Abstract
Based on Chinese provincial-level data from 2012 to 2020,this paper construct the regional digital economic development index by the CRITIC method and empirically finds an inhibiting effect of the digital economy development on innovation in renewable energy technology (IRETs). A mediation mechanism test shows that the inhibiting effect may be due to serious free-rider problem in IRETs as patent infringements increase in the digital era. Further studies show that moderation effect of public environmental appeal on the relationship between digital economy development and IRETs depends on the level of patent judicial protection. It is found that increasing public environmental appeal will mitigate the negative impact of digital economy development on IRETs for regions with high level of patent judicial protection while enhance the above negative impact for regions with low level of patent judicial protection.
Similar content being viewed by others
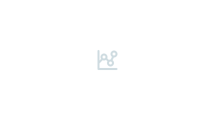
Explore related subjects
Discover the latest articles, news and stories from top researchers in related subjects.Avoid common mistakes on your manuscript.
1 Introduction
China has become the largest coal producer in the world (Govindaraju and Tang 2013). The long-term energy structure dominated by coal has brought irreversible ecological destruction represented by resource waste and environmental pollution (Hao et al. 2015). In the process of transformation to a low-carbon economy and society, research into and use of renewable energy has become an important focus of sustainable development strategies (Lin and Zhu 2019a). At present, the digital economy has become the main driver of global economic growth (Litvinenko 2020) and contributes to low-carbon development (Chen 2023). As defined in the G20 Digital Economy Development and Cooperation Initiative released in 2016, the digital economy refers to a series of economic activities that use digital knowledge and information as key production factors, modern information networks as important carriers, and the effective use of information and communication technology as an important driving force for efficiency improvement and economic structure optimization. However, the broad complementarities brought by rapid digital economy development pose novel challenges in coordination and market design for innovators (Teece 2018) and may reduce regional investments in innovations under the poor environment of judicial protection on intellectual property (Chen and Wu 2022). Given this, will the digital economy drive or inhibit innovation in renewable energy technology (IRETs)?
To answer the above question, this paper construct the regional digital economic development index by the CRITIC method and empirically test the impact the digital economy development on IRETs.. It is empirically found that there exists an inhibiting effect of the digital economy development on IRETs due to the increasing patent infringements in the digital era. The conclusion holds for a variety of robust tests. Further studies show that moderation effect of public environmental appeal on the relationship between digital economy development and IRETs depends on the level of patent judicial protection. It is found that increasing public environmental appeal will mitigate the negative impact of digital economy development on IRETs for regions with high level of patent judicial protection while enhance the above negative impact for regions with low level of patent judicial protection.
The possible contributions of this paper lay in the following three aspects: (1) based on the existing literature, this paper comprehensively measures the digital economy development level using CRITIC method at the provincial level and discusses the impact of digital economy development on IRETs for the first time. Referring to the design of the international authoritative measurement systems, and considering that the development of China’s digital economy mainly revolves around digital industrialization and industrial digitization, this paper innovatively proposes six dimensions used to describe the development of China’s regional digital economy. (2) This paper finds that the increasing patent infringement contributes to the digital economy’s inhibiting IRETs. This finding confirms the new challenge for innovators in the digital era (Teece 2018; Chen 2020) and further points the rapid digital economy development may bring damage to the low-carbon development of the economy without solving the free-rider problem. (3) This paper discusses the value of public environmental appeal in eco-innovation in the digital era. Different from the previous mainstream literature confirming the positive impact of public appeal on eco-innovation (Hojnik and Ruzzier 2016; Du et al. 2019), this paper finds the moderation effect of public environmental appeal on the relationship between digital economy development and IRETs depends on the level of patent judicial protection. It is found that public environmental appeal can benfit IRETs only when the level of patent judicial protection is high.
The remainder of this paper is organized as follows. Sect. 2 summarizes the literature and proposes the basic hypotheses of the research. Section 3 describes the selected variables and data. Section 4 introduces methodology and model specifications. Section 5 analyzes and discusses the empirical results. Section 6 concludes and provides policy implications.
2 Literature review and hypotheses development
Regarded as the application of digital technologies to economy development, the digital economy is attaching more importance from the government and academia, since it can effectively promote technological innovation and transformation of industrial models, facilitating the attainment of the carbon reduction goal. Most research on the impact of digital technology and carbon emissions is limited to discussing the impact of ICT development represented by the penetration of the Internet and mobile phones (Lee and Brahmasrene 2014; Higón et al. 2017; Park et al. 2018).Footnote 1 The existing research conclusions are quite divergent. Some scholars believe that increased investment in ICT infrastructure and the use of ICT and other digital technologies can help reduce carbon emissions through improving productivity and energy efficiency in agriculture, transportation, industry, and other fields (Dehghan Shabani and Shahnazi 2019; Amri et al. 2019; Ben Lahouel et al. 2021), or stimulating the development and use of clean energy (Azam et al. 2021; Murshed et al. 2020), while others contend that the increase in the production, use, and recycling of ICT hardware equipment will lead to an increase in energy consumption, especially electricity demand, and bring adverse effects on the environment (Andrae and Edler 2015; Belkhir and Elmeligi 2018).
The progress of innovation in renewable energy technologies is an important factor that influences the development of renewable energy, which supports the transition to a low-carbon economy and society (Lin and Zhu 2019a). However, with the rapid development of digital economy, regional performance of IRETs may be not promising due to the internal characteristics of eco-innovations, which differs from that of no-environmental innovations (Kemp and Oltra 2011; De Marchi 2012). There is an issue of double externality: eco-innovations produce positive spillovers in both innovation and diffusion stages. In the presence of (positive) externalities, firms have smaller incentives to develop innovations, which might result in underinvestment in eco-technologies given that their benefits are not valued by the market (Cecere et al. 2014). In fact, the rapid development of digital economy provided “soil” for the occurrence of intellectual property infringements (Bessen et al. 2018; Cohen et al. 2019; Kim et al. 2018; Mezzanotti 2021; Xu 2019). According to data published by Westlaw–Thomson Reuters, the number of patent litigation cases has more than tripled in the past 30 years. The broad complementarities of innovations in the digital era pose novel challenges in coordination and market design for innovators (Teece 2018), and the rapid digital economy development may reduce regional investments in innovations under the poor environment of judicial protection on intellectual property (Chen and Wu 2022).
Thus, we give the following hypothesis:
Hypothesis 1
The regional digital economy development harms the IRETs.
More recently, scholars also discussed the effects of the public environmental appeal on corporate eco-innovation. Most of scholars believe that public environmental appeal is a key driver of eco-innovation by enterprises. First, according to the theory of information asymmetry, public environmental appeal can alleviate the “information asymmetry” between external stakeholders and internal managers and the actual demand of the public for environmental protection products may also be transmitted to enterprises (Li et al. 2017). Second, based on the reputation mechanism, environmental innovation is the centralized embodiment of enterprises’ commitment and fulfillment of social responsibilities (Hojnik and Ruzzier 2016). Du et al. (2019) pointed that the increase of public environmental appeal can promote innovation level of heavy-polluting enterprises by promoting analyst coverage and reputation mechanism. Finally, public appeal promotes local governments’ enforcement of stricter environmental regulation, thereby encouraging firms to increase their green investment. (Liao et al. 2018).
However, some articles pointed that the public environmental appeal may not bring significantly beneficial impact to enterprises’ eco-innovation. Jensen and Meckling (1976) argued that public environmental appeal, while exerting its information intermediary effect on influence enterprise decision-making, may also place great market pressure on enterprises. This intensifies managers’ worries, easily inducing short-sighted behaviors, such as “pollution control” becoming “pollution transfer.” Further, according to cost–benefit theory, given that environmental performance is currently measured by emissions reduction rather than innovation, enterprises have to increase investment in pollution control. Liao et al.(2020) found that public surveillance had no significant effect on incremental eco-innovation. The increase in pollution control costs is bound to crowd out innovation capital, leading eventually to a decrease in the efficiency of green innovation (Liao 2018).
Therefore, combined with the background of digital transformation of the whole economy, we deeply explores the role of public environmental appeal on IRETs. When there is no effective solution to the free-rider problem of the development of digital economy, with the improvement of public environmental appeal, enterprises are more likely to control pollution emissions rather than actively carry out IRETs in the digital era. In this way, with the improvement of public environmental appeal, the negative effect of the digital economy development on IRETs is further enhanced. However, when the free-rider problem of digital economy development is effectively solved, the promotion of public environmental appeal can enhance the enthusiasm of enterprises in IRETs. In this way, with the improvement of public environmental appeal, the negative effect of digital economy development on IRETs can be alleviated. A unique form of legal protection should be designed to deal with the free-rider problem in innovations (Diwan and Rodrik 1991; Lemey 2004).
Based on the above discussions, we give the following hypothesis:
Hypothesis 2
The policy effect of public environment appeal on the relationship between digital economy development and IRETs depends on the regional patent judicial protection level.
3 Model specifications
Based on the above theoretical analysis, test the impact of digital economy development on IRETs, this paper constructs the following two-way fixed effects model:
where subscripts \(i\) and \(t\) represent the region (province) and year, respectively. \({\text{IRET}}_{it}\) denotes the level of IRETs of region \(i\) in \(t\) year, \({\text{Digital}}_{it}\) denotes the digital economy development level of region \(i\) in \(t\) year. Vector quantity \(X_{it}\) represents a series of control variables. \(\mu_i\) denotes a regional fixed effect; \(\delta_i\) denotes a year fixed effect; \(\varepsilon_{it}\) is a random disturbance term; and \(\alpha_1\) represents estimated parameters. \(\alpha_1\) is expected to be significantly negative in this model., which means that the digital economy development inhibits IRETs.
Besides, to explore the mechanisms of the effect of the digital economy on IRETs, this paper introduced the number of patent infringement cases to measure patent infringement. The method of Preacher and Kelley (2011) is used to verify whether patent infringement (\({\text{Regist}}\)) constitutes the mediating variable. The following regression models are set to test the mediation effect:
The mediation effect is determined by the significance of regression coefficients \(\beta_1\) and \(\gamma_1\). \(\beta_1\) is expected to be significantly positive, and \(\gamma_1\) is expected to be significantly negative.
4 Data and descriptive statistics
4.1 Explained variable: IRETs
The variable explained in this study is innovation in renewable energy technologies. In general, innovation can be measured both by means of input (such as R&D expenditure or investments made), and output (yield)-oriented activity, which, together, account for the results of the innovation process, such as patenting activity. Although investments made in renewable energy technologies may be a better indicator of renewable energy innovation efforts, there is no corresponding authoritative data source. In this paper, we have collected data for the latter. Despite this criticism and limitations, patent data are still the most commonly used determinant of innovation, and the one that this study uses to model innovation outcomes (Pitelis et al. 2020). Therefore, in the research topic of influencing factors on innovation in renewable energy technologies, the number of renewable energy patents is widely used as a proxy variable for innovation level (Lin and Zhu 2019a, b; Ren et al. 2021; Lee et al. 2022).
Following Lin and Zhu (2019a, b), this paper uses the latest International Patent Classification (IPC) code to count the number of patents in wind power, solar energy, marine (ocean) energy, hydro power, biomass energy, and storage. The sum of the patents of six kinds of renewable energy is used to describe the level of IRETs.
4.2 Core explanatory variable: the digital economy development
The core explanatory variable of this paper is the digital economy development at provincial level. So far, there has yet to be an authoritative index for describing the development of the Chinese digital economy. Some literature uses relevant data from the information and communication technology (ICT) industry to measure the digital economy development level (García-Herrero and Xu 2018; Moroz 2017)). However, only using the ICT industry to represent the digital economy has limitations as the digital economy has grown beyond the concept of ICT sectors (Barefoot et al. 2018). Thus, other literature develops the calculations of the digital economy index, for example, the "Internet Plus" digital economy index designed by the Tencent Research Institute (He et al. 2022) and the self-constructed indices (Pan et al. 2022). The "Internet Plus" digital economy index comprehensively covers data from Tencent and other large digital enterprises but can only be used as cross-sectional data. Due to the dynamic adjustment of subdivision indicators and weights yearly, the data over the years are different. Regarding the subdivision indicators of the digital economy growth index constructed by scholars, Pan et al. (2022) selected three-dimensional indicators of the infrastructure, the industrial scale, and the spillover value.
Authoritative measures of the level of digital economy development on a global scale include the systems of the Organization for Economic Cooperation and Development (OECD) and the US Bureau of Economic Analysis (BEA). The OECD uses four factors, including investment in intelligent infrastructure, the level of ICT’s promoting a digital society, the capacity of digital technology innovation, and the extent to which ICT’s facilitating employment and economic growth (OECD 2014). The BEA’s measurement of the development level covers digital infrastructure, e-commerce, and digital media. Referring to the design of these two international authoritative measurement systems and considering that the growth of the digital economy in China revolves around the two main paths of digital industrialization and industrial digitization,Footnote 2 This paper constitutes an index for describing the provincial growth of the digital economy in terms of the availability of provincial-level data. Table 1 lists the six dimensions of indicators used to calculate the index, including digital infrastructure construction level, society digital level advanced by ICT, digital technology innovation capacity, economic growth promoted by ICT, development level of emerging digital economy industries, and capitalization level of digital economy enterprises.
The usage rate of telecommunication services and the penetration rate of mobile phone are typically used to describe digital infrastructure (Brandt and Thun 2011). Domains and websites are important measures of digital economic activities (Mossberger et al. 2022). The investment and output value related to ICT can partially characterize the role of the digital economy in stimulating economic growth. Because technologies such as ABCD (artificial intelligence, blockchain, cloud computing, and big data) are the digital technology innovations most concerned by the Chinese government and the market and have the potential to bring about a new productivity revolution, we utilize the quantity of patent applications for these four technologies as a measure of the capacity for innovation in digital technology. We choose e-commerce-related indicators to depict the emerging digital economy industry’s state of development since China’s emerging digital economy sector best represents the e-commerce industry. In addition, this study draws on the method of Mueller et al. (2017) for measuring the development level of the transnational digital economy, and introduces the indicator “capitalization level of digital economy enterprises” to observe the development of digital economy in different regions from the perspective of capital markets. With logarithmic processing performed on the composite index values formed by the six dimensions, we obtain the final index (Digital) to calculate the digital development level of each provincial-level economy.
Considering the possible obvious correlation between the indicators, this paper uses the CRITIC method to generate the index weights in the process of the synthesis of the second-level indicators into the first-level indicators and the final synthesis of the first-level indicators into the digital economic development level index. As an objective weight weighting method, CRITIC can not only effectively avoid the arbitrariness of subjective weighting, but also more comprehensively consider the variability and correlation of indicators than other objective weight weighting methods, and its weight design is more accurate (Alinezhad and Khalili 2019; Wichapa et al. 2021). Its formula is as follows:
where \(C_i = \sigma_i \sum_j^n {(}1 - \gamma_{ij} {),}i = 1,2{\rm{ \ldots }},n,i \ne j\), \(\sigma_i\) is the standard deviation of index \(i\), and \(\gamma_{ij}\) is the correlation coefficient between index \(i\) and index \(j\).
Compared with existing digital economy indices, the differentiations of index construction in this article are reflected in the two aspects: firstly, the dimensions of constructing the digital economy index are more abundant, and the selection of sub indicators is more extensive, especially it includes not only ICT related indicators, but also the latest digital cutting-edge patent technology indicators such as ABCD, as well as capital market indicators such as the market value of digital economy enterprises; the second is that the method of indicator synthesis is more reasonable, fully considering the objective existence of the correlations between relevant indices, and minimizing the bias caused by this correlations as much as possible. Compared with the existing indicators, the digital economy growth index constructed in this paper has expanded both in depth and breadth.
Finally, the data for the four first-level indicators, including digital infrastructure construction, society digital level advanced by ICT, economic growth promoted by ICT, and development level of emerging digital economy industries, are taken from the China Statistical Yearbook. For the data about the development level of the emerging digital economy industries, since the China Statistical Yearbook only discloses data for 2013 and later, the data for 2012 are calculated by comparing and collating the year-on-year growth data disclosed by the website of the China Electronic Commerce Center, iResearch’s e-commerce-related consulting reports, reports of the departments of commerce of different provinces in China, special reports of the statistics bureau of different provinces in China, and government work reports of different provinces in China. At the same time, data on the digital technology innovation capability comes from Zero One Think Tanks, a professional think tank of financial technology in China. The original data are obtained by crawling the international patent application data published on the official website of the World Intellectual Property Organization (WIPO) over the years. In addition, the data on the capitalization level of digital economy enterprises are obtained by summing up the year-end market capitalization of China’s A-share listed companies in industries related to information transmission, software, and information technology services in the Wind database by province. Based on the availability of the software business revenue data and e-commerce related data, the sample period selected in this study is 2012–2020.
4.3 Control variables
The literature has shown that IRETs is determined by various influencing factors. Hence, in a gesture to alleviate omitted variable bias, we account for some control variables in the model. Economic development represents the outside environment of IRETs, and it will influence IRETs by the available (Ren et al. 2021; Pan et al. 2022). This paper uses the natural logarithm of GDP per capita (PGDP) for measuring economic development. IRET's activities are based on the accumulation of existing technological knowledge (Hille et al. 2020; Zhang et al. 2022), education helps foster IRETs development through improving the ability to absorb technological knowledge and providing more technological talents. This paper employs the higher education level rate (Highedu) to measure the education level. Since market demand is the final goal of IRETs, higher renewable energy consumption will stimulate firms and governments to invest more in eco-innovation activities (Herman and Xiang 2019; Zhang et al. 2022). This paper utilizes of renewable energy consumption per person (Enerate) as the proxy for market demand. Besides, government fund support or environmental regulation may affect IRETs (Huang et al. 2012; Hille et al. 2020). This paper introduces Government's willingness to protect the environment as a control variable. Finally, urban environmental infrastructure will contribute to IRETs. Following Yan et al. (2022), this paper uses greenery coverage rate of urban region (Greenrate) as the proxy for urban environmental infrastructure.
Table 2 lists the details of variable definitions. In addition to the regional digital economy index and renewable energy patent data, the data used in this study are from the China Statistical Yearbook, the China Environment Yearbook, the China Energy Statistical Yearbook, provincial statistical yearbooks, and the statistical annual report published by the China Intellectual Property Office.
4.4 Descriptive statistics
In view of the completeness and availability of data, we selected data from 30 provinces (municipalities directly under the central government) in China, excepting Tibet, from 2012 to 2020 for empirical research. We winsorize the extreme values of 1% for all continuous variables to eliminate the deviation caused by abnormal values. The descriptive statistics of the main variables are shown in Table 3. From the perspective of the explained variables, the minimum value of \({\text{IRET}}\) is 0.3586, and the maximum value is 27.7509. When it comes to the core explanatory variable, the minimum value of \({\text{Digital}}\) is 2.1899, while the maximum value is 80.0720. The results indicate that there are significant regional imbalances in IRETs capacity and digital economy development level in China.
5 Empirical results
5.1 Panel causality tests
It is worried that whether there exists a bidirectional causal relationship between digital economy development and IRETs. The results from the bootstrap panel causality test, reported in Table 4, confirm the unidirectional causality running from digital economy development to IRETs effectively. Indeed, the hypothesis that Digital does not Granger-cause IRET is rejected only in Italy and Spain at the 1% level. However, we do not find any evidence that digital economy development is affected by IRETs.
5.2 Benchmark regression results
Table 5 reports the linear estimates of the impact of the digital economy development on IRETs. To ensure the accuracy of the estimated results, we use the stepping-regression method (Lin and Zhu 2019a, b) to investigate, and the results are reported in Table 4. As shown in columns (1–5), the coefficients of \({\text{Digital}}\) are all significantly negative at the 1% level. In addition, according to the linear regression result in column (5), the level of IRETs reduces by 0.1589 standard deviation (0.0592 × 13.2313/4.9281) for every one standard deviation increase in the regional digital economy development level. It indicates that digital economy development has inhibited IRETs. The predication of Hypothesis 1 is supported.
5.3 Robustness test and endogenous test
To check the robustness of the results in Sect. 5.2, this paper tests a series of different classifications of variables. Solar energy technologies are the main kinds of renewable energy technologies, and their installed capacities in China ranked first globally (Lin and Chen 2019). Therefore, the natural logarithm value of the number of patents of solar energy technology (\({\text{SPC}}\)) is taken as the explained variable to represent the level of IRETs. As shown in columns (1) of Table 6, the corresponding coefficients of \({\text{Digital}}\) is significantly negative at 5% level.
Considering the potential endogeneity between the digital economy development and IRETs, we delay the core explanatory variable by one period in column (3), and delay the core explanatory variable and all control variables by one period in column (4). The coefficients of the core explanatory variable (\({\text{Digital}}\)) remain significantly negative at the 1% confidence level.
In addition, we select appropriate instrumental variable to conduct two-stage least square estimation of the model. We use the weighted average of the digital economy development level in other provinces except the given province (\({\text{Ivdigital}}\)) as the instrumental variable of the digital economy development level in column (5). These results show that the instrumental variable satisfies the exogenousness that is unrelated to the disturbance item and the correlation requirements related to the endogenous explanatory variables, and passes the unidentifiable test and the weak instrumental variable test. Therefore, the instrumental variable selected in this paper is effective. After endogeneity treatment, the coefficient of the core explanatory variable remains significantly negative at the 1% confidence level.
5.4 Mediation mechanism analysis
At present, China is trialing a case-filing registration system, which is an important measure to protect the litigants’ rights. Therefore, the number of patent infringement cases can better represent the patent infringement problem in China. Referring to the Measures for Evaluation and Management of National Intellectual Property Pilot and Demonstration Cities (Urban Areas) issued by China Intellectual Property Office on June 13, 2014, we use a relative quantity indicator, “the number of patent infringement disputes (per 10,000 people),” to measure the patent infringement situation of each province (\({\text{Regist}}\)).
The regression results of the mediation test are reported in Table 7. The empirical results show that the coefficient of \({\text{Regist}}\) in column (1) is significantly positive at 1% level, and the coefficient of \({\text{Digital}}\) in column (2) is significantly negative at 1% level. In addition, the P value of the Sobel test is 0.02, again indicating the existence of mediation effects. The above empirical results show that digital economy development is accompanied by the increasingly serious problem of patent infringement, which seriously hampers the improvement of innovation in renewable energy technologies.
5.5 Moderation effect analysis
5.5.1 The effect of public environmental appeal
The public is the backbone of ecological governance. Referring to Dowdle (1997) and Liao and Shi (2018), this paper introduces the number of proposals for environmental protection in the provincial people's congress to characterize public environmental appeal, and processes it logarithmically as the moderating variable of the relationship between the digital economy development and IRETs.
In view of there remain major disputes among scholars on the impact of public environmental appeal on eco-innovation of enterprises. Therefore, we believe that it is necessary to clarify the direct impact of public environmental appeal on IRETs before studying its moderation effect. The coefficients of \({\text{Public}}\) coefficient is significantly positive at 1% or 5% level in Columns (1) and (2) of Table 7. The regression results show that the public environmental appeal represented by the number of proposals of environmental protection can effectively stimulate IRETs, which supports the previous research conclusions of Liao (2018).
To test the mediation effect of public environmental appeal, we set the following regression models:
The moderation effect is determined by the significance of regression coefficients \(\phi_2\). If \(\phi_2\) is significantly positive, the increasing public environmental appeal is expected to mitigate the negative impact of digital economy on IRETs. If \(\phi_2\) is significantly negative, the increasing public environmental appeal is expected to enhance the negative impact of digital economy on IRETs.
The results for the moderation effect are reported in column (3) of Table 8. The regression results show that the coefficient of cross-term (\({\text{Digital}} \times {\text{Public}}\)) is significantly negative at 5% level. This indicates that increasing public environmental appeal enhance the negative impact of the digital economy development on IRETs.
We believe this moderation effect exists for several reasons. First, the effectiveness of public environmental appeal may be delayed, enhancing the “free-riding” tendency of enterprises and enabling them to choose to reap the benefits of energy conservation and emissions reduction created by the digital economy. Second, the failure probability of eco-innovation activities is high, investment returns are slow, and large amounts of expenditure cannot be capitalized, which will have a serious adverse impact on the short-term performance of enterprises. Therefore, the amplification effect of public opinion accompanied by the attention of information intermediaries represented by the public environmental appeal will often aggravate the short-term performance pressure and career anxiety of internal managers. Under the multiple suppression of short-term performance, environmental performance, self-interest, and social public opinion, managers are easily induced to deviate from the purpose of maximizing corporate social value and safeguarding social public interest, and engage in short-sighted behavior, which may inhibit regional IRETs. Third, given the lag of the promulgation and adjustment of public policies and the delay of “the pressure transmission mechanism” (Liao et al. 2018), the incentive effect of new energy policies is delayed.
5.5.2 Further analysis of heterogeneity
The mediation role of patent infringement in the relationship between digital economy development and IRETs has been thoroughly discussed above. Patent judicial protection is the “good medicine” for patent disputes and infringement problems. To evaluate the effectiveness of patent judicial protection in China, we also investigate the profound impact of the heterogeneity of patent judicial protection intensity on the moderation effect of public environmental appeal. Scholars have long studied the measurement of intellectual property protection intensity. Representative indexes include the legislative index scoring method (Rapp and Rozek 1990), the GP index calculation method (Ginarte and Park 1997), and the questionnaire method (Mansfield 1995). However, the first two intensity calculation methods mentioned above primarily examine the strength of legislative protection (Maskus and Fink 2005; Ostergard 2000), and focus on the investigation of patent laws and regulations, while the questionnaire method has the limitations of strong subjectivity and weak dependability. Thus, none are suitable for the study of the effectiveness of patent judicial protection in China.
Judicial relief is an important and powerful means of intellectual property protection. However, the case-filing registration system has lowered the threshold of case-filing in Chinese courts, increasing the pressure on judges to adjudicate, resulting in the emergence of the problem of “too many cases and not enough judges.” Similarly, in the field of intellectual property protection, the situation of “it is not difficult to file a case but difficult to settle a case” makes timely judicial relief difficult for all patent cases. Given this, the data on settled patent disputes can be used as an important indicator of the status of patent judicial protection in China. After referring to the Measures for Assessment and Administration of National Intellectual Property Pilot and Demonstration Cities (Urban Areas), and consider the correlation between patent judicial protection and patent R&D activities, represented by the number of patent applications and grants (Bessen et al. 2018; Cohen et al. 2019; Mezzanotti 2021; Xu 2019), we introduce the relative quantity index of patent judicial protection intensity (\(JP\)) to measure the strength of patent judicial protection in different provinces of China.
Since patent disputes in China may be divided into infringement disputes and other disputes, we collected and collated the settlement data of patent infringement disputes (\({\text{SPID}}\)) and other patent disputes (\({\text{OPDS}}\)) in 30 provinces (excluding Tibet) of China. In addition, since the number of patents is the main indicator used to measure patent research and development activities in this paper, we standardized the settlement data of patent disputes by the number of patent applications (\({\text{PA}}\)) and the number of patent grants (\({\text{PG}}\)) to better characterize the strength of patent judicial protection (\({\text{JP}}\)). To ensure the rigor and accuracy of the evaluation results, this study also measures the strength of patent judicial protection in various ways: \({\text{JP}}_1 { = }\frac{{{\text{SPID}}}}{{{\text{PA}}}}\), \({\text{JP}}_2 { = }\frac{{{\text{SPID}}}}{{{\text{PG}}}}\),\({\text{JP}}_3 { = }\frac{{{\text{SPID}} + {\text{OPDS}}}}{{{\text{PG}}}}\). The higher the values of \({\text{JP}}_1\), \({\text{JP}}_2\) and \({\text{JP}}_3\), the stronger the judicial protection of patents. The provincial data on of patent disputes, patent applications, and patent authorizations are taken from the statistical annual report issued by the China Intellectual Property Office.
To test the heterogeneous regulation of different degrees of patent judicial protection, the average value (0.0047 for JP1, 0.0080 for JP2, and 0.0085 for JP3) and the median (0.0020 for JP1, 0.0036 for JP2, and 0.0037 for JP3) of the intensity of patent judicial protection are used as different threshold levels to group the samples.
The heterogeneity analysis results with the average value of patent judicial protection intensity as the threshold level are shown in Table 9. Among them, columns (1), (3), and (5) form a group that is greater than the average level of patent judicial protection, while columns (2), (4), and (6) form the group that is less than the average level of patent judicial protection. Table 9 shows that when the intensity of patent judicial protection is higher than the threshold level, the coefficients of \({\text{Digital}} \times {\text{Public}}\) are all significant positive at 1% or 10% level. However, when the intensity of patent judicial protection is lower than the threshold level, the coefficients of \({\text{Digital}} \times {\text{Public}}\) are all significantly negative at 5% level.
Table 10 reports the heterogeneity analysis results with the median level of the patent judicial protection intensity as the threshold level. In this case, columns (1), (3), and (5) form a group that is greater than the median level of patent judicial protection, while columns (2), (4), and (6) form the group that is less than the median level of patent judicial protection. And the empirical results are basically consistent with the findings in Table 9. In conclusion, the empirical results of the grouping regression above prove that the moderation effect of public environmental appeal on the relationship between digital economy development and IRETs may varies under different levels of the patent judicial protection intensity. The prediction of Hypothesis 2 is supported.
For the regions with low patent judicial protection intensity, there exists a negative moderation effect for the public environmental appeal. Coupled with the “free rider” tendency of environmental governance and the distorting effect of infringement issues, enterprises will prefer to “sit back and enjoy” the spillover effect of the eco-innovation in the digital era and thus reduce investment in eco-innovation or try its best to control the environmental pollution rather than investing in eco-innovation when they face the increasing public environmental appeal. This is bound to form a crowding out effect on enterprises’ eco-innovation activities, thus affecting the level of IRETs. For the regions with high patent judicial protection intensity, there exists a positive moderation effect for the public environmental appeal. When the degree of patent judicial protection is high, the more benefits of eco-innovation can be internalized, and the judicial punishment risk for free riding activities in eco-innovation is large. In this way, when facing increasing public environmental appeal, enterprises are more encouraged to make full use of the function of digital technology to enable eco-innovation and thus improve the level of eco-innovation. Therefore, the two-wheel driving phenomenon formed by the strong judicial guarantee and the increasing public environmental appeal can effectively break the “free rider” problem of eco-innovation, curb the prevalence of opportunism, and help eliminate the inhibiting effect of digital economy on IRETs.
6 Conclusions and policy implications
Based on Chinese provincial-level data from 2012 to 2020, this paper construct the regional digital economic development index by the CRITIC method and empirically finds an inhibiting effect of the digital economy development on IRETs. The conclusion holds for a variety of robust tests. A mediation mechanism test shows that the inhibiting effect may be due to free-rider problem in IRETs as patent infringements increase in the digital era. Further studies show that moderation effect of public environmental appeal on the relationship between digital economy development and IRETs depends on the level of patent judicial protection. It is found that increasing public environmental appeal will mitigate the negative impact of digital economy development on IRETs for regions with high level of patent judicial protection while enhance the above negative impact for regions with low level of patent judicial protection.
Based on the above findings, we recommend the following policy suggestions.
First, we should fully recognize the potential damage to IRETs brought by the economy’s digital transformation, and take targeted measures. As this paper points out, acts such as patent infringement will exist more widely in the era of digital economy, which brings new challenges to the enterprises’ innovation environment. Especially for the renewable energy innovation, it faces more serious externality problems. In the process of promoting the development of digital economy, the government must pay full attention to the above problems and further strengthen the direct policy intervention on the innovation level of regional IRETs.
Second, from the perspective of promoting IRETs, we should coordinately promote the improvement of public environmental awareness and the improvement of patent judicial guarantee system. As pointed out in this paper, the increasing public environmental appeal may increase the short-sighted behavior of enterprise departments in IRETs. Only by improving the level of patent judicial protection can enterprise sector really invest more resources in IRETs. the two-wheel driving phenomenon formed by the strong judicial guarantee and the increasing public environmental appeal can effectively break the free-rider problem of eco-innovation and help eliminate the inhibiting effect of digital economy on IRETs.
Notes
The research idea of exploring the development of digital economy based on the perspective of digital industrialization and industrial digitization has been expressed and recognized by China's top decision-making levels. First, Digital Economy Development in China (2017) issued by the China Academy of Information and Communications Technology (CAICT) (2017) proposed two paths for the development of the digital economy: digital industrialization and industrial digitization. Second, on March 5, 2021, Chinese Premier Li Keqiang indicated in the Report on the Work of the Chinese Government that the government would accelerate digital development, create new advantages for the digital economy, and promote digital industrialization and industrial digital transformation in a coordinated manner. Finally, on March 11, 2021, the Fourth Session of the 13th National People's Congress of the People's Republic of China approved the 14th Five-Year Plan (2021–2025) for National Economic and Social Development and the Long-Range Objectives Through the Year 2035 that emphasized “accelerating the promotion of digital industrialization” and “promoting the digital transformation of industries.”
References
Alinezhad A, Khalili J (2019) CRITIC Method. In: Alinezhad A, Khalili J (eds) New methods and applications in multiple attribute decision making (MADM), international series in operations research & management science. Springer International Publishing, Cham, pp 199–203
Amri F, Zaied YB, Lahouel BB (2019) ICT, total factor productivity, and carbon dioxide emissions in Tunisia. Technol Forecast Soc Change 146:212–217
Andrae AS, Edler T (2015) On global electricity usage of communication technology: trends to 2030. Challenges 6(1):117–157
Azam A, Rafiq M, Shafique M, Yuan J (2021) An empirical analysis of the non-linear effects of natural gas, nuclear energy, renewable energy and ICT-Trade in leading CO2 emitter countries: policy towards CO2 mitigation and economic sustainability. J Environ Manage 286:112232
Barefoot K, Curtis D, Jolliff W, Nicholson JR, Omohundro R (2018) Defining and measuring the digital economy. Presented at the US Department of Commerce Bureau of Economic Analysis, Washington, DC,15
Belkhir L, Elmeligi A (2018) Assessing ICT global emissions footprint: trends to 2040 & recommendations. J of Clean Prod 177:448–463
Ben Lahouel B, Taleb L, Ben Zaied Y, Managi S (2021) Does ICT change the relationship between total factor productivity and CO2 emissions? Evidence based on a nonlinear model. Energy Econ 101:105406
Bessen J, Neuhäusler P, Turner JL, Williams J (2018) Trends in private patent costs and rents for publicly-traded United States firms. Int Rev Law Econ 56:53–69
Brandt L, Thun E (2011) Going mobile in China: shifting value chains and upgrading in the mobile telecom sector. Int J Technol Learn Innov Dev 4(1–3):148–180
Bukht R, Heeks R (2017) Defining, conceptualising and measuring the digital economy. Dev Inform Working Pap 68
Cecere G, Corrocher N, Gossart C, Ozman M (2014) Technological pervasiveness and variety of innovators in Green ICT: a patent-based analysis. Rese Policy 43(10):1827–1839
Chen W (2023) Digital economy development, corporate social responsibility and low-carbon innovation. Corp Soc Responsib Environ Manag 30(4):1664–1679
Chen W, Wu Y (2022) Does intellectual property protection stimulate digital economy development? J App Econ 25(1):723–730
Chen Y (2020) Improving market performance in the digital economy. China Econ Revi 62:101482
China Academy of Information and Communications Technology (CAICT) (2017) Digital Economy Development in China
Cohen L, Gurun UG, Kominers SD (2019) Patent trolls: evidence from targeted firms. Manag Sci 65:5461–5486
De Marchi V (2012) Environmental innovation and R&D cooperation: empirical evidence from Spanish manufacturing firms. Rese Policy 41(3):614–623
DehghanShabani Z, Shahnazi R (2019) Energy consumption, carbon dioxide emissions, information and communications technology, and gross domestic product in Iranian economic sectors: a panel causality analysis. Energy 169:1064–1078
Diwan I, Rodrik D (1991) Patents, appropriate technology, and North-South trade. J Int Econ 30(1–2):27–47
Domazet I, Zubović J, Lazić M (2018) Driving factors of Serbian competitiveness: digital economy and ICT. Strateg Manage 23(1):20–28
Dowdle MW (1997) The constitutional development and operations of the National People’s Congress. C J Asian Law 11:1
Du Y, Li Z, Du J, Li N, Yan B (2019) Public environmental appeal and innovation of heavy-polluting enterprises. J Clean Prod 222:1009–1022
García-Herrero A, Xu J (2018) How Big is China’s Digital Economy? (SSRN Scholarly Paper No. ID 3237275). Social Science Research Network, Rochester, NY
Ginarte JC, Park WG (1997) Determinants of patent rights: a cross-national study. Res Policy 26:283–301
Govindaraju V, Tang CF (2013) The dynamic links between CO2 emissions, economic growth and coal consumption in China and India. Appl Energy 104:310–318
Hao Y, Zhang Z-Y, Liao H, Wei Y-M (2015) China’s farewell to coal: a forecast of coal consumption through 2020. Energy Policy 86:444–455
He Y, Li K, Wang Y (2022) Crossing the digital divide: the impact of the digital economy on elderly individuals’ consumption upgrade in China. Technol Soc 71:102141
Herman KS, Xiang J (2019) Induced innovation in clean energy technologies from foreign environmental policy stringency? Technol Forecast Soc Change 147:198–207
Higón DA, Gholami R, Shirazi F (2017) ICT and environmental sustainability: a global perspective. Telematics Inform 34(4):85–95
Hille E, Althammer W, Diederich H (2020) Environmental regulation and innovation in renewable energy technologies: Does the policy instrument matter? Technol Forecast Soc Chang 153:119921
Hojnik J, Ruzzier M (2016) The driving forces of process eco-innovation and its impact on performance: insights from Slovenia. J Clean Prod 133:812–825
Huang C, Su J, Zhao X, Sui J, Ru P, Zhang H, Wang X (2012) Government funded renewable energy innovation in China. Energy Policy 51:121–127
Jensen MC, Meckling WH (1976) Theory of the firm: managerial behavior, agency costs and ownership structure. J Financ Econ 3:305–360
Johnstone N, Haščič I (2010) Directing Technological Change while Reducing the Risk of (not) Picking Winners: the case of renewable energy (OECD working paper) 30
Kemp R, Oltra V (2011) Research insights and challenges on eco-innovation dynamics. Indus Innova 18(3):249–253
Kim D, Kim N, Kim W (2018) The effect of patent protection on firms’ market value: the case of the renewable energy sector. Renew Sustain Energy Rev 82:4309–4319
Lee CC, He ZW, Xiao F (2022) How does information and communication technology affect renewable energy technology innovation? Int Evid Renew Energy 200:546–557
Lee JW, Brahmasrene T (2014) ICT, CO2 emissions and economic growth: evidence from a panel of ASEAN. Global Econ Revi 43(2):93–109
Lemley MA (2004) Property, intellectual property, and free riding. Tex l Rev 83:1031–1076
Li Q, Liu G, Cai B, Leamon G, Liu L-C, Chen Z-A, Li X (2017) Public awareness of the environmental impact and management of carbon dioxide capture, utilization and storage technology: the views of educated people in China. Clean Technol Environ Policy 19:2041–2056
Liao X, Shi X (Roc) (2018) Public appeal, environmental regulation and green investment: evidence from China. Energy Policy 119:554–562
Liao Z (2018) Environmental policy instruments, environmental innovation and the reputation of enterprises. J Clean Prod 171:1111–1117
Liao Z, Weng C, Shen C (2020) Can public surveillance promote corporate environmental innovation? The mediating role of environmental law enforcement. Sustain Deve 28(6):1519–1527
Lin B, Chen Y (2019) Does electricity price matter for innovation in renewable energy technologies in China? Energy Econ 78:259–266
Lin B, Zhu J (2019a) Determinants of renewable energy technological innovation in China under CO2 emissions constraint. J Environ Manag 247:662–671
Lin B, Zhu J (2019b) The role of renewable energy technological innovation on climate change: empirical evidence from China. Sci Total Environ 659:1505–1512
Litvinenko VS (2020) Digital economy as a factor in the technological development of the mineral sector. Nat Resour Res 29:1521–1541
Mansfield E (1995) Intellectual property protection, direct investment, and technology transfer: Germany, Japan, and the United States. World Bank Publications
Maskus KE, Fink C (2005) Intellectual Property and Development: lessons from recent economic research. World Bank and Oxford University Press, Washington, DC
Mezzanotti F (2021) Roadblock to innovation: the role of patent litigation in corporate R&D. Manag Sci 67(12):7362–7390
Moroz M (2017) The level of development of the digital economy in Poland and selected European countries: a comparative analysis. Found Manag 9:175–190
Mossberger K, LaCombe S, Tolbert CJ (2022) A new measure of digital economic activity and its impact on local opportunity. Telecomm Policy 46(1):102231
Mueller SC, Bakhirev A, Böhm M, Schröer M, Krcmar H, Welpe IM (2017) Measuring and mapping the emergence of the digital economy: a comparison of the market capitalization in selected countries. Digit Policy Regul Gov 19:367–382
Murshed M, Chadni MH, Ferdaus J (2020) Does ICT trade facilitate renewable energy transition and environmental sustainability? Evidence from Bangladesh, India, Pakistan, Sri Lanka, Nepal and Maldives. Energy Ecol Environ 5:470–495
Noailly J, Shestalova V (2017) Knowledge spillovers from renewable energy technologies: lessons from patent citations. Environ Innov Soc Transit 22:1–14
Organisation for Economic Co-operation and Development (2014) Measuring the digital economy: a new perspective. OECD Publishing
Ostergard RL (2000) The measurement of intellectual property rights protection. J Int Bus Stud 31:349–360
Pan W, Xie T, Wang Z, Ma L (2022) Digital economy: an innovation driver for total factor productivity. J Bus Res 139:303–311
Park Y, Meng F, Baloch MA (2018) The effect of ICT, financial development, growth, and trade openness on {\rm CO}_2 emissions: an empirical analysis. Environ Sci Pollut Res 25:30708–30719
Pitelis A, Vasilakos N, Chalvatzis K (2020) Fostering innovation in renewable energy technologies: choice of policy instruments and effectiveness. Renew Energy 151:1163–1172
Preacher KJ, Kelley K (2011) Effect size measures for mediation models: quantitative strategies for communicating indirect effects. Psycho Meth 16(2):93
Rapp RT, Rozek RP (1990) Benefits and costs of intellectual property protection in developing countries. J World Trade 24(5):75–102
Ren S, Hao Y, Wu H (2021) Government corruption, market segmentation and renewable energy technology innovation: evidence from China. J Environ Manage 300:113686
Teece DJ (2018) Profiting from innovation in the digital economy: enabling technologies, standards, and licensing models in the wireless world. Res Policy 47(8):1367–1387
Wichapa N, Khokhajaikiat P, Chaiphet K (2021) Aggregating the results of benevolent and aggressive models by the CRITIC method for ranking of decision-making units: a case study on seven biomass fuel briquettes generated from agricultural waste. Decis Sci Lett 10:79–92
Xu M (2019) How Does Patent Litigation Affect New Venture Funding? In: Academy of Management Proceedings (Vol. 2019, No. 1, p. 19480). Briarcliff Manor, NY 10510: Academy of Management
Yan C, Di D, Li G, Wang J (2022) Environmental regulation and the supply efficiency of environmental public services: evidence from environmental decentralization of 289 cities in China. Growth Change 53(2):515–535
Zhang D, Zheng M, Feng GF, Chang CP (2022) Does an environmental policy bring to green innovation in renewable energy? Renew Energy 195:1113–1124
Acknowledgements
I acknowledge the financial support of the National Natural Science Foundation of China (Grant No. 72003157).
Author information
Authors and Affiliations
Corresponding author
Ethics declarations
Conflict of interest
No potential conflict of interest was reported by the authors.
Additional information
Publisher's Note
Springer Nature remains neutral with regard to jurisdictional claims in published maps and institutional affiliations.
Appendix
Appendix
IPC codes of each kind of renewable energy technologies.
Technology | IPC classes |
---|---|
Wind power | F03D |
Solar energy | F03G6; F24J2; F26B3/28; H01L27/142; H01L31/042–058 |
Marine (ocean) energy | E02B9/08; F03B13/10–26; F03G7/05 |
Hydro power | E02B9 and not E02B9/08; [F03B3 or F03B7 or F03B13/06–08 or F03B15] and not F03B13/10–26 |
Biomass energy | C10L5/42–44; F02B43/08 |
Storage | H01M10/06–18; H01M10/24–32; H01M10/34; H01M10/36–40 |
Rights and permissions
Springer Nature or its licensor (e.g. a society or other partner) holds exclusive rights to this article under a publishing agreement with the author(s) or other rightsholder(s); author self-archiving of the accepted manuscript version of this article is solely governed by the terms of such publishing agreement and applicable law.
About this article
Cite this article
Chen, W. The impact of digital economy development on innovation in renewable energy technologies. Econ Change Restruct 56, 4285–4308 (2023). https://doi.org/10.1007/s10644-023-09553-1
Received:
Accepted:
Published:
Issue Date:
DOI: https://doi.org/10.1007/s10644-023-09553-1