Abstract
Natural disasters have caused over a million of deaths and $3 trillion in economic losses during the last 20 years. However, theoretical and empirical studies have not reached a conclusion as for their effect on economic growth, and the results can best be described as mixed. The present study proposes the use of a quantile on quantile (QQ) approach to shed more light on this complex relationship. This approach combines the standard quantile regression analysis with nonparametric estimations and allows us to examine how different quantiles of natural disasters affect different quantiles of GDP growth. Using data from over 100 countries over a 30-years period, we confirm that the results of the QQ approach differ from the ones obtained by standard approaches like fixed effects regressions. We document that the relationship between the intensity of natural disasters and economic growth is mostly negative. Nonetheless, there are some exceptions to this. Our findings reveal that the effect of natural disasters can be occasionally positive, depending on the quantiles that we examine. The magnitude of the effect also differs across different combinations of the quantile of economic growth and the quantile of natural disasters. Finally, we obtain somewhat different results when we estimate separate QQ regressions for groups of countries that differ in terms of climate, economic and democratic development, and across different year lags of the natural disaster index.
Similar content being viewed by others
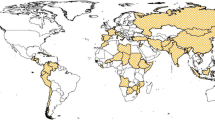
Avoid common mistakes on your manuscript.
1 Introduction
Large scale disasters regularly hit both poor and rich countries around the globe, causing huge destruction and damage. A recent global report by the United Nations Office for Disaster Risk Reduction and the Centre for Research on the Epidemiology of Disasters, reveals that between 1998 and 2017 climate-related and geophysical disasters killed 1.3 million people and left another 4.4 billion injured, homeless, displaced or in need of emergency assistance (Wallemacq and House 2018). Over the same period the disaster-hit countries reported direct economic losses valued at US$ 2908 billion.Footnote 1
Given these losses in human capital and in monetary terms, a question that naturally emerges is: How do natural disasters influence economic growth? While at first one would expect that the impact will always be negative, some argue that disasters can be associated with higher rates of human capital accumulation, updated capital stock, and adoption of new technologies which lead to improved total factor productivity, and subsequently economic growth (Skidmore and Toya 2002). Therefore, a growing number of empirical studies has attempted to answer this question. However, using standard tools of growth empirics the literature does not offer conclusive answers (Felbermayr and Gröschl 2014). In other words, the results are contradictory, ranging from positive to very large negative effects (Bakkensen and Barragey 2016).Footnote 2 To make things more complicated, some studies find mixed results that depend upon the choice of the database. For example, using a newly constructed database (GeoMet), Felbermayr and Gröschl (2014) find that disasters have a negative effect on growth. However, when they re-estimate their specifications with the use of data from the well-known Emergency Events Database (EM-DAT) database, this impact is no longer statistically significant.
In the light of these controversial findings, we propose to study how the quantiles of a natural disaster index could affect the conditional quantile of economic growth via the quantile-on-quantile (QQ) regression framework and compare our results with the ones of fixed effects regressions, commonly used in past studies.
Our approach is motivated by recent work on economic growth, which uses the standard quantile regression analysis (QRA) by Koenker and Bassett (1978) to examine whether the effect of a given country-specific attribute on economic growth is heterogeneous along quantiles of the conditional growth distribution. However, to the best of our knowledge, none of these studies has considered the impact of national disasters on economic growth.Footnote 3 As discussed in Andini and Andini (2014), the underlying idea for the use of QRA is that the impact of a given attribute on the mean of the conditional growth distribution, can be driven, at least in principle, by something that is happening at specific quantiles of the conditional growth distribution, i.e. something which is not highlighted by mean-regression techniques. Additionally, Andini and Andini (2014) highlight that the issue of quantile parameter heterogeneity is something worth studying even in the presence of homogeneity, serving at the very least as proof of the robustness of mean-regression results.
While the standard QRA used in the above-mentioned studies allows one to examine different quantiles of GDP growth, it does not offer insights on what happens across the entire distributions of both the dependent variable (i.e. GDP growth) and the key variable of interest (i.e. natural disaster). This can be particularly important in our context, since the results may also differ across different quantiles of the natural disaster. For example, Hochrainer (2009) mentions that The negative effects may be small, yet they can become more pronounced depending on the size of the shock (p. 2). Fomby et al. (2013) also argue that Under some conditions, moderate disasters may even bring about an increase in economic growth by, for instance, raising land productivity or inducing capital transformation. However, if the disaster is of such magnitude that it overwhelms public and private responses, its effect is likely to be more detrimental (p. 413).
One way to address this issue is to use a QQ approach, as recently proposed Sim and Zhou (2015). This approach combines the techniques of QRA and nonparametric estimation and it has been used in recent studies in economics and finance to examine various issues (Sim and Zhou 2015; Bouri et al. 2017; Gupta et al. 2018; Shahzad et al. 2019; Mallick et al. 2019).Footnote 4 While two recent studies focus on economic growth (Shahzad et al. 2017; Shahbaz et al. 2018), none of them considers natural disasters.Footnote 5
Thus, there appears to be no study using either a standard QRA or a QQ approach to investigate the relationship between natural disasters and economic growth and we aim to address this gap in the literature. The main rationale for the use of QQ in our context is it allows to better explore this complex relationship between natural disasters and economic growth under various combinations of economic conditions and levels of natural disaster (i.e. small, moderate, large). In other words, the QQ approach allows us to relate the quantile of GDP growth with the quantile of a natural disaster index, so that the relationship between national disasters and GDP growth could vary at different points in their respective distributions. Therefore, it provides a more complete picture of dependence than either the standard QRA or OLS based approaches.
We use the dataset of Felbermayr and Gröschl (2014) which gives us the opportunity to consider the impact of national disasters on economic growth over a 30 years period in around 100 countries, both developed and developing ones. Using their dataset has one more advantage. As discussed earlier, Felbermayr and Gröschl (2014) find different results when they use the GeoMet database and the EM-DAT one. We therefore apply the QQ approach using data from both the GeoMet and the EM-DAT. Our results show that natural disaster has generally a distinguishing effect on the distribution of GDP growth, suggesting that the use of a conditional mean approach gives a partial picture on the role of disaster as a determinant of economic growth, which might lead to incorrect inferences.
The next of the paper is as follows. Section 2 describes the methodology and data. Section 3 discusses the empirical results. Section 4 concludes the study.
2 Data and methodology
All the data that we use in the present study are obtained from Felbermayr and Gröschl (2014).Footnote 6 In the discussion that follows, we first discuss the QQ approach. Then, as we outline our specification for panel data analysis, we present the indices of natural disasters, along with the control variables used in the analysis. The dataset includes 108 countries for the period 1979–2010, but with gaps in time coverage.
As discussed in Koenker (2014): (i) quantile regression offers an extension of univariate quantile estimation to the estimation of conditional quantile functions via an optimization of a piecewise linear objective function in the residuals, (ii) computation of quantile regression estimators may be formulated as a linear programming problem and efficiently solved by simplex or barrier methods. The main advantage of QRA compared to classical linear or even non-linear regression methods, is that it provides a more precise and accurate analysis of the stochastic relationships among random variables (Koenker 2014; Bouri et al. 2017), which is achieved by estimating an entire set of conditional quantile functions in addition to the estimation of conditional mean function (Koenker 2014). However, the shortcoming of the QRA is its inability to capture dependence in its entirety (Sim and Zhou 2015; Bouri et al. 2017; Shahzad et al. 2017), since it neglects, in our context, the possibility that the nature of the disaster (i.e. small, moderate, large) could also influence the way natural disasters and economic growth are related.
Modeling this type of dependence requires a QQ approach, like the one proposed by Sim and Zhou (2015). Their framework, in our context, works as follows. First, it models the quantile of GDP growth as the dependent variable, since it is informative about how well or poorly the economy is performing. Second, it models the quantile of the natural disaster as an explanatory variable as this quantile contains information about the magnitude of the disaster. This is achieved by selecting a number of quantiles of the natural disasters index (indexed by D) and estimating the local effect that the θth-quantile of natural disasters might have on the τth-quantile of the GDP growth.
The QQ specification used in this study to investigate the effect of the quantiles of national disasters on the quantiles of GDP growth is given as follows.
Let \( y_{i} \) be the response variable, where i (i = 1, 2, …, M) denotes the country level, conditionally on a q × 1 vector of random effect \( u_{i} \), independently distributed to an unknown distribution \( F_{{y_{i} |u_{i} }} \).. The τ-th linear quantile mixed model (LQMM) is given by:
with independent and identically distributed errors, i.e. where \( \mu^{(\tau )} = \left( {\mu_{1}^{(\tau )} , \ldots ,\mu_{M}^{(\tau )} } \right)^{T} {\text{and}} \tau \). being the skewness parameter, set a priori, defining the quantile level to be estimated.ollowing Geraci and Bottai (2014), Eq. (1) can be compactly written in a matrix for such as:
where \( \beta_{x}^{()} \in {\mathbb{R}}^{P} \). is a vector of unknown fixed effects. It is also worth mentioning that \( u_{i} = \left( {u_{i1} , \ldots ,u_{id} } \right)^{T} \), for i = 1, …, M, is assumed to be a zero-median random vector independent from the model’s error term, i.e. \( u \bot \varepsilon \).. Moreover, although \( F_{{y_{i} |u_{i} }} \). is assumed unknown, the LQMM based \( \tau \)-th quantile of the conditional distribution is estimated as the location parameter \( \mu^{(\tau )} \) given by Eq. (2) of an AL (Asymmetric Laplace) distribution with scale \( \sigma \left( \tau \right) \) and skewness \( \tau \).
As with Sim and Zhou (2015), we examine the relationship between the θ-quantile of the independent variable and the τ-quantile of the dependent variable.Footnote 7 Hence, letting \( \theta \) denoting the quantile of a chosen measure of disaster intensity \( D_{i}^{\left( \theta \right)} \)., and after the linearization of the unknown function \( \beta^{\tau } \left( {D_{i}^{\left( \theta \right)} } \right) \) by taking the first order Taylor expansion around the quantile of the chosen measure of disaster intensity, let us redefine \( \beta^{{\tau {\prime }}} \left( {D_{i}^{\left( \theta \right)} } \right) \), \( \beta^{\tau } \left( {D_{i}^{\left( \theta \right)} } \right) \) and \( \beta_{i}^{\left( \tau \right)} \) as \( \beta_{0} \left( {\tau , \theta } \right) \). and \( \beta_{2} \left( {\tau , \theta } \right) \), and \( \beta_{i} \left( \tau \right) \) respectively. In this context, the specification of our Quantile-on-Quantile Mixed Effect Model (QQMM) can be written as:
where \( \Delta lny_{i,t} \). is the growth rate of real GDP per capita, \( lny_{i,t - 1} \). is the lagged log of GDP per capit,Footnote 8\( CV_{{l,t\text{ - }1}} \) are lagged Control Variables, and \( \hat{D}_{i,t} = D_{i,t} - D_{i}^{\left( \theta \right)} \), with \( D_{i,t} \) denoting a measure of disaster intensity, and \( D_{i}^{(\theta )} \) representing its θth-quantile.Footnote 9 Given that \( \beta_{0 } \) and \( \beta_{1 } \) are doubly indexed in \( \tau \) and \( \theta \), Eq. (3) captures the relationship between the θ-th quantile of the disaster intensity and τ-th quantile of the GDP growth.
As clearly mentioned in Geraci and Bottai (2014), embracing the assumption of normality for random effects, where \( u \sim N \left( {0;\Psi } \right) \), will directly result into a Gauss–Hermite quadrature for the approximate AL- based log-likelihood
where \( \nu_{{k_{1} , \ldots ,k_{q} }} = \left( {\nu_{{k_{1} }} , \ldots , \nu_{{k_{q} }} } \right)^{T} \). are the nodes and \( w_{{k_{l} }} , l = 1, \ldots ,q, \) as kernel function based weights respectively.
Various types of disasters happening in a country during a year are included in Eq. (3). The dataset that Felbermayr and Gröschl (2014) make available includes information from the EM- DAT that is the most commonly used one in the literature. This database is provided by the Centre for Research on the Epidemiology of Disasters (CRED) at Université Catholique de Louvain, and it is compiled from various sources. However, Felbermayr and Gröschl (2014) argue that one of the drawbacks of EM-DAT is that disaster intensity measures are a function of economic development which can be problematic when estimating causal relations between disasters and economic variables. To tackle this issue, Felbermayr and Gröschl (2014) constructed and proposed a novel and comprehensive database of national disasters, called GeoMet, which contains exogenous measures of physical disaster intensity, such as Richter scale and wind speed. They highlight that Virtually all earthquakes, volcanic eruptions, storms, floods, droughts, and extreme temperature events reported by EM-DAT can be also found in GeoMet, too, but the opposite is not true: Out of 10,448 earthquakes with strength at least 5 on the Richter scale, EMDAT reports only 6.2%. That rate is highest for the strongest earthquakes (40.9%), but falls quickly with decreasing earthquake intensity. Volcano eruptions are better covered, with 14.7% of all events with VEI measure higher than 2 being included into EM-DAT and half of all events stronger than VEI 4 covered (p. 95).
Following Felbermayr and Gröschl (2014), we use four versions of the EM-DAT and GeoMet indices.Footnote 10 The first index, “EMDAT all disaster” (EMALL), is the sum of all disasters in the EM-DAT database, normalized by the land area. The second index, “EMDAT Large Disaster” (EMLAR), includes only Large-scale disasters normalized by land area.Footnote 11 The third index, is the “GeoMet Disaster Index” (GEOLAND), defined as the unweighted sum of physical intensity measures of disasters that happened in a specific country. In the case of the fourth index, the “GeoMet Disaster Index weighted” (GEOSDL), the physical intensity measures of different disaster types have been weighted with their inverse sample standard deviations. As discussed in Felbermayr and Gröschl (2014), this approach ensures that no single disaster component dominates the movement of the disaster index. In both cases, the indices are scaled by the log of the land area in each country to account for size effects. In general, the units of observation are country-year pairs with higher values indicating a higher number of aggregated events in a single year in a given country.
As in Felbermayr and Gröschl (2014) and following the related literature (Noy 2009; Skidmore and Toya 2002; Loayza et al. 2012), the control vector \( CV_{{i,t\text{ - }1}} \) is constituted of the following variables: democratization (POLITY), openness to trade (OPEN), real interest rates (INTEREST), domestic credit (CREDIT), gross capital formation (CAPITAL), foreign direct investment (FDI), inflation (CPI), and current account balance (BALANCE). Further information about these variables is available in “Appendix”. To reduce potential endogeneity problems, the above control variables enter Eq. (3) with their lagged values. \( \nu_{i} \) denotes the country-specific fixed effects, whereas year fixed effects are captured by \( \nu_{t} \).
Table 1 presents descriptive statistics of the variables used in the analysis. The growth rate of real GDP per capita ranges from − 0.624 (Rwanda in 1994) to 0.392 (Azerbaijan in 2005) with an average value of 0.049 and a standard deviation of 0.058. The EM-DAT all disasters index that considers all the disasters in the EM-Data database while being normalized by land area (EMALL) ranges between 0.000 (e.g. Australia, Brazil) and 0.039 (Trinidad and Tobago) with an average of 0.001. The corresponding figures for the large disasters index from the same database are 0.000 (min), 0.014 (max) and 0.000 (average). Turning to the GeoMet indices, both have an average of 0.041, and we observe minor differences in the standard deviation (0.203 in the case of GEOLAND, and 0.212 in the case of GEOSDL) and the range of their values.Footnote 12
One may worry about panel unit roots. Given the unbalanced nature of our dataset we conduct Fisher-type unit root tests using the Phillips–Perron test and the Augmented Dickey Fuller test.Footnote 13 Both tests strongly reject the null hypothesis that all the panels contain unit roots.Footnote 14
3 Empirical results
3.1 Baseline results
This section reports the estimation results of the relationship between natural disasters and economic growth. For illustration purposes, Table 2 presents a country fixed effects model with clustered robust standard errors without time dummies (columns 1 to 4) and with time dummies (columns 5 to 8). Thus, the estimations in columns 5 to 8 are similar to the ones in Felbermayr and Gröschl (2014).Footnote 15 In each case, we present the results for all four indices of natural disasters. All these models include a constant and the following control variables (lagged by 1 year) which are not shown to conserve space: LCGDP, POLITY, OPEN, INTEREST, CAPITAL, FDI, CPI, BALANCE.
Consistent with Felbermayr and Gröschl (2014), the two indicators that are based on EM-DAT (i.e. EMALL and EMLAR) are insignificant in most of the cases. The only exception is the model with the country-fixed effects without time dummies in which case, EMALL enters the regression with a positive and statistically significant coefficient (column 3). Additionally, as in Felbermayr and Gröschl (2014) the coefficient of GEOSDL is negative and statistically significant in these fixed effects regressions.
We now turn to the QQ approach to shed further light on the relationship between natural disasters and economic growth. Consistent with past studies, for illustration purposes we present these results in Figures rather than in Tables (e.g. Shahzad et al. 2017; Shahbaz et al. 2018). Therefore, Fig. 1 (EM-DAT) and Fig. 2 (GeoMet) display the estimates of the slope coefficient that captures the effect of the θ-th quantile of the natural disasters and the τ-th quantile of GDP growth, at different values of θ and τ.Footnote 16
Two interesting findings emerge from these results. First, the relation between the intensity of natural disasters and economic growth is mostly negative. However, there are also some exceptions to this. According to these QQ estimates, the effect of natural disasters on economic growth is statistically significant in the case of all four indices, contradicting the results in Table 2. Second, there are sizeable variations of the slope coefficient across different combinations of the quantiles of the two variables of interest. Thus, the association between natural disasters and economic growth is not uniform across quantiles, and the relationship depends on both the size of the natural disaster shock and the specific phase of the economic cycle that a country is experiencing. In the discussion that follows, we discuss the findings for each natural disaster index, in turn.
In the case of both EM-DAT indices, the impact of natural disasters on economic growth is mostly negative. However, we observe that the sign of the coefficient is reversed (i.e. becomes positive) in the case of the lowest quantiles of GDP growth (0.05–0.15). Common to both indices is the variation across different combinations of the θ-quantile and the τ-quantile. For example, in the case of EMALL, the coefficient in the area that combines the 95th quantile of GDP growth and the 5th quantile of EMALL equals − 0.223 (statistically significant at the 1% level) which increases to − 0.727 when we turn our attention to the combination of the upper quantiles of GDP growth (0.95) and the upper quantiles of EMALL (0.95). The corresponding figures in the case of EMLAR are much higher, being − 3.983 and − 5.052 (in both cases statistically significant at the 1% level), respectively. The higher effect of EMLAR is not surprising since this index tracks only large natural disasters which are expected to have a greater impact on economic growth.
Turning our attention to the GeoMet indices, we find that in the case of GEOLAND, the results are quite mixed. In more detail, for intermediate to upper quantiles of GDP growth (0.45–0.85), the impact of natural disasters on economic growth is positive across the entire range of the quantiles of the disaster index. Within this range of GEOLAND, there are only a few cases where the coefficient is either insignificant (e.g. combination of 0.45 quantile of GDP growth and 0.35 quantile of GEOLAND) or negative (combination of 0.55 quantile of GDP growth and 0.85 quantile of GEOLAND). In contrast, the impact is always negative in the case of the 15th, 35th and 95th quantiles of economic growth.
In the case of GEOSDL, which accounts for the standard deviation of disaster types, we observe that the impact of natural disasters on economic growth is negative. The only exception is the intermediate quantile (0.55) of economic growth, in which case the relationship is, in general, either positive or insignificant across the different quantiles of the disaster index. Having said that, there is a lot of variation on the magnitude of the impact across different combinations of the θ-quantile and the τ-quantile. For example, at the 5th quantile of GDP growth and the 5th quantile of GEOSDL the coefficient is − 0.034 (statistically significant at the 1% level), which increases to − 0.093 (statistically significant at the 1% level) at the 95th quantile of GDP growth and the 95th quantile of GEOSDL.
3.2 Further analysis
In this section we extend our analysis towards two dimensions. First, we examine whether and how the relationship between natural disasters and economic growth differs across countries with different levels of economic and democratic development. We present this analysis in Sect. 3.2.1. Second, consistent with most of the existing studies, the analysis so far focused on the immediate impact of the natural disasters occurring in time t on the GDP growth from t − 1 to t. In the analysis outlined in Sect. 3.2.2, we extend our analysis to the short to medium term, using lags of the natural disaster for up to 5 years.
In the analysis presented in Sects. 3.2.1 and 3.2.2, we focus on the GEOSDL index. There are two reasons for this. First, as discussed earlier, the GeoMet indices have certain advantages over the EM-DAT ones in terms of both coverage and definition. Second, as discussed earlier the GEOSDL index is adjusted not only for the size of a country but also for the standard deviation of a disaster type within a country over all years.
3.2.1 Heterogeneity across country groups
Various studies report that the results differ across different levels of economic and democratic development. For example, Noy (2009) concludes that: (i) developing countries face much larger shock to their macro-economies following a disaster of similar relative magnitude than developed ones, and (ii) small economies (defined as the ones below the median size of the economy measured in constant US$) also seem to be more vulnerable than larger ones. Similarly, Raddatz (2009) finds that in the case of low-income countries, the climatic disasters result in a cumulative per-capita output loss of about 1%, whereas the corresponding figure for middle and high-income countries, is 0.5% and 0.25% respectively. The results in Felbermayr and Gröschl (2014) are rather mixed. When they use the “disaster index, weighted” (i.e. GEOSDL) they find that the impact is statistically significant in the non-OECD, high income and autocratic countries, and insignificant in the remaining cases.Footnote 17
Following Felbermayr and Gröschl (2014), in Tables 3, 4 and 5 we present the results when we run separate QQ regressions for: (i) OECD versus non-OECD countries in Table 3, (ii) low-middle income countries versus high income countries in Table 4, and (iii) democratic countries (polity index above 0.5) versus autocracies (polity index below 0.5) in Table 5.Footnote 18 Additionally, we present results for tropical and non-tropical countries (Table 6).Footnote 19
As discussed above, Felbermayr and Gröschl (2014) report that the impact of GEOSDL is negative and statistically significant for the group of non-OECD countries and insignificant in the case of OECD countries. In unreported regressions with a model similar to the one of their study we confirm this finding.Footnote 20 However, the results in Table 3 provide a somewhat different picture. While we confirm the negative impact of GEOSDL on GDP growth in the case of non-OECD countries we also find that there are a few cases at the lowest quantile of GDP growth (0.05) where the impact of the natural disasters index is positive. Most importantly, in the case of the OECD countries we find that: (i) the impact of natural disasters is always positive and statistically significant when focusing on the lowest quantile of GDP growth (0.05), and (ii) the relationship is positive or insignificant in several combinations of low to intermediate quantiles of GDP growth (0.05–0.45) and intermediate to upper quantiles of GEOSDL (0.45–0.95). One potential explanation for these findings is that among the OECD member countries that experience the lowest GDP change are countries like Canada, Germany, Japan, Italy, and UK the US. Due to their overall development status and size, such countries usually have the means to rebuild the destroyed housing and social infrastructure and increase government spending that boosts the economy. Furthermore, large countries have the ability to enact inter-sectoral or inter-regional transfers that can play a role in mitigating the economic impact of natural disasters (Duggar et al. 2016). Finally, another point being made in Duggar et al. (2016) is that while direct losses might be higher in large countries due to the exposure of assets, the greater capacity to absorb shocks eventually results in lower indirect losses and the size of the damage being lower relative to the size of the economy.
The results in Table 4 show that in the case of high-income countries, the coefficient of the effect of natural disasters on economic growth ranges from − 0.238 (0.15 quantile of GDP growth and 0.55 quantile of GEOSDL) to 0.101 (0.95 quantile of GDP growth and 0.75 quantile of GEOSDL). Overall, while there appears to be a negative relationship in the low to intermediate quantiles of GDP growth (0.05–0.45), we also detect a positive relationship in the upper quantiles of GDP growth (0.75–0.95). This latter finding is intuitive given that in rich countries experiencing high economic growth and hit by natural disaster, industries are more likely to fast replace their damaged machinery to resume normal production because they are more confident of a quick economic rebound than in the case of rich countries experiencing low growth.Footnote 21 This partially contradicts the results of Felbermayr and Gröschl (2014) which showed a mean-based association between natural disaster and economic growth; instead, our results based on the QQ approach allowed us to reveal more nuanced association that seems to differ according to both the intensity of the natural disaster and the level of economic growth.
Turning to the group of the low-middle income countries we find a negative association between natural disaster and economic growth, which is statistically significant across more combinations of the quantiles of GEOSDL and GDP growth. Again, this contradicts the findings of Felbermayr and Gröschl (2014) who report an insignificant coefficient in the case of the low-middle income countries. We also find that the magnitude of this negative coefficient is, in general, higher in the area that combines various quantiles of GEOSDL with the upper quantiles of GDP growth rather than in the area that combines the intermediate quantiles of GEOSDL (0.35 to 0.55) with the intermediate quantiles of GDP growth (0.35 to 0.55). We also observe that the coefficient can turn to positive in the case of the lowest quantile of GDP growth. This finding could be possibly related to the beta-convergence hypothesis under which lower-income countries experience a higher growth rate than higher income countries. In our context, it could mean that the disaster forces these low-income countries with the lowest income growth to introduce some changes that eventually boost their GDP and result in a positive GEOSDL impact.Footnote 22 Another possible explanation is that such poor countries receive immediate support for post-disaster relief from other countries and international organizations, that helps them to sustain and even enhance their GDP growth.Footnote 23
In general, private sector reinsurance could also explain the observed differences between countries with high income and low/medium income. While we do not have data to conduct an empirical test, Duggar et al. (2016) highlight that according to information from SwissRe, over 40% of the direct loses from natural disasters are insured in developed countries compared to less than 10% of losses being covered in middle-income countries and less than 5% in low-income countries.
Consistent with Felbermayr and Gröschl (2014), we find that in the case of autocratic countries, natural disasters have a negative effect on economic growth. This effect is observed across all quantiles of both variables; however, it is somewhat higher at the lowest (0.05) and highest (0.95) quantiles of economic growth. Nonetheless, our results differ when we turn to the democratic countries. While Felbermayr and Gröschl (2014) report an insignificant coefficient, we find a statistically significant relationship which is negative up to the 75th quantile of GDP growth and turns to positive after that point (i.e. GDP growth quantiles of 0.85–0.95). These findings hold across the entire range of the quantiles of the disasters index. Overall, these results are similar to the ones obtained when looking at the high-income countries, which are countries typically scoring higher in democracy. Strömberg (2007) discusses at least two reasons that justify these findings. First, disasters may be less severe in countries with efficient and accountable governments, simply because it is the government that typically handles many of the measures that aim to mitigate the impact of natural disaster. Second, disasters can be less severe in those countries that are more democratic with a free press as previously discussed in the literature (Sen 1990).
In Table 6 we present estimations while distinguishing between tropical and non-tropical countries. In general, GEOSDL has a negative impact of GDP growth. Nonetheless, the results also show that in the case of the non-tropical countries, GEOSDL has a positive impact on growth when we look at the upper quantiles of GDP growth.Footnote 24 In the case of the tropical countries, the impact of GEOSDL on GDP growth appears to be positive in the lowest (0.05) and highest (0.95) quantiles of GDP growth. These differences between the two group of countries could be attributed to differences in the type and intensity of natural disasters between tropical and non-tropical countries. For example, tropical countries might be more frequently hit by tropical storms (e.g. Philippines, Haiti) and floods (e.g. Indonesia, Vietnam). In general, as discussed in Duggar et al. (2016), there can be differences between “low-frequency, high-severity” events (e.g. geophysical hazards) which cause large one-time losses from high-frequency, low-severity” events (e.g. climate-related hazards) which have a recurrent long-term impact on the economy.
3.2.2 Lagged effects of disasters
The results presented so far reveal that in most cases there is a negative relationship between natural disasters and economic growth. However, if it is true that natural disasters are followed by the replacement of capital stock and the adoption of new technologies then we could observe an increase in the economic growth in the medium term. To account for this, following Strobl (2011), Fomby et al. (2013) and Felbermayr and Gröschl (2014), we enter the disasters index in the regressions with a lag up to 5 years. This allows us to examine the impact of natural disasters not only in the year of occurrence (year t) but also in the years that follow. As in past studies, we examine up to five post disaster years, which provide an indication of the impact of natural disasters on economic growth in the short to medium term. We present these results in Table 7.
Consistent with Strobl (2011) with find that in several cases the coefficient of the t − 1 lag value is positive and significant. One potential explanation is that in the year immediately after the natural disaster, the receipt of disaster assistance, cleanup, and the production of replacement capital result in a net positive boost to the economy (Strobl 2011). However, our QQ approach reveals that in most cases, this finding holds only for low to intermediate quantiles of economic growth (0.05–0.65), and it is negative in the upper quantiles of GDP growth (0.75 − 0.95). A year later, at t − 2, the effect is mostly negative which is consistent with both Strobl (2011) and Felbermayr and Gröschl (2014). Nonetheless, we also find that in the case of the lower quantiles of GDP growth (0.05–0.25), natural disasters retain their positive effect on economic growth. In most cases, we observe a switch in the sign of the effect between years t − 3 and t − 4. More detailed, in the case of t − 3, the effect is negative for low to intermediate quantiles of GDP growth (0.15 to 0.45) and positive in the remaining cases. We observe the opposite in the case of t − 4. Finally, as it concerns t − 5, the effect is mostly positive for GDP growth quantiles in the rage of 0.15 to 0.85 (except for the 0.25 quantile that in some cases is negative or insignificant), and negative in the lowest (0.05) and highest (0.95) quantiles.
4 Conclusions
Theory suggests that the effects of natural disasters on economic growth are ambiguous, and the empirical studies that focus on the mean response of GDP growth provide inconclusive results. In the present study we propose the use of a quantile on quantile approach to shed more light on the complex relationship between natural disasters and economic growth. This approach combines the well-known attributes of standard quantile regression analysis with nonparametric estimations, allowing us to examine how different quantiles of natural disasters affect different quantiles of GDP growth.
Using yearly data over a 30 years period from around 100 developed and developing countries we obtain several interesting results. First, the immediate impact of natural disasters on economic growth is in general negative. However, for some quantiles of economic growth or certain combinations of the quantiles of natural disasters and the quantiles of economic growth, the effect is positive. Second, the magnitude of the effect also differs across different combinations of the two quantiles. Third, there appears to be some heterogeneity across groups of countries with different climate, and levels of economic and democratic development. Fourth, when we use lags to extend the analysis over a 5 years period after the natural disaster, we find that for certain yearly lags and for certain combinations of the quantiles of natural disaster and the quantiles of economic growth the effect is positive.
Our work has implications for policy making decisions, since climate change is expected to increase the frequency of natural disasters and extreme weather events (Kahn 2005; Sant’Anna 2018). While we recognize that it is difficult for policy makers to take actions that will prevent all the kinds of natural disasters this does not necessarily mean that there is nothing that they can do or that all the factors fall outside their control. For example, Sant’Anna (2018) concludes that public policies, like urban infrastructure, have an important role in reducing the impact of extreme rainfall. At a cross-country level, while there is no doubt that joint governmental decisions require long term efforts and willingness to cooperate, international actions to address climate change have received a lot of attention in recent years. However, as discussed in Kahn (2005) the political economy of who supports costly climate change policy hinges on the expected benefits of mitigating climate change. Along the same lines, Loayza et al. (2012) highlight the need to understand better the effect of natural disasters on economic growth in order to inform policy makers for the benefits from disaster risk reduction and mitigation.
To serve this task, our study went beyond the averages and explored how different quantiles of natural disasters affect different quantiles of GDP growth, and we also estimated separate QQ regressions for groups of countries that differ in terms of economic and democratic development, and for up to 5 five years in the post-disaster era. Although there are some exceptions to this, our study documents that national disasters have, in general, a negative effect on economic growth. This reveals the necessity to take actions that could possibly mitigate these negative effects and provides support to the literature that approaches natural disasters from a macroeconomic perspective suggesting the implementation of economic and environmental policy reforms with a particular focus on disaster preparedness (Rajapaska et al. 2017). Proper management before and after a disaster can be particularly important. For example, Özdamar et al. (2004) propose a planning model that can be integrated into a natural disaster logistics decision support system. Along the same lines, Jana et al. (2019) measure the importance of various humanitarian aid attributes with the use of a discrete choice multi-attributed value method. Kim et al. (2019) develop a hybrid multi-criteria decision-making model for the selection of logistics service providers in the disaster preparedness stage. While it is out of the scope of the present paper to develop such a model, future research could examine how the adoption of such operational research driven models helps in faster recovery and economic growth in the post-disaster era.Footnote 25
One shortcoming of our work, that is common to most empirical studies, is that our estimates do not assess the long-run impact of natural disasters on growth. However, as discussed in Noy (2009) this requires an entirely different framework due to numerous differences between countries that is difficult to control in the long turn. An interesting extension of our work would be to adopt the quantile on quantile approach and examine the impact of natural disasters on other country attributes like poverty, income inequality, and bilateral trade. One could also examine the impact of national disasters on individual components of GDP. For example, potential differences between personal consumption, business investment, government spending and balance of trade may shed further light on the impact of natural disasters on economic growth, and lead to the adoption of policies that will make the economy more resilient to natural disasters. We leave these issues to be addressed in future research.
Notes
The costs are even higher considering the underreporting of direct economic costs in relevant databases. The report highlights that The direct economic costs of disasters have been systematically under-reported worldwide for decades, both in wealthier countries and, most especially, in poorer ones. Throughout the period 1998–2017, economic losses data only exist for 37% of disasters; the direct cost of the majority of disasters (63%) is unknown or not well documented (p. 14).
Studies finding a negative impact are, among others, the ones of Noy (2009), Raddatz (2009), Strobl (2011), Felbermayr and Gröschl (2014). In contrast, Skidmore and Toya (2002) find that higher frequencies of climatic disasters are corelated with economic growth. Additionally, Loayza et al. (2012) conclude that moderate disasters (such as moderate floods) can have a positive growth effect in some sectors. Others claim that the impact is not significant. For instance, Albala-Bertrand (1993) concludes that The main conclusions are that capital loss is unlikely to have an important effect on growth and that a very moderate response expenditure may be sufficient to prevent the growth rate of output from falling (p. 1417). Felbermayr and Gröschl, (2014) review fourteen studies and highlight that from the 368 point estimates of the effect of disasters on GDP per capita, about 38% of all estimates are statistically insignificant at the 10% level, about 44% of the statistically significant effects are positive, while the remaining 56% of significant estimates are negative.
This literature has so far employed quantile regression to examine the growth effect of social capital (Deng et al. 2012), financial development (Andini and Andini 2014), political stability (Uddin et al. 2017), foreign direct investment (Cai et al. 2018), and energy consumption (Gozgor et al. 2018).
Existing studies in the field use a QQ approach to examine the relationship between oil prices and US equities (Sim and Zhou 2015), whether Bitcoin can hedge global uncertainty (Bouri et al. 2017), whether a partisan conflict predicts stock market volatility (Gupta et al. 2018), the relationship between economic policy uncertainty and equity premium (Raza et al. 2018), the association between uncertainty and currency performance (Han et al. 2019), the inflation—hedging property of gold (Shahzad et al. 2019) and the relationship between income distribution and environmental quality (Mallick et al. 2019).
The data are available online at: https://www.cesifo-group.de/ifoHome/facts/EBDC/Ifo-Research-Data/Ifo_GAME_Dataset.html.
The convenience of using asymmetric Laplace Distribution (ALD) is to easily allow the estimation of the τ-th conditional using the maximum likelihood methods.
The lagged natural logarithm of GDP per capita is included to account for the initial wealth per capita in a country (Felbermayr and Gröschl 2014; Strobl 2011). As opposed to Felbermayr and Gröschl (2014); Graham et al. (2015) clearly describe the expectations-based transformation used to sweep out fixed effects; for that reason, no endogeneity arises when the lagged dependent variable is introduced. Consequently, there is no biasness due to endogeneity when using a lagged dependent variable on the right-hand side of Eq. (3). Moreover, one avoids data transformation that could affect the interpretation of results. Nevertheless, Felbermayr and Gröschl (2014) clearly mentioned that they do not explicitly deal with the bias that arises from the inclusion of a lagged endogenous variable on the right-hand-side of the equation since the bias is small in panels drawn over a long time period like the one of our study (Nickell 1981; Judson and Owen 1999).In more detail, Nickell (1981) shows that the problem with the fixed effects model arises because the demeaning process subtracts the individual’s mean value of y and each X from the respective variable, creating a correlation between regression and error. Nickell demonstrates that the inconsistency of \( \widehat{\text{p}}\;{\text{as}}\;{\text{N}} \to \infty \) is of order 1/T. For reasonably large values of T, the limit of \( (\widehat{\text{p}} - {\text{p)}} \) as \( {\text{N}} \to \infty \) will be approximately − (1 + p)/(T − 1). Additionally, using a Monte Carlo approach, Judson and Owen (1999) reach several interesting conclusions. First, they mention that with the exception of OLS all the other estimators (least squares dummy variable—LSDV, Anderson-Hsiao—AH, GMM, Corrected LSDV) generally perform better as N and T increase, and therefore the differences in efficiency, bias and RMSES of the different techniques become quite small for large N and T. Second, using an RMSE criterion, the LSDV performs just as well or better than the viable alternatives when T = 30. Third, while GMM produces the lowest RMSEs when T = 20, the difference in performance is not that great and computation issues may become more important.
The literature on the macroeconomic effects of disasters can be divided into studies looking into the short- to-medium term and the longer term, with almost all studies taking a shorter-term perspective (Hochrainer, 2009). Long-run analysis raises questions of endogeneity in disaster impact that are, to a large extent, not relevant for the short-run (Noy 2009). Therefore, as in Noy (2009), Strobl (2011) and Felbermayr and Gröschl (2014), among others, we examine the impact of the disasters occurring in time t on the GDP growth over t − 1 to t (i.e. the change from year t − 1 to year t). In further analysis in Sect. 3.2.2. we extend the analysis to the medium term using up to five lags of the natural disaster index.
Felbermayr and Gröschl (2014) use one more index from the insurance firm Munich Re, called NatCatSERVICE. However, this is not included in the dataset that they make available online. Most likely this is due to the fact that the database NatCatSERVICE-in contrast to EM-DAT and GeoMet—is not publicly available.
The decision rule for large-scale disasters from EM-DAT and NatCatSERVICE bases on the convention of ‘great natural disasters’ by Munich Re (2006) and the United Nations. Disasters are defined as large if (i) 1000 or more were killed, or (ii) at least 1 billion US dollar monetary damage (made comparable over time using the deflator on US dollars fromWDI), and with EM-DAT also if (iii) 100,000 or more affected.
For example, GEOLAND ranges between 0.000 (e.g. Australia, Brazil) to 2.155 (Singapore in 1985), while GEOSDL ranges between 0.000 (e.g. Australia, Brazil) and 2.228 (Singapore in 1984).
Fisher (1925) proposed the combination of the p-values from independent tests to obtain an overall test statistic and is frequently called a Fisher-type test. We perform the test in Stata which uses the p-values from the panel-specific unit-root tests based on the methods proposed by Choi (2001). The null hypothesis being tested is that all panels contain a unit root. For a finite number of panels, the alternative is that at least one panel is stationary.
All the p-values (available upon request) are equal to either 0.000 or 0.001.
Felbermayr and Gröschl (2014) also include the natural logarithm of population, which we drop for two reasons. First, when we examine the within country correlation of GDP and population, we find that in several occasions there are large correlations which raise concerns about multicollinearity issues. Second, having both elements of the dependent variable (i.e. GDP and population) in the right-hand side of the regression could raise additional concerns. In unreported Fixed effects regressions we also estimated the specifications of Table 2 with the inclusion of the logarithm of population. The only difference is that with the inclusion of the logarithm of population, GEOLAND was also statistically significant (at the 5% level) in the specification in Column 5. However, given the multicollinearity concerns, these results should be treated with caution.
As in the case of the country-fixed effects model, the QQ specifications include lagged values of the following control variables, which are not shown to conserve space: LCGDP, POLITY, OPEN, INTEREST, CAPITAL, FDI, CPI, BALANCE.
In the case of the “Disaster index” (i.e. GEOLAND) they find that it has a negative and statistically significant impact on both non-OECD countries (at the 1% level) and OECD countries (at the 10% level), the low/middle economies (at the 10% level) and in autocratic countries (at the 10% level), and an insignificant impact in the case of high income and democratic economies.
To classify countries as tropical or non-tropical ones, we use information on the percentage of population in each country living in tropical and subtropical zones, taken from Ashraf and Galor (2013).
As before, we estimate a model with country fixed effects, time dummies and robust errors clustered at the country level. Estimating this model without time dummies does not influence the results.
One could expect the results for the high vs medium/low income group countries to be identical to the ones of the OECD versus non-OECD countries. The estimations in Tables 4 and 5 show that this is not necessarily the case. The reason for this is that despite similarities, these group are not identical. The correlation between the dummy for OECD membership and the one for High income group membership is 0.786. In more detail, the group of high-income countries includes numerous countries like Cyprus, Israel, South Korea, Singapore, Kuwait, Oman, Slovenia, Trinidad and Tobago that are not OECD members, and at the same time most of them appear to be among the countries that experience the highest GDP difference (e.g. South Korea, Kuwait, Oman, Singapore, Trinidad and Tobago) among the high-income ones.
There exist many studies that test the conditional convergence of GDP per capita across countries. For discussions of the literature and alternative methodologies see de la Fuente (1997), Rassekh (1998) and Diaz del Hoyo et al. (2017). Conducting such an analysis does not fall into the purpose of the present study.
For example, Becerra et al. (2012) conclude that, controlling for the magnitude of the disaster, countries with higher real GDP per capita receive less post-disaster foreign aid. Strömberg (2007) also reports that there is a negative association between the real GDP per capita of a country and whether the Office of U.S. Foreign Disaster Assistance (OFDA) provided relief for a given disaster between 1968 and 2002.
The results for the group of non-tropical countries are, to some extent, close to the ones of the high-income group. This could be partially justified by the fact that many high − income countries are located in non-tropical areas.
See Zhang et al. (2019) for a similar model, and Hoskins and Medal (2019) for a stochastic programming solution for the placement of satellite ground stations. Additionally, for a discussion on the recent use of big data in humanitarian supply chain management in the case of disaster relief see Gupta et al. (2019).
References
Albala-Bertrand, J. M. (1993). Natural disaster situations and growth: a macroeconomic model for sudden disaster impacts. World Development, 21, 1417–1434.
Andini, M., & Andini, C. (2014). Finance, growth and quantile parameter heterogeneity. Journal of Macroeconomics, 40, 308–322.
Ashraf, Q., & Galor, O. (2013). The ‘Out of Africa’ hypothesis, human genetic diversity, and comparative economic development. American Economic Review, 103, 1–46.
Bakkensen, L., & Barragey, L. (2016). Do disasters affect growth? A macro model-based perspective on the empirical debate, Working Paper, No. 2016-9, Brown University, Department of Economics, Providence, RI.
Becerra, O., Cavallo, E., & Noy, I. (2012) Foreign Aid in the Aftermath of Large Natural Disasters, IDB Working paper series No. IDB—WP—333, August.
Bouri, E., Gupta, R., Tiwari, A. K., & Roubaud, D. (2017). Does Bitcoin hedge global uncertainty? Evidence from wavelet-based quantile-in-quantile regressions. Finance Research Letters, 23, 87–95.
Cai, Z., Chen, L., & Fang, Y. (2018). A semiparametric quantile panel data model with an application to estimating the growth effect of FDI. Journal of Econometrics, 206, 531–553.
Choi, I. (2001). Unit root tests for panel data. Journal of International Money and Finance, 20, 249–272.
De la Fuente, A. (1997). The empirics of growth and convergence: A selective review. Journal of Economic Dynamics and Control, 21, 23–73.
Deng, W.-S., Lin, Y.-C., & Gong, J. (2012). A smooth coefficient quantile regression approach to the social capital–economic growth nexus. Economic Modelling, 29, 185–197.
Diaz del Hoyo, J. L., Dorrucci, E., Heinz, F. F., & Muzikarova, S. (2017). Real convergence in the euro area: A long-term perspective, ECB Occasional Paper Series No 203, December.
Duggar, E. H., Li, Q., & Praagh, A. V. (2016). Understanding the impact of natural disasters: Exposure to direct damages across countries, global credit strategy—environmental risks, Moody’s Investor Service, 28 November.
Felbermayr, G., & Gröschl, J. (2014). Naturally negative: The growth effects of natural disasters. Journal of Development Economics, 111, 92–106.
Fisher, R. A. (1925). Statistical methods for research workers. Edinburgh, Scotland: Oliver and Boyd.
Fomby, T., Ikeda, Y., & Loayza, N. V. (2013). The growth aftermath of natural disasters. Journal of Applied Econometrics, 28, 412–434.
Geraci, M., & Bottai, M. (2014). Linear quantile mixed models. Statistics and Computing, 24(3), 461–479.
Gozgor, G., Lau, C. K. M., & Lu, Z. (2018). Energy consumption and economic growth: New evidence from the OECD countries. Energy, 153, 27–34.
Graham, B. S., Hahn, J., Poirier, A., & Powell, J. L. (2015). Quantile regression with panel data, Working Paper No. 21034, National Bureau of Economic Research.
Gupta, S., Altay, N., & Luo, Z. (2019). Big data in humanitarian supply chain management: a review and further research directions. Annals of Operations Research, 283, 1153–1173.
Gupta, R., Pierdzioch, C., Selmi, R., & Wohar, M. E. (2018). Does partisan conflict predict a reduction in US stock market (realized) volatility? Evidence from a quantile-on-quantile regression model. North American Journal of Economics and Finance, 43, 87–96.
Han, L., Liu, Y., & Yin, L. (2019). Uncertainty and currency performance: A quantile-on-quantile Approach. North American Journal of Economics and Finance, 48, 702–729.
Hochrainer, S. (2009). Assessing the macroeconomic impacts of natural disasters are there any? Policy Research Working Paper No. 4968, June, World Bank.
Hoskins, A. B., & Medal, H. R. (2019). Stochastic programming solution for placement of satellite ground stations. Annals of Operations Research, 283, 267–288.
Jana, P. K., Chandra, C. P., & Tiwari, A. K. (2019). Humanitarian aid delivery decisions during the early recovery phase of disaster using a discrete choice multi-attribute value method. Annals of Operations Research, 283, 1211–1225.
Judson, R., & Owen, A. (1999). Estimating dynamic panel data models: a guide for macroeconomists. Economics Letters, 65, 9–15.
Kahn, M. E. (2005). The deal toll from natural disasters: The role of income, geography, and institutions. Review of Economics and Statistics, 87, 271–284.
Kim, S., Ramkumar, M., & Subramanian, N. (2019). Logistics service provider selection for disaster preparation: A socio-technical systems perspective. Annals of Operations Research, 283, 1259–1282.
Koenker, R. (2014). Quantile regression, Wiley StatsRef: Statistics reference online (originally published online in 2006 Encyclopedia of Environmetrics).
Koenker, R., & Bassett, G., Jr. (1978). Regression quantiles. Econometrica, 46, 33–50.
Loayza, N. V., Olaberria, E., Rigolini, J., & Christiaensen, L. (2012). Natural disasters and growth: Going beyond the averages. World Development, 40, 1317–1336.
Mallick, H., Padhan, H., & Mahalik, M. K. (2019). Does skewed pattern of income distribution matter for the environmental quality? Evidence from selected BRICS economies with an application of Quantile-on-Quantile regression (QQR) approach. Energy Policy, 129, 120–131.
Munich, Re. (2006). Topics geo annual review: Natural catastrophes 2005. Münchener Rückversicherungs-Gesellschaft.
Nickell, S. (1981). Biases in dynamic models with fixed effects. Econometrica, 49, 1417–1426.
Noy, I. (2009). The macroeconomic consequences of disasters. Journal of Development Economics, 88, 221–231.
Özdamar, L., Ekinci, E., & Küçükyazici, B. (2004). Emergency logistics planning in natural disasters. Annals of Operations Research, 129, 217–245.
Raddatz, C. (2009). The wrath of God: Macroeconomic costs of natural disasters, Policy Research Working Paper No. 5039, September, World Bank.
Rajapaska, D., Islam, M., & Managi, S. (2017). Natural capital depletion, the impact of natural disasters on inclusive growth. Economics of Disasters and Climate Change, 1, 233–244.
Rassekh, F. (1998). The convergence hypothesis: History. Theory, and Evidence, Open economies review, 9, 85–105.
Raza, S. A., Zaighum, I., & Shah, N. (2018). Economic policy uncertainty, equity premium and dependence between their quantiles: Evidence from quantile-on-quantile approach. Physica A, 492, 2079–2091.
Sant’Anna, A. A. (2018). Not so natural: Unequal effects of public policies on the occurrence of disasters. Ecological Economics, 152, 273–281.
Sen, A. (1990). Public action to remedy hunger. In The fourth annual Arturo Tanco memorial lecture, delivered August 2, 1990, at Queen Elizabeth II Conference Centre London. (Printed In: The Hunger Project. New York; 1990. And Republished in Interdisciplinary Science Reviews, 1991, Volume, 16, Issue 4, pp. 324–336).
Shahbaz, M., Zakaria, M., Shahzad, S. J. H., & Mahalik, M. K. (2018). The energy consumption and economic growth nexus in top ten energy-consuming countries: Fresh evidence from using the quantile-on-quantile approach. Energy Economics, 71, 282–301.
Shahzad, S. J. H., Mensi, W., Hammoudeh, S., Sohailf, A., & Al-Yahyaee, K. H. (2019). Does gold act as a hedge against different nuances of inflation? Evidence from Quantile-on-Quantile and causality-in- quantiles approaches. Resources Policy, 62, 602–615.
Shahzad, S. J. H., Shahbaz, M., Ferrer, R., & Kumar, R. R. (2017). Tourism-led growth hypothesis in the top ten tourist destinations: New evidence using the quantile-on-quantile approach. Tourism Management, 60, 223–232.
Sim, N., & Zhou, H. (2015). Oil prices, US stock return, and the dependence between their quantiles. Journal of Banking & Finance, 55, 1–8.
Skidmore, M., & Toya, H. (2002). Do natural disasters promote long-run growth? Economic Inquiry, 40, 664–687.
Strobl, E. (2011). The economic growth impact of hurricanes: Evidence from U.S. coastal counties. Review of Economics and Statistics, 93, 575–589.
Strömberg, D. (2007). Natural disasters, economic development, and humanitarian aid. Journal of Economic Perspectives, 21, 199–222.
Uddin, M. A., Ali, M. H., & Masih, M. (2017). Political stability and growth: An application of dynamic GMM and quantile regression. Economic Modelling, 64, 610–625.
Wallemacq, P., & House, R. (2018). Economic losses, poverty & disasters 1998–2017, Centre for Research on the Epidemiology of Disasters and United Nations Office for Disaster Risk Reduction, Available at: https://www.unisdr.org/we/inform/publications/61119. Retrieved 15 Apr 2019.
Zhang, J., Wang, Z., & Ren, F. (2019). Optimization of humanitarian relief supply chain reliability: A case study of the Ya’an earthquake. Annals of Operations Research, 283, 151–172.
Acknowledgements
We are thankful to Professor Nicholas Sim for providing us with the code developed in Sim and Zhou (2015), which has been used in this study to extend the QQ regression to panel data. In this regard, special thanks are also due to Professor Naji Jalkh for his valuable comments and suggestions. We would also like to thank two anonymous referees for insightful comments that helped us improve an earlier version of the manuscript. Any remaining errors are our own. Montpellier Business School (MBS) is a founding member of the public research center Montpellier Research in Management, MRM (EA 4557, Univ. Montpellier).
Author information
Authors and Affiliations
Corresponding author
Additional information
Publisher's Note
Springer Nature remains neutral with regard to jurisdictional claims in published maps and institutional affiliations.
Appendix: Definition of variables
Appendix: Definition of variables
Acronym | Definition |
---|---|
GDP growth | Per capita GDP PPP growth, calculated as first difference in ln of GDP per capita from year t− 1 to year t |
EMALL | EM-DAT All disasters index, defined as the sum of all disasters in EM-DAT database normalized by land area |
EMLAR | EM-DAT Large disasters index, defined as the sum of Large disasters in EM-DAT database normalized by land area |
GEOLAND | GeoMet Disaster Index, defined as the unweighted sum of disasters types in GeoMet database, normalized by land area |
GEOSDL | GeoMet Disaster Index_weighted, defined as the sum of disaster types in GeoMet database, weighted by the country specific inverse of standard deviation of a disaster type within a country over all years, and normalized by land area |
LCGDP | ln of per capita GDP PPP |
POLITY | Polity index from Polity IV (2010), capturing the degree of democracy/autocracy in a country, normalized between 0 (strongly autocratic) and 1 (strongly democratic) |
OPEN | Indicator of trade openness calculated as imports plus exports over GDP |
INTEREST | Real interest rate |
CREDIT | Domestic credit in banking sector as a share of GDP |
CAPITAL | Gross capital formation (share of growth) |
FDI | Foreign direct investment, net inflows as a share of GDP |
CPI | Inflation, consumer prices |
BALANCE | Current account balance as a share of GDP |
Rights and permissions
About this article
Cite this article
Atsalakis, G.S., Bouri, E. & Pasiouras, F. Natural disasters and economic growth: a quantile on quantile approach. Ann Oper Res 306, 83–109 (2021). https://doi.org/10.1007/s10479-020-03535-6
Published:
Issue Date:
DOI: https://doi.org/10.1007/s10479-020-03535-6