Abstract
Joint analysis of genetic and neuroimaging data, known as Imaging Genetics (IG), offers an opportunity to deepen our knowledge of the biological mechanisms of neurodevelopmental domains. There has been exponential growth in the literature on IG studies, which challenges the standardization of analysis methods in this field. In this review we give a complete up-to-date account of IG studies on attention deficit hyperactivity disorder (ADHD) and related neurodevelopmental domains, which serves as a reference catalog for researchers working on this neurological disorder. We searched MEDLINE/Pubmed and identified 37 articles on IG of ADHD that met our eligibility criteria. We carefully cataloged these articles according to imaging technique, genes and brain region, and summarized the main results and characteristics of each study. We found that IG studies on ADHD generally focus on dopaminergic genes and the structure of basal ganglia using structural Magnetic Resonance Imaging (MRI). We found little research involving multiple genetic factors and brain regions because of the scarce use of multivariate strategies in data analysis. IG of ADHD and related neurodevelopmental domains is still in its early stages, and a lack of replicated findings is one of the most pressing challenges in the field.
Similar content being viewed by others
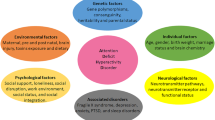
Avoid common mistakes on your manuscript.
Introduction
The joint analysis of genomic and neuroimaging data, known as Imaging Genetics (IG), offers an opportunity to deepen our knowledge of the biological mechanisms of behavioral and neurodevelopmental domains. Neuroimaging assessment is used to understand neurodevelopment, cognition and behavior at the brain level. Considering genomic information simultaneously, we can make substantial gains in the biological interpretation and understanding of these processes.
IG studies aim to discover the relationships between specific genetic variants and brain structure and function (Glahn et al. 2007). IG studies initially attempted to evaluate the contribution of specific single candidate genes (Risacher et al. 2010), and more recently have begun to evaluate the entire genome to discovering genetic polymorphisms that influence brain structure (Bis et al. 2012; Hibar et al. 2015), brain function (Papassotiropoulos et al. 2013; Potkin et al. 2009), and related neurodevelopmental disorders (Franke et al. 2016; Stein et al. 2010). IG studies have made notable progress in explaining neurological conditions such as Alzheimer’s disease (Weiner et al. 2015) and schizophrenia (Meyer-Lindenberg 2010), but there is still a dearth of evidence on Attention-Deficit/Hyperactivity Disorder (ADHD).
ADHD is behaviorally characterized by hyperactivity, impulsivity and inattention (Olfson 1992), reaching a prevalence of 2–7% (van der Meer et al. 2015). Although ADHD has an important social burden, and has been widely studied in relation to environmental and social risk factors (Tarver et al. 2014), its underlying biological mechanisms remain unknown. For instance, structural or functional abnormalities of the brain may play an important role in the development of the disease, as observed in neuroimaging studies. ADHD appears to be linked to altered brain volume, changes in the activity of specific brain areas, and abnormal brain connectivity. A meta-analysis of structural magnetic resonance imaging in children (sMRI) (Valera et al. 2007) found that the brain regions with the largest volumetric reduction in ADHD individuals were the posterior inferior cerebellar vermis, the splenium of the corpus callosum, the right caudate, right putamen and the total and right cerebral volume. In addition, a meta-analysis of functional magnetic resonance imaging (fMRI) studies showed significant hyperactivation in the ventral attention, somatomotor and default mode networks (Cortese 2012). In their literature review of diffusion tensor imaging studies, van Ewijk et al. (2012) noted that ADHD patients exhibit widespread abnormalities in white matter integrity.
In terms of genetics, Genome-wide association studies (GWAS) have revealed genes that are involved in the dopaminergic, adrenergic, serotonergic and cholinergic pathways (Faraone and Mick 2010). So far, the main candidate genes for ADHD are the dopamine receptors (DRD2, DRD3, DRD4, DRD5), and transporters (SLC6A also known as DAT1), and dopamine beta-hydroxylase (DBH). However, while ADHD is estimated to have ~70% heritability (Cortese 2012; Franke et al. 2012) candidate genes account for only 1% of its phenotypic variance. The remaining unexplained heritability is a recurrent problem in complex traits, where the added contribution of single nucleotide variations cannot account for the total heritability observed in family studies (Maher 2008; Manolio et al. 2009). This gap may be accounted for by rare variants, other types of common mutations (deletion, translocations, inversions), or by epistasis. In addition, higher power to detect significant associations may be obtained by analyzing intermediate phenotypes. In the case of IG studies, the intermediate phenotypes are derived from neuroimaging data, based on the premise that there is greater correlations between brain structure/function and genetic variability (heritability) because brain physiology is etiologically closer to molecular biology than behavior (Zayed and Robinson 2012). In the case of ADHD, these may be even greater potential to advance, since the heritability gap is much wider than for other neurological disorders.
Since the work of Durston (2010), there has not been a thorough systematic review of the use of IG in ADHD. Given the increasing interest of ADHD, we aimed to update the main findings of IG studies on ADHD and to pinpoint the priorities in current IG research on ADHD. We review the evidence on the key genetic, the relevant brain phenotypes, and the neuroimaging technologies that have been used to explore this relationship, specifically Structural MRI, Functional MRI, Single-photon emission CT, Diffusion Tensor Imaging, and EEG.
Materials and methods
Literature search
We searched articles indexed in PubMed (National Library of Medicine) under the terms: “Imaging Genetics”, “Neuroimaging and Genetics”, “Neuroimage and Genetics” combined with the following terms “Attention deficit hyperactivity disorder”, “ADHD”.
Selection criteria
Selected studies fulfilled the following criteria: a) included both genetic and neuroimaging data, b) directly focused on neurodevelopmental domains as the principal outcome of the study, or on brain intermediate phenotypes linked to neurodevelopmental domains, c) were written in English, d) were original research articles with accessible information. We excluded abstracts only, case reports and letters to the editor. Articles that appear duplicated at PUBMED were counted once.
To reduce the subjectivity of the classification criteria, studies were selected by two independent reviewers.
Manuscript classification
Selected articles were cataloged in tables corresponding to four imaging modalities: “Brain structure” (Supplementary Table 1), “Brain function” (Supplementary Table 2), “Brain chemistry” (Supplementary Table 3) and “Brain connectivity” (Supplementary Table 4). Each table contains information on: participants, neuroimaging modality and methods, main brain region, main genomic biomarkers, main results, and the significance of the main results.
Results
Literature search results
The selection process is summarized in Fig. 1. A total of 353 articles were identified in PUBMED, of which 306 were excluded by an initial title and abstract screen, and the remaining 47 were all assessed for eligibility. One study was duplicated, and 9 further studies were excluded because they did not provide information about both neuroimaging and genomic studies. As a result, 37 articles were chosen for evaluation of the full-text.
Genetic variants
We found that the most commonly analyzed genetic variants in IG studies of ADHD are located in candidate genes within the dopaminergic, serotoninergic and cholinergic systems, which may be altered in ADHD (Cortese 2012) [Fig. 2]. Dopaminergic genes include: the dopamine receptor D4 (DRD4), and the dopamine transporter gene (SLC6A3), which was also an early candidate gene of ADHD (Faraone et al. 2014; LaHoste et al. 1996). In the serotonergic pathway the most widely investigated gene is TPH2, which participates in the biosynthesis of serotonin. Other candidate genes have also been studied, such as monoamine oxidase A (MAOA), Kell blood group complex subunit-related family member 4 (XKR4), and α-2A-adrenergic receptor neurotrasmissor (ADRA2A) (Gizer et al. 2009; Sánchez-Mora et al. 2015; Tortajada-Genaro et al. 2016; Zhang et al. 2012).
Brain phenotypes
Most of the studies that correlate genetic and imaging data in ADHD focus on identifying genetic variants associated with neuroimaging endophenotypes in ADHD patients (Mulder et al. 2008). These studies assume that fronto-subcortical-cerebellar circuits are etiologically closer to genetic variations than ADHD symptoms. Some of these studies also focused on whole brain analyses. However, we did not find well established brain regions that, in combination with genomics, are linked to ADHD. We found that sMRI studies mainly focused on basal ganglia (BG), prefrontal cortex (PC) and cerebellum structures, while fMRI studies mainly focused on the striatum, and also on the PC and BS regions. Single photon emission computed tomography (SPECT) studies also focused on Dopamine transporter binding potential in the BG and striatum regions. Diffusion tensor imaging (DTI) studies focused on white matter structure and connectivity, and electroencephalography (EEG) studies focused on prefrontal brain regions [Fig. 2].
Neuroimaging technologies
Twenty five of the selected studies used MRI technologies, eleven sMRI, eleven fMRI and three DTI. Six studies used SPECT and two used EEG. Since the review by Durston (2010), there has been an increase in the number of publications based on MRI, with six new studies using sMRI, eight using fMRI and three using DTI. Two new studies on brain chemistry used SPECT [Supplemental Fig. 1].
Structural magnetic resonance imaging (sMRI)
Dopaminergic and serotonergic genes: SLC6A3, DRD4 and 5-HTTLPR
Three of the selected sMRI studies analyzed SLC6A3 polymorphisms (Durston et al. 2005; Onnink et al. 2016; Shook et al. 2011). Durston et al. (2005) observed smaller caudate nucleus volume in SLC6A3-10R carriers than in SLC6A3-10R carriers (p = 0.035). Shook et al. (2011) also found smaller caudate nucleus volumes in SLC6A3-10R homozygotes than in SLC6A3-9R carriers, restricting the sample to preadolescents in order to control for brain variations during the developmental stage (p = 0.01). Onnink et al. (2016) reported larger striatum volumes in carriers of the of the SLC6A3-9R/6R haplotype compared to non-carriers (p = 0.0055).
Five IG studies have reported a correlation between brain volume measurements and DRD4 variants (Bobb et al. 2005; Castellanos et al. 1998; Monuteaux et al. 2008; Mous et al. 2015; Shaw et al. 2007). Specifically, Shaw et al. (2007) reported thinner right orbitofrontal/inferior prefrontal and posterior parietal cortex in DRD4-7R carriers (p < e-20). Monuteaux et al. (2008) observed significantly smaller mean volumes in the superior frontal cortex (p = 0.021) and cerebellum cortex (p < 0.001). In contrast, Castellanos et al. 1998 and Bobb et al. 2005 did not find significant genotype effects (p > 0.05). Mous et al. 2015 performed gene-set analysis to investigate the role of dopamine and serotonin pathway genes in ADHD symptom severity and basal ganglia volume, but found no significant associations (p > 0.1).
Non dopaminergic and serotonergic genes: XKR4
While most sMRI studies have analyzed dopaminergic genes, other candidate genes involved in other disorders have also been studied. For instance, XKR4, previously associated with schizophrenia and ADHD (Lantieri et al. 2010; Neale et al. 2008). Although no main genetic effects were found, De Zeeuw et al., 2013 reported a significant interaction between birth weight and a genetic variant in XKR4, as a predictor of the grey matter structure of cerebellum (p = 0.017).
Genome-whole analysis
Two IG studies used genome-wide data to assess associations with ADHD symptoms and brain volume measurements using novel analytical strategies (Khadka et al. 2016; N Vilor-Tejedor et al. 2016). Vilor-Tejedor et al. 2016 proposed modelling ADHD symptoms using a zero-inflated negative binomial distribution, and found that a genetic variant in MAOA was associated with ADHD symptoms and bilateral (left and right) perivascular brain volumes (p = 3.52e-4; p = 0.037). Khadka et al. 2016 used a multivariate parallel independent component analysis (pICA) to identify several imaging genetic components between regional gray matter volume and genetic data, which in turn are related to biological pathways linked to structural abnormalities that are common in ADHD (p < e-5).
Functional magnetic resonance imaging (fMRI)
Dopaminergic genes: SLC6A3 and DRD4
Most IG studies of ADHD based on fMRI have studied SLC6A3 (Aarts et al. 2015; Bédard et al. 2010; Braet et al. 2011; Brown et al. 2010; Durston et al. 2008; Hoogman et al. 2013; Paloyelis et al. 2012; Szobot et al. 2005; van Rooij et al. 2015). Durston et al. (2008) reported reduced striatal activity in a sample of male adolescents with ADHD who were carriers of the SLC6A3-10R allele (p < 0.001). In a sample of adults with ADHD, Bédard et al. (2010) also found an association between the striatum volume and ADHD symptoms as a function of SLC6A3 polymorphisms (p < 5e-3). van Rooij et al. (2015) reported significant genotype group interaction for striatal activation, in which SLC6A3 genotypes were associated with MR signal changes in individuals at familial risk of ADHD (p < 0.005).
These last three studies used a Go/No-Go task (GNG), which uses a binary classification to evaluate sustained attention and response control; the participant passes the test only when the Go condition is met and the No-go condition fails. Aarts et al. (2015) also showed an association between SLC6A3 and the striatal signal under a switching task evaluating executive functioning and cognitive flexibility. Specifically, they observed greater striatal signal in SLC6A3-9R-carriers with ADHD than in healthy carriers (p = 0.023).
Brown et al. (2010) showed evidence of significant hypoactivation of the left anterior cingulate cortex (p = 0.036) and the right prefrontal cortex (p = 0.049) in homozygous SLC6A3-10R carriers from a sample of children with ADHD under a Multi-Source Interference task (MSIT). The MSIT is used to identify the cognitive/attention network in normal volunteers, and to test its integrity in people with neuropsychiatric disorders. Also, three of these studies analyzed the effects of SLC6A3 genotypes on brain activity. (Braet et al. (2011) found that SLC6A3-10R carriers had increased activation of frontal (p < 0.001) and parietal regions (p < 0.05) during a sustained attention to response-GNG task. Hoogman et al. (2013) suggested that SLC6A3-10R/6R dosage differentially modulated neural activation in the caudate nucleus during a Motivated Incentive Learning Task (MILT), although their results were not statistically significant (p = 0.24). The MILT task is used to evaluate reaction times in a reward and no-rewards condition; participants are asked to respond as quickly as possible to a target by pressing a button, conditioned to the fact that some targets provide a reward and others do not. In a third study, (Paloyelis et al. 2012) did not find any significant results (p = 0.17).
Two IG studies have reported a correlation between brain volume measurements and DRD4 variants (Gehricke et al., 2015; Mulligan et al., 2014). While Mulligan et al. (2014) did not find significant IG effects (p > 0.05), Gehricke et al. (2015) showed a significant increase in BOLD activity in the frontal lobe in DRD4-4R/7R and DRD4-4R/4R carriers under an emotional stimulus task (p = 0.0001).
Non dopaminergic genes: MAOA
In terms of non-dopaminergic genes, one study focused on how a genetic polymorphism at MAOA modulates the link between ADHD symptoms and striatal and inferior frontal activation patterns (Nymberg et al. 2013) under a Stop Signal Task (SST) and a Motivated Incentive Delay Task (MIDT). The SST is used to measure reaction times to primary stimulus by measuring the latency between the presentation of two distinct stimuli and the response. In the MIDT, a target stimulus is presented after an incentive cue to win or to avoid losing the indicated reward. The MIDT allows the operator to examine different stages of reward processing and different reward conditions. These studies found that ADHD symptoms were associated with increased BOLD response in the inferior frontal gyrus during SST (p = 0.014) and in the bilateral (left and right) ventral striatum during MIDT (p = 0.002; p = 0.015) in homozygote for the risk allele.
Single-photon emission computed tomography
Dopaminergic genes: SLC6A3 and DRD4
SPECT was also used to study ADHD in individuals stratified according to genotypes in dopaminergic genes. One study found significantly higher regional cerebral blood flow in medial frontal and left basal ganglia areas in children who were homozygous for the SLC6A3-10R allele (p < 0.05) (Rohde et al. 2003). A second study analyzed the SLC6A3-10R and DRD4-7R alleles (Szobot et al. 2005), and found significantly higher perfusion in the right middle temporal gyrus among individuals who carried both risk alleles (p < 0.05).
When applied to measure brain chemistry, SPECT has been used to evaluate ADHD response to medication as a function of polymorphisms in the dopaminergic genes (Cheon et al. 2005; Krause et al. 2009; Szobot et al. 2011). Methylphenidate (MPH) is a commonly prescribed psychostimulant for treating ADHD (Fone and Nutt 2005), and a variety of SPECT studies have analyzed its effects on the brain. Cheon et al. (2005) evaluated eleven healthy children with ADHD who were undergoing 8 weeks of MHP treatment, and found a poor response to MPH among SLC6A3-10R homozygotes (p = 0.008). Krause et al. (2009) analyzed the homozygosity of the SLC6A3-10R allele in adults with ADHD who had been administered 740 MBq [99 m Tc] of TRODAT-1, but found no significant associations (p > 0.1).
Finally, in a more recent study, Szobot et al. (2011) evaluated the combination of the DRD4-7R allele and homozygosity for the SLC6A3-10R allele (10/10) in a sample of 17 adolescents treated with MPH, and found a significant reduction in dopamine transporter blocker occupancy in the right and left caudate and putamen (p < 0.02).
Non dopaminergic genes: ADRA2A
A recent study evaluated how brain perfusion was related to ADHD symptoms as a function of genotypes at the Mspl locus of the ADRA2A gene (Kim et al. 2010), and found that subjects with ADHD and who carried C-allele had lower perfusion in the bilateral orbitofrontal regions than non-carriers (p < 0.0005).
Diffusion tensor imaging
Dopaminergic genes
We did not find any studies on dopaminergic genes that used this neuroimaging modality.
Non dopaminergic genes: ADRA2A and COMT
One study examined the association between the MspI (N = 25: GG, N = 28: CG + CC) and DraI polymorphisms of the ADRA2A gene and white-matter connectivity during a Continuous Performance Test (CPT, which measures sustained and selective attention) following MPH treatment in ADHD children (Park et al. 2013). The results suggest that ADRA2A genotypes have a role in abnormal white-matter integrity and in improving MPH-induced attentional performance. Specifically, carriers of the DraI T-allele showed fewer changes in mean commission error scores following 8 weeks of medication, and reduced diffusion in the right middle frontal cortex than subjects without the T-allele (p = 0.009). Also, carriers of the MspI C-allele showed decreased connectivity (Fractional Anisotropy) in the right postcentral gyrus (p < 0.0005).
A second study investigated the association between white-matter connectivity and the COMT Val158Met polymorphism in ADHD children (Hong et al. 2015). Attention performance was also assessed using CPT before and after an 8-week open-label trial of MPH. This study provided evidence of altered white-matter connectivity in COMT Val-homozygotes (p = 0.049). Another recent study (Kabukcu Basay et al. 2016) also evaluated the association between COMT Val158Met and white matter structure, and found significant gene-environment interactions in the cingulated gyrus, in which ADHD individuals had significantly lower connectivity (p < 0.005). Also, WM tracts had significantly different DTI measurements between COMT genotypes (p < 0.01).
Electroencephalography
The conduct of studies using EEG technology appears to have stagnated, in that we did not identify any new EEG studies since the last review by Durston (2010).
Dopaminergic genes
In an earlier study, Loo et al. (2003) showed that the SLC6A3 polymorphism was associated with medication-induced changes in cortical activity. SLC6A3-10R carriers presented EEG changes, namely increased central and parietal β power (p = 0.015; p = 0.045), decreased right frontal θ power (p = 0.034), and lower θ/β ratios (p = 0.021).
Non dopaminergic genes: TPH2
One study reported associations with two candidate polymorphisms in TPH2 (Baehne et al. 2009). This study found that the previously reported risk alleles for ADHD in both polymorphisms were associated with reduced GNG measurements of prefrontal brain function (p = 0.002).
Discussion
Following the initial study by Hariri & Weinberger, IG studies have become increasingly popular. However, the number of IG studies on ADHD is still limited, and the common biological patterns remain unclear and incompletely reported. In this work, we provide an updated systematic review of published IG research on ADHD and related neurodevelopmental domains.
Since the last review, there has been an increase in the number of sMRI, fMRI, DTI and SPECT studies, and stagnation in the number of EEG studies. The small sample size still represents the greatest limitation in IG studies of ADHD, although this issue can be addressed by pooling information in meta-analyses from large consortiums, such as ENIGMA (Enhancing NeuroImaging Genetics through Meta-Analysis) (Thompson et al. 2014), which can then provide sufficient statistical power and accurate conclusions (Ioannidis 2005).
Larger studies also require statistical techniques based on multivariate assessment, for the following reasons: (i) the multidimensionality of genomic and neuroimaging data, which challenges statistical significance because of the need to correct for multiple comparisons; (ii) the computational burden of most procedures; and (iii) the fact that it is not possible to account for complex multivariate relationships because univariate strategies usually ignore the joint effects that may exist between genes and potential covariations between brain regions.
We found that all studies performed univariate analyses of one gene variant and one brain region at a time. Using multivariate analytical strategies could improve the identification of genetic patterns affecting brain development in ADHD, for example (Liu and Calhoun 2014). The polygenic nature of ADHD symptoms highlights the importance of accounting for the joint effect of genetic variants or entire biological pathways, and not only the effect of a single genetic variant. For instance, Bralten et al. (2013) showed that pathway-based analyses (PBA) increases power to identify genetic factors involved in ADHD symptoms. Also, we recently illustrated the importance of using gene-set analysis (GSA) methods to explore new susceptibility genes related to the development of ADHD (Vilor-Tejedor et al. 2015). An application of PBA and GSA methods in an IG study of ADHD symptoms was proposed by Mous et al. (2015). These authors demonstrated that such multivariate strategies have an emerging role in discovering the relationships between genes and brain regions within ADHD symptoms, and that these are preferable to conventional univariate strategies. To our knowledge, this is the first application of PBA and GSA methods to evaluate the joint effects of candidate pathways, brain regions and ADHD symptoms. However, these applications do not focus on linking novel genes to ADHD symptoms, but on evaluating previously defined candidate pathways. Khadka et al. (2016) propose the use of parallel Independent Component Analysis (pICA) (Liu et al. 2009) to elucidate the relationships between clusters of single nucleotide polymorphisms and measurements of grey matter (GM) regions related to abnormality in ADHD individuals. This methodology allows us to link gene clusters to deficient GM volume in novel biological pathways.
While we found few examples of the use of multivariate strategies to assess complex relationships in IG studies of ADHD, research is increasingly focused on evaluating the biological mechanisms underlying the symptoms of ADHD.
In summary, IG research on ADHD is in its early stages, and the field is currently characterized by a small number of non-replicated studies. These studies have evaluated the combination of genetic variants and intermediate brain phenotypes linked to ADHD, assessed using neuroimaging methods. While combining this kind of information can provide insights on how genetic polymorphisms affect brain structure, function and chemistry, there is a clear need for large studies and the development of novel multimodal analytical strategies. The need of larger studies to improve statistical power and reproducibility can be met by designing multi-centre consortia. In addition, small targeted studies could help to replicate previous findings. Research could also benefit from adopting existing and more powerful analysis strategies that take advantage of current data and contribute to the identification of environmental, social and epidemiological synergistic effects. A number of methods have been successfully used in IG studies on other outcomes, such as schizophrenia (Cao et al. 2014; Cao et al. 2013; Chen et al. 2012; Jamadar et al. 2011; Meda et al. 2010; Mounce et al. 2014) and Alzheimer’s disease (Meda et al. 2012; Vounou et al. 2012; Zhang et al. 2014), which provides an encouraging precedent for their success application to ADHD in the future.
References
Aarts, E., van Holstein, M., Hoogman, M., Onnink, M., Kan, C., Franke, B., et al. (2015). Reward modulation of cognitive function in adult attention-deficit/hyperactivity disorder: a pilot study on the role of striatal dopamine. Behavioural Pharmacology, 26(1–2), 227–240. doi:10.1097/FBP.0000000000000116.
Baehne, C. G., Ehlis, A.-C., Plichta, M. M., Conzelmann, A., Pauli, P., Jacob, C., et al. (2009). Tph2 gene variants modulate response control processes in adult ADHD patients and healthy individuals. Molecular Psychiatry, 14(11), 1032–1039. doi:10.1038/mp.2008.39.
Bédard, A.-C., Schulz, K. P., Cook, E. H., Fan, J., Clerkin, S. M., Ivanov, I., et al. (2010). Dopamine transporter gene variation modulates activation of striatum in youth with ADHD. NeuroImage, 53(3), 935–942. doi:10.1016/j.neuroimage.2009.12.041.
Bis, J. C., DeCarli, C., Smith, A. V., van der Lijn, F., Crivello, F., Fornage, M., et al. (2012). Common variants at 12q14 and 12q24 are associated with hippocampal volume. Nature Genetics, 44(5), 545–551. doi:10.1038/ng.2237.
Bobb, A. J., Addington, A. M., Sidransky, E., Gornick, M. C., Lerch, J. P., Greenstein, D. K., et al. (2005). Support for association between ADHD and two candidate genes: NET1 and DRD1. American Journal of Medical Genetics. Part B, Neuropsychiatric Genetics : The Official Publication of the International Society of Psychiatric Genetics, 134B(1), 67–72. doi:10.1002/ajmg.b.30142.
Braet, W., Johnson, K. A., Tobin, C. T., Acheson, R., McDonnell, C., Hawi, Z., et al. (2011). fMRI activation during response inhibition and error processing: the role of the DAT1 gene in typically developing adolescents and those diagnosed with ADHD. Neuropsychologia, 49(7), 1641–1650. doi:10.1016/j.neuropsychologia.2011.01.001.
Bralten, J., Franke, B., Waldman, I., Rommelse, N., Hartman, C., Asherson, P., et al. (2013). Candidate genetic pathways for attention-deficit/hyperactivity disorder (ADHD) show association to hyperactive/impulsive symptoms in children with ADHD. Journal of the American Academy of Child and Adolescent Psychiatry, 52(11), 1204–1212.e1. doi:10.1016/j.jaac.2013.08.020.
Brown, A. B., Biederman, J., Valera, E. M., Doyle, A. E., Bush, G., Spencer, T., et al. (2010). Effect of dopamine transporter gene (SLC6A3) variation on dorsal anterior cingulate function in attention-deficit/hyperactivity disorder. American Journal of Medical Genetics. Part B, Neuropsychiatric Genetics : The Official Publication of the International Society of Psychiatric Genetics, 153B(2), 365–375. doi:10.1002/ajmg.b.31022.
Cao, H., Duan, J., Lin, D., Calhoun, V., & Wang, Y.-P. (2013). Integrating fMRI and SNP data for biomarker identification for schizophrenia with a sparse representation based variable selection method. BMC Medical Genomics, 6(Suppl 3), S2. doi:10.1186/1755-8794-6-S3-S2.
Cao, H., Duan, J., Lin, D., Shugart, Y. Y., Calhoun, V., & Wang, Y.-P. (2014). Sparse representation based biomarker selection for schizophrenia with integrated analysis of fMRI and SNPs. NeuroImage, 102(Pt 1), 220–228. doi:10.1016/j.neuroimage.2014.01.021.
Castellanos, F.X., Lau, E., Tayebi, N., Lee, P., Long, R.E., Giedd, J.N., Sharp, W., Marsh, W.L., Walter, J.M., Hamburger, S.D., Ginns, E.I., Rapoport, J.L., Sidransky, E. (1998). Lack of an association between a dopamine-4 receptor polymorphism and attentiondeficit/hyperactivity disorder: genetic and brain morphometric analyses. Retrieved September 9, 2015, from http://www.nature.com/mp/journal/v3/n5/pdf/4000430a.pdf?origin=publication_detail.
Chen, J., Calhoun, V. D., Pearlson, G. D., Ehrlich, S., Turner, J. A., Ho, B.-C., et al. (2012). Multifaceted genomic risk for brain function in schizophrenia. NeuroImage, 61(4), 866–875. doi:10.1016/j.neuroimage.2012.03.022.
Cheon, K.-A., Ryu, Y.-H., Kim, J.-W., & Cho, D.-Y. (2005). The homozygosity for 10-repeat allele at dopamine transporter gene and dopamine transporter density in Korean children with attention deficit hyperactivity disorder: relating to treatment response to methylphenidate. European Neuropsychopharmacology : The Journal of the European College of Neuropsychopharmacology, 15(1), 95–101. doi:10.1016/j.euroneuro.2004.06.004.
Cortese, S. (2012). The neurobiology and genetics of Attention-Deficit/Hyperactivity Disorder (ADHD): what every clinician should know. European Journal of Paediatric Neurology : EJPN : Official Journal of the European Paediatric Neurology Society, 16(5), 422–433. doi:10.1016/j.ejpn.2012.01.009.
De Zeeuw, P., van Belle, J., van Dijk, S., Weusten, J., Koeleman, B., Janson, E., … Durston, S. (2013). Imaging gene and environmental effects on cerebellum in Attention-Deficit/Hyperactivity Disorder and typical development. NeuroImage: Clinical, 2, 103–110. doi:10.1016/j.nicl.2012.11.010.
Durston, S. (2010). Imaging genetics in ADHD. Retrieved September 3, 2015, from http://www.ncbi.nlm.nih.gov/pubmed/20206707.
Durston, S., Fossella, J. A., Casey, B. J., Hulshoff Pol, H. E., Galvan, A., Schnack, H. G., et al. (2005). Differential effects of DRD4 and DAT1 genotype on fronto-striatal gray matter volumes in a sample of subjects with attention deficit hyperactivity disorder, their unaffected siblings, and controls. Molecular Psychiatry, 10(7), 678–685. doi:10.1038/sj.mp.4001649.
Durston, S., Fossella, J. A., Mulder, M. J., Casey, B. J., Ziermans, T. B., Vessaz, M. N., & Van Engeland, H. (2008). Dopamine transporter genotype conveys familial risk of attention-deficit/hyperactivity disorder through striatal activation. Journal of the American Academy of Child and Adolescent Psychiatry, 47(1), 61–67. doi:10.1097/chi.0b013e31815a5f17.
Faraone, S. V., & Mick, E. (2010). Molecular genetics of attention deficit hyperactivity disorder. The Psychiatric Clinics of North America, 33(1), 159–180. doi:10.1016/j.psc.2009.12.004.
Faraone, S. V., Sergeant, J., Gillberg, C., & Biederman, J. (2003). The worldwide prevalence of ADHD: is it an American condition? World Psychiatry: Official Journal of the World Psychiatric Association (WPA), 2(2), 104–113 Retrieved from http://www.pubmedcentral.nih.gov/articlerender.fcgi?artid=1525089&tool=pmcentrez&rendertype=abstract.
Faraone, S. V., Bonvicini, C., & Scassellati, C. (2014). Biomarkers in the diagnosis of ADHD--promising directions. Current Psychiatry Reports, 16(11), 497. doi:10.1007/s11920-014-0497-1.
Fone, K. C. F., & Nutt, D. J. (2005). Stimulants: use and abuse in the treatment of attention deficit hyperactivity disorder. Current Opinion in Pharmacology, 5(1), 87–93. doi:10.1016/j.coph.2004.10.001.
Franke, B., Faraone, S. V., Asherson, P., Buitelaar, J., Bau, C. H. D., Ramos-Quiroga, J. A., et al. (2012). The genetics of attention deficit/hyperactivity disorder in adults, a review. Molecular Psychiatry, 17(10), 960–987. doi:10.1038/mp.2011.138.
Franke, B., Stein, J. L., Ripke, S., Anttila, V., Hibar, D. P., van Hulzen, K. J. E., et al. (2016). Genetic influences on schizophrenia and subcortical brain volumes: large-scale proof of concept. Nature Neuroscience, 19(3), 420–431. doi:10.1038/nn.4228.
Gehricke, J.G., Swanson, J.M., Duong, S., Nguyen, J., Wigal, T.L., Fallon, J., et al. (2015) Increased brain activity to unpleasant stimuli in individuals with the 7R allele of the DRD4 gene. Psychiatry Res, 30;231(1), 58–63. doi:10.1016/j.pscychresns.2014.10.021.
Gizer, I. R., Ficks, C., & Waldman, I. D. (2009). Candidate gene studies of ADHD: a meta-analytic review. Human Genetics, 126(1), 51–90. doi:10.1007/s00439-009-0694-x.
Glahn, D. C., Paus, T., & Thompson, P. M. (2007). Imaging genomics: mapping the influence of genetics on brain structure and function. Human Brain Mapping, 28(6), 461–463. doi:10.1002/hbm.20416.
Hibar, D. P., Stein, J. L., Renteria, M. E., Arias-Vasquez, A., Desrivières, S., Jahanshad, N., et al. (2015). Common genetic variants influence human subcortical brain structures. Nature, 520(7546), 224–229. doi:10.1038/nature14101.
Hong, S.-B., Zalesky, A., Park, S., Yang, Y.-H., Park, M.-H., Kim, B., et al. (2015). COMT genotype affects brain white matter pathways in attention-deficit/hyperactivity disorder. Human Brain Mapping, 36(1), 367–377. doi:10.1002/hbm.22634.
Hoogman, M., Onnink, M., Cools, R., Aarts, E., Kan, C., Arias Vasquez, A., et al. (2013). The dopamine transporter haplotype and reward-related striatal responses in adult ADHD. European Neuropsychopharmacology : The Journal of the European College of Neuropsychopharmacology, 23(6), 469–478. doi:10.1016/j.euroneuro.2012.05.011.
Ioannidis, J. P. A. (2005). Why most published research findings are false. PLoS Medicine, 2(8), e124. doi:10.1371/journal.pmed.0020124.
Jamadar, S., Powers, N. R., Meda, S. A., Gelernter, J., Gruen, J. R., & Pearlson, G. D. (2011). Genetic influences of cortical gray matter in language-related regions in healthy controls and schizophrenia. Schizophrenia Research, 129(2–3), 141–148. doi:10.1016/j.schres.2011.03.027.
Kabukcu Basay, B., Buber, A., Basay, O., Alacam, H., Ozturk, O., Suren, S., et al. (2016). White matter alterations related to attention-deficit hyperactivity disorder and COMT val(158)met polymorphism: children with valine homozygote attention-deficit hyperactivity disorder have altered white matter connectivity in the right cingulum. Neuropsychiatric Disease and Treatment, 12, 969–981. doi:10.2147/NDT.S104450.
Khadka, S., Pearlson, G. D., Calhoun, V. D., Liu, J., Gelernter, J., Bessette, K. L., & Stevens, M. C. (2016). Multivariate Imaging Genetics Study of MRI Gray Matter Volume and SNPs Reveals Biological Pathways Correlated with Brain Structural Differences in Attention Deficit Hyperactivity Disorder. Frontiers in Psychiatry, 7, 128. doi:10.3389/fpsyt.2016.00128.
Kim, B.-N., Kim, J.-W., Kang, H., Cho, S.-C., Shin, M.-S., Yoo, H.-J., et al. (2010). Regional differences in cerebral perfusion associated with the alpha-2A-adrenergic receptor genotypes in attention deficit hyperactivity disorder. Journal of Psychiatry & Neuroscience : JPN, 35(5), 330–336. doi:10.1503/jpn.090168.
Krause, J., Dresel, S.H., Krause, K.-H., Fougère, C. La, Zill, P., Ackenheil, M. (2009). Striatal dopamine transporter availability and DAT-1 gene in adults with ADHD: no higher DAT availability in patients with homozygosity for the 10-repeat allele. The World Journal of Biological Psychiatry. Retrieved from http://www-tandfonline-com.sare.upf.edu/doi/abs/10.1080/15622970500518444#.VfA9PhG8PRY.
LaHoste, G. J., Swanson, J. M., Wigal, S. B., Glabe, C., Wigal, T., King, N., & Kennedy, J. L. (1996). Dopamine D4 receptor gene polymorphism is associated with attention deficit hyperactivity disorder. Molecular Psychiatry, 1(2), 121–124 Retrieved from http://www.ncbi.nlm.nih.gov/pubmed/9118321.
Lantieri, F., Glessner, J. T., Hakonarson, H., Elia, J., & Devoto, M. (2010). Analysis of GWAS top hits in ADHD suggests association to two polymorphisms located in genes expressed in the cerebellum. American Journal of Medical Genetics Part B: Neuropsychiatric Genetics, 153B(6), n/a–n/a. doi:10.1002/ajmg.b.31110.
Liu, J., & Calhoun, V. D. (2014). A review of multivariate analyses in imaging genetics. Frontiers in Neuroinformatics, 8, 29. doi:10.3389/fninf.2014.00029.
Liu, J., Pearlson, G., Windemuth, A., Ruano, G., Perrone-Bizzozero, N. I., & Calhoun, V. (2009). Combining fMRI and SNP data to investigate connections between brain function and genetics using parallel ICA. Human Brain Mapping, 30(1), 241–255. doi:10.1002/hbm.20508.
Loo, S. K., Specter, E., Smolen, A., Hopfer, C., Teale, P. D., & Reite, M. L. (2003). Functional effects of the DAT1 polymorphism on EEG measures in ADHD. Journal of the American Academy of Child and Adolescent Psychiatry, 42(8), 986–993. doi:10.1097/01.CHI.0000046890.27264.88.
Maher, B. (2008). Personal genomes: The case of the missing heritability. Nature, 456(7218), 18–21. doi:10.1038/456018a.
Manolio, T. A., Collins, F. S., Cox, N. J., Goldstein, D. B., Hindorff, L. A., Hunter, D. J., et al. (2009). Finding the missing heritability of complex diseases. Nature, 461(7265), 747–753. doi:10.1038/nature 08494.
Meda, S. A., Jagannathan, K., Gelernter, J., Calhoun, V. D., Liu, J., Stevens, M. C., & Pearlson, G. D. (2010). A pilot multivariate parallel ICA study to investigate differential linkage between neural networks and genetic profiles in schizophrenia. NeuroImage, 53(3), 1007–1015. doi:10.1016/j.neuroimage.2009.11.052.
Meda, S. A., Narayanan, B., Liu, J., Perrone-Bizzozero, N. I., Stevens, M. C., Calhoun, V. D., et al. (2012). A large scale multivariate parallel ICA method reveals novel imaging-genetic relationships for Alzheimer’s disease in the ADNI cohort. NeuroImage, 60(3), 1608–1621. doi:10.1016/j.neuroimage.2011.12.076.
Meyer-Lindenberg, A. (2010). Imaging genetics of schizophrenia. Dialogues in Clinical Neuroscience, 12(4), 449–456 Retrieved from http://www.pubmedcentral.nih.gov/articlerender.fcgi?artid=3181991&tool=pmcentrez&rendertype=abstract.
Monuteaux, M. C., Seidman, L. J., Faraone, S. V., Makris, N., Spencer, T., Valera, E., et al. (2008). A preliminary study of dopamine D4 receptor genotype and structural brain alterations in adults with ADHD. American Journal of Medical Genetics. Part B, Neuropsychiatric Genetics : The Official Publication of the International Society of Psychiatric Genetics, 147B(8), 1436–1441. doi:10.1002/ajmg.b.30870.
Mounce, J., Luo, L., Caprihan, A., Liu, J., Perrone-Bizzozero, N. I., & Calhoun, V. D. (2014). Association of GRM3 polymorphism with white matter integrity in schizophrenia. Schizophrenia Research, 155(1–3), 8–14. doi:10.1016/j.schres.2014.03.003.
Mous, S. E., Hammerschlag, A. R., Polderman, T. J. C., Verhulst, F. C., Tiemeier, H., van der Lugt, A., et al. (2015). A Population-Based Imaging Genetics Study of Inattention/Hyperactivity: Basal Ganglia and Genetic Pathways. Journal of the American Academy of Child and Adolescent Psychiatry, 54(9), 745–752. doi:10.1016/j.jaac.2015.05.018.
Mulder, M. J., Baeyens, D., Davidson, M. C., Casey, B. J., van den Ban, E., van Engeland, H., & Durston, S. (2008). Familial vulnerability to ADHD affects activity in the cerebellum in addition to the prefrontal systems. Journal of the American Academy of Child and Adolescent Psychiatry, 47(1), 68–75. doi:10.1097/chi.0b013e31815a56dc.
Mulligan, R. C., Kristjansson, S. D., Reiersen, A. M., Parra, A. S., Anokhin, A. P. (2014). Neural correlates of inhibitory control and functional genetic variation in the dopamine D4 receptor gene. Neuropsychologia, 62, 306–318. doi:10.1016/j.neuropsychologia.2014.07.033.
Neale, B. M., Lasky-Su, J., Anney, R., Franke, B., Zhou, K., Maller, J. B., et al. (2008). Genome-wide association scan of attention deficit hyperactivity disorder. American Journal of Medical Genetics. Part B, Neuropsychiatric Genetics : The Official Publication of the International Society of Psychiatric Genetics, 147B(8), 1337–1344. doi:10.1002/ajmg.b.30866.
Nymberg, C., Jia, T., Lubbe, S., Ruggeri, B., Desrivieres, S., Barker, G., et al. (2013). Neural mechanisms of attention-deficit/hyperactivity disorder symptoms are stratified by MAOA genotype. Biological Psychiatry, 74(8), 607–614. doi:10.1016/j.biopsych.2013.03.027.
Olfson, M. (1992). Diagnosing mental disorders in office-based pediatric practice. Journal of Developmental and Behavioral Pediatrics : JDBP, 13(5), 363–365 Retrieved from http://www.ncbi.nlm.nih.gov/pubmed/1401121.
Onnink, A. M. H., Franke, B., van Hulzen, K., Zwiers, M. P., Mostert, J. C., Schene, A. H., et al. (2016). Enlarged striatal volume in adults with ADHD carrying the 9-6 haplotype of the dopamine transporter gene DAT1. Journal of Neural Transmission (Vienna, Austria : 1996), 123(8), 905–915. doi:10.1007/s00702-016-1521-x.
Paloyelis, Y., Mehta, M. A., Faraone, S. V., Asherson, P., & Kuntsi, J. (2012). Striatal sensitivity during reward processing in attention-deficit/hyperactivity disorder. Journal of the American Academy of Child and Adolescent Psychiatry, 51(7), 722–732.e9. doi:10.1016/j.jaac.2012.05.006.
Papassotiropoulos, A., Stefanova, E., Vogler, C., Gschwind, L., Ackermann, S., Spalek, K., et al. (2013). A genome-wide survey and functional brain imaging study identify CTNNBL1 as a memory-related gene. Molecular Psychiatry, 18(2), 255–263. doi:10.1038/mp.2011.148.
Park, S., Hong, S.-B., Kim, J.-W., Yang, Y.-H., Park, M.-H., Kim, B.-N., … Cho, S.-C. (2013). White-Matter Connectivity and Methylphenidate-Induced Changes in Attentional Performance According to α2A–Adrenergic Receptor Gene Polymorphisms in Korean Children With Attention-Deficit Hyperactivity Disorder. The Journal of Neuropsychiatry and Clinical Neurosciences. Retrieved from http://neuro.psychiatryonline.org/doi/ref/10.1176/appi.neuropsych.12050121.
Polanczyk, G., de Lima, M. S., Horta, B. L., Biederman, J., & Rohde, L. A. (2007). The worldwide prevalence of ADHD: a systematic review and metaregression analysis. The American Journal of Psychiatry, 164(6), 942–948. doi:10.1176/ajp.2007.164.6.942.
Potkin, S. G., Turner, J. A., Guffanti, G., Lakatos, A., Fallon, J. H., Nguyen, D. D., et al. (2009). A genome-wide association study of schizophrenia using brain activation as a quantitative phenotype. Schizophrenia Bulletin, 35(1), 96–108. doi:10.1093/schbul/sbn155.
Risacher, S. L., Shen, L., West, J. D., Kim, S., McDonald, B. C., Beckett, L. A., et al. (2010). Longitudinal MRI atrophy biomarkers: relationship to conversion in the ADNI cohort. Neurobiology of Aging, 31(8), 1401–1418. doi:10.1016/j.neurobiolaging.2010.04.029.
Rohde, L. A., Roman, T., Szobot, C., Cunha, R. D., Hutz, M. H., & Biederman, J. (2003). Dopamine transporter gene, response to methylphenidate and cerebral blood flow in attention-deficit/hyperactivity disorder: a pilot study. Synapse (New York, N.Y.), 48(2), 87–89. doi:10.1002/syn.10186.
Sánchez-Mora, C., Richarte, V., Garcia-Martínez, I., Pagerols, M., Corrales, M., Bosch, R., et al. (2015). Dopamine receptor DRD4 gene and stressful life events in persistent attention deficit hyperactivity disorder. American Journal of Medical Genetics. Part B, Neuropsychiatric Genetics : The Official Publication of the International Society of Psychiatric Genetics. doi:10.1002/ajmg.b.32340.
Shaw, P., Gornick, M., Lerch, J., Addington, A., Seal, J., Greenstein, D., et al. (2007). Polymorphisms of the dopamine D4 receptor, clinical outcome, and cortical structure in attention-deficit/hyperactivity disorder. Archives of General Psychiatry, 64(8), 921–931. doi:10.1001/archpsyc.64.8.921.
Shook, D., Brady, C., Lee, P. S., Kenealy, L., Murphy, E. R., Gaillard, W. D., et al. (2011). Effect of dopamine transporter genotype on caudate volume in childhood ADHD and controls. American Journal of Medical Genetics. Part B, Neuropsychiatric Genetics : The Official Publication of the International Society of Psychiatric Genetics, 156B(1), 28–35. doi:10.1002/ajmg.b.31132.
Stein, J. L., Hua, X., Lee, S., Ho, A. J., Leow, A. D., Toga, A. W., et al. (2010). Voxelwise genome-wide association study (vGWAS). NeuroImage, 53(3), 1160–1174. doi:10.1016/j.neuroimage.2010.02.032.
Szobot, C., Roman, T., Cunha, R., Acton, P., Hutz, M., & Rohde, L. A. (2005). Brain perfusion and dopaminergic genes in boys with attention-deficit/hyperactivity disorder. American Journal of Medical Genetics. Part B, Neuropsychiatric Genetics : The Official Publication of the International Society of Psychiatric Genetics, 132B(1), 53–58. doi:10.1002/ajmg.b.30096.
Szobot, C. M., Roman, T., Hutz, M. H., Genro, J. P., Shih, M. C., Hoexter, M. Q., et al. (2011). Molecular imaging genetics of methylphenidate response in ADHD and substance use comorbidity. Synapse (New York, N.Y.), 65(2), 154–159. doi:10.1002/syn.20829.
Tarver, J., Daley, D., & Sayal, K. (2014). Attention-deficit hyperactivity disorder (ADHD): an updated review of the essential facts. Child: Care, Health and Development, 40(6), 762–774. doi:10.1111/cch.12139.
Thompson, P. M., Stein, J. L., Medland, S. E., Hibar, D. P., Vasquez, A. A., Renteria, M. E., et al. (2014). The ENIGMA Consortium: large-scale collaborative analyses of neuroimaging and genetic data. Brain Imaging and Behavior, 8(2), 153–182. doi:10.1007/s11682-013-9269-5.
Tortajada-Genaro, L. A., Mena, S., Niñoles, R., Puigmule, M., Viladevall, L., & Maquieira, Á. (2016). Genotyping of single nucleotide polymorphisms related to attention-deficit hyperactivity disorder. Analytical and Bioanalytical Chemistry, 408(9), 2339–2345. doi:10.1007/s00216-016-9332-3.
Valera, E. M., Faraone, S. V., Murray, K. E., & Seidman, L. J. (2007). Meta-analysis of structural imaging findings in attention-deficit/hyperactivity disorder. Biological Psychiatry, 61(12), 1361–1369. doi:10.1016/j.biopsych.2006.06.011.
van der Meer, D., Hoekstra, P. J., Zwiers, M., Mennes, M., Schweren, L. J., Franke, B., et al. (2015). Brain Correlates of the Interaction Between 5-HTTLPR and Psychosocial Stress Mediating Attention Deficit Hyperactivity Disorder Severity. The American Journal of Psychiatry, 172(8), appiajp201514081035. doi:10.1176/appi.ajp.2015.14081035.
van Ewijk, H., Heslenfeld, D. J., Zwiers, M. P., Buitelaar, J. K., & Oosterlaan, J. (2012). Diffusion tensor imaging in attention deficit/hyperactivity disorder: a systematic review and meta-analysis. Neuroscience and Biobehavioral Reviews, 36(4), 1093–1106. doi:10.1016/j.neubiorev.2012.01.003.
van Rooij, D., Hoekstra, P. J., Bralten, J., Hakobjan, M., Oosterlaan, J., Franke, B., et al. (2015). Influence of DAT1 and COMT variants on neural activation during response inhibition in adolescents with attention-deficit/hyperactivity disorder and healthy controls. Psychological Medicine, 45(15), 3159–3170. doi:10.1017/S0033291715001130.
Vilor-Tejedor, N., Gonzalez, J. R., & Calle, M. L. (2015). Efficient and powerful method for combining p-values in Genome-wide Association Studies. IEEE/ACM Transactions on Computational Biology and Bioinformatics. doi:10.1109/TCBB.2015.2509977.
Vilor-Tejedor, N., Alemany, S., Forns, J., Cáceres, A., Murcia, M., Macià, D., et al. (2016). Assessment of Susceptibility Risk Factors for ADHD in Imaging Genetic Studies. Journal of Attention Disorders. doi:10.1177/1087054716664408.
Vounou, M., Janousova, E., Wolz, R., Stein, J. L., Thompson, P. M., Rueckert, D., & Montana, G. (2012). Sparse reduced-rank regression detects genetic associations with voxel-wise longitudinal phenotypes in Alzheimer’s disease. NeuroImage, 60(1), 700–716. doi:10.1016/j.neuroimage.2011.12.029.
Weiner, M. W., Veitch, D. P., Aisen, P. S., Beckett, L. A., Cairns, N. J., Cedarbaum, J., et al. (2015). Impact of the Alzheimer’s Disease Neuroimaging Initiative, 2004 to 2014. Alzheimer’s & Dementia : The Journal of the Alzheimer’s Association, 11(7), 865–884. doi:10.1016/j.jalz.2015.04.005.
Zayed, A., & Robinson, G. E. (2012). Understanding the relationship between brain gene expression and social behavior: lessons from the honey bee. Annual Review of Genetics, 46, 591–615. doi:10.1146/annurev-genet-110711-155517.
Zhang, L., Chang, S., Li, Z., Zhang, K., Du, Y., Ott, J., & Wang, J. (2012). ADHDgene: a genetic database for attention deficit hyperactivity disorder. Nucleic Acids Research, 40(Database issue), D1003–D1009. doi:10.1093/nar/gkr992.
Zhang, Z., Huang, H., & Shen, D. (2014). Integrative analysis of multi-dimensional imaging genomics data for Alzheimer’s disease prediction. Frontiers in Aging Neuroscience, 6, 260. doi:10.3389/fnagi.2014.00260.
Acknowledgments
Natalia Vilor-Tejedor is funded by a pre-doctoral grant from the Agència de Gestió d’Ajuts Universitaris i de Recerca (2015 FI_B 00636), Generalitat de Catalunya - Fons Social Europeu. This research was also supported by grants MTM2015-68140-R from the Ministerio de Economía e Innovación (Spain) and the European Research Council under the ERC Grant Agreement number 268479. Also, the authors are grateful to Mireia Gascón and Mariona Bustamante for their support and advice.
Author information
Authors and Affiliations
Corresponding author
Ethics declarations
This study was funded by a pre-doctoral grant from the Agència de Gestió d’Ajuts Universitaris i de Recerca (2015 FI_B 00636), Generalitat de Catalunya - Fons Social Europeu, grant MTM2015–68140-R from the Ministerio de Economía e Innovación (Spain) and the European Research Council under the ERC Grant Agreement number 268479.
Ethical approval
This article does not contain any studies with human participants or animals performed by any of the authors.
Electronic supplementary material
ESM 1
(XLSX 30 kb)
Rights and permissions
About this article
Cite this article
Vilor-Tejedor, N., Cáceres, A., Pujol, J. et al. Imaging genetics in attention-deficit/hyperactivity disorder and related neurodevelopmental domains: state of the art. Brain Imaging and Behavior 11, 1922–1931 (2017). https://doi.org/10.1007/s11682-016-9663-x
Published:
Issue Date:
DOI: https://doi.org/10.1007/s11682-016-9663-x