Abstract
Joint probability behavior of droughts is important for China due to the fact that China is the agricultural country with the largest population in the world and it is particularly the case in the backdrop of intensifying weather extremes in a warming climate. In this case, regionalization of droughts is done using Fuzzy C- Means (FCM) clustering technique and also multivariate L-moment method. Besides, copula is used to estimate regional joint probability in terms of drought duration and severity. Evaluation of uncertainty in the joint probability curves is done using the Bootstrap resampling technique. The results indicate that: (1) five homogenous regions of droughts are subdivided. Regionalization in this study clarified the changing properties or nature of droughts, i.e., the blurred or ambiguous boundaries of the drought-impacted regions; (2) droughts in the northwest China are characterized by longer drought duration and larger drought severity, and the occurrence of the droughts in the northwest China is subject to be higher due to longer waiting time between drought events. Adverse is found for changes of droughts in the southeast China. The droughts in the north China are moderate in terms of drought duration and severity and also waiting time between drought events when compared to those in the northwest and southeast China; (3) the regional joint frequency curves are obtained with respect to drought duration and severity using the bivariate copula functions. Then the joint probabilities of droughts can be calculated using the regional probability curves and also results of mean drought duration, drought severity and waiting time between drought events. Furthermore, droughts in the regions without meteorological data can also be estimated in terms of joint probability using index-drought method proposed in this study. This study will provides theoretical and practical grounds for development and enhancement of human mitigation to drought hazards in China, and is of great importance in terms of planning and management of water resources and agricultural activities in the backdrop of intensifying weather extremes under the influences of warming climate.
Similar content being viewed by others
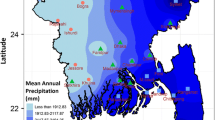
Avoid common mistakes on your manuscript.
1 Introduction
Drought is among the most complex climatic phenomena affecting society and the environment (Wilhite 1993), and is also perceived as one of the most expensive and least understood natural disasters (Kao and Govindaraju 2010). The complexity of drought is compounded by its identification based on its effect or impact on different types of systems, such as agriculture, water resources, ecology, forestry, economy, and so on (Vicente-Serrano et al. 2012a, b). Also, it is hard to determine the moment when a drought starts and ends and hence to quantify its duration, magnitude, and spatial extent (Wilhite and Buchanan-Smith 2005). Therefore, much effort has been devoted to provide a quantitative evaluation of drought. Occurrences, changing characteristics and risk evaluation of droughts have been receiving increasing concerns in recent years (e.g., Bazrafshan, J. et al. 2014; Ganguli and Reddy 2014; Hao and AghaKouchak 2013; Vicente-Serrano et al. 2014; Yusof, F. et al. 2013; Zhang et al. 2012, 2013, 2014). And also much attention has been paid on the drought mitigation (e.g., Rossi, G. 2009; Rossi, G. and Cancelliere, A. 2013; Tsakiris, G. et al. 2013).
Actually, environmental droughts generally include (Heim 2002): a) meteorological drought, b) hydrological drought, and c) agricultural drought. This study focuses on the meteorological drought which is defined by the lack of precipitation over a region for a period of time. Besides, there is a multitude of drought indices that have been defined to monitor drought conditions at a regional or global scale. Every index has its own strengths and weaknesses and it is hard to conclude that which drought index is the best. Mishra and Singh (2010) have presented a comprehensive review of the different drought indices summarizing their usefulness and limitations.
One of the first and most highly used drought indices is the Palmer drought severity index (PDSI; Palmer 1965; McEvoy et al. 2012), which is based on a simplified soil-water balance. However, this technique lacks the ability to detect drought for a wide range of time scales as Standardized Precipitation Index (SPI) (Vicente-Serrano et al. 2010). It is commonly accepted that drought is a multiscalar phenomenon, so the SPI has also been widely used. However, the main criticism of the SPI is that its calculation is based only on precipitation data. To consider the influence of potential evapotranspiration simultaneously, the Reconnaissance Drought Index (RDI) has been proposed by Tsakiris et al. (2007). Afterwards, the Standardized Precipitation-Evapotranspiration Index (SPEI) has also been introduced by Vicente-Serrano et al. (2010). Theoretically similar to SPI, SPEI is based on the accumulated difference between rainfall and potential evapotranspiration (PET) instead of the accumulated rainfall. The use of SPEI in drought monitoring has two main advantages (Spinoni et al. 2013): it has a better connection to soil water balance than SPI and it also considers temperature (used to compute PET), which is important in a changing environment. Hence, SPEI has been widely used in drought monitoring practice (e.g., Potop et al. 2014). In this study, SPEI was used for drought monitoring across China.
Water resources in China are extremely uneven in terms of spatiotemporal distribution, with northwest China being dry and southeast China wet. This unevenness takes on an added significance, because China is the third largest country in the world in terms of territorial area with the largest population and booming economy. Under the influence of climate change, intensifying human activities and rapidly developing economy also degrade water resources. Further, China is the largest agricultural country in the world and development of agriculture heavily depends on the availability of water resources. Therefore, it is important to evaluate the risk of drought in China and its societal response, particularly for agriculture. However, drought risks, based on SPEI method from a multivariate perspective using copula functions, have not been evaluated so far. In particular, climate across China is complicated due to complex topography properties and various underlying features. Therefore, regionalization of climate and regional frequency of droughts should be done to evaluate drought risks across China. Furthermore, to evaluate the validity and reliability of regional frequency analysis results, uncertainty in the estimation of joint probability curves for each homogeneous sub-region is also assessed using the bootstrap resampling technique. This study will be important to evaluate drought response to climate change and planning and management of water resources and agricultural activities.
The objectives of this study therefore are: (1) to classify the territory of China into subdivisions with homogenous climate change and drought; (2) to develop joint probability curves to quantify drought risks; (3) to evaluate the uncertainty of drought analysis; and (4) to evaluate drought frequency, duration and severity for each climatic subdivision. Results of this study will provide a background for developing measures for mitigation of drought hazards and management and planning of water resources and agricultural activities across China.
2 Data
Daily meteorological data, such as precipitation, temperature, relative humidity and so on, for a period of 1960–2005 from 588 stations were analyzed. The data were obtained from the National Meteorological Information Center of China Meteorological Administration that exercises strict quality control of data. The spatial distribution of rain gauge stations is shown in Fig. 1. The missing data for 1 day or 2 days were filled in by the average values of neighbouring days. If consecutive days had missing data, the missing values were filled in with long term averages of the same days (Zhang et al. 2011). Figure 1 shows sparse distribution of meteorological stations in the southern parts of northwest China and also in the southern parts of the Tibet Plateau. Thus, drought changes of these regions are not discussed in this study.
3 Methodology
3.1 Standardized Precipitation Evapotranspiration Index (SPEI)
The SPEI (Vicente-Serrano et al. 2010) is a multi-scalar drought index based on climatic data. It is used to quantify the onset, duration and magnitude of drought regimes in terms of normal conditions in a variety of natural and managed systems, such as crops, ecosystems, rivers, and water resources. Compared to the SPI technique, SPEI includes temperature in drought analysis which can represent the true drought conditions of the study region under the influences of warming climate.
In the SPEI analysis, the potential evapotranspiration (PET) was calculated based on the FAO-56 Penman-Monteith equation (Allen et al. 1998). With a value for PET, the difference between monthly precipitation (P) and monthly PET for the month i is calculated as: D i = P i - PET i, providing a simple measure of the water surplus or deficit for the analyzed month. The calculated D i values are aggregated at different time scales following:
where k is the time scales, and n is the time unit.
As suggested by Vicente-Serrano et al. (2010), these three-parameter log-logistic distribution has been selected to model the D series.
Then, with the cumulative distribution function F(x) of the log-logistic distribution, SPEI was obtained as the standardized value of F(x), and details of the calculation can be referred to Vicente-Serrano et al. (2010). The average value of the SPEI is 0, and the standard deviation is 1. SPEI is a standardized variable and can thus be compared with other SPEI values over space and time. An SPEI of 0 indicates a value corresponding to 50 % of the cumulative probability of D based on the log-logistic distribution. Comparing performances of SPEI, PDSI and SPI, Vicente-Serrano et al. (2010) found good relations between SPEI and PDSI at time scales of 10–18 months. In this study, a time scale of 12 months was used for SPEI-based drought/wetness.
3.2 Drought Attributes
The drought characteristics were identified by the theory of runs (Yevjevich,1967) (Fig. 2). The drought duration is the period when SPEI is continuously below the truncation level and drought severity is the cumulative deficit below the truncation level for the duration of the drought event. Then, three important properties, drought duration, drought severity and drought intensity, were analyzed for each drought event. As the wet and dry conditions are divided by the value of 0 for SPEI, the truncation level was set to be 0 in this study.
3.3 Fuzzy C- Means (FCM) Clustering
FCM was proposed by Ruspini (1969) and was further improved by Dunn (1974). Furthermore, Bezdek (1980) established the convergence of a class of clustering procedures, also known as the fuzzy ISODATA algorithm. Let X = {x 1, x 2, …, x n} ⊂ ℜ s be a finite data set in feature space ℜ s, the FCM algorithm partitions the matrix X into c clusters by minimizing the objective function (Rao and Srinivas 2006):
where U is the membership of each feature vector in each fuzzy cluster, V = (v 1, v 2, …, v c ), v i ∈ R n is the cluster center, D 2 ikA = (x k − v i )T A(x k − v i ) is the distance from kth feature vector x k to the centroid of ith cluster v i, and the parameter m∈[1, ∞] refers to the weight exponent for each fuzzy membership. Besides, u ik denotes the membership degree that the ith group data belongs to the kth cluster. u ik should satisfy the following equations:
In this study, c and m in Eq. (2) were obtained by extended Xie-Beni Index (V XB,m) (Xie and Beni 1991):
The validity of V XB,m has been verified by Rao and Srinivas (2006). The smaller V XB,m implies better regionalization. Besides, the regionalization was done based on membership matrix by the FCM algorithm using a certain threshold value. The selection of the threshold value was based on Srinivas et al. (2008), i.e.,
3.4 Multivariate L-moments
Multivariate L-moments were developed by Serfling and Xiao (2007). Let X(j) be a random variable with distribution F j , for j = 1, 2. By analogy with a covariance representation of L-moments of order k ≥ 1, multivariate L-moments are matrices Λ k with L-comoment elements defined by:
where P k * is the so-called shifted Legendre polynomial. Note that elements λ k[ij] and λ k[ji] are not necessarily equal. Particularly, the first L-comoment elements are:
which are, respectively, the L-covariance, L-coskewness, and L-cokurtosis. The L-comoment coefficients are given by:
where λ (j) k = λ k[jj] is the classical kth L-moment of variable X(j), j = 1, 2, as defined by Hosking (1990). A hierarchy of intuitively appealing analogues of the classical covariance and central comoments was thus provided by L-comoments. Their interpretation and comparison are facilitated by their definition in terms of the classical covariance operator. The matrix of the L-comoment coefficients is written as (Chebana and Ouarda 2007):
Particularly, for k = 2 the L-covariance matrix is given by:
According to Chebana and Ouarda (2007), the L-comoments are similar in structure to the univariate L-moments and capture their attractive properties. The multivariate L-moments defined previously are based on a theoretical population distribution; however their finite sample versions are useful to define statistical tests and also to estimate multivariate distribution parameters, as presented by Serfling and Xiao (2007). Computation was conducted based on the R software package ‘lmomco’ by Asquith (2011), who proposed an implementation of these finite sample L-comoments.
3.5 Copula Functions
Copulas model the dependence structure between random variables (Nelsen 2006). More particularly, the copula method is being used in describing the statistical behavior of hydro-meteorological extremes (e.g., Zhang and Singh 2007; Zhang et al. 2012 and 2013). Sklar (1959) advocated that the most general marginal-free description of the dependence structure of multivariate distributions is through its copula. Let F and G denote the marginal distribution functions of random variables x and y, and let H be a joint distribution function with F and G. Then, there exists a copula C such that for all real x and y
There are many copula families and Archimedean and extreme value copulas represent classes of particular interest in hydrology. A bivariate Archimedean copula is characterized by a generator ϕ(⋅), which is a convex decreasing function satisfying ϕ(1) = 0, where:
An extreme value copula is defined as
where A is a convex function defined on [0, 1] with max(t,1-t) ≤ A(t) ≤ 1. A simple and popular copula is the Gumbel-Hougaard copula:
where m ≥ 1, 0 ≤ x, y ≤ 1. The Gumbel-Hougaard copula is the only one that can simultaneously satisfy the conditions of the extreme-value copula and the Archimedean copulas.
The correlations between two variables can be used to estimate the parameter, Ө, of single station-based copula function. Furthermore, the estimation of parameter, Ө, of copula functions for regional droughts within a subdivision depends on the regional mean Kendall correlation, τ R , between drought duration and drought severity. Based on the regional mean L-moment ratio proposed by Hosking (1990) and Hosking and Wallis (1997), the regional mean Kendall correlation, τ R , between drought duration and drought severity for a certain region can be defined as the weighted mean Kendall correlation coefficient for each station with the length of drought series as the weights, i.e.,
where N c is the total number of the stations within a certain sub-region c; n i is the total number of the drought events at the station i; and τ i is the Kendall correlation between drought duration and drought severity at station i.
3.6 Index-Drought Method
The assumption behind the index-drought method is that the drought series within the subdivisions follow the identical frequency distribution with the same parameters except for the scale parameter (Dalrymple 1960; Hosking and Wallis 1997). Assume X is a random variable, F is a kind of distribution and percentile function, x(F), the percentile function of a drought series at station i being located in a subdivision can be written as
where i = 1, 2, …, N; μ i is the scale parameter of station i and can be denoted as the mean of a random variable; q(F) is the regional frequency curve, being recognized as dimensionless variable. It should be noted here that more than one variable is necessary to describe the changing properties of drought regimes, and then the above-mentioned univariate analysis should be extended to multivariate conditions. Assume that X and Y are random variables that follow the marginal distribution, FX and FY and related percentile functions x(F X ) and y(F Y ), and the copula functions are used to describe the joint distribution, F XY , of random variables X and Y, then the percentile functions of station i can be written as:
where C is the link function of Copula, q X and q Y are the marginal regional frequency curves, respectively, for random variables X and Y.
4 Results and Discussions
4.1 Regionalization Analysis
Figure 3a illustrates the computation procedure of regionalization, including: (1) initial clustering based on FCM; (2) modification of initial clustering based on multivariate non-homogeneity test; (3) multivariate homogeneity test to verify whether the classified subdivisions are climatically homogeneous; and (4) final clustering.
The exact steps for initial clustering are shown in Fig. 3b. Considering the independence of homogeneity test, Hosking and Wallis (1997) suggested that the variables for regional frequency analysis can be taken as input variables for clustering analysis. In this study, the meteorological variables that are related to drought events such as drought duration and drought severity were taken as input variables in the FCM analysis. Besides, the long term monthly mean precipitation, potential evapotranspiration and temperature were also selected as the input variables and the dimensions are 3 × 12 = 36.
In this study, the principle component analysis was used to reduce the dimension due to the correlations amongst meteorological variables (Satyanarayana and Srinivas 2011) and results are shown in Table 1. The principle components with the accumulative variance of larger than 85–95 % were taken as the major meteorological features. The leading n PC principle components, n PC = (3, 4, 5, 6), and also longitude, latitude and altitude were grouped into four scenarios. For each scenario, the number of clustering, c, and weight index, m, should be decided. Let c = 2, 3, …, 20, and m = 1.1, 1.2, …, 2.5. The FCM was done on the 19 × 25 = 475 conditions and V XB,m was used to evaluate the regionalization results. Then the optimal number of subdivisions, c, was 9, 5, 5, 5, and the optimal weight index, m, was 2.4, 2.5, 2.5, 2.5 for each scenario respectively. Furthermore, the 2-dimension homogeneity test results (H 2) was used to decide the optimal value of n PC , results (not shown in the paper) indicated that the optimal value of n PC is 4, and the clustering is shown in Fig. 4a.
4.2 Homogeneity Test
The initial clustering needs to be tested for homogeneity (Fig. 5a). It can be seen from Fig. 5a that each clustering involves some stations that are not exactly categorized into that subdivision, i.e., D i ≥ 3. The number of stations with 5 ≥ D i ≥ 3 in the clusters 1–5 is 21, 10, 21, 17 and 15, respectively, and that of stations with D i > 5 is 4, 3, 7, 8, 6, respectively. In this sense, about 20 % of the stations for each cluster are not homogeneous. To decide the non-homogeneity of stations for each cluster, a novel processing method is proposed in this study, as shown in Fig. 1c. In the FCM-based analysis, the meteorological conditions are fully considered. Therefore, membership degrees were taken into account in the processing of non-homogeneity of the stations for each cluster (Fig. 5b), which is in line with the processing method of Rao and Srinivas (2006) and Sadri and Burn (2011). The adjustment of stations for homogeneity based on the method is shown in Fig. 1c, and there are no non-homogenous stations and the spatial patterns of stations can be found in Fig. 4b.
Test results of non-homogeneity. a shows the initial results of non-homogeneity; b denotes the modified non-homogeneity test results. The numbers marked in the pies show the number of stations corresponding to D i of each regionalization. The bold blue numbers denote the range that cannot pass the test
4.3 Consistency Test
Results of the consistency test for the adjusted clustering are shown in Table 2. The H 2 values for each cluster are all negative. In this sense, the adjusted clusters are statistically consistent. Besides, the univariate consistency test is done for the drought duration and drought severity in each cluster. Results also indicate the consistency of each cluster in terms of marginal distributions. Therefore, the consistent drought-based clustering is attained and this paves the way for the bivariate regional drought frequency analysis as introduced in what follows.
4.4 Final Regionalization
For clarification of regionalization, stations for each cluster were outlined and the final regionalization is shown in Fig. 4c that shows that some regions can be classified into two neighboring subdivisions simultaneously, which implies ambiguous boundary of drought regimes. And this points out the difficulty in research on drought hazards. Besides, superimposed regions also suggest uncertainty in regionalization analysis. However, practical planning for mitigation of droughts underlines the exact estimation of quartiles for the superimposed regions between two subdivisions. Rao and Srinivas (2006) suggested that the quartiles of the superimposed regions between two or more subdivisions should be estimated by the weighted mean of the quartiles of these subdivisions, and the weight is membership degree of these subdivisions.
4.5 Regional Frequency Analysis
Hosking and Wallis (1997) and Martins and Stedinger (2002) underlined importance of regional frequency analysis for extreme hydrological regimes. The workflow of regional frequency analysis is outlined in Fig. 3. The bootstrap resampling technique was used to quantify the uncertainty of joint probability analysis results. It should be noted here that stationarity and independence are the two assumptions to be considered in regional frequency analysis. The Mann-Kendall trend test (Mann 1945; Kendall 1995) and persistence test were used to test stationarity and independence (Yang et al. 2010), and no significant trends or persistence were identified at the 5 % significance level (Results are not shown here). These results justified the validity of the regional frequency analysis.
The first step to analyze regional frequency was to decide the marginal distribution function for each sub-region. The procedure for bivariate regional frequency analysis is illustrated in Fig. 1d and major computations include deduction of marginal distribution and joint distribution. For the robustness of analysis, parameters of the marginal distribution were analyzed using the sample L-moment technique (Hosking and Wallis 1997). In this case, the marginal distribution functions are from the following candidate functions, such as General Extreme Value (GEV), General Logistic Distribution (GLO), General Pareto Distribution (GPA), General Normal Distribution (GNO), Log-Normal Distribution (LN3), Pearson-III Distribution (PE3) and Weibull Distribution (WEI) and two-parameter distribution such as Exponential Distribution (EXP) and Gamma Distribution (GAM). For the goodness-of-fit test for 3-parameter distribution, Hosking and Wallis (1997) recommended the Z test whose results are shown in Table 2. Table 2 indicates that PE3 only passed the test for drought duration in sub-region 1. Besides, researchers (Zelenhastic and Salvai 1987; Mathier et al. 1992; Shiau et al. 2007) indicated that the marginal distribution should be exponential distribution if the drought duration is the continuous random variable, and should be the gamma distribution if the drought severity is the continuous random variable. Due to the unique statistical properties of drought events, the goodness-of-fit test was done specifically for the exponential and gamma distributions.
It should be noted here that the Z test was not recommended for the goodness-of-fit test for 2-parameter distributions, and the goodness-of-fit test was used only for station-based frequency analysis and regional frequency analysis. In this case, the K-S test was used for goodness-of-fit test and results are shown in Table 2. It can be identified from Table 2 that the exponential and gamma distributions passed the K-S test at the 95 % confidence level for drought duration and drought severity series. Thus, the exponential and gamma Distributions were accepted as marginal distribution functions for regional frequency analysis of drought duration and severity, and parameters for each sub-division are listed in Table 3. For joint regional frequency curves, two problems have to be addressed, i.e., the selection of copula functions and the estimation of copula parameters. The estimation of copula parameters can be based on Eq. (19). The selection of the copula functions was based on the Cramer-von Mises functional Sn defined in Eq. (2) of Genest and Rémillard (2009). It can be observed from Table 2 that the goodness-of-fit of the Clayton copula functions was not acceptable for drought series of any sub-division. However, the goodness-of-fit of the Gumbel and Frank copulas was acceptable with p values ranging between 0.20 and 0.28 for the Gumbel copula and 0.27–0.33 for the Frank copula. A graphical selection of the copula function is illustrated in Fig. 6. It can be seen from Fig. 6a1-a5 that the differences between color of data points and that of the theoretical copula curves are not apparent, showing an acceptable goodness-of-fit performance of the Gumbel copula for describing the drought probability behavior within sub-divisions. Distinctly different color grades between data points and theoretical copula curves, as shown in Figs. 6b1-b5, c1 and 7c5, indicate unacceptable goodness-of-fit performances of the Frank and Calyton copulas. In this case, the Gumbel copula was the selected copula function for description of the joint probability behavior of drought regimes within each sub-division, and the parameters of the Gumbel copula are displayed in Table 3. Based on the above-mentioned analysis, the joint probability curves of drought duration versus drought severity for each sub-division are illustrated in Fig. 7. Based on observed meteorological data during the period of 1960–2005, the mean drought duration, mean drought severity and mean waiting time between drought regimes are also analyzed in terms of spatial patterns (Fig. 8).
Fitting performances of copula functions. FD and FS denote cumulative distribution function of the drought duration and severity, respectively. Solid curves denote the theoretical curves of copula functions; scatters in the figure denote observed data; a denotes the Gumbel copula; b the Frank copula and c the Clayton copula. The numbers along with a, b and c denote the corresponding cluster, e.g., a1 denotes the Gumbel copula fitting for cluster 1
It can be observed from Fig. 8 that drought regimes in northwest China are dominated by longer drought duration, larger drought severity, and the waiting time between drought events is relatively longer, about 2 years. However, southeast China is dominated by shorter drought duration, smaller drought severity and shorter waiting time between drought events. Therefore, northwest China is characterized by heavy drought events with lower occurrence frequency and the reverse is in southeast China. However, the occurrence properties of droughts in northeast China are similar to those in northwest China. The drought duration, drought severity and waiting time between drought events in north China are moderate when compared to those in northwest and southeast China, showing transitional properties. The importance of this study lies in the fact that if the mean values of drought duration and drought severity (Fig. 8a and b) are known in advance, the joint probability of a drought event can be obtained based on regional joint probability curves, as shown in Fig. 8 for each sub-division. This result provides important information for planning and management of water resources and agricultural activities within each sub-division and even some places or regions of a certain subdivision or cluster that are without data.
4.6 Confidence Intervals for Regional Joint Probability Curves
The above estimation of regional joint probability curves is the point estimation. However, evaluation of uncertainty is critical for the validity of results. In this study, the bootstrap resampling technique (Burn 2003) was used to estimate the confidence intervals of the joint probability curves. In the bootstrap resampling analysis, the resample was done for N re = 999 times and then 999 samples were obtained. The regional frequency analysis was done following the procedure shown in Fig. 3d. Thus, 999 regional joint probability curves were obtained, and then the 2.5 % and the 97.5 % percentiles of these 999 regional joint probability curves were extracted and taken as the upper and lower limits. The upper and the lower limits are shown as black and gray dashed lines, respectively, in Fig. 7. It can be seen from the figure that the confidence interval is widening when the joint cumulative probability is increasing, implying increasing uncertainty for increased return periods of drought events. This kind of uncertainty in the estimation of return periods of drought events will influence planning and management of drought-impacted water resources and agricultural activities (e.g., Hailegeorgis et al. 2013). Fortunately, Fig. 7 indicates that the uncertainty in the estimation of joint probability is not evident. The black and gray dashed lines almost overlay each other when the joint cumulative probability ranges between 0.5 and 0.95. The confidence interval is larger when the joint cumulative probability is larger than 0.98. In this sense, the estimation of regional joint probability is not sensitive to spatial resampling. The estimation of return periods for extreme drought events is robust and stable with acceptable uncertainty, which also implies an advantage of regional frequency analysis in the estimation of return periods of weather extremes.
5 Conclusions
In this study, regionalization is done with respect to droughts using FCM algorithm and multivariate L-moment technique. Copula and Bootstrap resampling technique are used to estimate the regional joint probability of drought duration and severity and further to evaluate the uncertainty of joint probability curves. Important conclusions drawn from this study are as follows:
-
(1)
Five homogenous regions are demarcated, based on statistical properties of droughts across China. However, regionalization of this study considers the changing properties of droughts, i.e., the boundaries of drought-impacted regions are usually blurred and ambiguous. The FCM-based regionalization produces overlaid parts between two or three sub-divisions, showing uncertainty of regionalization and also ambiguous boundaries of drought-impacted regions. Therefore, regionalization of this study is relatively objective.
-
(2)
Droughts in northwest China are characterized by longer duration and larger severity. However, the occurrence of droughts in northwest China is subject to lower frequency or longer waiting time between drought events. Droughts in southeast China, however, are dominated by shorter duration and smaller severity. The occurrence of droughts in southeast China is subject to higher frequency or shorter waiting time between drought events. Droughts in north China are moderate in terms of duration and severity and also waiting time between drought events when compared to those in northwest and southeast China. This result is significant for management and planning of water resources and agricultural activities in different regions of China.
-
(3)
With the help of bivariate copula functions, regional joint frequency curves are obtained for drought duration and severity. Parameters of frequency curves for each cluster are also obtained. Mean drought duration, mean drought severity and mean waiting time between drought events are also obtained. The joint probabilities of droughts can be extracted from regional probability curves and results of mean drought duration, mean severity and mean waiting time between drought events. Furthermore, droughts in the regions without meteorological data can also be estimated in terms of joint probability using the index-drought method proposed in this study. In this way, this study provides a theoretical basis for evaluation of droughts even in regions having no meteorological data. Besides, uncertainty in the estimation of joint probability curves for each cluster is also assessed using the bootstrap resampling technique, showing the validity and reliability of regional frequency analysis results.
-
(4)
This study provides a basis for development and enhancement of mitigation measures for drought hazards in China. It also provides a theoretical reference for similar studies in other regions of the world, and is important for planning and management of water resources and agricultural activities in the backdrop of intensifying weather extremes under the influence of climate warming.
References
Allen RG, Pereira LS, Raes D, Smith M (1998) Crop evapotranspiration: guidelines for computing crop requirements, irrigation and drainage paper 56. FAO, Roma
Asquith WH (2011) lmomco-L-moments, trimmed L-moments, L-comoments, censored L-moments, and many distributions. R package version 1.3.6, http://www.cran.r-project.org/package=lmomco, Texas Tech University, Lubbock, Texas
Bazrafshan J, Hejabi S, Rahimi J (2014) Drought monitoring using the multivariate standardized precipitation index (MSPI). Water Resour Manag 28(4):1045–1060
Bezdek JC (1980) A convergence theorem for the fuzzy ISODATA clustering algorithm. IEEE Trans Pattern Anal Mach Intell 2(1):1–8
Burn DH (2003) The use of resampling for estimating confidence intervals for single site and pooled frequency analysis. Hydrol Sci J 48(1):25–38
Chebana F, Ouarda T (2007) Multivariate L-moment homogeneity test. Water Resour Res 43(8), W08406
Dalrymple T (1960) Flood frequency analysis, US Geol. Surv Water Supply Paper 1543A:11–51
Dunn JC (1974) A fuzzy relative of the ISODATA process and its use in detecting compact, well-separated clusters. J Cybern 3(3):32–57
Ganguli P, Reddy JM (2014) Evaluation of trends and multivariate frequency analysis of droughts in three meteorological subdivisions of western India. Int J Climatol 34:911–928
Genest C, Rémillard BD (2009) Goodness-of-fit tests for copulas: a review and a power study. Insur: Math Econ 44:199–213
Hailegeorgis TT, Thorolfsson ST, Alfredsen K (2013) Regional frequency analysis of extreme precipitation with consideration of uncertainties to update IDF curves for the city of Trondheim. J Hydrol 498:305–318
Hao Z, AghaKouchak A (2013) Multivariate standardized drought index: a parametric multi-index model. Adv Water Resour 57:12–18
Heim Richard R (2002) A review of twentieth-century drought indices used in the United States. Bull Am Meteorol Soc 83:1149–1165
Hosking JRM (1990) L-moments: analysis and estimation of distributions using linear combinations of order statistics. J R Stat Soc 52(1):105–124
Hosking JRM, Wallis JR (1997) Regional frequency analysis: an approach based on L-moments. Cambridge University Press, Cambridge
Kao S-C, Govindaraju RS (2010) A copula-based joint deficit index for droughts. J Hydrol 380:121–134
Kendall MG (1995) Rank correlation methods. Griffin, London
Mann HB (1945) Nonparametric tests against trend. Econometrica 13(3):245–259
Martins ES, Stedinger JR (2002) Cross correlations among estimators of shape. Water Resour Res 38(11):1252. doi:10.1029/2002WR001589
Mathier L, Perreault L, Bobe B, Ashkar F (1992) The use of geometric and gamma-related distributions for frequency analysis of water deficit. Stoch Hydrol Hydraul 6(4):239–254
McEvoy DJ, Huntington JL, Abatzoglou JT, Edwards LM (2012) An evaluation of multiscalar drought indices in Nevada and Eastern California. Earth Interact 16(18):1–18
Mishra KA, Singh PV (2010) A review of drought concepts. J Hydrol 391:202–216
Nelsen RB (2006) An introduction to copulas. Springer, Verlag
Palmer WC (1965) Meteorological drought. U.S. Department of Commerce Weather Bureau Research Paper, 45, 58 pp
Potop V, Boroneanţ C, Možný M, Štĕpánek P, Skalák P (2014) Observed spatiotemporal characteristics of drought on various time scales over the Czech Republic. Theor Appl Climatol 115:563–581
Rao AR, Srinivasb VV (2006) Regionalization of watersheds by fuzzy cluster analysis. J Hydrol 318:57–79
Rossi G (2009) European Union policy for improving drought preparedness and mitigation. Water Int 34(4):441–450
Rossi G, Cancelliere A (2013) Managing drought risk in water supply systems in Europe: a review. Int J Water Resour Dev 29(2):272–289
Ruspini EH (1969) A new approach to clustering. Inf Control 15(1):22–32
Sadri S, Burn DH (2011) A Fuzzy C-Means approach for regionalization using a bivariate homogeneity and discordancy approach. J Hydrol 401:231–239
Satyanarayana P, Srinivas VV (2011) Regionalization of precipitation in data sparse areas using large scale atmospheric variables - A fuzzy clustering approach. J Hydrol 405:462–473
Serfling R, Xiao P (2007) A contribution to multivariate L-moments: L-comoment matrices. J Multivar Anal 98(9):1765–1781
Shiau JT, Feng S, Nadarajah S (2007) Assessment of hydrological droughts for the Yellow River, China, using copulas. Hydrol Process 21:2157–2163
Sklar A (1959) Fonctions de répartition àn dimensions et leurs marges. Publ Inst Stat Univ Paris 8:229–231
Spinoni J, Antofie T, Barbosa P, Bihari Z, Lakatos M, Szalai S, Szentimrey T, Vogt J (2013) An overview of drought events in the Carpathian Region in 1961–2010. Adv Sci Res 10:21–32
Srinivas VV, Tripathi S, Rao AR, Govindaraju RS (2008) Regional flood frequency analysis by combined self-organizing feature map and fuzzy clustering. J Hydrol 348:148–166
Tsakiris G, Pangalou D, Vangelis H (2007) Regional drought assessment based on the Reconnaissance Drought Index (RDI). Water Resour Manag 21(5):821–833
Tsakiris G, Nalbantis I, Vangelis H, Verbeiren B, Huysmans M, Tychon B, Jacquemin I, Canters F, Vanderhaegen S, Engelen G, Poelmans L, de Becker P, Batelaan O (2013) A system-based paradigm of drought analysis for operational management. Water Resour Manag 27(15):5281–5297
Vicente-Serrano SM, Beguería S, López-Moreno JI (2010) A multiscalar drought index sensitive to global warming: the standardized precipitation evapotranspiration index. J Clim 23:1696–1718
Vicente-Serrano SM, Beguería S, Lorenzo-Lacruz J, Camarero JJ, López-Moreno JI, Azorin-Molina C, Revuelto J, Morań-Tejeda E, Sanchez-Lorenzo A (2012a) Performance of drought indices for ecological, agricultural, and hydrological applications. Earth Interact 16(10):1–27
Vicente-Serrano SM, López-Moreno JI, Beguería S, Lorenzo-Lacruz J, Azorin-Molina C, Morán-Tejeda E (2012b) Accurate computation of a streamflow drought index. J Hydrol Eng 17:318–332
Vicente-Serrano SM, JI Lopez-Moreno, S Beguería, J Lorenzo-Lacruz, A Sanchez-Lorenzo, JM García-Ruiz, C Azorin-Molina, E Morán-Tejeda, J Revuelto, R Trigo, F Coelho and F Espejo (2014) Evidence of increasing drought severity caused by temperature rise in southern Europe. Environ. Res. Lett. 9:044001(9pp), doi:10.1088/1748-9326/9/4/044001
Wilhite DA (1993) Drought assessment, management and planning: theory and case studies. Kluwer, 293 pp
Wilhite DA and M Buchanan-Smith (2005) Drought as hazard: Understanding the natural and social context. Drought and Water Crises: Science, Technology, and Management Issues, D. A. Wilhite, Ed., CRC Press, 3–29
Xie XL, Beni G (1991) A validity measure for fuzzy clustering. IEEE Trans Pattern Anal Mach Intell 13(8):841–847
Yang T, Shao Q, Hao Z-C, Chen X, Zhang Z, Xu C-Y, Sun L (2010) Regional frequency analysis and spatio-temporal pattern characterization of rainfall extremes in the Pearl River Basin, China. J Hydrol 380:386–405
Yusof F, Hui-Mean F, Suhaila J, Yusof Z (2013) Characterisation of drought properties with bivariate copula analysis. Water Resour Manag 27(12):4183–4207
Zelenhastic E, Salvai A (1987) A method of streamflow drought analysis. Water Resour Res 23(1):156–168
Zhang L, Singh VP (2007) Bivariate rainfall frequency distributions using Archimedean copulas. J Hydrol 332:93–109
Zhang Q, Singh VP, Li J, Chen X (2011) Analysis of the periods of maximum consecutive wet days in China. J Geophys Res 116, D23106. doi:10.1029/2011JD016088
Zhang Q, VP Singh, M Xiao, J Li (2012) Regionalization and spatial changing properties of droughts across the Pearl River basin, China. Journal of Hydrology 472–473:355–366
Zhang Q, Xiao M, Singh VP, Chen X (2013) Copula-based risk evaluation of droughts across the Pearl River basin, China. Theor Appl Climatol 111(1):119–131
Zhang Q, Sun P, Li J, Singh VP, Liu J (2014) Spatiotemporal properties of droughts and related impacts on agriculture in Xinjiang, China. Int J Climatol. doi:10.1002/joc.4052
Acknowledgments
This work is financially supported by the National Science Foundation for Distinguished Young Scholars of China (Grant No.: 51425903), the Xinjiang Science and Technology Planning Project (Grant No.: 201331104), the Leading Expert Project by Anhui Province and fully supported by a grant from the Research Grants Council of the Hong Kong Special Administrative Region, China (Project No. CUHK441313). Our cordial gratitude should be extended to the editor-in-chief, Prof. Dr. George P. Tsakiris, and three anonymous reviewers for their careful and insightful review and also for their pertinent and relevant comments and suggestions which are greatly helpful for further improvement of the quality of this manuscript.
Author information
Authors and Affiliations
Corresponding author
Rights and permissions
About this article
Cite this article
Zhang, Q., Qi, T., Singh, V.P. et al. Regional Frequency Analysis of Droughts in China: A Multivariate Perspective. Water Resour Manage 29, 1767–1787 (2015). https://doi.org/10.1007/s11269-014-0910-x
Received:
Accepted:
Published:
Issue Date:
DOI: https://doi.org/10.1007/s11269-014-0910-x