the following aspects. Firstly, MHFSs emphasise on the frequencies of repeated values, thereby providing flexibility for data collection phase. Secondly, multi-hesitant fuzzy numbers (MHFNs), which act as elements of MHFSs, are appropriate for depicting opinions of several DMs or when more than one DM can provide the same value. For example, five consumers are assumed to provide their evaluation values for the quality of a commodity. Among them, four consumers provide their degrees of satisfaction with regard to this commodity by utilising the concept of ‘good’ with a possibility of 0.8, whereas one person assigns a value of 0.2 denoting ‘good’. Thereafter, this situation can be represented by MHFN (i.e. \(A = \left\{ {0.8,\;0.8,\;0.8,\;0.8,\;0.2} \right\}\)) or in the form of a hesitant fuzzy number (HFN, i.e. \(B = \left\{ {0.8,\;0.2} \right\}\)). Afterwards, the mean value of A and B can be calculated as \(m\left( A \right) = 0.68\) and \(m\left( B \right) = 0.5.\) In addition, the mean satisfaction degree of \(A\) is apparently higher than that of \(B,\) thereby reflecting the differences in the opinions and importance of the assessment of group members. Moreover, MHFSs can depict further initial data related to DMs than HFSs, neglecting the repeated values, thereby causing data loss. Accordingly, MHFNs should be more appropriate than HFNs in depicting initial decision-making information.
Hence, it is recommended to conduct in-depth studies on MHFSs because it has an appropriate capacity in dealing with multi-hesitant fuzzy information, whereas some special forms (e.g. HFSs) fail to do that. However, only few studies have been conducted since the emergence of MHFSs. For example, Peng et al. [49] presented an extended ELECTRE III method with multi-hesitant fuzzy information. Moreover, Ji et al. [50] and Tian et al. [51] extended multi-hesitant fuzzy information to hesitant fuzzy linguistic term sets. Reviewing those studies associated with HFSs indicated that if the methods were directly extended to MHFSs, then a number of their shortcomings that were involved in aggregation operators and distance measures may emerge, as discussed previously [49]. Furthermore, such methods have been developed on the basis of an assumption that the criteria are independent of each other. However, the correlation among the criteria is universal, and no study on the multi-hesitant fuzzy aggregation operator has taken such correlation into account.
The main motivations and contributions of this study are summarised based on the preceding analyses as follows:
-
1.
MHFNs can appropriately express the hesitance of DMs and divergence of several DMs. Particularly, MHFNs can effectively handle some special cases, where more than one DM can provide the same assessment value during the decision-making process. Hence, MHFNs can generate a remarkable superiority than the existing forms in terms of recording the actual frequencies of repeated items, as well as retaining the initial evaluation information.
-
2.
In the decision-making process, criteria are often interdependent or interactive. Choquet integral operator can determine the importance and correlation among the criteria. Therefore, the Choquet integral operator is more applicable than the majority of other aggregation operators because they do not consider dependency among criteria.
-
3.
The current study initially developed a limited number of outranking relations on MHFNs motivated by the ELECTRE. After combining the proposed outranking relations and Choquet integral, we provided a multi-hesitant fuzzy MCDM method that considered the interaction existed among the criteria. The results showed that the proposed method possesses several advantages over the extant methods.
The remainder of this paper is presented as follows. Section 2 presents some basic definitions and a comparison method for HFSs and MHFSs. Section 3 defines several outranking relations of MHFNs and their corresponding properties. Section 4 proposes an extended multi-hesitant fuzzy ELECTRE I method by combining with the Choquet integral. Section 5 provides an application example of MHFNs. Section 6 draws several concluding remarks.
2 Preliminaries
This section presents some basic definitions related to HFSs and MHFSs.
Definition 1
([23, 24]) Let \(X\) denote a reference set, and HFS \(E\) on \(X\) is characterised by a function \(\tilde{h}_{E} \left( x \right)\). The function \(\tilde{h}_{E} \left( x \right)\) in \(X\) is a subset of [0, 1], which is a group of different values in [0, 1].
HFSs can be reduced to HFNs if and only if X has one element. Generally, we can denote the set of all HFNs as HFNS.
Definition 2
( [26]) Let \(\tilde{h}_{\alpha } = \bigcup {_{{\gamma_{\alpha } \in \tilde{h}_{\alpha } }} } \left\{ {\gamma_{\alpha } } \right\}\) and \(\tilde{h}_{\beta } = \bigcup {_{{\gamma_{\beta } \in \tilde{h}_{\alpha } }} } \left\{ {\gamma_{\beta } } \right\}\) be any two HFNs and \(\lambda \ge 0.\) Thereafter, the following four operations can be defined:
-
1.
Exponentiation: \(\left( {\tilde{h}_{\alpha } } \right)^{\lambda } = \bigcup {_{{\gamma \in \tilde{h}_{\alpha } }} } \left\{ {\gamma^{\lambda } } \right\};\)
-
2.
Multiplication: \(\lambda \tilde{h}_{\alpha } = \bigcup {_{{\gamma \in \tilde{h}_{\alpha } }} } \left\{ {1 - \left( {1 - \gamma } \right)^{\lambda } } \right\};\)
-
3.
\(\oplus\)-Union: \(\tilde{h}_{\alpha } \oplus \tilde{h}_{\beta } = \bigcup {_{{\gamma_{\alpha } \in \tilde{h}_{\alpha } ,\gamma_{\beta } \in \tilde{h}_{\alpha } }} } \left\{ {\gamma_{\alpha } + \gamma_{\beta } - \gamma_{\alpha } \gamma_{\beta } } \right\};\) and
-
4.
\(\otimes\)-Intersection: \(\tilde{h}_{\alpha } \otimes \tilde{h}_{\beta } = \bigcup {_{{\gamma_{\alpha } \in \tilde{h}_{\alpha } ,\gamma_{\beta } \in \tilde{h}_{\alpha } }} } \left\{ {\gamma_{\alpha } \gamma_{\beta } } \right\}.\)
Definition 3
( [23, 27]) Let \(X\) denote a reference set, and MHFSs \(E_{M}\) can be characterised as \(E_{M} = \left\{ {x,\tilde{H}_{E} \left( x \right)\left| {x \in X} \right.} \right\}\). The function \(\tilde{H}_{E} \left( x \right)\) in \(X\) is a multi-subset of [0, 1]. In \(\tilde{H}_{E} \left( x \right)\), the possible values can be repeated more than once. If \(X\) has a single element, then \(\tilde{H}_{E} \left( x \right)\) is an MHFN. We can denote the set of all MHFNs as MHFNS. Particularly, if \(\tilde{H}_{E} \left( x \right)\) is a set of possible values with no repeated values, then MHFS are reduced to HFS. In other words, HFS is a special form of MHFS, i.e. HFN is also a special form of MHFN. Therefore, all operations and comparison method proposed on the basis of MHFNs can be applied to HFNs.
Definition 4
Let \(\tilde{H}_{\alpha } = \left\{ {\gamma_{i} |\gamma_{i} \in \tilde{H}_{\alpha } ,\;{\text{and}}\;i = 1,2, \ldots ,l_{{\tilde{H}_{\alpha } }} } \right\}\) and \(\tilde{H}_{\beta } = \left\{ {\gamma_{j} |\gamma_{j} \in \tilde{H}_{\beta } ,\;{\text{and}}\;j = 1,2, \ldots ,l_{{\tilde{H}_{\beta } }} } \right\}\) be any two MHFNs and \(\lambda \ge 0.\) Thereafter, the followings can be defined:
-
1.
Exponentiation: \(\tilde{H}_{\alpha }^{\lambda } = \left\{ {\gamma_{i}^{\lambda } |\gamma_{i} \in \tilde{H}_{\alpha } ,\;{\text{and}}\;i = 1,2, \ldots ,l_{{\tilde{H}_{\alpha } }} } \right\};\)
-
2.
Multiplication: \(\lambda \tilde{H}_{\alpha } = \left\{ {1 - \left( {1 - \gamma_{i} } \right)^{\lambda } |\gamma_{i} \in \tilde{H}_{\alpha } ,\;{\text{and}}\;i = 1,2, \ldots ,l_{{\tilde{H}_{\alpha } }} } \right\};\)
-
3.
Intersection: \(\tilde{H}_{\alpha } \cap \tilde{H}_{\beta } = \left\{ {\gamma |\gamma \in \tilde{H}_{\alpha } \;{\text{and}}\,\gamma \in \tilde{H}_{\beta } } \right\};\)
-
4.
Union: \(\tilde{H}_{\alpha } \cup \tilde{H}_{\beta } = \left\{ {\gamma |\gamma \in \tilde{H}_{\alpha } \;{\text{or}}\;\gamma \in \tilde{H}_{\beta } } \right\};\)
-
5.
\(\oplus\)-Union: \(\tilde{H}_{\alpha } \oplus \tilde{H}_{\beta } = \left\{ {\gamma_{i} + \gamma_{j} - \gamma_{i} \gamma_{j} |\gamma_{i} \in \tilde{H}_{\alpha } \;{\text{and}}\;\gamma_{j} \in \tilde{H}_{\beta } } \right\};\)
-
6.
\(\otimes\)-Intersection: \(\tilde{H}_{\alpha } \otimes \tilde{H}_{\beta } = \left\{ {\gamma_{i} \gamma_{j} |\gamma_{i} \in \tilde{H}_{\alpha } \;{\text{and}}\;\gamma_{j} \in \tilde{H}_{\beta } } \right\};\)
-
7.
Complement: \(\tilde{H}_{\alpha }^{c} = \left\{ {1 - \gamma_{i} |\gamma_{i} \in \tilde{H}_{\alpha } ,\;{\text{and}}\;i = 1,2, \ldots ,l_{{\tilde{H}_{\alpha } }} } \right\}.\).
where \(l_{{\tilde{H}_{\alpha } }}\) represents the number of elements in \(\tilde{H}_{\alpha }\). It can be proved that the operations of MHFNs are also effective and reasonable for HFNs. In particular, if both \(\tilde{H}_{\alpha }\) and \(\tilde{H}_{\beta }\) have no repeated values, and then the operations (1), (2), (5) and (6) presented in Definition 4 are deduced to the operations of HHNs, as shown in Definition 2.
Example 1
Assume that \(\tilde{H}_{\alpha } = \left\{ {0.2,\;\;0.2,\;0.3} \right\}\) and \(\tilde{H}_{\beta } = \left\{ {0.3,\;0.4} \right\}\) are three MHFNs, and \(\lambda = 2;\) then, we have the following results:
-
1.
\(\tilde{H}_{\alpha }^{2} = \left\{ {0.04,\;0.04,\;0.09} \right\};\)
-
2.
\(2 \cdot \tilde{H}_{\alpha } = \left\{ {0.36,\;0.36,\;0.51} \right\};\)
-
3.
\(\tilde{H}_{\alpha } \oplus \tilde{H}_{\beta } = \left\{ {0.44,\;0.52,\;0.44,\;0.52,\;0.51,\;0.58} \right\};\)
-
4.
\(\tilde{H}_{\alpha } \otimes \tilde{H}_{\beta } = \left\{ {0.06,\;0.08,\;0.06,\;0.08,\;0.09,\;0.12} \right\}.\)
Based on the ranking method of HFNs [10, 11, 42], the corresponding comparison method of MHFNs is defined in the following.
Definition 5
Let \(\tilde{H} = \left\{ {\gamma_{i} |\gamma_{i} \in \tilde{H},\;{\text{and}}\;i = 1,2, \ldots ,l_{{\tilde{H}}} } \right\}\) be a MHFN, thus, \(\tilde{s}\left( {\tilde{H}} \right) = \frac{1}{{l_{{\tilde{H}}} }}\sum\nolimits_{{\tilde{\gamma }_{i} \in \tilde{H}}} {\tilde{\gamma }_{i} }\) and \(\tilde{a}\left( {\tilde{H}} \right) = \frac{1}{{l_{{\tilde{H}}} - 1}}\sum\nolimits_{{\tilde{\gamma }_{i} \in \tilde{H}}} {\left( {\tilde{s}\left( {\tilde{H}} \right) - \tilde{\gamma }_{i} } \right)^{2} }\) be called the score function and accuracy function of \(\tilde{H}\), respectively. For any two MHFNs \(\tilde{H}_{\alpha }\) and \(\tilde{H}_{\beta }\), the comparison method for MHFNs is as follows:
-
1.
if \(\tilde{s}\left( {\tilde{H}_{\alpha } } \right) < \tilde{s}\left( {\tilde{H}_{\beta } } \right)\), then \(\tilde{H}_{\alpha } < \tilde{H}_{\beta } ;\)
-
2.
if \(\tilde{s}\left( {\tilde{H}_{\alpha } } \right) = \tilde{s}\left( {\tilde{H}_{\beta } } \right),\) then
-
if \(\tilde{a}\left( {\tilde{H}_{\alpha } } \right) = \tilde{a}\left( {\tilde{H}_{\beta } } \right),\) then \(\tilde{H}_{\alpha } \sim \tilde{H}_{\beta } ;\)
-
if \(\tilde{a}\left( {\tilde{H}_{\alpha } } \right) < \tilde{a}\left( {\tilde{H}_{\beta } } \right),\) then \(\tilde{H}_{\alpha } \succ \tilde{H}_{\beta } ;\)
-
if \(\tilde{a}\left( {\tilde{H}_{\alpha } } \right) > \tilde{a}\left( {\tilde{H}_{\beta } } \right),\) then \(\tilde{H}_{\beta } \succ \tilde{H}_{\alpha } .\)
-
where \(l_{{\tilde{H}_{\alpha } }}\) represents the number of elements in \(\tilde{H}_{\alpha }\) and ‘\(\succ\)’ indicates ‘superior to’.
Example 2
If \(\tilde{H}_{\alpha } = \left\{ {0.3,\;\;0.4,\;0.4} \right\}\) and \(\tilde{H}_{\beta } = \left\{ {0.3,\;0.4} \right\}\) are two MHFNs, then we have \(\tilde{s}\left( {\tilde{H}_{\alpha } } \right) = 0.3667\) and \(\tilde{s}\left( {\tilde{H}_{\beta } } \right) = 0.35\). Apparently, \(\tilde{s}\left( {\tilde{H}_{\alpha } } \right) > \tilde{s}\left( {\tilde{H}_{\beta } } \right);\) thus, \(\tilde{H}_{\alpha } > \tilde{H}_{\beta }\) can be achieved.
3 Outranking Relations on MHFNs
Subsequently, the binary relationship between two MHFNs is defined based on the ELECTRE [1,2,3,4].
Definition 6
For any two MHFNs \(\tilde{H}_{\alpha }\) and \(\tilde{H}_{\beta } ,\) all elements in MHFNs are placed in ascending sequence, and \(\tilde{\gamma }_{{\tilde{H}_{i} }}^{\sigma \left( j \right)} \left( {i = \alpha ,\beta } \right)\) represents the \(j\) th largest value in \(\tilde{H}_{i} .\) Thereafter, the strong dominant, weak dominant and indifferent relationships of MHFNs are defined as follows:
-
1.
If \(\forall \;\tilde{\gamma }_{\alpha }^{\sigma \left( j \right)} \in \tilde{H}_{\alpha } ,\;\tilde{\gamma }_{\beta }^{\sigma \left( j \right)} \in \tilde{H}_{\beta } ,\), \(\;\tilde{\gamma }_{\alpha }^{\sigma \left( j \right)} \ge \;\tilde{\gamma }_{\beta }^{\sigma \left( j \right)}\) and \(\;\tilde{\gamma }_{\alpha }^{{\sigma \left( {l_{{\tilde{H}_{\alpha } }} } \right)}} \ge \;\tilde{\gamma }_{\beta }^{{\sigma \left( {l_{{\tilde{H}_{\beta } }} } \right)}}\) (two equals are not held simultaneously), then \(\tilde{H}_{\alpha } \succ_{S} \tilde{H}_{\beta }\); that is, \(\tilde{\rm H}_{\alpha }\) is strongly dominated by \(\tilde{H}_{\beta } .\)
-
2.
If \(\forall \;\tilde{\gamma }_{\alpha }^{\sigma \left( j \right)} \in \tilde{H}_{\alpha } ,\;\tilde{\gamma }_{\beta }^{\sigma \left( j \right)} \in \tilde{H}_{\beta } ,\), \(\;\tilde{\gamma }_{\alpha }^{\sigma \left( j \right)} \le \;\tilde{\gamma }_{\beta }^{\sigma \left( j \right)}\) and \(\;\tilde{\gamma }_{\alpha }^{{\sigma \left( {l_{{\tilde{\rm H}_{\alpha } }} } \right)}} \le \tilde{\gamma }_{\beta }^{{\sigma \left( {l_{{\tilde{\rm H}_{\beta } }} } \right)}}\) (two equals are not held simultaneously), then \(\tilde{H}_{\beta } \succ_{S} \tilde{H}_{\alpha } ;\) that is, \(\tilde{H}_{\beta }\) is strongly dominated by \(\tilde{H}_{\alpha } .\)
-
3.
If conditions (1) and (2) cannot hold, and \(\tilde{s}\left( {\tilde{H}_{\alpha } } \right) > \tilde{s}\left( {\tilde{H}_{\beta } } \right)\) or \(\tilde{s}\left( {\tilde{H}_{\alpha } } \right) = \tilde{s}\left( {\tilde{H}_{\beta } } \right)\) and \(\tilde{a}\left( {\tilde{H}_{\alpha } } \right) < \tilde{a}\left( {\tilde{H}_{\beta } } \right)\), then \(\tilde{\rm H}_{\alpha } \succ_{W} \tilde{\rm H}_{\beta }\); that is, \(\tilde{H}_{\alpha }\) is weakly dominated by \(\tilde{H}_{\beta } .\)
-
4.
If conditions (1) and (2) cannot hold, and \(\tilde{s}\left( {\tilde{H}_{\alpha } } \right) < \tilde{s}\left( {\tilde{H}_{\beta } } \right)\) or \(\tilde{s}\left( {\tilde{H}_{\alpha } } \right) = \tilde{s}\left( {\tilde{H}_{\beta } } \right)\) and \(\tilde{a}\left( {\tilde{H}_{\alpha } } \right) > \tilde{a}\left( {\tilde{H}_{\beta } } \right),\) then \(\tilde{H}_{\beta } \succ_{W} \tilde{H}_{\alpha } ;\) that is, \(\tilde{H}_{\beta }\) is weakly dominated by \(\tilde{H}_{\alpha } .\)
-
5.
If \(\tilde{s}\left( {\tilde{H}_{\alpha } } \right) = \tilde{s}\left( {\tilde{H}_{\beta } } \right)\) and \(\tilde{a}\left( {\tilde{H}_{\alpha } } \right) = \tilde{a}\left( {\tilde{H}_{\beta } } \right),\) then \(\tilde{H}_{\alpha } \sim_{I} \tilde{H}_{\beta } ;\) that is, \(\tilde{\rm H}_{\alpha }\) is indifferent to \(\tilde{H}_{\beta } .\)
where \(\;\tilde{\gamma }_{\alpha }^{\sigma \left( j \right)} \in \tilde{H}_{\alpha } ,\;\tilde{\gamma }_{\beta }^{\sigma \left( j \right)} \in \tilde{H}_{\beta } ,j = 1,2, \ldots ,l_{{\tilde{H}}}\) and \(l_{{\tilde{H}}} = \hbox{min} \left( {l_{{\tilde{H}_{\alpha } }} ,l_{{\tilde{H}_{\beta } }} } \right)\) (\(l_{{\tilde{H}_{i} }}\)) denotes the number of elements in \(\tilde{H}_{i} ;\) and \(\tilde{s}( \cdot )\) and \(\tilde{a}( \cdot )\) denote the score and accuracy functions of MHFNs, respectively.
Property 1
For any three MHFNs \(\tilde{H}_{\alpha } ,\;\tilde{H}_{\beta } \;and\;\tilde{H}_{\chi } ,\) if \(\tilde{H}_{\alpha } \succ_{S} \tilde{H}_{\beta }\) and \(\tilde{H}_{\beta } \succ_{S} \tilde{H}_{\chi } ,\) then \(\tilde{H}_{\alpha } \succ_{S} \tilde{H}_{\chi } .\)
Proof
If \(\tilde{H}_{\alpha } \succ_{S} \tilde{H}_{\beta } ,\) then we have \(\forall \;\tilde{\gamma }_{\alpha }^{\sigma \left( j \right)} \in \tilde{H}_{\alpha } ,\;\tilde{\gamma }_{\beta }^{\sigma \left( j \right)} \in \tilde{H}_{\beta } ,\)\(\;\tilde{\gamma }_{\alpha }^{\sigma \left( j \right)} \ge \;\tilde{\gamma }_{\beta }^{\sigma \left( j \right)}\) and \(\;\tilde{\gamma }_{\alpha }^{{\sigma \left( {l_{{\tilde{H}_{\alpha } }} } \right)}} > \;\tilde{\gamma }_{\beta }^{{\sigma \left( {l_{{\tilde{H}_{\beta } }} } \right)}}\) or \(\;\tilde{\gamma }_{\alpha }^{\sigma \left( j \right)} > \;\tilde{\gamma }_{\beta }^{\sigma \left( j \right)}\) and \(\;\tilde{\gamma }_{\alpha }^{{\sigma \left( {l_{{\tilde{H}_{\alpha } }} } \right)}} \ge \;\tilde{\gamma }_{\beta }^{{\sigma \left( {l_{{\tilde{H}_{\beta } }} } \right)}}\). Similarly, if \(\tilde{H}_{\beta } \succ_{S} \tilde{H}_{\chi }\), then \(\forall \;\tilde{\gamma }_{\beta }^{\sigma \left( j \right)} \in \tilde{H}_{\beta } ,\;\tilde{\gamma }_{\chi }^{\sigma \left( j \right)} \in \tilde{H}_{\chi } ,\)\(\;\tilde{\gamma }_{\beta }^{\sigma \left( j \right)} \ge \;\tilde{\gamma }_{\chi }^{\sigma \left( j \right)}\) and \(\;\tilde{\gamma }_{\beta }^{{\sigma \left( {l_{{\tilde{H}_{\beta } }} } \right)}} > \;\tilde{\gamma }_{\chi }^{{\sigma \left( {l_{{\tilde{H}_{\chi } }} } \right)}}\) or \(\;\tilde{\gamma }_{\beta }^{\sigma \left( j \right)} > \tilde{\gamma }_{\chi }^{\sigma \left( j \right)}\) and \(\;\tilde{\gamma }_{\beta }^{{\sigma \left( {l_{{\tilde{H}_{\beta } }} } \right)}} \ge \;\tilde{\gamma }_{\chi }^{{\sigma \left( {l_{{\tilde{H}_{\chi } }} } \right)}}\). Therefore, \(\forall \tilde{\gamma }_{\alpha }^{\sigma \left( j \right)} \in \tilde{H}_{\alpha } ,\;\tilde{\gamma }_{\beta }^{\sigma \left( j \right)} \in \tilde{H}_{\beta } ,\;\tilde{\gamma }_{\chi }^{\sigma \left( j \right)} \in \tilde{H}_{\chi } .\). Further derivations are presented as follows:
Applying Definition 6 yields \(\tilde{H}_{\alpha } \succ_{S} \tilde{H}_{\chi } .\)
Property 2
For any three MHFNs\(\tilde{H}_{\alpha } ,\;\tilde{H}_{\beta } \;and\;\tilde{H}_{\chi } ,\) if \(\tilde{H}_{\alpha } \succ_{W} \tilde{H}_{\beta }\)and\(\tilde{H}_{\beta } \succ_{W} \tilde{H}_{\chi } ,\)then\(\tilde{H}_{\alpha } \succ_{W} \tilde{H}_{\chi } .\).
Proof
Given \(\tilde{H}_{\alpha } \succ_{W} \tilde{H}_{\beta } ,\) we have \(\tilde{s}\left( {\tilde{H}_{\alpha } } \right) > \tilde{s}\left( {\tilde{H}_{\beta } } \right)\) or \(\tilde{s}\left( {\tilde{H}_{\alpha } } \right) = \tilde{s}\left( {\tilde{H}_{\beta } } \right)\) and \(\tilde{a}\left( {\tilde{H}_{\alpha } } \right) < \tilde{a}\left( {\tilde{H}_{\beta } } \right)\). If \(\tilde{H}_{\beta } \succ_{W} \tilde{H}_{\chi } ,\) then \(\tilde{s}\left( {\tilde{H}_{\beta } } \right) > \tilde{s}\left( {\tilde{H}_{\chi } } \right)\) or \(\tilde{s}\left( {\tilde{H}_{\beta } } \right) = \tilde{s}\left( {\tilde{H}_{\chi } } \right)\) and \(\tilde{a}\left( {\tilde{H}_{\beta } } \right) < \tilde{a}\left( {\tilde{H}_{\chi } } \right).\) Thus, the following derivations are presented by applying Definition 6.
If \(\left. \begin{aligned} \tilde{s}\left( {\tilde{H}_{\alpha } } \right) > \tilde{s}\left( {\tilde{H}_{\beta } } \right) \hfill \\ \tilde{s}\left( {\tilde{H}_{\beta } } \right) > \tilde{s}\left( {\tilde{H}_{\chi } } \right) \hfill \\ \end{aligned} \right\} \Rightarrow \tilde{s}\left( {\tilde{H}_{\alpha } } \right) > \tilde{s}\left( {\tilde{H}_{\chi } } \right),\) then we obtain \(\tilde{H}_{\alpha } \succ_{W} \tilde{H}_{\chi } .\)
If \(\left. \begin{aligned} \tilde{s}\left( {\tilde{H}_{\alpha } } \right) > \tilde{s}\left( {\tilde{H}_{\beta } } \right) \hfill \\ \tilde{s}\left( {\tilde{H}_{\beta } } \right) = \tilde{s}\left( {\tilde{H}_{\chi } } \right) \hfill \\ \end{aligned} \right\} \Rightarrow \tilde{s}\left( {\tilde{H}_{\alpha } } \right) > \tilde{s}\left( {\tilde{H}_{\chi } } \right),\) then we obtain \(\tilde{H}_{\alpha } \succ_{W} \tilde{H}_{\chi } .\)
If \(\left. \begin{aligned} \tilde{s}\left( {\tilde{H}_{\alpha } } \right) = \tilde{s}\left( {\tilde{H}_{\beta } } \right) \hfill \\ \tilde{s}\left( {\tilde{H}_{\beta } } \right) > \tilde{s}\left( {\tilde{H}_{\chi } } \right) \hfill \\ \end{aligned} \right\} \Rightarrow \tilde{s}\left( {\tilde{H}_{\alpha } } \right) > \tilde{s}\left( {\tilde{H}_{\chi } } \right),\) then we obtain \(\tilde{H}_{\alpha } \succ_{W} \tilde{H}_{\chi } .\)
If \(\left. \begin{aligned} \tilde{s}\left( {\tilde{H}_{\alpha } } \right) = \tilde{s}\left( {\tilde{H}_{\beta } } \right)\;{\text{and}}\;\tilde{a}\left( {\tilde{H}_{\alpha } } \right) < \tilde{a}\left( {\tilde{H}_{\beta } } \right) \hfill \\ \tilde{s}\left( {\tilde{H}_{\beta } } \right) = \tilde{s}\left( {\tilde{H}_{\chi } } \right)\;{\text{and}}\;\tilde{a}\left( {\tilde{H}_{\beta } } \right) < \tilde{a}\left( {\tilde{H}_{\chi } } \right) \hfill \\ \end{aligned} \right\} \Rightarrow \tilde{s}\left( {\tilde{H}_{\alpha } } \right) = \tilde{s}\left( {\tilde{H}_{\chi } } \right)\;{\text{and}}\)\(\tilde{a}\left( {\tilde{H}_{\alpha } } \right) < \tilde{a}\left( {\tilde{H}_{\beta } } \right),\) then we obtain \(\tilde{H}_{\alpha } \succ_{W} \tilde{H}_{\chi } .\)
Property 3
For any three MHFNs \(\tilde{H}_{\alpha } ,\;\tilde{H}_{\beta } \;and\;\tilde{H}_{\chi } ,\) if \(\tilde{H}_{\alpha } \sim_{I} \tilde{H}_{\beta }\) and \(\tilde{H}_{\beta } \sim_{I} \tilde{H}_{\chi } ,\) then we have \(\tilde{H}_{\alpha } \sim_{I} \tilde{H}_{\chi } .\)
Proof
If \(\tilde{H}_{\alpha } \sim_{I} \tilde{H}_{\beta } ,\) then we have \(\tilde{s}\left( {\tilde{H}_{\alpha } } \right) = \tilde{s}\left( {\tilde{H}_{\beta } } \right)\) and \(\tilde{a}\left( {\tilde{H}_{\alpha } } \right) = \tilde{a}\left( {\tilde{H}_{\beta } } \right).\) Similarly, if \(\tilde{H}_{\beta } \sim_{I} \tilde{H}_{\chi } ,\) then \(\tilde{s}\left( {\tilde{H}_{\beta } } \right) = \tilde{s}\left( {\tilde{H}_{\chi } } \right)\) and \(\tilde{a}\left( {\tilde{H}_{\beta } } \right) = \tilde{a}\left( {\tilde{H}_{\chi } } \right).\) Therefore, further derivations are formulated as follows:
Thus, \(\tilde{H}_{\alpha } \sim_{I} \tilde{H}_{\chi } .\)
Property 4
For any \(\tilde{H}_{\alpha } ,\tilde{H}_{\beta } \;and\;\tilde{H}_{\chi } \in MHFNS,\) the following results must hold.
-
1.
The strong dominant relations satisfy the non-reflexivity, asymmetry and transitivity, which can be respectively expressed as follows:
-
①
\(\forall \tilde{H}_{\alpha } \in MHFNS,\;\tilde{H}_{\alpha } \not \succ_{S} {\rm H}\tilde{H}_{\alpha } ;\)
-
②
\(\forall \tilde{\rm H}_{\alpha } ,\tilde{H}_{\beta } \in MHFNS,\;\tilde{H}_{\alpha } \succ_{S} \tilde{H}_{\beta } \Rightarrow \tilde{H}_{\beta } \not \succ_{S} \tilde{H}_{\alpha } ;\) and
-
③
\(\forall \tilde{H}_{\alpha } ,\tilde{H}_{\beta } ,\tilde{H}_{\chi } \in MHFNS,\;\tilde{H}_{\alpha } \succ_{S} \tilde{H}_{\beta } ,\tilde{H}_{\beta } \succ_{S} \tilde{H}_{\chi } \Rightarrow \tilde{H}_{\alpha } \succ_{S} \tilde{H}_{\chi } .\)
-
①
-
2.
The weak dominant relations satisfy the non-reflexivity, asymmetry and transitivity, that can be respectively expressed as follows:
-
④
\(\forall \tilde{H}_{\alpha } \in MHFNS,\;\tilde{H}_{\alpha } \not \succ_{W} \tilde{H}_{\alpha } ;\)
-
⑤
\(\forall \tilde{H}_{\alpha } ,\tilde{H}_{\beta } \in MHFNS,\;\tilde{H}_{\alpha } \succ_{W} \tilde{H}_{\beta } \Rightarrow \tilde{H}_{\beta } \not \succ_{W} \tilde{H}_{\alpha } ;\) and
-
⑥
\(\forall \tilde{H}_{\alpha } ,\tilde{H}_{\beta } ,\tilde{H}_{\chi } \in MHFNS,\;\tilde{H}_{\alpha } \succ_{W} \tilde{H}_{\beta } ,\tilde{H}_{\beta } \succ_{W} \tilde{H}_{\chi } \Rightarrow \tilde{H}_{\alpha } \succ_{W} \tilde{H}_{\chi } .\)
-
④
-
3.
The indifferent relations satisfy the reflexivity, symmetry and transitivity, which can be respectively expressed as follows:
-
⑦
\(\forall \tilde{H}_{\alpha } \in MHFNS,\;\tilde{H}_{\alpha } \sim_{I} \tilde{H}_{\alpha } ;\)
-
⑧
\(\forall \tilde{H}_{\alpha } ,\tilde{H}_{\beta } \in MHFNS,\;\tilde{H}_{\alpha } \sim_{I} \tilde{H}_{\beta } \Rightarrow \tilde{H}_{\beta } \sim_{I} \tilde{H}_{\alpha } ;\) and
-
⑨
\(\forall \tilde{H}_{\alpha } ,\tilde{H}_{\beta } ,\tilde{H}_{\chi } \in MHFNS,\;\tilde{H}_{\alpha } \sim_{I} \tilde{H}_{\beta } ,\tilde{H}_{\beta } \sim_{I} \tilde{H}_{\chi } \Rightarrow \tilde{H}_{\alpha } \sim_{I} \tilde{H}_{\chi } .\)
-
⑦
Example 3
② and ⑤ in Property 4 are exemplified as follows:
-
1.
For two MHFNs \(\tilde{H}_{\alpha } = \left\{ {0.1,\;0.2,\;0.2,\;0.5} \right\}\) and \(\tilde{H}_{\beta } = \left\{ {0.1,\;0.1,\;0.2} \right\},\) we obtain \(\tilde{H}_{\alpha } \succ_{S} \tilde{H}_{\beta } ;\) however, \(\tilde{H}_{\beta } \not \succ_{S} \tilde{H}_{\alpha } .\)
-
2.
For two MHFNs \(\tilde{H}_{\alpha } = \left\{ {0.2,\;0.2,\;0.3} \right\}\) and \(\tilde{H}_{\beta } = \left\{ {0.1,\;0.3,\;0.3} \right\},\) we obtain \(\tilde{s}\left( {\tilde{H}_{\alpha } } \right) = \tilde{s}\left( {\tilde{H}_{\beta } } \right) = 0.233,\)\(\tilde{a}\left( {\tilde{H}_{\alpha } } \right) = 0.003\) and \(\tilde{a}\left( {\tilde{H}_{\beta } } \right) = 0.013.\) Thus, \(\tilde{H}_{\alpha } \succ_{W} \tilde{H}_{\beta } ;\) however, \(\tilde{H}_{\beta } \not \succ_{W} \tilde{H}_{\alpha } .\)
Property 5
For any two actions\(\tilde{a}_{\alpha }\)and\(\tilde{a}_{\beta } ,\)the performances for actions\(\tilde{a}_{\alpha }\)and\(\tilde{a}_{\beta }\)are expressed by MHFNs. In addition,\(P = S \cup W \cup I\)indicates that ‘\(\tilde{a}_{\alpha }\)is at least as good as\(\tilde{a}_{\beta }\)’. Thereafter, the following results must hold.
-
1.
\(\tilde{a}_{\alpha } P\tilde{a}_{\beta }\) and not \(\tilde{a}_{\beta } P\tilde{a}_{\alpha } ,\) that is, \(\tilde{a}_{\alpha } \succ_{S} \tilde{a}_{\beta }\) or \(\tilde{a}_{\alpha } \succ_{W} \tilde{a}_{\beta } ;\)
-
2.
\(\tilde{a}_{\beta } P\tilde{a}_{\alpha }\) and not \(\tilde{a}_{\alpha } P\tilde{a}_{\beta } ,\) that is, \(\tilde{a}_{\beta } \succ_{S} \tilde{a}_{\alpha }\) or \(\tilde{a}_{\beta } \succ_{W} \tilde{a}_{\alpha } ;\)
-
3.
\(\tilde{a}_{\alpha } P\tilde{a}_{\beta }\) and \(\tilde{a}_{\beta } P\tilde{a}_{\alpha } ,\) that is, \(\tilde{a}_{\alpha } \sim_{I} \tilde{a}_{\beta } .\)
4 Extended ELECTRE I Method Based on Combination of the Choquet Integral with MHFNs
MCDM problems with multi-hesitant fuzzy information can be any number of alternatives and criteria. We represent the \(n\) alternatives and \(m\) criteria as \(A = \left\{ {\alpha_{1} ,\alpha_{2} , \ldots ,\alpha_{n} } \right\}\) and \(C = \left\{ {c_{1} ,c_{2} , \ldots ,c_{m} } \right\},\) respectively. The characteristics of the corresponding weight and data are denoted by \(w = \left( {w_{1} ,w_{2} , \ldots ,w_{m} } \right)\) and \(\alpha_{ij} = \left\{ {\gamma_{ij}^{k} ,k = 1,2, \ldots ,l_{{\alpha_{ij} }} } \right\}\)\(\left( {i = 1, \ldots ,n;\; j = 1, \ldots ,m} \right),\) respectively, where \(\alpha_{ij}\) is the evaluation value of the alternative \(\alpha_{i}\) for the criterion \(c_{j}\) given by the DMs and expressed by MHFNs, and \(l\left( {\alpha_{ij} } \right)\) denotes the number of elements in \(\alpha_{ij}\) and is constantly the number of DMs. In the decision-making process, DMs can anonymously assess these alternatives with regard to the criteria, while they cannot refuse providing the assessment values. Particularly, if two or more DMs provide the same value, then this value is counted several times. Consequently, \(\alpha_{ij}\) denotes the set of all assessment values provided by the DMs. In other words, if the decision-making institution consists of \(r\) DMs, then \(l_{{\alpha_{ij} }} = r,\) that is, the number of DMs is equal to the number of elements in MHFNs. Moreover, the order of elements in MHFNs does not influence decision-making results. Thereafter, these types of group decision-making problems can constantly be translated into MCDM problems. The implementation procedure of multi-hesitant fuzzy ELECTRE I method can be expressed as follows.
-
Step 1: The decision matrix is transformed into a normalised decision matrix.
Suppose that the decision-making matrix is denoted by \(R = \left( {\alpha_{ij} } \right)_{n \times m} ,\)\(\alpha_{ij} = \left\{ {\gamma_{ij}^{k} ,\;k = 1,2, \ldots ,l_{{\alpha_{ij} }} } \right\}\)\(\left( {i = 1,2, \ldots ,n;j = 1,2, \ldots ,m} \right)\) is MHFN and \(l\left( {\alpha_{ij} } \right)\) denotes the number of elements in \(\alpha_{ij} .\) The benefit criteria do not need to be transformed, that is, \(\tilde{\gamma }_{ij}^{k} = \gamma_{ij}^{k} ,k = 1,2, \ldots ,l_{{\alpha_{ij} }} .\) However, we should transform the cost criteria to gain an advantage by using \(\tilde{\gamma }_{ij}^{k} = 1 - \gamma_{ij}^{k} ,k = 1,2, \ldots ,l_{{\alpha_{ij} }} .\) The corresponding translated decision-making matrix is denoted by \(\tilde{R} = \left( {\tilde{\alpha }_{ij} } \right)_{n \times m} ,\) where \(\tilde{\alpha }_{ij} = \left\{ {\tilde{\gamma }_{ij}^{k} ,k = 1,2, \ldots ,l_{{\alpha_{ij} }} } \right\}\)\(\left( {i = 1,2, \ldots ,n;j = 1,2, \ldots ,m} \right).\) The translated values \(\tilde{\alpha }_{ij} = \left\{ {\tilde{\gamma }_{ij}^{1} ,\tilde{\gamma }_{ij}^{2} , \ldots ,\tilde{\gamma }_{ij}^{k} } \right\}\)\(\left( {i = 1,2, \ldots ,n;j = 1,2, \ldots ,m} \right)\) are constantly MHFNs.
-
Step 2: The weight of criterion is determined.
If \(X = \left\{ {x_{1} ,x_{2} , \ldots ,x_{n} } \right\}\) is a finite set, then \(\rho\)-fuzzy measure is provided as follows [52]:
Accordingly, \(\rho\) is determined from \(\mu \left( x \right) = 1,\) that is, \(\rho + 1 = \prod\limits_{i = 1}^{n} {\left( {1 + \rho \mu \left( i \right)} \right)} .\)
Therefore, the corresponding discrete Choquet integral can be presented as follows [16]:
where \(\left( {\delta \left( 1 \right),\delta \left( 2 \right), \ldots ,\delta \left( n \right)} \right)\) is a permutation of \(\left( {1,2, \ldots ,n} \right),\) satisfying \(0 \le f\left( {x_{\delta \left( 1 \right)} } \right) \le f\left( {x_{\delta \left( 2 \right)} } \right) \le \ldots \le f\left( {x_{\delta \left( n \right)} } \right),\)\(f\left( {x_{\delta \left( 0 \right)} } \right) = 0,\)\(A_{\delta \left( i \right)} = \left\{ {x_{\delta \left( i \right)} ,x_{{\delta \left( {i + 1} \right)}} , \ldots ,x_{\delta \left( n \right)} } \right\},\) and \(\mu \left( {A_{{\delta \left( {n + 1} \right)}} } \right) = 0.\)
Thus, the corresponding weight of the criterion is given as follows:
where \(\left( {\delta \left( 1 \right),\delta \left( 2 \right), \ldots ,\delta \left( m \right)} \right)\) is a permutation of \(\left( {1,2, \ldots ,m} \right)\) that satisfies \(0 \le \mu \left( {A_{\delta \left( 1 \right)} } \right) \le \mu \left( {A_{\delta \left( 2 \right)} } \right) \le \ldots \le \mu \left( {A_{\delta \left( m \right)} } \right),\;\;\mu \left( {A_{\delta \left( 0 \right)} } \right) = \mu \left( {A_{{\delta \left( {m + 1} \right)}} } \right) = 0\). Then, we can obtain the weight of criterion as \(w = \left( {w_{1} ,w_{2} , \ldots ,w_{m} } \right).\)
-
Step 3: The weighted normalised matrix is calculated.
In view of the weight of criterion, we can obtain the weighted normalised decision matrix \(\bar{R} = \left( {\bar{\alpha }_{ij} } \right)_{n \times m}\) as follows:
where \(w_{j}\) denotes the weight with regard to criterion j.
-
Step 4: The concordance and discordance subscript sets are determined.
The constraint \(\bar{\alpha }_{ij} P\bar{\alpha }_{qj}\) is used as basis to obtain the concordance subscript set as follows:
where \(\bar{\alpha }_{ij} P\bar{\alpha }_{qj}\) denotes \(\bar{\alpha }_{ij} >_{S} \bar{\alpha }_{qj}\) or \(\bar{\alpha }_{ij} >_{W} \bar{\alpha }_{qj}\) or \(\bar{\alpha }_{ij} \sim \bar{\alpha }_{qj} .\)
We can obtain the discordance subscript set by using the following equation based on the concordance subscript set.
-
Step 5: The multi-hesitant fuzzy concordance and discordance matrix is calculated.
We can obtain the following multi-hesitant fuzzy concordance index \(\tilde{C}\left( {\alpha_{i} ,\alpha_{k} } \right)\) by using the weight of criterion.
Thus, we can construct the multi-hesitant fuzzy concordance matrix \(\tilde{C}\) as follows:
The multi-hesitant fuzzy discordance index \(\tilde{D}\left( {\alpha_{i} ,\alpha_{s} } \right)\) is expressed as follows:
where \(d(\bar{\alpha }_{ij} ,\bar{\alpha }_{qj} ) = \frac{1}{2}\left( {\mathop {\hbox{max} }\limits_{{\bar{\gamma }_{{\bar{\alpha }_{ij} }} \in \bar{\alpha }_{ij} }} \mathop {\hbox{min} }\limits_{{\bar{\gamma }_{{\bar{\alpha }_{qj} }} \in \bar{\alpha }_{qj} }} \left| {\bar{\gamma }_{{\bar{\alpha }_{ij} }} - \bar{\gamma }_{{\bar{\alpha }_{qj} }} } \right| + \mathop {\hbox{max} }\limits_{{\bar{\gamma }_{{\bar{\alpha }_{qj} }} \in \bar{\alpha }_{qj} }} \mathop {\hbox{min} }\limits_{{\bar{\gamma }_{{\bar{\alpha }_{ij} }} \in \bar{\alpha }_{ij} }} \left| {\bar{\gamma }_{{\bar{\alpha }_{qj} }} - \bar{\gamma }_{{\bar{\alpha }_{ij} }} } \right|} \right)\) denotes the multi-hesitant Hamming–Hausdorff distance between \(\bar{\alpha }_{ij}\) and \(\bar{\alpha }_{qj} ;\) and \(\bar{\gamma }_{{\bar{\alpha }_{ij} }}\) and \(\bar{\gamma }_{{\bar{\alpha }_{qj} }}\) are any elements in \(\bar{\alpha }_{ij}\) and \(\bar{\alpha }_{qj} ,\) respectively.
Thus, the multi-hesitant fuzzy discordance matrix \(\tilde{D}\) is constructed as follows:
-
Step 6: The general concordance and discordance multi-hesitant fuzzy matrix is constructed.
For a preference threshold value \(\tilde{p},\) we can obtain the general concordance matrix as follows:
where \(\tilde{p}\) determines the superior possibility of \(\alpha_{i}\) with respect to \(\alpha_{k}\) and that can be determined based on the preference of the DM and \(\tilde{p} = \sum\nolimits_{i = 1}^{n} {\sum\nolimits_{k = 1}^{n} {{{\tilde{C}\left( {\alpha_{i} ,\alpha_{q} } \right)} \mathord{\left/ {\vphantom {{\tilde{C}\left( {\alpha_{i} ,\alpha_{q} } \right)} {n\left( {n - 1} \right)}}} \right. \kern-0pt} {n\left( {n - 1} \right)}}} } .\).
Similarly, for a veto threshold value \(\tilde{v}\), we can construct the general discordance matrix as follows:
where \(\tilde{v}\) determines the inferior possibility of \(\alpha_{i}\) with respect to \(\alpha_{q}\) and that can be determined based on the DMs and \(\tilde{d} = \sum\nolimits_{i = 1}^{n} {\sum\nolimits_{k = 1}^{n} {{{\tilde{D}\left( {\alpha_{i} ,\alpha_{q} } \right)} \mathord{\left/ {\vphantom {{\tilde{D}\left( {\alpha_{i} ,\alpha_{q} } \right)} {n\left( {n - 1} \right)}}} \right. \kern-0pt} {n\left( {n - 1} \right)}}} } .\)
-
Step 7: The multi-hesitant fuzzy outranking matrix is constructed.
We can construct the outranking matrix based on Step 6 as follows:
-
Step 8: All alternatives are ranked.
The outranking matrix \(\tilde{\pi }\) indicates that we can determine the partial ordering of the alternatives. If \(\tilde{\pi }_{iq} = 1,\) then \(\alpha_{i}\) is superior to \(\alpha_{q}\) in terms of the concordance and discordance indexes. However, \(\alpha_{i}\) may be dominated by other alternatives. Therefore, if \(\alpha_{i}\) is an effective alternative, then it should satisfy the following condition.
If the number of elements of the column equal to 1 is above 1, then the corresponding column is dominated by the row. Subsequently, we should remove the column that only has one element of 1. Moreover, the threshold values \(\tilde{p}\) and \(\tilde{v},\) which affect the final order, can be changed to obtain the optimal alternative.
5 Numerical Example
This section provides a multi-hesitant fuzzy MCDM problem for selecting a third-party logistics service provider (adapted from Wang et al. [53]).
Several companies have implemented logistics outsourcing due to its various advantages in terms of cost reduction, improved performance, enhanced core competitiveness and formation of strategic alliances via virtual organisations [53]. Nevertheless, the logistics outsourcing provider selection is a typical type of MCDM problem, involving several DMs and multiple interrelated criteria [53, 54]. For example, ABC company tends to select logistics outsourcing to expand its businesses and enhance core competencies. The company selects a third-party logistics service provider for logistics outsourcing. The company’s preliminary investigation indicates five candidate countries, which are denoted by \(\alpha_{1} ,\alpha_{2} , \ldots ,\alpha_{5} .\) Thereafter, three DMs, who are experienced in logistics outsourcing, are determined by the company to assist in decision-making. During the assessment processes, DMs should consider four factors, namely, \(c_{1}\) (service), \(c_{2}\) (relationship), \(c_{3}\) (quality), and \(c_{4}\) (information and equipment systems). The correlations of criteria are present in these four criteria. For example, service and quality can be considered redundant because the weight of the combination of service and quality is less than the sum of their weights. Moreover, the weight of three DMs is not taken into consideration. They can anonymously assess these criteria based on their knowledge and experience and can also provide their evaluation values for alternative \(a_{k}\) with criterion \(c_{l}\). Then, the set of evaluation values is expressed by MHFNs (i.e. \(\alpha_{ij} = \left\{ {\gamma_{ij}^{k} ,k = 1,2,3} \right\}\left( {i = 1,2,3,4,5;\;j = 1,2,3,4} \right)\)). If at least two DMs provide the same assessment value, then the evaluation value is counted repeatedly. Subsequently, the multi-hesitant fuzzy decision matrix can be constructed (see Table 1).
5.1 Illustration of the Multi-hesitant Fuzzy ELECTRE I Method
We used the proposed multi-hesitant fuzzy ELECTRE I method to achieve an optimal alternative as follows:
-
Step 1: The decision matrix is transformed into a normalised decision matrix.
Given that all the criteria are of benefit type, we can obtain the corresponding normalised matrix \(\tilde{R} = \left( {\tilde{\alpha }_{ij} } \right)_{5 \times 4} = \left( {\alpha_{ij} } \right)_{5 \times 4} .\)
-
Step 2: The weight of criterion is determined.
The fuzzy measures and criteria sets of \(C = \left\{ {c_{1} ,c_{2} ,c_{3} ,c_{4} } \right\}\) are used as bases to assume that \(\mu \left( {c_{1} } \right) = 0.4,\)\(\mu \left( {c_{2} } \right) = 0.25,\)\(\mu \left( {c_{3} } \right) = 0.37,\) and \(\mu \left( {c_{4} } \right) = 0.20.\) Then, \(\rho = - 0.44\) can be obtained. Equation (1) indicates that \(\mu \left( {c_{1} ,c_{2} } \right) = 0.52,\)\(\mu \left( {c_{1} ,c_{3} } \right) = 0.65,\)\(\mu \left( {c_{1} ,c_{4} } \right) = 0.50,\)\(\mu \left( {c_{2} ,c_{3} } \right) = 0.45,\)\(\mu \left( {c_{2} ,c_{4} } \right) = 0.34,\)\(\mu \left( {c_{3} ,c_{4} } \right) = 0.42,\)\(\mu \left( {c_{1} ,c_{2} ,c_{3} } \right) = 0.85,\)\(\mu \left( {c_{1} ,c_{2} ,c_{4} } \right) = 0.68,\)\(\mu \left( {c_{2} ,c_{3} ,c_{4} } \right) = 0.57,\)\(\mu \left( {c_{1} ,c_{3} ,c_{4} } \right) = 0.76,\) and \(\mu \left( {c_{1} ,c_{2} ,c_{3} ,c_{4} } \right) = 1.\)
With respect to the score function presented in Definition 5, \(\tau \left( {c_{1} } \right) = {{\left( {\tilde{s}\left( {a_{11} } \right) + \tilde{s}\left( {a_{21} } \right) + \tilde{s}\left( {a_{31} } \right) + \tilde{s}\left( {a_{41} } \right) + \tilde{s}\left( {a_{51} } \right)} \right)} \mathord{\left/ {\vphantom {{\left( {\tilde{s}\left( {a_{11} } \right) + \tilde{s}\left( {a_{21} } \right) + \tilde{s}\left( {a_{31} } \right) + \tilde{s}\left( {a_{41} } \right) + \tilde{s}\left( {a_{51} } \right)} \right)} 5}} \right. \kern-0pt} 5} = 0.6067\) can be obtained. Similarly, \(\tau \left( {c_{2} } \right) = 0.5133,\;\;\tau \left( {c_{3} } \right) = 0.6800\) and \(\tau \left( {c_{4} } \right) = 0.4933\). Subsequently, \(\tau \left( {c_{4} } \right) < \tau \left( {c_{2} } \right) < \tau \left( {c_{1} } \right) < \tau \left( {c_{3} } \right),\)\(c_{\sigma \left( 1 \right)} = c_{4} ,\;\)\(c_{\sigma \left( 2 \right)} = c_{2} ,\)\(c_{\sigma \left( 3 \right)} = c_{1}\) and \(c_{\sigma \left( 4 \right)} = c_{3} .\) Thus, the following results are provided.
Therefore, the weight vector is \(w = \left( {0.28,0.2,0.37,0.15} \right).\)
-
Step 3: The weighted normalised matrix is constructed.
We can construct the weighted normalised decision matrix \(\bar{R}\left( {\bar{\alpha }_{ij} } \right)_{5 \times 4}\) using the weight of criterion and Eq. (4) (see Table 2).
-
Step 4: The concordance and discordance subscript sets are determined.
We can obtain the concordance subscript sets from Eq. (5) as follows:
Then, the discordance subscript sets are presented as follows:
\(\emptyset\) denotes ‘empty’.
-
Step 5: The multi-hesitant fuzzy concordance and discordance matrix is constructed.
With regard to the weight of criterion, we can construct the multi-hesitant fuzzy concordance matrix as follows:
The multi-hesitant fuzzy discordance matrix is presented as follows:
Moreover, we can calculate the multi-hesitant fuzzy discordance index as follows:
Hence,
-
Step 6: The general multi-hesitant fuzzy concordance and discordance matrix is constructed.
We can use Eq. (11) to achieve the preference threshold value \(\tilde{p}\) as follows:
Thus, the general multi-hesitant fuzzy concordance matrix is given as follows:
Similarly, the veto threshold value \(\tilde{v}\) and general multi-hesitant fuzzy discordance matrix are calculated as follows:
-
Step 7: The multi-hesitant fuzzy outranking matrix is constructed.
In view of Step 6, we can construct the multi-hesitant fuzzy outranking matrix as follows:
-
Step 8: All alternatives are ranked.
The third column can be discarded given that it is the only column in matrix \(\pi\) that lacks at least one unit element. Then, we can change the threshold values \(\tilde{p}\) and \(\tilde{v}\) in Step 6, and alternative \(\alpha_{4}\) is arranged in the first place. Furthermore, \(\alpha_{5} ,\)\(\alpha_{1} ,\)\(\alpha_{2} ,\) and \(\alpha_{3}\) are arranged in the second to the fifth places, respectively. Thus, we have \(\alpha_{3} \prec \alpha_{2} \prec \alpha_{1} \prec \alpha_{5} \prec \alpha_{4} ;\) and the optimal alternative is \(\alpha_{4} ,\) whereas the worst alternative is \(\alpha_{3} .\)
5.2 Comparative Analysis and Discussion
This section compares the existing methods presented by Chen et al. [10], Yu et al. [19], Wei [27], Zhang et al. [29], Qin et al. [30], Yu [32], Xu and Xia [34], Zhang and Wei [38], Zhang and Xu [41], Peng et al. [49] and the proposed method. Although the method presented by Peng et al. [49] can directly handle multi-hesitant fuzzy information, however, others cannot handle the repeated values in the assessment process. Therefore, we conducted a comparative analysis by using the same application example, and the same value would be counted only once in Table 1.
Case 1
Comparing hesitant fuzzy methods with independent criteria.
The methods concerned cannot consider the correlation of the criteria. Therefore, the weight vector calculated using the proposed method is utilised for making comparison. Suppose that the weight vector of the criteria is \(w = \left( {0.28,0.20,0.37,0.15} \right),\) we can obtain the comparison results, as presented in Table 3.
The following remarks can be categorically drawn based on the results presented in Table 3. Firstly, the repetitive values in HFNs are disregarded in the majority of the existing methods. Secondly, in comparison with the methods based on aggregation operators, the result obtained by utilising the proposed method is different from that of Wei [27], Qin et al. [30] and Yu [32], while is the same as that of Zhang et al. [29]. Furthermore, DMs experience difficulty in making a judgement regarding these operators with similar characteristics. Thirdly, in comparison with the methods that rely on distance measures, the result obtained by utilising the proposed method is the same as that of Xu and Xia [34] and Zhang and Wei [38], whereas it is different from that of Zhang and Xu [41] because the methods using distance measures have certain shortcomings. That is, the condition should be satisfied that all HFNs not only must be placed in ascending sequence, but also have the same length. If the lengths of two HFNs are different, then the value of the short one should be increased until both lengths are equal. Although this method can determine the distance between HFNs, different results may be generated by adding different values. Therefore, this process may distort the original information provided. Eventually, the results obtained by the methods presented by Peng et al. [49] and Chen et al. [10] are found to be different from those of the proposed method. The former two methods often involve the ELECTRE, which are similar to the proposed method. However, the present study proposes an approach based on outranking relations that is different from the method introduced by Chen et al. [10], which is structured by combining the score function, deviation degree and existing distance. Moreover, Peng et al.’s method [49] was developed based on the ELECTRE III, whereas the proposed method was derived from the ELECTRE I. The former cannot consider the correlation of criteria, affecting the allocation of the weight of criterion and eventually influence the accuracy of the results. That is, the three methods consistently lead to different rankings.
Case 2
Comparing hesitant fuzzy methods with correlated criteria.
For Yu et al.’s method [19], the Choquet integral was used to obtain the weight of criterion to handle the correlation of criteria. The fuzzy measure \(\mu\) is used with these values. Then, we can obtain the comparison results, as presented in Table 4.
Table 4 shows that the final result, which was derived by using the proposed method, is consistent with Yu et al.’s method [19]. The correlation among the criteria is considered in two methods. However, the method presented by Yu et al. [19] involved aggregation operators, thereby possibly creating problems as previously discussed.
Thus, the primary advantages of the extended multi-hesitant fuzzy ELECTRE I method proposed in this study compared with the existing methods are as follows:
-
1.
The proposed method based on the ELECTRE can avoid deficiencies originating from methods based on different aggregation operators and distance measures.
-
2.
In some practical decision-making problems, various types of relationships existing among the criteria and the available methods cannot consider the interaction among criteria. However, the proposed method based on the Choquet integral has more flexibility than the others and is substantially appropriate for actual decision-making problems.
-
3.
For the compared methods, in which the collected hesitant fuzzy data are dependent on several DMs, the repetitive values cannot be taken within a group decision-making process into account. However, the assessment value which is counted more than once is fundamentally different from the assessment value that is counted only once. The repeated value can relatively express the inconsistent opinions of experts or DMs regarding their preferences, in addition to the importance of the alternative in the case of a contingency.
Thus, the comparative analyses demonstrate that the proposed method is potentially appropriate for practical applications. This method can assist DMs with numerous options and flexibilities to handle multi-hesitant fuzzy MCDM problems, where the criteria are interdependent.
6 Conclusions
This study proposes an extended multi-hesitant fuzzy ELECTRE I method based on the Choquet integral. The proposed method for the expression of multi-hesitant fuzzy information substantially reflects hesitation in an actual decision-making process. This study applies MHFNs as alternative ratings with respect to the criteria utilised by DMs. MHFNs better express the deep-seated hesitation manifested by DMs and collect more completed decision-making data than HFSs. The main advantages of the proposed multi-hesitant fuzzy ELECTRE I method compared with others are as follows. On the one hand, the proposed method’s consideration of outranking relations and the interaction among criteria can notably produce reasonable results that are substantially consistent with the preference of DMs. On the other hand, this method can also overcome the distortion of the original data that occurred previously. Thus, the final outcomes substantially approximate the actual decision-making problems. In the future research, the consensus [55, 56] approaches of MHFNs will be extended. We also will investigate the process of obtaining the threshold value by a specific model in a multi-hesitant fuzzy environment.
References
Benayoun, R., Roy, B., Sussman, B.: ELECTRE: Une méthode pour guider le choix en présence de points de vue multiples. Rev. franaise Informat. recherche Opérationnelle 3, 31–56 (1969)
Roy, B.: The outranking approach and the foundations of ELECTRE methods. Theor. Decis. 31(1), 49–73 (1991)
Rohaninejad, M., Kheirkhah, A., Fattahi, P., Vahedi-Nouri, B.: A hybrid multi-objective genetic algorithm based on the ELECTRE method for a capacitated flexible job shop scheduling problem. Int. J. Adv. Manuf. Technol. 77, 51–66 (2015)
Wang, X., Triantaphyllou, E.: Ranking irregularities when evaluating alternatives by using some ELECTRE methods. Omega 36(1), 45–63 (2008)
Ermatita, S., Hartati, S., Wardoyo, R., Harjoko, A.: Electre methods in solving group decision support system bioinformatics on gene mutation detection simulation. Int. J. Comput. Sci. Inf. Technol. 3(1), 40–52 (2011)
Hashemi, S.S., Hajiagha, S.H.R., Zavadskas, E.K., Mahdiraji, H.A.: Multicriteria group decision making with ELECTRE III method based on interval-valued intuitionistic fuzzy information. Appl. Math. Model. 40(2), 1554–1564 (2016)
Fahmi, A., Kahraman, C., Bilen, Ü.: ELECTRE I method using hesitant linguistic term sets: an application to supplier selection. Int. J. Comput. Intell. Syst. 9(1), 153–167 (2016)
Peng, H.G., Shen, K.W., He, S.S., Zhang, H.Y., Wang, J.Q.: Investment risk evaluation for new energy resources: an integrated decision support model based on regret theory and ELECTRE III. Energy Convers. Manag. 183, 332–348 (2019)
Hatami-Marbini, A., Tavana, M.: An extension of the Electre I method for group decision-making under a fuzzy environment. Omega 39(4), 373–386 (2011)
Chen, N., Xu, Z.S., Xia, M.M.: The ELECTRE I multi-criteria decision-making mtheod based on hesitant fuzzy sets. Int. J. Inf. Technol. Decis. Mak. 14(3), 621–657 (2015)
Chen, N., Xu, Z.S.: Hesitant fuzzy ELECTRE II approach: a new way to handle multi-criteria decision making problems. Inf. Sci. 292, 175–197 (2015)
Peng, J.J., Wang, J.Q., Wu, X.H.: An extension of the ELECTRE approach with multi-valued neutrosophic information. Neural Comput. Appl. 28(Suppl 1), S1011–S1022 (2017)
Grabisch, M.: Fuzzy integral in multicriteria decision making. Fuzzy Sets Syst. 69(3), 279–298 (1995)
Liang, R.X., Wang, J.Q., Li, L.: Multi-criteria group decision making method based on interdependent inputs of single valued trapezoidal neutrosophic information. Neural Comput. Appl. 30, 241–260 (2018)
Peng, H.G., Wang, X.K., Wang, T.L., Wang, J.Q.: Multi-criteria game model based on the pairwise comparisons of strategies with Z-numbers. Appl. Soft Comput. 74, 451–465 (2019)
Choquet, G.: Theory of capacities. Ann. Inst. Fourier 5, 131–295 (1954)
Yager, R.R.: Induced aggregation operators. Fuzzy Sets Syst. 137(1), 59–69 (2003)
Meyer, P., Roubens, M.: On the use of the Choquet integral with fuzzy numbers in multiple criteria decision support. Fuzzy Sets Syst. 157(7), 927–938 (2006)
Yu, D.J., Wu, Y.Y., Zhou, W.: Multi-criteria decision making based on Choquet integral under hesitant fuzzy environment. J. Comput. Inf. Syst. 7(12), 4506–4513 (2011)
Deepa, J., Sanjay, K.: Interval-valued intuitionistic hesitant fuzzy Choquet integral based TOPSIS method for multi-criteria group decision making. Eur. J. Oper. Res. 248(1), 183–191 (2016)
Wang, L., Zhang, H.Y., Wang, J.Q.: Frank Choquet Bonferroni mean operators of bipolar neutrosophic sets and their application to multi-criteria decision-making problems. Int. J. Fuzzy Syst. 20(1), 13–28 (2018)
Peng, J.J., Wang, J.Q., Hu, J.H., Tian, C.: Multi-criteria decision-making approach based on single-valued neutrosophic hesitant fuzzy geometric weighted Choquet integral heronian mean operator. J. Intell. Fuzzy Syst. 35(5), 1–14 (2018)
Torra, V.: Hesitant fuzzy sets. Int. J. Intell. Syst. 25(6), 529–539 (2010)
Torra, V., Narukawa, Y.: On hesitant fuzzy sets and decision. In: The 18th IEEE international conference on fuzzy systems, Jeju Island, Korea, pp. 1378–1382 (2009)
Zadeh, L.A.: Fuzzy sets. Inf. Control 8(3), 338–353 (1965)
Xia, M.M., Xu, Z.S.: Hesitant fuzzy information aggregation in decision making. Int. J. Approx. Reason. 52(3), 395–407 (2011)
Wei, G.W.: Hesitant fuzzy prioritized operators and their application to multiple attribute decision making. Knowl. Based Syst. 31(7), 176–182 (2012)
Zhao, H., Xu, Z., Cui, F.: Generalized hesitant fuzzy harmonic mean operators and their applications in group decision making. Int. J. Fuzzy Syst. 18(4), 685–696 (2016)
Zhang, Z.M., Wang, C., Tian, D.Z., Li, K.: Induced generalized hesitant fuzzy operators and their application to multiple attribute group decision making. Comput. Ind. Eng. 67(1), 116–138 (2014)
Qin, J., Liu, X., Pedrycz, W.: Frank aggregation operators and their application to hesitant fuzzy multiple attribute decision making. Appl. Soft Comput. 41, 428–452 (2015)
Zhang, Z.M.: Hesitant fuzzy power aggregation operators and their application to multiple attribute group decision making. Inf. Sci. 234(10), 150–181 (2013)
Yu, D.J.: Some hesitant fuzzy information aggregation operators based on Einstein operational laws. Int. J. Intell. Syst. 29(4), 320–340 (2014)
Qian, G., Wang, H., Feng, X.Q.: Generalized hesitant fuzzy sets and their application in decision support system. Knowl. Based Syst. 37(4), 357–365 (2013)
Xu, Z.S., Xia, M.M.: Distance and similarity measures for hesitant fuzzy sets. Inf. Sci. 181(11), 2128–2138 (2011)
Zhang, Z.M.: Hesitant fuzzy multi-criteria group decision making with unknown weight information. Int. J. Fuzzy Syst. 19(3), 615–636 (2017)
Farhadinia, B.: Distance and similarity measures for higher order hesitant fuzzy sets. Knowl. Based Syst. 55, 43–48 (2014)
Wei, C., Yan, F., Rodríguez, R.M.: Entropy measures for hesitant fuzzy sets and their application in multi-criteria decision-making. J. Intell. Fuzzy Syst. 31(1), 673–685 (2016)
Zhang, N., Wei, G.W.: Extension of VIKOR method for decision making problem based on hesitant fuzzy set. Appl. Math. Model. 37(7), 4938–4947 (2013)
Liang, R.X., Wang, J.Q., Zhang, H.Y.: Projection-based PROMETHEE methods based on hesitant fuzzy linguistic term sets. Int. J. Fuzzy Syst. 20(7), 2161–2174 (2018)
Sun, R.X., Hu, J.H., Zhou, J.D., Chen, X.H.: A hesitant fuzzy linguistic projection-based MABAC method for patients’ prioritization. Int. J. Fuzzy Syst. 20(7), 2144–2160 (2018)
Zhang, X.L., Xu, Z.S.: The TODIM analysis approach based on novel measured functions under hesitant fuzzy environment. Knowl. Based Syst. 61(2), 48–58 (2014)
Lin, Y., Wang, Y.M., Chen, S.Q.: Hesitant fuzzy multiattribute matching decision making based on regret theory with uncertain weights. Int. J. Fuzzy Syst. 19(4), 955–966 (2017)
Hu, J.H., Yang, Y., Zhang, X.L., Chen, X.H.: Similarity and entropy measures for hesitant fuzzy sets. Int. Trans. Oper. Res. 25(3), 857–886 (2018)
Liao, H.C., Xu, Z.S., Zeng, X.J.: Novel correlation coefficients between hesitant fuzzy sets and their application in decision making. Knowl. Based Syst. 82(C), 115–127 (2015)
Li, D.Q., Zeng, W.Y., Li, J.H.: New distance and similarity measures on hesitant fuzzy sets and their applications in multiple criteria decision making. Eng. Appl. Artif. Intell. 40, 11–16 (2015)
Farhadinia, B.: Weighted hesitant fuzzy information measures in decision making. J. Intell. Fuzzy Syst. 33(6), 1–10 (2017)
Khalid, A., Beg, I.: Incomplete hesitant fuzzy preference relations in group decision making. Int. J. Fuzzy Syst. 19(3), 637–645 (2017)
Zhang, Z.M.: A framework of group decision making with hesitant fuzzy preference relations based on multiplicative consistency. Int. J. Fuzzy Syst. 19(4), 982–996 (2017)
Peng, J.J., Wang, J.Q., Wang, J., Yang, L.J., Chen, X.H.: An extension of ELECTRE to multi-criteria decision-making problems with multi-hesitant fuzzy sets. Inf. Sci. 307(C), 113–126 (2015)
Ji, P., Zhang, H.Y., Wang, J.Q.: A projection-based outranking method with multi-hesitant fuzzy linguistic term sets for hotel location selection. Cogn. Comput. 10(5), 737–751 (2018)
Tian, Z.P., Wang, J.Q., Zhang, H.Y., Wang, T.L.: Signed distance-based consensus in multi-criteria group decision-making with multi-granular hesitant unbalanced linguistic information. Comput. Ind. Eng. 124, 125–138 (2018)
Wang, Z., Klir, G.J.: Fuzzy measure theory. Plenum press, New York (1992)
Wang, J., Wang, J.Q., Tian, Z.-P., Zhao, D.Y.: A multi-hesitant fuzzy linguistic multi-criteria decision-making approach for logistics outsourcing with incomplete weight information. Int. Trans. Oper. Res. 25(3), 831–856 (2018)
Wan, S.P., Wang, F., Lin, L.L., Dong, J.Y.: An intuitionistic fuzzy linear programming method for logistics outsourcing provider selection. Knowl. Based Syst. 82, 80–94 (2015)
Cabrerizo, F.J., Chiclana, F., Al-Hmouz, R., Morfeq, A., Balamash, A.S., Herrera-Viedma, E.: Fuzzy decision making and consensus: challenges. J. Intell. Fuzzy Syst. 29(3), 1109–1118 (2015)
Xu, Y., Cabrerizo, F.J., Herrera-Viedma, E.: A consensus model for hesitant fuzzy preference relations and its application in water allocation management. Appl. Soft Comput. 58, 265–284 (2017)
Acknowledgements
The authors would like to acknowledge the editors and anonymous referees for their valuable and constructive comments and suggestions that immensely facilitated the improvement of this paper. This study was supported by the National Natural Science Foundation of China (Nos. 71701065 and 71871228), and China Postdoctoral Science Foundation (No. 2017M610511).
Author information
Authors and Affiliations
Corresponding author
Rights and permissions
About this article
Cite this article
Peng, Jj., Wang, Jq. & Wu, Xh. Extended ELECTRE I Method with Multi-hesitant Fuzzy Information. Int. J. Fuzzy Syst. 21, 2192–2203 (2019). https://doi.org/10.1007/s40815-019-00716-9
Received:
Revised:
Accepted:
Published:
Issue Date:
DOI: https://doi.org/10.1007/s40815-019-00716-9