Abstract
Background
This study aimed at assessing the cross-sectional and longitudinal clinimetrics and feasibility of the Frontal Assessment Battery (FAB) in non-demented Parkinson’s disease (PD) patients.
Methods
N = 109 PD patients underwent the FAB and the Montreal Cognitive Assessment (MoCA). A subsample of patients further underwent a thorough motor, functional and behavioral evaluation (the last including measures of anxiety, depression and apathy). A further subsample was administered a second-level cognitive battery tapping on attention, executive functioning, language, memory, praxis and visuo-spatial abilities. The following properties of the FAB were tested: (1) concurrent validity and diagnostics against the MoCA; (2) convergent validity against the second-level cognitive battery; (4) association with motor, functional and behavioral measures; (5) capability to discriminate patients from healthy controls (HCs; N = 96); (6) assessing its test–retest reliability, susceptibility to practice effects and predictive validity against the MoCA, as well as deriving reliable change indices (RCIs) for it, at a ≈ 6-month interval, within a subsample of patients (N = 33).
Results
The FAB predicted MoCA scores at both T0 and T1, converged with the vast majority of second-level cognitive measures and was associated with functional independence and apathy. It accurately identified cognitive impairment (i.e., a below-cut-off MoCA score) in patients, also discriminating patients from HCs. The FAB was reliable at retest and free of practice effects; RCIs were derived according to a standardized regression-based approach.
Discussion
The FAB is a clinimetrically sound and feasible screener for detecting dysexecutive-based cognitive impairment in non-demented PD patients.
Similar content being viewed by others
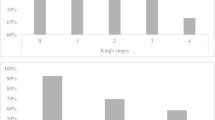
Avoid common mistakes on your manuscript.
Background
In Parkinson’s disease (PD) patients, executive functioning (EF) deficits represent an early, major driver of cognitive impairment (Kudlicka et al. 2011), detrimentally affect functional (Cahn et al. 1998; Puente et al. 2016; Vlagsma et al. 2017) and motor outcomes (Amboni et al. 2008; Smulders et al. 2013; Chung et al. 2021) and may predict incident dementia (Paulwoods and Tröster 2003). Hence, a timely detection of dysexecutive-based cognitive inefficiency via clinimetrically sound and feasible screeners is clinically pivotal in this population (Kudlicka et al. 2011; Rodriguez-Oroz et al. 2009). In addition, cognitive screening measures are often employed within clinical trials targeting both motor and non-motor features of PD (Chou et al. 2010; Litvan et al. 2018; Skorvanek et al. 2018).
Among performance-based screeners, the Frontal Assessment Battery (FAB) (Dubois et al. 2000) has proved clinimetrically sound and feasible to the aim of detecting dysexecutive-based cognitive inefficiency in PD patients at an international level (Kulisevsky and Pagonabarraga 2009; Hurtado-Pomares et al. 2018), with evidence being also available on its association with motor outcomes (Marconi et al. 2012; Kataoka et al. 2014; Varalta et al. 2015).
Within the Italian scenario, evidence has been provided on the diagnostic soundness of the FAB (Appollonio et al. 2005) to the aim of identifying, in PD patients, both mild cognitive impairment (PD-MCI) and dementia (PD-D)—with ad hoc cut-offs having been also delivered for both syndromes (Biundo et al. 2013, 2014; Federico et al. 2017). Furthermore, the Italian FAB Appollonio et al. (Appollonio et al. 2005) was shown to be related to and/or predictive of motor outcomes in PD patients (Amboni et al. 2008; Marconi et al. 2012; Varalta et al. 2015).
Nevertheless, no study has thus far specifically focused on either the psychometrics or the cross-sectional and longitudinal feasibility of the Italian FAB in this population—in spite of such features being critical to determine whether a cognitive screener is adequately recommended for use in clinical practice and research (Aiello et al. 2022a). In addition, previous Italian reports on the diagnostics of the FAB (Biundo et al. 2013, 2014; Federico et al. 2017) did not deliver its likelihood ratios – although such metrics are pivotal towards clinical decision-making (Larner 2021).
Given the above premises, the present study focused on filling a number of gaps within the Italian literature on the clinimetrics and feasibility of the FAB in PD patients. More specifically, the following aims were pursued—by addressing an Italian cohort of non-demented PD patients: (1) testing the concurrent validity of the FAB against a measure of global cognitive efficiency; (2) performing and in-depth evaluation of its diagnostic properties; (3) testing its convergence against a second-level cognitive battery; (4) exploring its association with measures of functional independence, motor abilities and behavioral status; (5) examining its capability to discriminate patients from healthy controls (HCs); (6) assessing its test–retest reliability, susceptibility to practice effects and predictive validity, as well as (7) deriving reliable change indices (RCIs) for it, at a ≈ 6-month interval.
Methods
Participants
Data on N = 109 PD patients (Postuma et al. 2015) consecutively referred to IRCCS Istituto Auxologico Italiano, Milano, Italy and IDC-Hermitage Capodimonte, Napoli, Italy between 2013 and 2019 and having been administered the FAB (Appollonio et al. 2005) were retrospectively collected. No patient presented with dementia due to PD, as defined according to the DSM-V criteria Psychiatric and Association (2013). Out of these patients, N = 33 were followed-up with the FAB at a 5-to-8-month interval (Mdn = 6.5). Additionally, N = 96 HCs were prospectively recruited via advertising and Authors’ personal acquaintances. Both patients and HCs were free of 1) neurological/psychiatric disorders (other than PD), 2) severe general-medical conditions possibly entailing encephalopathic features and 3) uncorrected hearing/vision deficits. Additionally, HCs had an above cut-off, age- and education-adjusted score on the Montreal Cognitive Assessment (MoCA) (Aiello et al. 2022b). This study received ethical approval, participants provided informed consent and data were treated according to current regulations.
Materials
All participants were administered the Italian versions of the FAB (Kulisevsky and Pagonabarraga 2009) and MoCA (Aiello et al. 2022b).
A subsample of patients (N = 73) further underwent motor-functional (i.e., Unified Parkinson’s Disease Rating Scale, UPDRS (1987),Schwab and England Scale, SES (1969), Modified Hoehn–Yahr Scale, H–Y (Jankovic et al. 1990) and behavioral assessments (i.e., State- and Trait-Anxiety Inventory-Form Y, STAI-Y1/-Y2 (Spielberger et al. 1971), Beck Depression Inventory (BDI) (Beck et al. 1961), Dimensional Apathy Scale (DAS) (Santangelo et al. 2017), while another subsample (N = 36) was administered a second-level cognitive battery targeting attention and EF (Trail-Making Test (Giovagnoli et al. 1996), Stroop Color–Word Test (Barbarotto et al. 1998), Phonemic Verbal Fluency (Carlesimo et al. 1996), Raven’s Colored Progressive Matrices (Caltagirone et al. 1979),Backward Digit Span (Monaco et al. 2013), memory (Rey Auditory Verbal Learning Test (Carlesimo et al. 1996), Babcock Memory Test (Novelli et al. 1986), language (Noun- and Verb-naming tasks from the Esame Neuropsicologico per l’Afasia (2001), Semantic Verbal Fluency (Spinnler and Tognoni 1987), praxis (Design Copy (Spinnler and Tognoni 1987) and visuo-spatial abilities (Benton Judgment of Line Orientation (Benton et al. 1978).
Patients undergoing the follow-up were re-administered both the FAB and the MoCA.
Statistics
Assumption checks
Normality was checked for on raw variables by addressing skewness and kurtosis values which were deemed as abnormal if >|1| and |3|, respectively (Kim 2013). Accordingly, either linear models or non-parametric techniques were employed to test associations/predictions of interest.
Outcome measure for criterion validity and diagnostic analyses
Within concurrent/predictive validity and diagnostic analyses, the MoCA was addressed as the outcome in the light of the fact that the Movement Disorders Society (MDS) strongly recommended it for use in PD patients (Skorvanek et al. 2018), as well as that such a screener was shown to be “executively-loaded” (Aiello et al. 2022b)—thus effectively working as an outcome for testing the diagnostics of the FAB within both normotypical (Aiello et al. 2022c) and clinical populations (Solca et al. 2022).
Cross-sectional clinimetric analyses
The concurrent validity of the FAB was tested against the MoCA via a multiple linear regression while covarying for age, sex, education, disease duration (in months) as well as UPDRS, SES and H–Y scores.
The construct validity of the FAB was tested against each measure of the second-level cognitive battery via Bonferroni-corrected Spearman’s partial correlations while covarying for age, education and sex.
The diagnostics of the FAB were examined via receiver-operating characteristics (ROC) analyses by addressing a below-cut-off, age- and education-adjusted MoCA score (Aiello et al. 2022b) as the positive state. Sensitivity (Se), specificity (Sp), positive and negative predictive values (PPV, NPV) and likelihood ratios (LR + , LR−) were computed at the optimal cut-off identified via Youden’s J statistic. LR + values ≥ 2 and LR− ones ≤ 0.5 were deemed as optimal (Larner 2021). The number needed for screening utility (NNSU) was also computed—with a value ≤ 1.02 being deemed as indexing an optimal screening performance (Larner 2019). Such diagnostics were tested both on raw and on age- and education-adjusted (Appollonio et al. 2005) FAB scores.
Cross-sectional feasibility analyses
Spearman’s partial correlation was employed to test the association between the FAB and the SES net of age, sex, education, disease duration and UPDRS and H-Y scores. Similarly, Bonferroni-corrected, Spearman’s partial correlations were run to explore the association between the FAB and behavioral measures (i.e., STAI-Y1, STAI-Y2, BDI and DAS scores)—while partialling out age, sex, education, disease duration and UPDRS and H–Y scores –, as well as between the FAB and motor measures (i.e., UPDRS-II, -III, -IV and H-Y scores)—while partialling out age, sex, education and disease duration.
Case–control discrimination was tested via a logistic regression addressing adjusted FAB scores as the predictor and group as the outcome, while covarying for sex—since the two groups were unmatched for this variable (sex: χ2(1) = 22.84, p < 0.001).
Longitudinal clinimetric and feasibility analyses
Test–retest reliability and practice effects were tested on T0 and T1 adjusted FAB scores via an intra-class correlation (ICC) coefficient and a dependent-sample t-test, respectively.
The predictive validity of T0 FAB scores were tested against T1 MoCA scores via a multiple linear regression while covarying for sex, education, T0 age, T0 disease duration as well as T0 UPDRS, SES and H–Y scores.
RCIs for both raw and adjusted FAB scores were derived via a standardized regression-based (SRB) approach (Duff 2012). The regression model for deriving RCIs on raw FAB scores encompassed as predictors T0 FAB scores, education, retest interval (in months), T0 age, T0 disease duration as well as T0 UPDRS and H-Y score. As to the model addressing adjusted FAB scores, the same set of predictors was kept by nevertheless dropping education and T0 age. A z-deviate >|1.645| was deemed as suggestive of clinically meaningful changes.
Software
Analyses were run with R 4.1 (https://cran.r-project.org/) and jamovi 2.3 (the jamovi project, 2022).
Results
Cross-sectional clinimetrics and feasibility
Table 1 summarizes the participants’ background, clinical, and neuropsychological measures.
The FAB was moderately predictive of MoCA scores (β = 0.39; t(65) = 3.85; p < 0.001), uniquely accounting for 12.6% of their variance (η2 = 0.13). Consistently, the FAB proved to be associated with the vast majority of second-level cognitive measures (Table 2).
20 out of 109 patients (18.3%) performed defectively on the MoCA. In identifying them, both raw (AUC = 0.88; SE = 0.05; CI 95% [0.78, 0.97]) and adjusted FAB scores (AUC = 0.88; SE = 0.04; CI 95% [0.8, 0.96]) proved to be highly accurate, also coming with sound diagnostics (Table 3) at the optimal cut-offs of ≤ 13 (J = 0.65) and < 13.25 (J = 0.65), respectively. The agreement rate between the classifications yielded by the raw and the adjusted cut-off was of 96% (Cohen’s k = 0.90; z = 9.45; p < 0.001), with discrepancies being mostly accounted for by the fact that the raw cut-off classified as impaired a slightly higher number of patients than the adjusted one (26.6% vs. 24.8%).
The FAB was positively related to SES scores (rs(72) = 0.33; p = 0.007). At variance, no association was detected, at αadjusted = 0.013, with motor measures (p ≥ 0.103). As to the association with behavioral measures, at αadjusted = 0.013, FAB scores proved to be inversely related to the DAS (rs(72) = -0.47; p < 0.001) and, albeit to a lesser extent, to the STAI-Y1 (rs(72) = -0.31; p = 0.011), but not with remaining measures (p ≥ 0.561).
The FAB was able to discriminate PD patients from HCs (b = -0.61; z = -5.36; p < 0.001; OR = 0.54, CI 95% [0.43, 0.68]).
Longitudinal clinimetrics and feasibility
Table 4 summarizes background, clinical and cognitive measures of the subsample of patients that underwent the follow-up.
The FAB proved to be moderately reliable at retest (ICC = 0.7) and not subjected to practice effects (t(32) = − 1.78; p = 0.084).
Baseline FAB scores were moderately predictive of MoCA scores at follow-up (β = 0.47; t(25) = 2.92; p = 0.007), uniquely accounting for 16.3% of their variance (η2 = 0.16).
The SRB revealed that T0 FAB scores were the only significant predictor of T1 FAB scores within both the model addressing raw scores (β = 0.47; t(25) = 2.92; p = 0.007) and that addressing adjusted scores (β = 0.47; t(25) = 2.92; p = 0.007). Table 5 reports the metrics for deriving RCIs on both raw and adjusted FAB scores, while an automated sheet for their computation is delivered within the Supplementary Material 1.
Discussion
The present study provides Italian practitioners and clinical researchers with further, and mostly unprecedented, evidence on the clinimetric soundness and feasibility of the FAB in non-demented PD patients—which adds to that already available on the topic (Amboni et al. 2008; Marconi et al. 2012; Varalta et al. 2015; Biundo et al. 2013, 2014; Federico et al. 2017). More specifically, it has herewith shown that, in non-demented PD patients, the FAB is (1) concurrently and predictively valid against a first-level measures of cognitive efficiency, (2) convergently valid against second-level cognitive measures, (3) diagnostically sound in the view of detecting cognitive impairment, 4) able to discriminate PD patients from HCs, (5) associated with functional independence and apathy measures, (5) reliable and not susceptible to practice effects at a 5-to-8-month interval. Additionally, SRB RCIs for the FAB have been derived within the present investigations—which can be adopted for longitudinally monitoring PD patients’ EF-based cognitive efficiency at a 5- to 8-month interval.
Relevantly, this report complements, at least to an extent, the 2018 call by the MDS for clinimetrically sound and feasible cognitive screeners in this population (Skorvanek et al. 2018): in fact, such MDS guidelines intentionally did not focus on the FAB given its domain-specificity. By contrast, the present study.
As to its criterion validity, the FAB proved to be both concurrently and predictively valid against the MoCA—this suggesting that FAB scores represent a valid estimate of executive-based cognitive efficiency in PD patients, which also appeared temporally stable over a 5- to 8-month timespan. Moreover, the FAB was found to be associated with several second-level measures of both attention/EF and instrumental cognitive domains (i.e., language, memory, visuo-spatial abilities and praxis) – this further supporting the notion of such a screener being an actual measure of executive-based cognitive efficiency.
The present investigation aligns with prior studies demonstrating the diagnostic soundness of the FAB in PD patients (Biundo et al. 2013, 2014; Federico et al. 2017), complementing them with additional, previously unreported metrics—namely, likelihood ratios and the NNSU. Albeit both the raw and adjusted cut-off herewith delivered proved to come with optimal diagnostics, the cut-off identified on adjusted scores happened to outperform that identified on raw scores as far as both individual—i.e., Se, PPV and likelihood ratios—and unitary metrics—i.e., Youden’s J statistic and NNSU—were concerned. Hence, a FAB score adjusted for age and education – according to Appollonio et al.’s (Appollonio et al. 2005) normative dataset—that falls below 13.25 is herewith suggested to be addressed in the view of identifying cognitive impairment in non-demented PD patients. Interestingly, such a threshold appeared to be looser when compared to the optimal cut-offs previously provided by Biundo et al. (Biundo et al. 2013) for identifying both PD-MCI—i.e., < 15.1 – and PD-D—i.e., < 13.7 –, despite all of them having been derived on the basis of the same normative dataset (Appollonio et al. 2005). Hence, such discrepancies are likely to be accounted for by the different operationalization of the positive state—represented, within Biundo et al.’s (Biundo et al. 2013) study, by PD-MCI/PD-D clinical diagnoses made on the basis of a full cognitive battery, which, however, also included the FAB, while, within the present one, by a defective performance on an independent test (namely, the MoCA).
It is worth noting that, within this report, the FAB proved to be associated with functional independence—even while covarying for demographics and disease-related, both motor and non-motor burden. Such a finding is supported by previous studies showing that both objective and subjective EF measures are associated with/predict a wide range of ecological outcomes in PD—e.g., quality of life and involvement in daily-life activities (Cahn et al. 1998; Puente et al. 2016; Vlagsma et al. 2017).
As to the association between the FAB and behavioral measures, the finding of it being related to “pure” apathetic features is fully in line with the current literature on the topic (D’Iorio et al. 2018). By contrast, the present investigation does not support the notion of depression and state-anxiety being linked to dysexecutive features in this population (Petkus et al. 2020), rather aligning with the view of apathy being, among all behavioral dysfunctions, the one mostly linked to worse cognitive outcomes (Varanese et al. 2011). As to the mild, inverse association that was detected between the FAB and the STAI-Y1, it is not unreasonable to hypothesize that it might have reflected a performance-related, situational anxiety state rather than an actual contribution of anxiety to EF.
Finally, the present study also specifically addressed the longitudinal feasibility of the FAB in non-demented PD patients—showing that it is free of practice effects and reliable at a retest interval of ≈ 6 months. Additionally, SRB RCIs have been herewith provided for this screener in the view of improving its adoption for repeated assessments over time in this population. With this last regard, users have to be nonetheless aware of the fact that such RCIs can be adopted only provided that the retest interval is comprised between 5 and 8 months.
This study is of course not free of limitations. First, a number of cross-sectional clinimetrics for the FAB in PD still need to be tested—namely, factorial and ecological validity, internal consistency as well as test–retest and inter-rater reliability. Similarly, further investigations should focus on testing its sensitivity to change over time by addressing longer retest intervals—e.g., 12 or 24 months. Second, the present investigation solely addressed PD patients without dementia—this partially limiting the generalizability of the findings herewith reported to the full cognitive spectrum of PD. Third, it has to be noted that, albeit age and sex were covaried within the case–control discrimination analysis, PD patients and HCs substantially differed as to such demographics: hence, future studies aimed at replicating such findings by nonetheless matching PD patients to HCs as to age and sex are advisable. Fourth, the present report, due to its retrospective nature, did not specifically stratify patients according to MDS criteria for mild cognitive impairment in PD (Litvan et al. 2012)—which is, however, an element that deserves attention by future studies on the topic. Finally, it is worth mentioning that the FAB, albeit able to detect overall cognitive inefficiency of a dysexecutive type, does not tap on memory functions—whose prognostic value towards dementia conversion has been demonstrated in PD (Gasca-Salas et al. 2020). Although EF deficits too have been recently identified as useful markers to predict dementia conversion in PD (Wallace et al. 2022), the assessment of memory should be nonetheless taken into account by practitioners.
In conclusion, the present study confirms that the FAB is a clinimetrically sound and feasible screener for detecting dysexecutive-based cognitive impairment in non-demented PD patients.
Data availability
Datasets associated with the present study are available upon reasonable request of interested researchers at the following link: https://zenodo.org/record/7774009#.ZCFpDnZBxPZ.
Change history
04 September 2023
A Correction to this paper has been published: https://doi.org/10.1007/s00702-023-02690-x
References
Aiello EN, Rimoldi S, Bolognini N, Appollonio I, Arcara G (2022a) Psychometrics and diagnostics of Italian cognitive screening tests: a systematic review. Neurol Sci 43:821–845
Aiello EN, Gramegna C, Esposito A, Gazzaniga V, Zago S, Difonzo T, Bolognini N (2022b) The montreal cognitive assessment (MoCA): updated norms and psychometric insights into adaptive testing from healthy individuals in Northern Italy. Aging Clin Exp Res 34: 375–382.
Aiello EN, Esposito A, Appollonio I, Bolognini N (2022c) Diagnostic properties of the Frontal Assessment Battery (FAB) in Italian healthy adults. Aging Clin Exp Res 34:1021–1026
Amboni M, Cozzolino A, Longo K, Picillo M, Barone P (2008) Freezing of gait and executive functions in patients with Parkinson’s disease. Mov Disord 23:395–400
American Psychiatric Association (2013) Diagnostic and statistical manual of mental disorders (5th ed.)
Appollonio I, Leone M, Isella V, Piamarta F, Consoli T, Villa ML, Nichelli P (2005) The frontal assessment battery (FAB): normative values in an Italian population sample. Neurol Sci 26:108–116
Barbarotto R, Laiacona M, Frosio R, Vecchio M, Farinato A, Capitani E (1998) A normative study on visual reaction times and two Stroop colour-word tests. Italian J Neurol Sci 19:161–170
Beck AT, Ward CH, Mendelson M, Mock J, Erbaugh J (1961) An inventory for measuring depression. Arch Gen Psychiatry 4:561–571
Benton AL, Varney NR, Hamsher KD (1978) Visuospatial judgment: a clinical test. Arch Neurol 35:364–367
Biundo R, Weis L, Facchini S, Formento-Dojot P, Vallelunga A, Pilleri M, Antonini A (2014) Cognitive profiling of Parkinson disease patients with mild cognitive impairment and dementia. Parkinsonism Relat Disord 20:394–399
Biundo R, Weis L, Pilleri M, Facchini S, Formento-Dojot P, Vallelunga A, Antonini A (2013) Diagnostic and screening power of neuropsychological testing in detecting mild cognitive impairment in Parkinson’s disease. J Neural Transm 120:627–633
Cahn DA, Sullivan EV, Shear PK, Pfefferbaum A, Heit G, Silverberg G (1998) Differential contributions of cognitive and motor component processes to physical and instrumental activities of daily living in Parkinson’s disease. Arch Clin Neuropsychol 13:575–583
Caltagirone C, Gainotti G, Masullo C, Miceli G (1979) Validity of some neuropsychological tests in the assessment of mental deterioration. Acta Psychiatr Scand 60:50–56
Capasso R, Miceli G (2001) Esame Neuropsicologico per l'Afasia: ENPA . Springer Science & Business Media
Carlesimo GA, Caltagirone C, Gainotti G (1996) The mental deterioration battery: normative data, diagnostic reliability and qualitative analyses of cognitive impairment. Eur Neurol 36:378–384
Chou KL, Amick MM, Brandt J, Camicioli R, Frei K, Gitelman D (2010) A recommended scale for cognitive screening in clinical trials of Parkinson’s disease. Movem Disord 25:2501–2507
Chung SJ, Yoo HS, Lee HS, Lee YH, Baik K, Jung JH, Lee PH (2021) Baseline cognitive profile is closely associated with long-term motor prognosis in newly diagnosed Parkinson’s disease. J Neurol 268:4203–4212
Dubois B, Slachevsky A, Litvan I, Pillon B (2000) The FAB: a frontal assessment battery at bedside. Neurology 55:1621–1626
Duff K (2012) Evidence-based indicators of neuropsychological change in the individual patient: relevant concepts and methods. Arch Clin Neuropsychol 27:248–261
D’Iorio A, Maggi G, Vitale C, Trojano L, Santangelo G (2018) “Pure apathy” and cognitive dysfunctions in Parkinson’s disease: a meta-analytic study. Neurosci Biobehav Rev 94:1–10
Fahn S, Elton RL, UPDRS program members (1987) Unified Parkinson’s disease rating scale. In: Fahn S, Marsden CD, Goldstein M, Calne DB (Eds) Recent developments in Parkinson’s disease, pp. 153–163
Federico A, Trentin M, Zanette G, Mapelli D, Picelli A, Smania N, Tamburin S (2017) Diagnosing mild cognitive impairment in Parkinson’s disease: which tests perform best in the Italian population? Neurol Sci 38:1461–1468
Gasca‐Salas, C., Duff‐Canning, S., Armstrong, M. J., Eslinger, P. J., Schneider, R. B., Kennedy, N., ... & Marras, C. (2020). Parkinson disease with mild cognitive impairment: Domain‐specific cognitive complaints predict dementia. Acta Neurologica Scandinavica, 142, 585–596.
Giovagnoli AR, Del Pesce M, Mascheroni S, Simoncelli M, Laiacona M, Capitani E (1996) Trail making test: normative values from 287 normal adult controls. Neurol Sci 17:305–309
Hurtado-Pomares M, Carmen Terol-Cantero M, Sánchez-Pérez A, Peral-Gómez P, Valera-Gran D, Navarrete-Muñoz EM (2018) The frontal assessment battery in clinical practice: a systematic review. Int J Geriatr Psychiatry 33:237–251
Jankovic J, McDermott M, Carter J, Gauthier S, Goetz C, Golbe L, Parkinson Study Group (1990) Variable expression of Parkinson’s disease: a base-line analysis of the DAT ATOP cohort. Neurology 40:1529–1529
Kataoka H, Tanaka N, Saeki K, Kiriyama T, Ueno S (2014) Low frontal assessment battery score as a risk factor for falling in patients with Hoehn-Yahr Stage III Parkinson’s disease: a 2-year prospective study. Eur Neurol 71:187–192
Kim HY (2013) Statistical notes for clinical researchers: assessing normal distribution (2) using skewness and kurtosis. Restor Dent Endod 38:52–54
Kudlicka A, Clare L, Hindle JV (2011) Executive functions in Parkinson’s disease: systematic review and meta-analysis. Mov Disord 26:2305–2315
Kulisevsky J, Pagonabarraga J (2009) Cognitive impairment in Parkinson’s disease: tools for diagnosis and assessment. Mov Disord 24:1103–1110
Larner AJ (2019) New unitary metrics for dementia test accuracy studies. Prog Neurol Psychiatry 23:21–25
Larner AJ (2021b). Paired measures. In: Larner AJ (ed) The 2x2 Matrix. Contingency, Confusion, and the Metrics of Binary Classification, pp. 15–48
Litvan I, Goldman JG, Tröster AI, Schmand BA, Weintraub D, Petersen RC, Emre M (2012) Diagnostic criteria for mild cognitive impairment in Parkinson’s disease: movement disorder society task force guidelines. Movem Disord 27:349–356
Litvan I, Kieburtz K, Tröster AI, Aarsland D (2018) Strengths and challenges in conducting clinical trials in Parkinson’s disease mild cognitive impairment. Mov Disord 33:520–527
Marconi R, Antonini A, Barone P, Colosimo C, Avarello TP, Bottacchi E, Morgante L (2012) Frontal assessment battery scores and non-motor symptoms in parkinsonian disorders. Neurol Sci 33:585–593
Monaco M, Costa A, Caltagirone C, Carlesimo GA (2013) Forward and backward span for verbal and visuo-spatial data: standardization and normative data from an Italian adult population. Neurol Sci 34:749–754
Novelli G, Papagno C, Capitani E, Laiacona M, Cappa SF, Vallar G (1986) Tre test clinici di ricerca e produzione lessicale. Taratura su sogetti normali. Arch Psicol Neurol Psichiatr 47:278–296
Paulwoods S, Tröster AI (2003) Prodromal frontal/executive dysfunction predicts incident dementia in Parkinson’s disease. J Int Neuropsychol Soc 9:17–24
Petkus AJ, Filoteo JV, Schiehser DM, Gomez ME, Hui JS, Jarrahi B, Petzinger GM (2020) Mild cognitive impairment, psychiatric symptoms, and executive functioning in patients with Parkinson’s disease. Int J Geriat Psychiatry 35:396–404
Postuma RB, Berg D, Stern M, Poewe W, Olanow CW, Oertel W, Deuschl, G (2015) MDS clinical diagnostic criteria for Parkinson's disease. Movem Disord 30: 1591–1601.
Puente AN, Cohen ML, Aita S, Brandt J (2016) Behavioral ratings of executive functioning explain instrumental activities of daily living beyond test scores in Parkinson’s disease. Clin Neuropsychol 30:95–106
Rodriguez-Oroz MC, Jahanshahi M, Krack P, Litvan I, Macias R, Bezard E, Obeso JA (2009) Initial clinical manifestations of Parkinson’s disease: features and pathophysiological mechanisms. Lancet Neurol 8:1128–1139
Santangelo G, Raimo S, Siciliano M, D’Iorio A, Piscopo F, Cuoco S, Trojano L (2017) Assessment of apathy independent of physical disability: validation of the Dimensional Apathy Scale in Italian healthy sample. Neurol Sci 38:303–309
Schwab RS, England AC (1969) Projection technique for evaluating surgery in Parkinson’s disease. In: Gillingham FJ, Donaldson MC (eds) Third Symposium on Parkinson’s Disease, pp. 152–157
Skorvanek M, Goldman JG, Jahanshahi M, Marras C, Rektorova I, Schmand B, Violante MR (2018) Global scales for cognitive screening in Parkinson’s disease: critique and recommendations. Movem Disord 33:208–218
Smulders K, van Nimwegen M, Munneke M, Bloem BR, Kessels RP, Esselink RA (2013) Involvement of specific executive functions in mobility in Parkinson’s disease. Parkinsonism Relat Disord 19:126–128
Solca F, Aiello EN, Migliore S, Torre S, Carelli L, Ferrucci R, Poletti B (2022) Diagnostic properties of the frontal assessment battery (FAB) in Huntington’s disease. Front Psychol 13:1031871
Spielberger CD, Gonzalez-Reigosa F, Martinez-Urrutia A, Natalicio LF, Natalicio DS (1971) The state-trait anxiety inventory. Interam J Psychol 5:145–158
Spinnler H, Tognoni G (1987) Standardizzazione e taratura italiana di una batteria di test neuropsicologici. Neurol Sci 6:S1–S120
Varalta V, Picelli A, Fonte C, Amato S, Melotti C, Zatezalo V, Smania N (2015) Relationship between cognitive performance and motor dysfunction in patients with Parkinson’s disease: a pilot cross-sectional study. BioMed Res Int 2015:365959
Varanese S, Perfetti B, Ghilardi MF, Di Rocco A (2011) Apathy, but not depression, reflects inefficient cognitive strategies in Parkinson’s disease. PLoS ONE 6:e17846
Vlagsma TT, Koerts J, Tucha O, Dijkstra HT, Duits AA, van Laar T, Spikman JM (2017) Objective versus subjective measures of executive functions: predictors of participation and quality of life in Parkinson disease? Arch Phys Med Rehabil 98:2181–2187
Wallace ER, Segerstrom SC, van Horne CG, Schmitt FA, Koehl LM (2022) Meta-analysis of cognition in Parkinson’s disease mild cognitive impairment and dementia progression. Neuropsychol Rev 32:149–160
Acknowledgements
The authors are thankful to patients and their caregivers. Roberta Ferrucci was supported from the Ravelli Research Center (CRC) for Neurotechnology and Brain Therapeutics.
Funding
This research was funded by the Italian Ministry of Health to IRCCS Istituto Auxologico Italiano (Ricerca Corrente, project 23C923) and partially supported by the Italian Ministry of Health to Fondazione IRCCS Ca’ Granda Ospedale Maggiore Policlinico.
Author information
Authors and Affiliations
Corresponding author
Ethics declarations
Conflict of interest
V. S. received compensation for consulting services and/or speaking activities from AveXis, Cytokinetics, Italfarmaco, Liquidweb S.r.l., Novartis Pharma AG and Zambon, receives or has received research supports from the Italian Ministry of Health, AriSLA, and E-Rare Joint Transnational Call. He is in the Editorial Board of Amyotrophic Lateral Sclerosis and Frontotemporal Degeneration, European Neurology, American Journal of Neurodegenerative Diseases, Frontiers in Neurology and Exploration of Neuroprotective Therapy. B.P. received compensation for consulting services and/or speaking activities from Liquidweb S.r.l B.P is Associated Editor for Frontiers in Neuroscience. N. T. received compensation for consulting services from Amylyx Pharmaceuticals and Zambon Biotech SA. He is Associate Editor for Frontiers in Aging Neuroscience.
Ethical approval
This study was approved by the Ethics Committees of IRCCS Istituto Auxologico Italiano (I.D.: 23C923_2019) and University of Campania “Luigi Vanvitelli”.
Informed consent
Participants provided informed consent.
Additional information
Publisher's Note
Springer Nature remains neutral with regard to jurisdictional claims in published maps and institutional affiliations.
Supplementary Information
Below is the link to the electronic supplementary material.
Rights and permissions
Springer Nature or its licensor (e.g. a society or other partner) holds exclusive rights to this article under a publishing agreement with the author(s) or other rightsholder(s); author self-archiving of the accepted manuscript version of this article is solely governed by the terms of such publishing agreement and applicable law.
About this article
Cite this article
Aiello, E.N., D’Iorio, A., Solca, F. et al. Clinimetrics and feasibility of the Italian version of the Frontal Assessment Battery (FAB) in non-demented Parkinson’s disease patients. J Neural Transm 130, 687–696 (2023). https://doi.org/10.1007/s00702-023-02624-7
Received:
Accepted:
Published:
Issue Date:
DOI: https://doi.org/10.1007/s00702-023-02624-7