Abstract
Humanitarian organizations work diligently to save lives using scarce resources, competing for donor money, and operating in complex environments. It is no surprise that they need information to effectively execute their task. As there have been tremendous developments in data analytics it is imperative that the domain of humanitarian supply chain management leverage the benefits offered by the advancement of big data. In this study, we have conducted a systematic literature review in the field of big data and humanitarian supply chain. The data was collected using Scopus which is the largest digital database. After careful screening, only 28 journal papers were selected for literature review. These papers have been classified and grouped into various categorizations. Future research directions in this field have been suggested that are based on various organizational theories.
Similar content being viewed by others
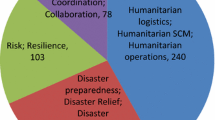
Explore related subjects
Discover the latest articles, news and stories from top researchers in related subjects.Avoid common mistakes on your manuscript.
1 Introduction
Disasters have taken place on innumerable counts and have caused severe damage to nature and civilization (Starr and Van Wassenhove 2014). In 2015, 160 disasters had struck Asia-Pacific region out of the total of 344 disasters that struck worldwide (UNESCAP Report 2016). Asia-Pacific region accounts for 47% of the disasters with over 16,000 deaths (64% of the total worldwide deaths caused by disasters) and this was more than double of the destruction caused in 2014 (UNESCAP Report 2016). Therefore, it is imperative that we understand how to mitigate, prepare for and respond to disasters more effectively in the future.
Disasters can be broadly classified as natural or man-made disasters. According to Van Wassenhove (2006), logistics operations constitute 80% of the total money allocated for disaster relief operations. Day et al. (2012) has argued that nearly 40% of this allocation is wasted because there is lack of time to perform data analysis and also there is duplication of the efforts. Akhtar et al. (2012), Li et al. (2016) and Papadopoulos et al. (2017) have also made similar claims in their studies as they mention that there is lack of collaboration and coordination during the disaster relief operations and thus the relationships between all the actors get adversely affected. Altay and Labonte (2014) and Aloysius et al. (2016) have strongly advocated that information processing is critical for decision making and coordination during humanitarian relief operations.
Data science and business analytics have made in-roads in our day to day life and advancements in this field has led to understanding the mystery that has been hidden in large sets of data (Agarwal and Dhar 2014). According to Huang and Chaovalitwongse (2015), the term “big data” has been defined as an umbrella term for any collection of large and complex data sets that are difficult to store, process, analyze, and comprehend using traditional database or data processing tools. Pu and Kitsuregawa (2013) have shown that National Science Foundation of USA and the Japan Science and Technology Agency have laid emphasis on big data and disaster management. These two scientific institutions have outlined that integration of multiple data sources and thereby achieving accurate information is a matter of concern in disaster management. There is no dearth of data and this is evident from the fact that a massive amount of remote sensing data is made freely available by NASA Open Government Initiative. Under this initiative, data is stored under archives and one such archive named Earth Science Data and Information System (ESDIS) has about 7.5 PB of data (\(1\,\hbox {petabyte}= 1024\,\hbox {terabytes}\)) (Ramapriyan et al. 2013). It is expected that the amount of data generated by 2020 will be more than 40 ZB (\(1\,\hbox {zettabyte} = 10^{6} \, \hbox {petabytes}\)) (Song et al. 2016).
Majority of the Asia-Pacific countries do not possess the capability to ascertain which place has suffered how much of the damage and where the disaster relief operations should be channelized so that disaster management plans are effectively executed (UNESCAP Report 2016). However, now there is a satellite named as South Asia Satellite (launched in May 2017 by India) which will benefit 8 countries in the South-East Asia region and this will hopefully enhance the capability of these countries to better manage disasters. On the other hand in Europe the Emergency Response Coordination Center working under the European Commission’s Humanitarian Aid and Civil Protection Department acts as emergency operation centers equipped with information systems to streamline the humanitarian operations during disasters (Ma and Zhang 2017). Similarly, there are operation centers like these which are established in various countries so that the relief operations are managed efficiently and effectively as well. Large amounts of data are created in these operation centers and the use of big data analytics has tremendous potential to improve upon humanitarian supply chain management (HSCM) practices (Prasad et al. 2016).
Since the interest in the use of big data within the context of humanitarian supply chain management has been increasing (adapted from Liu and Yi 2017), we decided to undertake a literature review in this field. The research objective of this study can be broadly considered as: (a) to showcase the state of the art in the field of big data and its role in supporting humanitarian supply chain decisions, (b) to understand the context from the perspective of organizational theories and (c) to suggest further research directions in the domain of big data and humanitarian supply chain management.
This paper has been divided into sections and sub-sections. Section 2 outlines the research methodology employed in undertaking the systematic literature review and this showcases the state of the art for the selected topic of study. Section 3 is the discussion section and it deals with answering the second and the third aspect of the research objectives.
2 Research methodology
In this section, we have discussed the process and methodology that has been followed to undertake this study. The systematic literature review that we have undertaken has been based on the guidelines laid out by Tranfield et al. (2003) and Dubey et al. (2017a). The research methodology has been broadly classified into three parts as (i) Planning the Review, (ii) Conducting the Review and (iii) Reporting the Review. We have addressed these three aspects in the following sub-sections and we have discussed the research methodology in detail. Section 2.1 describes the details that were considered while planning the review and brings out the manner in which the search was performed for this review. In Sects. 2.2 and 2.3, we have reported the various aspects of big data and humanitarian supply chain.
2.1 Identification of literature
The source of literature for this study is the Scopus database (https://www.scopus.com) which is now the biggest and the largest database for academic journals as well as conference proceedings. Scopus lists papers in four categories, namely (i) Life Sciences, (ii) Health Sciences, (iii) Physical Sciences and (iv) Social Sciences allowing for cross-disciplinary research, and this was another reason for selecting Scopus for this study. Disasters by their very nature lend themselves well to multi-disciplinary research. As the spectrum of our topic is represented by all of these four categories, use of Scopus has been a natural choice. There are other digital databases like Web of Science, DBLP and WorldCat but Scopus contains more academic journals than these databases.
Our study deals with the amalgamation of two independent concepts, one from the domain of information systems (big data) and the other from the domain of operations management (humanitarian supply chain). The keywords used to search the academic literature for these two concepts can be seen in Table 1.
The search for these concepts was performed independently on Scopus using ‘or’ operator for each keyword and thereafter the search results of these two concepts was merged using an ‘and’ operator in Scopus. The search syntax can be seen in Table 2. In order to replicate this search on Scopus.com, this syntax can be copied and pasted in the advanced search section of that digital database. The search result will vary in the number of documents as this database is actively updated. The data for this study has been taken from the first possible date of indexation of a journal paper to the date of search performed on Scopus database (March 03, 2017). Thus, this data is a true reflection of the information that was showcased on March 03, 2017.
Our search process described in Fig. 1 resulted in 28 journal articles that we reviewed in this paper. In the first stage we conducted a search for big data related keywords (see Table 1). This resulted in 49,778 hits. The second stage, consisted of a search for HSC keywords within the results of the first stage, resulting in 21,937 hits. In the third stage, an intersection of the data from stage one and stage two has been selected and this process yielded in 119 papers. In stage four, we have considered only journal articles (38 papers) for the literature review and discarded conference proceedings. During the search it was found that there were 70 conference proceedings, 7 conference review, 3 book chapters and 1 short survey paper. Within the 70 conference proceedings, 57 papers were from the field of Computer Science only and this outcome is heavily skewed. Except for the domain of Computer Science, researchers from other academic domains prefer to publish in journals rather than conferences (Hermenegildo 2012; Derntl 2014). Thus, for this reason we did not consider conference proceedings in this study. Also, only those journal articles were considered which were published in English language. In stage five, we had manually gone through each of the 38 papers to ascertain which of the papers can be finally considered for literature review. We had considered papers that have addressed the domain of humanitarian supply chain management while highlighting the emergence of big data. Only, 28 papers were found to be a good fit for this study. In Fig. 2, it can be clearly seen that the spectrum of these 28 papers truly reflect the various academic domains. Since, all of the academic domains have a balanced representation, our decision to only consider journal articles is justified. In Appendix A, the journals in which these 28 papers have been published are shown. It can be clearly seen from Appendix A that there is no pattern or a particular journal where the number of publications is high. Although, within the HSCM field there is a dedicated journal, namely the Journal of Humanitarian Logistics and Supply Chain Management, only one paper has been published in this journal in 2016 that fits into the search criteria. However, based on Fig. 3 we can see that the number of papers is increasing in the intersection of big data and HSCM. Appendix D lists the 28 journal papers that we have considered for this study.
In Fig. 3 it can be clearly seen that this field has gained importance from 2015 onwards in the academic parlance.
2.2 Classification of literature
The papers in this study could be classified on the basis of various organizational theories (Sarkis et al. 2011) or by using various building blocks (Gunasekaran and Spalanzani 2012) but we have used the classification scheme employed by Dubey et al. (2017a). The classification scheme outlined by Dubey et al. (2017a) encompasses the strengths and merits of the seminal work of Sarkis et al. (2011) and Gunasekaran and Spalanzani (2012). Their classification scheme has been inspired by the seminal works of Whetten (1989) and Sutton and Staw (1995). In Fig. 3, the classification scheme for the selected journals can be seen and in Appendix B, we have categorized the papers based on this scheme.
The literature has been broadly categorized into two classes, namely, Theory Building and Application Based Research. In the Theory Building category, the focus has been on identifying papers that contribute to the existing organizational theories by either supporting, extending or even criticizing the same. This has further been sub-divided into the Rationalist Approach where papers have been selected based on the contribution to the theories as well as advancing the current state of research in this field by developing critical review papers. The other aspect of the Theory Building category, Alternative Methods revolves around conceptual frameworks that have been tested by empirical analysis or by case studies. The second aspect of listing papers apart from the Theory Building category is the Application Based Research segment. In this segment, papers are considered as cases where industry focused research has been undertaken.
2.3 Understanding the concepts used in this study
Based on the literature review of 28 papers, various enablers as well as concerns of big data in HSCM have been identified that will lead to a better understanding of this field of. These enablers and concerns have been listed keeping in view of the fact that this study is dealing with how big data can aid the humanitarian supply chain management. The papers that have mentioned about these enablers and sources have been shown in Appendix C.
The enablers for big data in humanitarian supply chain management are:
- (a)
Volume This has been the most cited enabler amongst the rest of them. Disasters create chaotic environments which breeds uncertainty for the survivors. In such a situation people tend to reach out to their near and dear ones and thus this anxiety in the minds of people leads to the creation of a very large data set (Huang and Chaovalitwongse 2015; Zhan et al. 2016). The papers considered for this study have categorically mentioned this attribute in the following ways (Refer to Appendix C): (1) Scale of data, (2) Stored data and (3) Continuous creation of data.
- (b)
Variety Data is generated by many devices and in many formats. Broadly, the data can be classified as unstructured data, semi-structured data and structured data (Agarwal and Dhar 2014; Banerjee et al. 2016). During any humanitarian operation, there is a surge in the creation of data in all possible formats within these three broad categories. The papers have highlighted the following aspects (Refer to Appendix C): (1) Data variety and (2) Interoperability within the different data formats.
- (c)
Velocity The rate at which data is generated during humanitarian operations can be very fast. The aspects of velocity that have been considered in the papers we reviewed include (Refer to Appendix C): (1) Speed of data generation, (2) Data processing time and (3) Data transmission time.
- (d)
Veracity Accuracy and reliability of data refers to veracity (White 2012). The selected journal papers have highlighted this aspect in the following way (Refer to Appendix C): (1) Quality of information, (2) Accuracy of data, (3) Reliability and (4) Accessibility.
- (e)
Organizational mindfulness It is the capability to avert potential accidents by organizations which are highly reliable in their operations. Weick et al. (1999) list the five dimensions of organizational mindfulness as: (1) Preoccupation with failure, (2) Reluctance to simplify interpretations, (3) Sensitivity to operations, (4) Commitment to resilience and (5) Deference to expertise. These five dimensions have been reflected by few of the selected papers considered in this study as presented in Appendix C.
The concerns identified for big data in humanitarian supply chain management are:
- (a)
Humanitarian logistics Warehousing and delivery of essential commodities during disaster relief operations constitutes humanitarian logistics (Van Wassenhove 2006; Boone et al. 2016; Chu et al. 2016; Hazen et al. 2016). The concerns identified during the review of the selected papers are (Refer to Appendix C): (1) Identification of logistics service providers, (2) Collaboration between agencies during humanitarian operations, (3) Response time of logistics agencies/organizations.
- (b)
Remote sensing The capability to perform remote sensing monitoring before and after any disaster adds tremendous decision making capability during HSCM. The concerns identified from the selected papers are (Refer to Appendix C): (1) Real time rendering of disaster location, (2) High-speed buffering mechanisms, (3) Large-scale 3D model visualization.
- (c)
Information security: Transmission of large sets of data on a continuous basis during disaster relief operations is a regular activity. The key aspects identified from the papers are (Refer to Appendix C): (1) Privacy and confidentiality of data, (2) Encryption, (3) Accountability, (4) Maintenance.
- (d)
Social media (SM ): Although SM is a fairly new phenomenon it plays a key role when disasters strike. The amount of data generated by SM during humanitarian operations can help relief organizations to streamline their efforts (Amaye et al. 2016). For example, Facebook lets its users declare themselves safe if they are near the disaster zone and it also shares this information with various disaster relief organizations (TechCrunch 2017). This not only informs the near and dear ones but also serves as a tool for the aid agencies to estimate how many people are displaced. The concerns for SM identified from the selected papers are (Refer to Appendix C): (1) Diffusion of information by SM, (2) Validation of information, (3) Support coordination and collaboration among agencies.
3 Discussion
The results obtained from Scopus has been discussed in this section and we have expressed our understanding in the following three sub-sections.
3.1 Theoretical contributions and future research directions
This section focusses on addressing the second and third research objectives. We have identified the research gaps and future research directions based on the various organizational theories. We consulted the work of Sarkis et al. (2011) and Dubey et al. (2017b) in identifying the organizational theories that could be considered for this study. The organizational theories we see as potential sources of research questions, their short synopses, and our proposed research directions are presented in Table 3.
3.2 Managerial implications
The field of Data Science has gathered enough momentum and use of big data analytics can provide answers to some of the strategic as well as tactical questions which were ignored until now (Ji-fan Ren et al. 2016). The analysis of unstructured data is the biggest innovation in HSCM. The amount of unstructured data generated during a disaster is immense and all of this takes place within a short span of time. One source for unstructured data is social media. Sentiment analysis of this unstructured data can assist in the execution of humanitarian relief operations. Furthermore, cognitive systems like IBM Watson have shown that machine-machine interaction to analyze large sets of data is going to be a reality in the near future (Chen et al. 2016). Until then however, man-machine interaction is vastly employed to better understand unstructured data. Thus, trained manpower in the domain of big data in HSCM will help in mitigating the limitations of this field.
3.3 Limitations of this study
First, in our search we concentrated only on journal papers and dismissed books and conference proceedings. It is possible that these academic avenues would contain information that would have been of much importance for this study. Second, other digital databases like Web of Science, DBLP and WorldCat have not been considered for collecting data for this study. The premise for this omission is based on the fact that Scopus is the largest and most inclusive digital database. Nonetheless, some papers might have been missed if the source is not covered by Scopus. Third, the data from Scopus has been collected in March 2017. We have made a conscious effort to show the result only till 2016 in Fig. 3. This is because the papers for 2017 are still in the process of being indexed and thus Fig. 3 will not show the true representation if we would have considered 2017 in that figure.
References
Agarwal, R., & Dhar, V. (2014). Editorial—Big data data science, and analytics: The opportunity and challenge for IS research. Information Systems Research, 25(3), 443–448.
Akhtar, P., Marr, N. E., & Garnevska, E. V. (2012). Coordination in humanitarian relief chains: chain coordinators. Journal of Humanitarian Logistics and Supply Chain Management, 2(1), 85–103.
Aloysius, J. A., Hoehle, H., Goodarzi, S., & Venkatesh, V. (2016). Big data initiatives in retail environments: Linking service process perceptions to shopping outcomes. Annals of Operations Research. https://doi.org/10.1007/s10479-016-2276-3.
Altay, N., & Labonte, M. (2014). Challenges in humanitarian information management and exchange: Evidence from Haiti. Disasters, 38, 50–72.
Amaye, A., Neville, K., & Pope, A. (2016). Big promises: Using organisational mindfulness to integrate big data in emergency management decision making. Journal of Decision Systems, 25, 76–84.
Banerjee, S., Sanghavi, S., & Shakkottai, S. (2016). Online collaborative filtering on graphs. Operations Research, 64(3), 756–769.
Barney, J. B. (1991). Firm resources and sustained competitive advantage. Journal of Management, 17(1), 99–120.
Barrow, C. W. (1998). State theory and the dependency principle: An institutionalist critique of the business climate concept. Journal of Economic Issues, 32(1), 107–144.
Boone, C. A., Hazen, B. T., Skipper, J. B., & Overstreet, R. E. (2016). A framework for investigating optimization of service parts performance with big data. Annals of Operations Research. https://doi.org/10.1007/s10479-016-2314-1.
Brammer, S., Jackson, G., & Matten, D. (2012). Corporate social responsibility and institutional theory: New perspectives on private governance. Socio-Economic Review, 10(1), 3–28.
Chen, Y., Argentinis, E., & Weber, G. (2016). IBM Watson: How cognitive computing can be applied to big data challenges in life sciences research. Clinical Therapeutics, 38(4), 688–701.
Chu, J. F., Wu, J., & Song, M. L. (2016). An SBM-DEA model with parallel computing design for environmental efficiency evaluation in the big data context: A transportation system application. Annals of Operations Research. https://doi.org/10.1007/s10479-016-2264-7.
Crisp, C. B., & Jarvenpaa, S. L. (2013). Swift trust in global virtual teams: Trusting beliefs and normative actions. Journal of Personnel Psychology, 12(1), 45–56.
Crozier, M., & Thoenig, J. C. (1976). The regulation of complex organized systems. Administrative Science Quarterly, 21, 547–570.
Day, J. M., Melnyk, S., Larsonf, P., Davis, E., & Whybark, D. C. (2012). Humanitarian and disaster relief supply chains: A matter of life and death. Journal of Supply Chain Management, 48(2), 2–36.
Derntl, M. (2014). Basics of research paper writing and publishing. International Journal of Technology Enhanced Learning, 6(2), 105–123.
DiMaggio, P. J., & Powell, W. W. (1983). The iron cage revisited: Institutional isomorphism and collective rationality in organizational fields. American Sociological Review, 48(2), 147–160.
Dubey, R., Gunasekaran, A., Childe, S. J., Papadopoulos, T., & Fosso-Wamba, S. (2017a). World class sustainable supply chain management: Critical review and further research direction. International Journal of Logistics Management. https://doi.org/10.1108/IJLM-07-2015-0112.
Dubey, R., Gunasekaran, A., & Papadopoulos, T. (2017b). Green supply chain management: Theoretical framework and further research directions. Benchmarking: An International Journal, 24(1), 184–218.
Freeman, R. E. (2001). Stakeholder theory of the modern corporation. In W. M. Hoffman, R. E. Frederick, & M. S. Schwartz (Eds.), Business ethics: Readings and cases in corporate morality (4th ed.). Boston: McGraw-Hill.
Glover, J. L., Champion, D., Daniels, K. J., & Dainty, A. J. D. (2014). An institutional theory perspective on sustainable practices across the dairy supply chain. International Journal of Production Economics, 152, 102–111.
Guide, V. D. R, Jr., & Van Wassenhove, L. N. (2009). OR FORUM—The evolution of closed-loop supply chain research. Operations Research, 57(1), 10–18.
Gunasekaran, A., & Spalanzani, A. (2012). Sustainability of manufacturing and services: Investigations for research and applications. International Journal of Production Economics, 140(1), 35–47.
Gunasekaran, A., Irani, Z., & Papadopoulos, T. (2014). Modelling and analysis of sustainable operations management: Certain investigations for research and applications. Journal of the Operational Research Society, 65(6), 806–823.
Hambrick, D. C. (2007). Upper echelons theory: An update. Academy of Management Review, 32(2), 334–343.
Hannan, M. T., & Carroll, G. R. (1992). Dynamics of organizational populations: Density, legitimation, and competition. New York: Oxford University Press.
Hazen, B. T., Skipper, J. B., Boone, C. A., & Hill, R. R. (2016). Back in business: Operations research in support of big data analytics for operations and supply chain management. Annals of Operations Research. https://doi.org/10.1007/s10479-016-2226-0.
Hermenegildo, M. V. (2012). Conferences vs. journals in CS, what to do? Evolutionary ways forward and the ICLP/TPLP model, Position paper for Dagstuhl meeting 12452: Publication Culture in Computing Research.
Huang, S., & Chaovalitwongse, W. A. (2015). Computational optimization and statistical methods for big data analytics: Applications in neuroimaging. INFORMS Tutorials in Operations Research. https://doi.org/10.1287/educ.2015.0135.
Ji-fan Ren, S., Fosso-Wamba, S., Akter, S., Dubey, R., & Childe, S. J. (2016). Modelling quality dynamics, business value and firm performance in a big data analytics environment. International Journal of Production Research. https://doi.org/10.1080/00207543.2016.1154209.
Jones, C., Hesterly, W. S., & Borgatti, S. P. (1997). A general theory of network governance: Exchange conditions and social mechanisms. Academy of Management Review, 22(4), 911–945.
Kovács, G., & Spens, K. M. (2009). Identifying challenges in humanitarian logistics. International Journal of Physical Distribution & Logistics Management, 39(6), 506–528.
Kovács, G., & Spens, K. M. (2011). Trends and developments in humanitarian logistics—A gap analysis. International Journal of Physical Distribution & Logistics Management, 41(1), 32–45.
Lantos, Geoffrey P. (2001). The boundaries of strategic corporate social responsibility. Journal of Consumer Marketing, 18(7), 595–632.
Li, L., Chi, T., Hao, T., & Yu, T. (2016). Customer demand analysis of the electronic commerce supply chain using big data. Annals of Operations Research. https://doi.org/10.1007/s10479-016-2342-x.
Liang, H., Saraf, N., Hu, Q., & Xue, Y. (2007). Assimilation of enterprise systems: The effect of institutional pressures and the mediating role of top management. MIS Quarterly, 31, 59–87.
Liu, P., & Yi, S. (2017). A study on supply chain investment decision-making and coordination in the big data environment. Annals of Operations Research. https://doi.org/10.1007/s10479-017-2424-4.
Ma, Y., & Zhang, H. (2017). Enhancing knowledge management and decision-making capability of China’s emergency operations center using big data. Intelligent Automation and Soft Computing, 1–8. https://doi.org/10.1080/10798587.2016.1267249
McIvor, R. (2009). How the transaction cost and resource-based theories of the firm inform outsourcing evaluation. Journal of Operations Management, 27, 45–63.
Meyerson, D., Weick, K. E., & Kramer, R. M. (1996). Swift trust and temporary groups. In R. M. Kramer & T. R. Tyler (Eds.), Trust in organizations: Frontiers of theory and research (pp. 166–195). Thousand Oaks: Sage.
Murphy, J., & Gouldson, A. (2000). Environmental policy and industrial innovation: Integrating environment and economy through ecological modernization. Geoforum, 31(1), 33–44.
Oliver, C. (1997). Sustainable competitive advantage: Combining institutional and resource based views. Strategic Management Journal, 18, 697–713.
Papadopoulos, T., Gunasekaran, A., Dubey, R., Altay, N., Childe, S. J., & Fosso-Wamba, S. (2017). The role of big data in explaining disaster resilience in supply chains for sustainability. Journal of Cleaner Production, 142, 1108–1118.
Park, J., Sarkis, J., & Wu, Z. (2010). Creating integrated business and environmental value within the context of China’s circular economy and ecological modernization. Journal of Cleaner Production, 18(15), 1494–1501.
Pfeffer, J., & Salancik, G. (1978). The external control of organizations: A resource dependence perspective. NewYork: Harper & Row.
Prasad, S., Zakaria, R., & Altay, N. (2016). Big data in humanitarian supply chain networks: A resource dependence perspective. Annals of Operations Research, 1–31. https://doi.org/10.1007/s10479-016-2280-7
Pu, C., & Kitsuregawa, M. (2013). Big data and disaster management—A report from the JST / NSF joint workshop. Technical report no. GIT-CERCS-13-09, Georgia Institute of Technology, CERCS.
Ramapriyan, H., Brennan, J., Walter, J., & Behnke, J. (2013). Managing big data: NASA tackles complex data challenges, Earth Imaging Journal. http://eijournal.com/print/articles/managing-bigdata?doing_wp_cron=1506950162.6216919422149658203125
Rao, P., & Holt, D. (2005). Do green supply chains lead to competitiveness and economic performance? International Journal of Operations and Production Management, 25(9), 898–916.
Rowley, T. (1997). Moving beyond dyadic ties: A network theory of stakeholder influences. Academy of Management Review, 22(4), 887–910.
Sarkis, J., Zhu, Q., & Lai, K. (2011). An organizational theoretic review of green supply chain management literature. International Journal of Production Economics, 130(1), 1–15.
Song, M. L., Fisher, R., Wang, J. L., & Cui, L. B. (2016). Environmental performance valuation with big data: Theories and methods. Annals of Operations Research. https://doi.org/10.1007/s10479-016-2158-8.
Spina, G., Caniato, F., Luzzini, D., & Ronchi, S. (2016). Assessing the use of external grand theories in purchasing and supply management research. Journal of Purchasing & Supply Management, 22, 18–30.
Starr, M. K., & Van Wassenhove, L. N. (2014). Introduction to the special Issue on humanitarian operations and crisis management. Production and Operations Management, 23(6), 925–937.
Sutton, R. I., & Staw, B. M. (1995). What theory is not. Administrative Science Quarterly, 40(3), 371–384.
Tatham, P., & Kovács, G. (2010). The application of ’swift trust’ to humanitarian logistics. International Journal of Production Economics, 126(1), 35–45.
TechCrunch. (2017). Facebook will share anonymized location data with disaster relief organizations. Posted on June 7, 2017 by Devin Coldewey. https://techcrunch.com/2017/06/07/facebook-will-share-anonymized-location-data-with-disaster-relief-organizations/. Accessed on July 20, 2017.
Thomas, A., & Mizushima, M. (2005). Logistics training: Necessity or luxury? Forced Migration Review, 22, 60–61.
Tranfield, D., Denyer, D., & Smart, P. (2003). Towards a methodology for developing evidence-informed management knowledge by means of systematic review. British Journal of Management, 14, 207–222.
Trunick, P. (2005). Special report: Delivering relief to tsunami victims. Logistics Today, 46(2), 1–3.
UNESCAP Report (2016). Disasters in Asia and the Pacific: 2015 year in review. A Report by United Nations Economic and Social Commission for Asia and the Pacific (UNESCAP).
Van Wassenhove, L. N. (2006). Blackett memorial lecture humanitarian aid logistics: Supply chain management in high gear. Journal of the Operational Research Society, 57, 475–489.
Weick, K. E., Sutcliffe, K. M., & Obstfeld, D. (1999). Organizing for high reliability. Research in Organizational Behavior, 21, 81–123.
Wernerfelt, B. (1984). A resource-based view of the firm. Strategic Management Journal, 5, 171–180.
Whetten, D. A. (1989). What constitutes a theoretical contribution? Academy of Management Review, 14(4), 490–495.
White, M. (2012). Digital workplaces: Vision and reality. Business Information Review, 29(4), 205–214.
Williamson, O. E. (2008). Outsourcing: Transaction cost economics and supply chain management. Journal of Supply Chain Management, 44(3), 5–16.
Wu, G. C., Ding, J., & Chen, P. S. (2012). The effects of GSCM drivers and institutional pressures on GSCM practices in Taiwan’s textile and apparel industry. International Journal of Production Economics, 135(2), 618–636.
Young-Ybarra, C., & Wiersema, M. (1999). Strategic flexibility in information technology alliances: The influence of transaction cost economics and social exchange theory. Organization Science, 10, 439–459.
Zhan, Y., Tan, K. H., Li, Y., & Tse, Y. K. (2016). Unlocking the power of big data in new product development. Annals of Operations Research. https://doi.org/10.1007/s10479-016-2379-x.
Zhu, Q., & Sarkis, J. (2004). Relationships between operational practices and performance among early adopters of green supply chain management practices in Chinese manufacturing enterprises. Journal of Operations Management, 22(3), 265–89.
Author information
Authors and Affiliations
Corresponding author
Appendices
Appendix A
Source title versus year.
Source title | 2005 | 2008 | 2009 | 2010 | 2012 | 2013 | 2014 | 2015 | 2016 | 2017 | Total |
---|---|---|---|---|---|---|---|---|---|---|---|
ACM transactions on embedded computing systems | 1 | 1 | |||||||||
Advances in intelligent and soft computing | 1 | 1 | |||||||||
Annals of operations research | 1 | 1 | |||||||||
Applied geography | 1 | 1 | |||||||||
Automation in construction | 1 | 1 | |||||||||
Big data | 1 | 1 | |||||||||
Computers and geosciences | 1 | 1 | |||||||||
CyberGEO | 1 | 1 | |||||||||
Environmental earth sciences | 1 | 1 | |||||||||
Eos | 1 | 1 | |||||||||
Expert systems with applications | 1 | 1 | |||||||||
IEEE Cloud computing | 1 | 1 | |||||||||
IEEE Transactions on smart grid | 1 | 1 | |||||||||
Intelligent automation and soft computing | 2 | 2 | |||||||||
International journal of critical infrastructures | 1 | 1 | 2 | ||||||||
International journal of digital earth | 1 | 1 | |||||||||
International journal of emergency management | 1 | 1 | |||||||||
Journal of applied remote sensing | 1 | 1 | |||||||||
Journal of cleaner production | 1 | 1 | |||||||||
Journal of decision systems | 1 | 1 | |||||||||
Journal of humanitarian logistics and supply chain management | 1 | 1 | |||||||||
Proceedings of the IEEE | 1 | 1 | |||||||||
Science China information sciences | 1 | 1 | |||||||||
SpringerPlus | 1 | 1 | |||||||||
Transportation research record | 1 | 1 | |||||||||
Wiley interdisciplinary reviews: Computational statistics | 1 | 1 | |||||||||
Total | 1 | 1 | 2 | 2 | 3 | 1 | 1 | 4 | 10 | 3 | 28 |
Appendix B
Classification of literature (from Fig. 4).
Theory building | Application based research | |||
---|---|---|---|---|
Rationalist approach | Alternative methods | Cases | ||
Theory building | Theory building and Theory testing | Critical review | Conceptual Framework through Survey | Industry focused research/ Survey to explore the existing scenario |
Amaye et al. (2016) | Papadopoulos et al. (2017), Prasad et al. (2016), Puthal et al. (2016) | Dusse et al. (2016), Wybo et al. (2015), Yang et al. (2013) | Bealt et al. (2016), Gao et al. (2008), Houghton et al. (2012), Ofli et al. (2016), Onorati and Diaz, (2016), Sarkar et al. (2014), Schultz, (2012), Thekdi and Joshi (2016), Wang et al. (2015), Wang et al. (2017), Zhu et al. (2016) | Chi et al. (2016), El-Anwar et al. (2009), El-Askary et al. (2012), Fan et al. (2009), Kulawiak et al. (2010), Lu et al. (2005), Lu et al. (2015), Ma and Zhang (2017), Moran et al. (2010), Onyewuchi et al. (2015) |
Appendix C
Enablers of big data and Humanitarian supply chain.
Enablers for big data and humanitarian supply chain | Measures | References |
---|---|---|
Volume | (1) Scale of data, (2) Stored data, (3) Continuous creation of data | Chi et al. (2016), Dusse et al. (2016), El-Askary et al. (2012), Fan et al. (2009), Gao et al. (2008), Kulawiak et al. (2010), Lu et al. (2005), Lu et al. (2015), Ma and Zhang (2017), Ofli et al. (2016), Onorati and Diaz (2016), Onyewuchi et al. (2015), Papadopoulos et al. (2017), Prasad et al. (2016), Puthal et al. (2016), Sarkar et al. (2014), Schultz (2012), Thekdi and Joshi (2016), Wang et al. (2015), Wang et al. (2017), Wybo et al. (2015), Yang et al. (2013), Zhu et al. (2016) |
Variety | (1) Data variety, (2) Interoperability | Amaye et al. (2016), Chi et al. (2016), El-Askary et al. (2012), Gao et al. (2008), Houghton et al. (2012), Kulawiak et al. (2010), Lu et al. (2005), Lu et al. (2015), Ofli et al. (2016), Onorati and Diaz, (2016), Papadopoulos et al. (2017), Prasad et al. (2016), Puthal et al. (2016), Sarkar et al. (2014), Thekdi and Joshi (2016), Wang et al. (2015), Wang et al. (2017), Wybo et al. (2015), Yang et al. (2013), Zhu et al. (2016) |
Velocity | (1) Speed of data generation, (2) Data processing time, (3) Data transmission time | Chi et al. (2016), Gao et al. (2008), Houghton et al. (2012), Kulawiak et al. (2010), Lu et al. (2015), Ofli et al. (2016), Onorati and Diaz (2016), Papadopoulos et al. (2017), Prasad et al. (2016), Puthal et al. (2016), Sarkar et al. (2014), Thekdi and Joshi (2016), Wang et al. (2015), Wybo et al. (2015) |
Veracity | (1) Quality of information, (2) Accuracy of data, (3) Reliability, (4) Accessibility | Amaye et al. (2016), Fan et al. (2009), Gao et al. (2008), Houghton et al. (2012), Lu et al. (2005), Lu et al. (2015), Ofli et al. (2016), Onorati and Diaz (2016), Papadopoulos et al. (2017), Prasad et al. (2016), Puthal et al. (2016), Sarkar et al. (2014), Thekdi and Joshi (2016), Wang et al. (2015), Wybo et al. (2015) |
Organizational mindfulness | (1) Preoocupation with failure, (2) Reluctance to simplify interpretations, (3) Senstivity to operations, (4) Commitment to resilience, (5) Deference to expertise | Amaye et al. (2016), El-Anwar et al. (2009), Ma and Zhang (2017), Moran et al. (2010), Papadopoulos et al. (2017), Prasad et al. (2016), Thekdi and Joshi (2016), Zhu et al. (2016) |
Concerns for big data in humanitarian supply chain.
Concerns for big data in humanitarian supply chain | Measures | References |
---|---|---|
Humanitarian logistics | (1) Identification of logistics service providers, (2) Collaboration between agencies during humanitarian operations, (3) Response time of logistics agencies/organizations | Bealt e al. (2016), Gao et al. (2008), Papadopoulos et al. (2017), Prasad et al. (2016), Thekdi and Joshi (2016), Zhu et al. (2016) |
Remote sensing | (1) Real time rendering of disaster location, (2) High-speed buffering mechanisms, (3) Large-scale 3D model visualization | Fan et al. (2009), Gao et al. (2008), Houghton et al. (2012), Kulawiak et al. (2010), Lu et al. (2015), Ofli et al. (2016), Wang et al. (2015) |
Information security | (1) Privacy and confidentiality of data, (2) Encryption, (3) Accountability, (4) Maintenance | Gao et al. (2008), Houghton et al. (2012), Kulawiak et al. (2010), Lu et al. (2015), Moran et al. (2010), Onorati and Diaz (2016), Papadopoulos et al. (2017), Prasad et al. (2016), Puthal et al. (2016), Wang et al. (2015), Yang et al. (2013) |
Social media (SM) | (1) Diffusion of information by SM, (2) Validation of information, (3) Support coordination and collaboration among agencies | Amaye et al. (2016); Ma and Zhang (2017), Ofli et al. (2016), Onorati and Diaz (2016), Papadopoulos et al. (2017), Sarkar et al. (2014), Wang et al. (2015), Wang et al. (2017), Wybo et al. (2015) |
Appendix D
Papers Considered for Literature Review
-
Amaye, A., Neville, K., & Pope, A. (2016). Big Promises: Using organisational mindfulness to integrate big data in emergency management decision making. Journal of Decision Systems, 25, 76–84. https://doi.org/10.1080/12460125.2016.1187419.
-
Bealt, J., Fernández Barrera, J. C., & Mansouri, S. A. (2016). Collaborative relationships between logistics service providers and humanitarian organizations during disaster relief operations. Journal of Humanitarian Logistics and Supply Chain Management, 6(2), 118–144. https://doi.org/10.1108/JHLSCM-02-2015-0008.
-
Chi, M., Plaza, A., Benediktsson, J. A., Sun, Z., Shen, J., & Zhu, Y. (2016). Big data for remote sensing: Challenges and opportunities. Proceedings of the IEEE, 104(11), 2207–2219. https://doi.org/10.1109/JPROC.2016.2598228.
-
Dusse, F., Júnior, P. S., Alves, A. T., Novais, R., Vieira, V., & Mendonça, M. (2016). Information visualization for emergency management: A systematic mapping study. Expert Systems with Applications, 45, 424–437. https://doi.org/10.1016/j.eswa.2015.10.007.
-
El-Anwar, O., El-Rayes, K., & Elnashai, A. (2009). An automated system for optimizing post-disaster temporary housing allocation. Automation in Construction, 18(7), 983–993. https://doi.org/10.1016/j.autcon.2009.05.003.
-
El-Askary, H., Allali, M., Rakovski, C., Prasad, A., Kafatos, M., & Struppa, D. (2012). Computational methods for climate data. Wiley Interdisciplinary Reviews: Computational Statistics, 4(4), 359–374. https://doi.org/10.1002/wics.1213.
-
Fan, X., Du, X., Tan, J., & Zhu, J. (2009). Three-dimensional visualization simulation assessment system based on multi-source data fusion for the wenchuan earthquake. Journal of Applied Remote Sensing, 3(1), 1–9. https://doi.org/10.1117/1.3154425.
-
Gao, S., Mioc, D., Yi, X., Anton, F., Oldfield, E., & Coleman, D. J. (2008). The Canadian geospatial data infrastructure and health mapping. CyberGEO, pp. 434.
-
Houghton, A., Prudent, N., Scott, J. E., Wade, R., & Luber, G. (2012). Climate change-related vulnerabilities and local environmental public health tracking through GEMSS: A web-based visualization tool. Applied Geography, 33(1), 36–44. https://doi.org/10.1016/j.apgeog.2011.07.014.
-
Kulawiak, M., Prospathopoulos, A., Perivoliotis, L., Łuba, M., Kioroglou, S., & Stepnowski, A. (2010). Interactive visualization of marine pollution monitoring and forecasting data via a web-based GIS. Computers and Geosciences, 36(8), 1069–1080. https://doi.org/10.1016/j.cageo.2010.02.008.
-
Lu, C., Sripada, L. N., Shekhar, S., & Liu, R. (2005). Transportation data visualisation and mining for emergency management. International Journal of Critical Infrastructures, 1(2-3), 170–194. https://doi.org/10.1504/IJCIS.2005.006118.
-
Lu, P., Wu, H., Qiao, G., Li, W., Scaioni, M., Feng, T. & Li, R. (2015). Model test study on monitoring dynamic process of slope failure through spatial sensor network. Environmental Earth Sciences, 74(4), 3315–3332. https://doi.org/10.1007/s12665-015-4369-8.
-
Ma, Y., & Zhang, H. (2017). Enhancing knowledge management and decision-making capability of China’s emergency operations center using big data. Intelligent Automation and Soft Computing, 1–8. https://doi.org/10.1080/10798587.2016.1267249.
-
Moran, A. P., Thieken, A. H., Schöbel, A., & Rachoy, C. (2010). Documentation of flood damage on railway infrastructure, in: Data and Mobility, edited by: Düh, J., Hufnagl, H., Juritsch, E., Pfliegl, R., Schimany, H.-K., and Schönegger, H., AISC 81, Heidelberg, 61–70. https://doi.org/10.1007/978-3-642-15503-1_6.
-
Ofli, F., Meier, P., Imran, M., Castillo, C., Tuia, D., Rey, N. & Joost, S. (2016). Combining human computing and machine learning to make sense of big (aerial) data for disaster response. Big Data, 4(1), 47–59. https://doi.org/10.1089/big.2014.0064.
-
Onorati, T., & Díaz, P. (2016). Giving meaning to tweets in emergency situations: A semantic approach for filtering and visualizing social data. SpringerPlus, 5(1), 1782. https://doi.org/10.1186/s40064-016-3384-x.
-
Onyewuchi, U. P., Shafieezadeh, A., Begovicieee, M. M., & Desroches, R. (2015). A probabilistic framework for prioritizing wood pole inspections given pole geospatial data. IEEE Transactions on Smart Grid, 6(2), 973–979. https://doi.org/10.1109/TSG.2015.2391183.
-
Papadopoulos, T., Gunasekaran, A., Dubey, R., Altay, N., Childe, S. J., & Fosso-Wamba, S. (2017). The role of big data in explaining disaster resilience in supply chains for sustainability. Journal of Cleaner Production, 142, 1108–1118. https://doi.org/10.1016/j.jclepro.2016.03.059.
-
Prasad, S., Zakaria, R., & Altay, N. (2016). Big data in humanitarian supply chain networks: A resource dependence perspective. Annals of Operations Research, pp. 1–31. https://doi.org/10.1007/s10479-016-2280-7.
-
Puthal, D., Nepal, S., Ranjan, R., & Chen, J. (2016). DLSeF: A dynamic key-length-based efficient real-time security verification model for big data stream. ACM Transactions on Embedded Computing Systems, 16(2), Article 51, 1–24. https://doi.org/10.1145/2937755.
-
Sarkar, S., Chatterjee, S., & Misra, S. (2014). Evacuation and emergency management using a federated cloud. IEEE Cloud Computing, 1(4), 68–76. https://doi.org/10.1109/MCC.2014.72.
-
Schultz, C. (2012). Extreme events and natural hazards: The complexity perspective. Eos, 93(44), 444. https://doi.org/10.1029/2012EO440015.
-
Thekdi, S. A., & Joshi, N. N. (2016). Risk-based vulnerability assessment for transportation infrastructure performance. International Journal of Critical Infrastructures, 12(3), 229–247. https://doi.org/10.1504/IJCIS.2016.079018.
-
Wang, W., Hu, C. B., Chen, N. C., Xiao, C. J., Wang, C., & Chen, Z. Q. (2015). Spatio-temporal enabled urban decision-making process modeling and visualization under the cyber-physical environment. Science China Information Sciences, 58(10), 1–17. https://doi.org/10.1007/s11432-015-5403-x.
-
Wang, X., Liu, Y., Zhang, H., Ma, Q., & Cao, Z. (2017). Public health emergency management and multi-source data technology in china. Intelligent Automation and Soft Computing, 1–8. https://doi.org/10.1080/10798587.2016.1267246.
-
Wybo, J. L., Fogelman-Soulié, F., Gouttas, C., Freyssinet, E., & Lions, P. (2015). Impact of social media in security and crisis management: A review. International Journal of Emergency Management, 11(2), 105–128. https://doi.org/10.1504/IJEM.2015.071045.
-
Yang, C., Xu, Y., & Nebert, D. (2013). Redefining the possibility of digital earth and geosciences with spatial cloud computing. International Journal of Digital Earth, 6(4), 297–312. https://doi.org/10.1080/17538947.2013.769783.
-
Zhu, Y., Ozbay, K., Xie, K., & Yang, H. (2016). Using big data to study resilience of taxi and subway trips for hurricanes sandy and Irene, Transportation Research Record, 2599, 70–80. https://doi.org/10.3141/2599-09.
Rights and permissions
About this article
Cite this article
Gupta, S., Altay, N. & Luo, Z. Big data in humanitarian supply chain management: a review and further research directions. Ann Oper Res 283, 1153–1173 (2019). https://doi.org/10.1007/s10479-017-2671-4
Published:
Issue Date:
DOI: https://doi.org/10.1007/s10479-017-2671-4