Abstract
Spatial variations of the water quality in the Haicheng River during April and October 2009 were evaluated for the national monitoring program on water pollution control and treatment in China. The spatial autocorrelation analysis with lower Moran’s I values displayed the spatial heterogeneity of the 12 physicochemical parameters among all the sampling sites of the river. The one-way ANOVA showed that all variables at different sampling sites had significant spatial differences (p < 0.01). Based on the similarity of water quality characteristics, cluster analysis grouped the 20 sampling sites into three clusters, related with less polluted, moderately polluted and highly polluted sites. The factor analysis extracted three major factors explaining 76.4 % of the total variance in the water quality data set, i.e., integrated pollution factor, nitrogen pollution factor and physical factor. The results revealed that the river has been severely polluted by organic matter and nitrogen. The major sources leading to water quality deterioration are complex and ascribed to anthropogenic activities, e.g., domestic and industrial wastewater discharges, agricultural runoff, and animal rearing practices.
Similar content being viewed by others
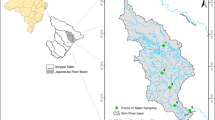
Explore related subjects
Discover the latest articles, news and stories from top researchers in related subjects.Avoid common mistakes on your manuscript.
Introduction
China has been increasingly facing severe water deficiency, especially in the northern part of the country (Bu et al. 2010). China’s water scarcity is attributed to insufficient local water resources as well as reduced water quality due to increasing pollution (Jiang 2009), especially agricultural non-point source pollution (Ongley 2004; Wang 2006). Meanwhile, poor water quality further exacerbates the shortage of water availability in water-scarce areas (Zhu et al. 2001; Liu and Diamond 2005). The water shortage in northeastern China has caused serious adverse impacts on society, economics and environment. Therefore, it is an urgent need for water quality protection to alleviate the pressure on water shortage. Accordingly, a national monitoring program on water pollution control and treatment in China has been carried out since 2008.
Characterization of the spatial variation of water quality variables can provide useful information of the environmental conditions (Huang et al. 2009) and help researchers identify the pollution sources for available water management (Chang 2008). Such researches on spatial variation of river water quality have been reported around the world in recent years. Perona et al. (1999) analyzed the environmental factors associated with the physicochemical variability in a Spanish River. Chang (2008) determined the significant spatial trends for water quality data at different scales in the Han River basin, South Korea. In other studies for spatial variations on water quality, the pollution sources and potential influences by natural and anthropogenic inputs were also identified (Pillsbury and Byrne 2007; Kannel et al. 2007; Mendiguchía et al. 2007).
In this study, the Haicheng River, as a tributary of the lower Liao River in Northeast China was selected to examine the water quality for its representativeness in water quality deterioration due to agricultural and industrial development in Northeast China. The pollution sources of the river water are mainly generated from agricultural fertilizers, animal wastes, domestic sewage and industrial wastewater from textile printing and dyeing, and paper industry. The objectives of the study are to (1) reveal spatial variations in water quality characteristics, (2) identify the major pollution factors and sources influencing the river water quality, and (3) determine the physicochemical variables affecting spatial variations of water quality in the Haicheng River. The results obtained from this research could provide useful information for water quality control in the Liao River basin.
Materials and methods
Study area
The Haicheng River (40.48º–41.01º N, 122.48º–123.14º E) is one of the largest tributaries of the Taizi River in the lower reaches of the Liao River basin, located in Haicheng City, Liaoning Province of China (Fig. 1). It drains a total length of 87.5 km and covers a total area of 1,249.3 km2. The river stems from the Mt. Xiongdi in the southeast of Gushan Town, and then flows into Chagou Town, Ximu Town, Haicheng City, Dongsi Town, Zhongxiao Town and Niuzhuang Town, finally discharges into the Taizi River (Fig. 1).
The Haicheng River basin is in a warm temperate monsoon climate zone. The annual average temperature is approximately 10.4 °C, with the highest in summer and the lowest in winter. The annual average rainfall is 701.7 mm, most of which occurs in the summer season (from June to September). The multi-annual average discharge measured in the whole river is 2.17 m3/s. Due to the seasonality of the river, the changes of the river discharges are consistent with the variation of rainfall, with the maximum discharge occurring during the rainy season. Vegetation coverage in the river basin reaches to 52.9 % of the drainage area, including forest land (51.5 %) and grass land (1.4 %). Agricultural land covers 34.3 % of the total area along the course of the river, while built-up land centralized in downstream of the river covers 10.3 %.
Water sampling and analytical methods
Water samples were collected in April and October 2009, and 20 sampling sites (H1–H20) in the mainstream of the Haicheng River were selected (Fig. 1). The first site H1 is near the headstream of the Haicheng River. Sites of H2–H9 are located in the upstream of the river. Sites H10–H14 are distributed in the midstream of the river, where non-point source pollutions (i.e., agricultural activities) also contribute to the river water pollution besides local domestic wastewater. Sites of H15–H20 lie in the downstream of the river, where municipal and industrial wastewater and partial runoff from agricultural lands and livestock rearing farms are mainly received.
Twelve water quality parameters are chosen for the water chemistry data set obtained at the 20 sampling sites in the Haicheng River basin. Water temperature (temp), pH, dissolved oxygen (DO), electrical conductivity (EC) and total dissolved solids (TDS) were directly determined in situ using multiparameter water quality monitoring instrument (YSI Incorporated, Yellow Springs, OH, USA). Calibration of sensors was performed before measurement. The discharge (Q) values used in this study were measured at the sampling sites by a current meter.
Water samples at each site were collected in polyethylene plastic bottles rinsed three times prior to use with distilled water and kept at 4 °C for laboratory analysis of total suspended solids (TSS), chemical oxygen demand (Cr) (CODCr), ammoniacal nitrogen (NH3–N), nitrate nitrogen (NO3–N), total nitrogen (TN) and total dissolved phosphorus (TP). Pretreatment and determination for these parameters analyzed in the laboratory were all conducted following the national standard methods (SEPA 2002). Before analysis for NH3–N, NO3–N and TN, water samples were acidified to pH < 2 by sulfuric acid, while samples for TP analysis were acidified to pH < 2 with nitric acid. TSS was determined gravimetrically at 103–105 °C by filtration with 0.45 μm MF-Millipore membrane filters. CODCr was measured by potassium dichromate method. NH3–N was measured with Nessler’s reagent, NO3–N and TN determined by UV spectrophotometric method, respectively. TP was analyzed by digestion and a colorimetric method (ammonium molybdenum blue method/ascorbic acid method) after the digestion of samples with concentrated nitric and sulfuric acid to convert all the phosphates into the orthophosphate form (SEPA 2002; Kannel et al. 2008).
Statistical analysis
In order to see if the values of observations in each sample were independent of one another, the spatial autocorrelations were performed using the GeoDa 0.9.5-i software (Anselin et al. 2006). One-way analysis of variance (ANOVA), cluster analysis (CA) and factor analysis (FA) were performed to further explain the monitoring data using SPSS 13.0 (Wunderlin et al. 2001; Simeonov et al. 2003; Bu et al. 2010). The one-way ANOVA with least significant difference (LSD) method in post hoc tests and the Levene statistic in homogeneity of variance test was applied to estimate the spatial differences of the river water quality among the 20 sampling sites (Mendiguchía et al. 2007). For abnormally distributed and unequal-variance variables (Temp, pH, DO, EC and TDS), non-parametric Dunnett’s test was used. The CA using the squared Euclidean distance with Ward’s method was also applied to assess the similarity among the sampling sites (Kazi et al. 2009). The FA was used to identify pollution factors affecting water quality. Kaiser–Meyer–Olkin (KMO) and Bartlett’s sphericity tests were also conducted to examine the suitability of the data for FA (Helena et al. 2000). For CA and FA, the water quality data had been initially standardized by z-scale transformation in order to avoid misclassification due to wide differences in data units and dimensionality (Singh et al. 2005; Kannel et al. 2007). A detailed description of the CA and FA can be found in a recent paper (Bu et al. 2010).
Results and discussion
Spatial heterogeneity of water quality
The results from the spatial autocorrelation analysis show the Moran’s I values (Table 1) range from −0.122 (NO3–N) to 0.575 (pH). There are weak to moderate positive and negative spatial autocorrelations among all the sampling sites. Therefore, it displays the spatial heterogeneity of chemical parameters along the course of the river, and also indicates there is no noise to interpret the spatial water quality trends (Chang 2008). By the one-way ANOVA, all variables show significant spatial differences (p < 0.01) at different sampling sites, which is consistent with the results from the spatial autocorrelation analysis.
Cluster analysis and spatial similarity
Based on the 12 variables, the 20 sampling sites are classified into three distinct clusters at (D link/D max) × 25 < 10 by cluster analysis, illustrated as a dendrogram (Fig. 2). Comparing the values of the 12 variables in different clusters (Fig. 3), the water qualities are significantly different (p < 0.001) by the one-way ANOVA except the variables of pH and NH3–N. Thus, the three clusters correspond to relatively less polluted sites (LPS), moderately polluted sites (MPS) and highly polluted sites (HPS), respectively.
Variations of the 12 parameters in different clusters (LPS, MPS and HPS) in the Haicheng River, China (open circle and asterisk denote outliers with 1.5 × interquartile range (IQR) and 3 × IQR, respectively; (LPS, MPS and HPS denote less polluted sites, moderately polluted sites and highly polluted sites, respectively)
In cluster 1, four sampling sites (H3, H4, H7 and H8) are included and situated at the upper reaches of the river, where human activities are minimal and the water quality is less influenced (Bu et al. 2010). The mean values of EC, TDS, TSS, CODCr, NH3–N and TN in cluster 1 are all lowest among the three clusters (Fig. 3), reaching to 444 μS/cm, 176.5, 8.8, 14.1, 0.36 and 1.24 mg/l, respectively.
While cluster 3, covering only two sampling sites of H19 and H20 at the down reaches of the river, was named HPS since its mean concentrations of EC, TDS, TSS, NH3–N, CODCr and TP all reach to the largest values of 1,083 μS/cm, 582, 95, 1.2, 151 and 0.4 mg/l among the three clusters (Fig. 3). The water discharges (Q) in cluster 3 are also distinctly higher (1.68 m3/s) than other two clusters (Fig. 3), which may be receiving wastewater and local pollutions mostly from industrial effluents, agricultural runoff and partially domestic wastewater in these river reaches. It was reported that about 8.5 × 106 tons of industrial wastewater generated from textile printing and dyeing, and paper industry was discharged to the river in 2009 (EPAHCLPC 2010), including 1.3 × 103 tons of CODCr and 22.0 tons of NH3–N. The massive amounts of industrial wastewater contribute to the organic and nitrogen pollution of the river. In cluster 3, the mean concentrations of CODCr and TN (151 and 2.4 mg/l, respectively; Fig. 3) far more exceed their Class V guidelines of 40 and 2.0 mg/l, respectively, according to the national quality standards for surface waters, China (GB3838-2002).
The other 14 sampling sites (H1, H2, H5, H6 and H9–H18) lie in the different reaches of the river are ascribed to cluster 2 and called as MPS for their moderate values of water quality variables (Fig. 3). Of these sampling sites, H1, H2, H5, H6, H9–H14 and H17 are located in rural areas, where pollutions are mostly derived from local agricultural practices in the vicinity of the Haicheng River. In 2009, about 5.6 × 104 tons of fertilizers were applied to agricultural lands in the Haicheng River basin (Environmental Protection Agency of the Haicheng City of Liaoning Province, China (EPAHCLPC) 2010). However, only 30–35 % of fertilizers can be used by crops (Bu et al. 2011). Thus, the excessive fertilizers influenced the river water quality through surface runoff and/or direct volatilization. In addition, more than 95.7 × 104 tons of animal wastes in livestock rearing farms were also produced and contributed to river pollution during 2009 (EPAHCLPC 2010). Sites of H15, H16 and H18 are mainly polluted by municipal wastewater of Haicheng City and Zhongxiao Town. It was estimated that about 14.8 × 106 tons of domestic wastewater directly discharged into the river during 2009 (EPAHCLPC 2010), including 4.1 × 103 tons of COD and 388 tons of NH3–N.
The results of cluster analysis indicate that the CA technique in the present study is very useful in offering reliable classification of surface waters, and it can reduce sampling sites for further study by designing a future spatial sampling strategy in an optimal manner (Bu et al. 2010). Many successful applications of CA on water quality studies have been introduced in recent years (Simeonov et al. 2003; Singh et al. 2004, 2005; Shrestha and Kazama 2007; Kazi et al. 2009), providing good experience for similar researches.
Factor analysis and source identification
In factor analysis, the correlation matrix shows that most variables are significantly correlated with each other (p < 0.01 or p < 0.05; Table 2). Their correlations display that the variance of each variable could be well explained by others (Singh et al. 2005). The results from the KMO and Bartlett’s sphericity test are 0.841 and 212.4 (df = 66, p < 0.001), respectively, suggesting that factor analysis is effective to reduce the dimensionality of the data set. Accordingly, the first three rotated factors with eigenvalues ≥1 are extracted, explaining 76.4 % of the total variance in water quality data set (Table 3).
Factor 1 explaining 37.5 % of the total variance has strong negative loading on DO (−0.946), while strong positive loadings on EC, TDS, TSS, CODCr and TP. These variables in factor 1 represent the total pollutions and chemical changes along the course of the river (Perona et al. 1999). Thus, factor 1 can be named as integrated pollution factor. Factor 2 explaining 21.3 % of the total variance shows strong positive loadings on NH3–N, NO3–N and TN, reflecting the nutrient enrichment for nitrogen in river water. So, factor 2 is called nitrogen pollution factor. Factor 3 explaining about 17.6 % of the total variance is highly negatively correlated with Temp and positively associated with pH and Q. This factor reflects the physical characteristic of the river water (Bu et al. 2010), named physical factor since the three variables are the main reaction conditions in chemical changes of water body in rivers (Zilberbran et al. 2001). In aquatic environment, water temperature will impact on the acceptability of a number of other inorganic constituents and chemical contaminants (WHO 2008), while pH can not only regulate redox process but also control nitrification and denitrification of organic matter (Jain 2002). The river discharges influence the self-purified capacity by dilution effects due to variation in river flow (Perona et al. 1999). Consequently, the factor 3, to some degree, dominates the main pollutant concentrations of dissolved matters in river water, such as CODCr, TDS and nutrient elements.
The space distributions of the 20 sampling sites defined by the first two factor scores (explaining 58.8 % of the total variance, Table 3) are illustrated in Fig. 4, showing the similar three clusters from CA (Fig. 2). The higher the factor scores, the higher the factor’s influence (Felipe-Sotelo et al. 2007). Cluster 3 (sampling sites of H19 and H20) obtains the highest factor scores (>2.5; Fig. 4) in factor 1, firmly corresponding to variables of DO, EC, TDS, TSS, CODCr and TP since the two sampling sites show greater values of EC, TDS, TSS, CODCr and TP and lower concentrations of DO (Fig. 3). This result indicates that anthropogenic pollution sources for the two sampling sites are complicated, not only from domestic wastewater and agricultural runoff but also from industrial wastewater (Bu et al. 2011). The sampling sites of H2, H15 and H17 in cluster 2 load with much higher factor scores in factor 2 (>1.0; Fig. 4) associated with nitrogen variables of NH3–N, NO3–N and TN, showing nitrogen pollution mainly from agricultural runoff (H2 and H17) and domestic wastewater (H15) without treatment since agricultural lands are scattered in these areas and domestic wastewater from the Haicheng City directly discharges downstream by way of the sampling site H15 (Fig. 1). In addition, NO3–N in these sampling sites is the main pollution form of nitrogen and accounts for 58.2 (H15), 73.9 (H17) and 86.5 % (H2) of TN concentrations, respectively; while the second largest portion is NH3–N with the proportions from 11.1 (H2) to 32.1 % (H15) in TN (Fig. 5). However, cluster 1 (sites H3, H4, H7 and H8) has the lowest factor scores in the two factors (Fig. 4), displaying the much better water quality there. Finally, the Haicheng River pollution is centralized in lower reaches with higher total factor scores (Fig. 6).
Conclusions
This monitoring in the Haicheng River (a tributary of the Liao River) is an important part for the national monitoring program on water pollution control and treatment in China. The 12 studied variables show significant spatial variations. Three clusters are classified in CA, representing LPS, MPS and HPS, respectively. Three factors are extracted in FA, regarded as integrated pollution factor, nitrogen pollution factor and physical factor. The results indicate that the Haicheng River, as a major tributary of the Liao River, is subject to severe organic and nitrogen pollution due to anthropogenic activities, such as industrial discharges, agricultural activities, animal rearing practices, etc. However, the pollution sources are not individually distinguished from current conditions in this study. Further studies for the river pollution are needed. This research provides a basis for the knowledge of water quality characteristics in the Haicheng River and a reference for pollution control of the Liao River in the future.
References
Anselin L, Syabri L, Kho Y (2006) GeoDa: an introduction to spatial data analysis. Geogr Anal 38:5–22
Bu H, Tan X, Li S, Zhang Q (2010) Water quality assessment of the Jinshui River (China) using multivariate statistical techniques. Environ Earth Sci 60:1631–1639
Bu H, Meng W, Zhang Y (2011) Nitrogen pollution and source identification in the Haicheng River basin in Northeast China. Sci Total Environ 409:3394–3402
Chang H (2008) Spatial analysis of water quality trends in the Han River basin, South Korea. Water Res 42:3285–3304
Environmental Protection Agency of the Haicheng City of Liaoning Province, China (EPAHCLPC) (2010) Environmental quality report in Haicheng City during 2009. Haicheng (in chinese)
Felipe-Sotelo M, Andrade JM, Carlosena A, Tauler R (2007) Temporal characterization of river waters in urban and semi-urban areas using physico-chemical parameters and chemometric methods. Anal Chim Acta 583:128–137
Helena B, Pardo R, Vega M, Barrado E, Fernandez JM, Fernandez L (2000) Temporal evolution of groundwater composition in an alluvial aquifer (Pisuerga River, Spain) by principal component analysis. Water Res 34:807–816
Huang F, Wang X, Lou L, Zhou Z, Wu J (2009) Spatial variation and source apportionment of water pollution in Qiantang River (China) using statistical techniques. Water Res 44:1562–1572
Jain CK (2002) A hydro-chemical study of a mountainous watershed: the Ganga, India. Water Res 36:1262–1274
Jiang Y (2009) China’s water scarcity. J Environ Manage 90:3185–3196
Kannel PR, Lee S, Kanel SR, Khan SP (2007) Chemometric application in classification and assessment of monitoring locations of an urban river system. Anal Chim Acta 582:390–399
Kannel PR, Lee S, Lee YS (2008) Assessment of spatial–temporal patterns of surface and ground water qualities and factors influencing management strategy of groundwater system in an urban river corridor of Nepal. J Environ Manage 86:595–604
Kazi TG, Arain MB, Jamali MK, Jalbani N, Afridi HI, Sarfraz RA, Baig JA, Shah AQ (2009) Assessment of water quality of polluted lake using multivariate statistical techniques: a case study. Ecotoxicol Environ Saf 72:301–309
Liu J, Diamond J (2005) China’s environment in a globalizing world. Nature 435:1179–1186
Mendiguchía C, Moreno C, García-Vargas M (2007) Evaluation of natural and anthropogenic influences on the Guadalquivir River (Spain) by dissolved heavy metals and nutrients. Chemosphere 69:1509–1517
Ongley ED (2004) Non-point source water pollution in China: current status and future prospects. Water Int 29:299–306
Perona E, Bonilla I, Mateo P (1999) Spatial and temporal changes in water quality in a Spanish river. Sci Total Environ 241:75–90
Pillsbury LA, Byrne RH (2007) Spatial and temporal chemical variability in the Hillsborough River system. Mar Chem 104:4–16
Shrestha S, Kazama F (2007) Assessment of surface water quality using multivariate statistical techniques: a case study of the Fuji river basin, Japan. Environ Model Softw 22:464–475
Simeonov V, Stratis JA, Samara C, Zachariadis G, Voutsa D, Anthemidis A, Sofoniou M, Kouimtzis T (2003) Assessment of the surface water quality in Northern Greece. Water Res 37:4119–4124
Singh KP, Malik A, Mohan D, Sinha S (2004) Multivariate statistical techniques for the evaluation of spatial and temporal variations in water quality of Gomti River (India)—a case study. Water Res 38:3980–3992
Singh KP, Malik A, Sinha S (2005) Water quality assessment and apportionment of pollution sources of Gomti River (India) using multivariate statistical techniques: a case study. Anal Chim Acta 538:355–374
State Environmental Protection Administration (SEPA) (2002) Standard methods for the examination of water and wastewater, version 4. China Environmental Science Publish Press, Beijing (in Chinese)
Wang X (2006) Management of agricultural non-point source pollution in China: current status and challenge. Water Sci Technol 53:1–9
World Health Organization (WHO) (2008) Guidelines for drinking-water quality, 3rd edn, incorporating the first and second addenda, Vol 1—Recommendations. Geneva
Wunderlin DA, Diaz MP, Ame MV, Pesce SF, Hued AC, Bistoni MA (2001) Pattern recognition techniques for the evaluation of spatial and temporal variation in water quality—a case study: Suquia River basin (Cordoba Argentina). Water Res 35:2881–2894
Zhu Z, Zhou H, Ouyang T, Deng Q, Kuang Y, Huang N (2001) Water shortage: a serious problem in sustainable development of China. Int J Sustain Dev World 8:233–237
Zilberbran M, Rosenthal E, Shachnai E (2001) Impact of urbanization on hydro-chemical evolution of groundwater and on unsaturated-zone gas composition in the coastal city of Tel Aviv, Israel. J Contam Hydrol 50:175–208
Acknowledgments
This research was supported by the “Major Science and Technology Program for Water Pollution Control and Treatment in China” (Grant No. 2008ZX07526-001). The authors express their sincere gratitude to Drs. Weijing Kong and Nan Zhang for their valuable discussion, Xiaobo Jia and Libin Chen for their assistance during the fieldwork, and anonymous reviewers for their valuable comments.
Author information
Authors and Affiliations
Corresponding authors
Rights and permissions
About this article
Cite this article
Bu, H., Wan, J., Zhang, Y. et al. Spatial characteristics of surface water quality in the Haicheng River (Liao River basin) in Northeast China. Environ Earth Sci 70, 2865–2872 (2013). https://doi.org/10.1007/s12665-013-2348-5
Received:
Accepted:
Published:
Issue Date:
DOI: https://doi.org/10.1007/s12665-013-2348-5