Abstract
This paper studies the emissions of SO2 and COD in China using fine-scale, countylevel data. Using a widely used spatial autocorrelation index, Moran’s I statistics, we first estimate the spatial autocorrelations of SO2 and COD emissions. Distinct patterns of spatial concentration are identified. To investigate the driving forces of emissions, we then use spatial econometric models, including a spatial error model (SEM) and a spatial lag model (SLM), to evaluate the effects of variables that reflect level of economic development, population density, and industrial structure. Our results show that these explanatory variables are highly correlated with the level of SO2 and COD emissions, though their impacts on SO2 and COD vary. Compared to ordinary least square regression, the advantages of SLM and SEM are demonstrated as they effectively reveal the existence and significance of spatial dependence. The SEM, in particular, is chosen over the SLM as the role of spatial correlation is stronger in the error model than in the lag model. Based on the research results, we present some preliminary policy recommendations, especially for those high–high cluster regions that face significant environmental degradation and challenge.
Similar content being viewed by others
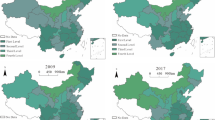
Avoid common mistakes on your manuscript.
1 Introduction
China’s rapid economic growth in the last three decades has been well documented and widely touted. By 2010, China’s gross domestic product (GDP) reached $5.8 trillion, replacing Japan as the world’s second largest economy (NBSC 2011b). The country is quickly moving from an agrarian society to an urban one, with over half of its population now living in urban areas (NBSC 2011b; Yue et al. 2012). Meanwhile, the rapid industrialization and urbanization have been accompanied by soaring uses of resources and massive increases in discharge of pollutants.
In 2006, the Chinese government set a goal of reducing the emissions of major pollutants by ten percent during the 11th “Five-Year Guideline” period (2006–2010).Footnote 1 That target, according to China’s Ministry of Environmental Protection (MEP), had been met. By 2010, the total emissions of sulfur dioxide (SO2) and chemical oxygen demand (COD, a measure of water pollution) dropped respectively by 12.45 and 14.29 % from the levels of 2005 (MEP 2010). Still, a vast amount of pollutants is being released into water and air every year. In 2010, the amount of waste water discharged reached 61.7 billion tons, which included 23.75 billion tons of industrial waste water and 37.98 billon tons of domestic sewage, and had a concentration of pollutants equivalent to 12.38 million tons COD (MEP 2011b). In the same year, 21.85 million of tons of SO2 were emitted into the air (MEP 2011b). According to the 12th Five-Year Guideline (2011–2015), China’s real GDP will double between 2010 and 2020, quadrupling the 2000 GDP. Without doubt, China’s large and fast growing economy will continue to put more pressure on its environment and natural resources. Thus, it is imperative to study the relationship between economic growth and environmental degradation and assess the environmental consequences of economic development in order to offer policy recommendations for environmental pollution control and a long-term, sustainable plan of social economic development.
This paper studies the emissions of SO2 and COD in China, two major pollutants marked for control under the 11th and 12th Five-Year Guidelines. Using fine-scale, county-level data, we estimate the spatial autocorrelations of SO2 and COD emissions in China. The spatial analysis reveals distinct concentrations of SO2 and COD emissions in space. To investigate the driving forces of emissions, we use spatial econometric models, including a spatial error model (SEM) and a spatial lag model (SLM) to evaluate the effects of variables that reflect level of economic development, population density, and industrial structure. Our results show that these explanatory variables are highly correlated with the level of SO2 and COD emissions, though their impacts on SO2 and COD vary.
The rest of the paper is organized as follows. The next section provides a brief review of literature on economic growth and environmental degradation. In section three, we measure the spatial distribution of SO2 and COD emissions in China to identify possible patterns of spatial concentration. We use the spatial autocorrelation index, Moran’s I statistics, to reveal the geographic patterns of SO2 and COD emissions. Section 4 focuses on investigating the driving forces of pollutant emissions using spatial econometric models (SEM and SLM models). The last section concludes the paper with a brief summary of findings and discussion of policy implications.
2 Literature review
Many theoretical and empirical studies have examined the relationship between economic growth and environmental degradation (Beckerman 1992; Dasgupta et al. 2002; John and Pecchenino 1994; Taylor and Copeland 2004). The debate continues whether environmental quality will improve or deteriorate as countries develop. One point of view argues that environmental degradation increases with economic growth due to increased need for energy and materials (Dinda 2004; Roca et al. 2001). Yet, other authors argues that according to the environmental Kuznets curve (EKC) theory, the pollution level first increases with income but will eventually decrease after income reaches certain turning point (Franklin and Ruth 2012; Grossman and Krueger 1991, 1995; Shafik and Bandyopadhyay 1992). A fairly large number of empirical studies have been conducted to test relationship between environment and economic growth. Nevertheless, mixed findings are reported. In addition, there are also evidences that the testing results depended on the type of pollutants and specific econometric models (Chowdhury and Moran 2012; Tamazian and Rao 2010).
Previous studies have shown that socioeconomic factors, including population density, urbanization, industry construction, technology impacts, environmental policy, etc., have strong influence on environmental degradation (He and Wang 2012; Qi et al. 2013; Stern 2004; Suri and Chapman 1998; Wu et al. 2012). Brajer et al. (2011) tested the emission of several air pollutants in Chinese cities and their results provide some support of the inverted-U type EKC trend. Shen (2006) formulated a SEM model to investigate the relationship between income and pollutant emissions in China. The study provides evidence that verifies the impacts effects of environmental policy and industrial structures as well as economic growth on pollution. In a cross-section study of countries, Gangadharan and Valenzuela (2001) demonstrated that population density and urbanization level are both positively related to CO2 emissions while the level of income inequality was inversely related to environmental quality. Overall, previous studies demonstrated that many factors have influence on environmental quality. However, there seems to be no conclusive evidence on which variables have most impacts on the emission of a particular pollutant.
The new economic geography literature has claimed that the agglomeration or the clustering of economic activities occurs at all geographical levels (country, region, or city), which is influenced by scale effect, market externality, knowledge and technology spillover effect (Anselin 2007; Brülhart and Sbergami 2009; Drucker and Feser 2012; Krugman 1998; Patacchini 2008; Ying 2005). Given the fact that economic growth has increasingly been recognized as a key factor that influences the environmental degradation, one may naturally ask the following questions: do pollutant emissions also display patterns of spatial agglomeration or concentration? If they do, to what degree they spatially correspond to the agglomeration of economic activities; and what factors contribute to the spatial concentrations of pollutant emissions?
There are studies that examine the spatial relationships between economic growth and environmental quality. Stern (2000) used data for sixteen Western European countries over thirty-one time periods to indicate that sulfur emissions were spatio-temporally integrated, and GDP was a relevant explanatory variable for sulfur emissions. Other important variables were omitted from the model. Li and Zhang (2011) found two distinct characteristics of industrial SO2 emission in China: spatial clustering and spatial imbalance. Using panel data models that take spatial dependence into account, Su et al. (2009) found that pollutions were spatially dependent in China, and the estimation results seemed to be more robust than using ordinary least square (OLS) estimate.
When estimating the relationship between environmental degradation and economic growth, it seems necessary for us to take spatial dependence or spatial autocorrelation into account. Furthermore, other socio-economic variables also need to be taken into consideration as they have influence over the environmental degradation. However, the current literature has not given adequate attention to spatial factor and other related variables, which are needed to provide an accurate and generalizable account of the relationship between environmental degradation and economic growth.
This paper contributes to the literature in several ways. First, this paper represents the first study that uses detailed county-level data to investigate the relationship between economic growth and environmental pollution in China. Most studies in the current literature are based on national or provincial level data (Koop et al. 2010; Lindmark 2002; Li and Zhang 2008; Tol et al. 2009; Vehmas et al. 2007). In this study, we use a large dataset that includes data on pollutant emissions for 2,329 counties in China. We believe that fine-scale data provide a more accurate account of the spatial patterns and relationships of pollutant emissions.
Second, in addition to GDP, we incorporate other socioeconomic variables, including population density and industrial structure, as independent variables that help explain the spatial patterns of pollutant emissions. Despite their importance, such variables have generally not received sufficient attention in the literature.
Third, we employ spatial econometric models to reveal the relationship between economic growth and pollutant emissions and to ensure that the spatial dependence factor be taken into consideration, which has been proved to exist in environmental phenomena (He et al. 2013; Kim et al. 2003; McPherson and Nieswiadomy 2005). Spatial econometrics remains an underutilized technique in environmental and natural resource economics, and there are few papers using spatial econometric models when analyzing environmental data.
3 Measuring the distribution of environmental pollutants: a spatial autocorrelation analysis
3.1 Measuring spatial distribution
Anselin (1988) identified two properties as particularly important in the analysis of data that are spatial in nature: spatial autocorrelation (or spatial dependence) and spatial heterogeneity. Spatial autocorrelation refers to correlation of values of a variable through geographic space. It represents the interdependence of observations across space that can be attributed to their relative location (e.g., neighboring or non-neighboring). If exits, spatial autocorrelation often indicates a clustering or concentration tendency of attributes. Spatial heterogeneity, on the other hand, refers to variations in relationships (including clustering patterns) that are caused by absolute location of observations.
In this section, we first examine the patterns of spatial concentration of pollutant emissions by measuring spatial autocorrelation. With positive spatial autocorrelation, high or low values of an attribute tend to cluster in space whereas with negative spatial autocorrelation, locations tend to be surrounded by neighbors with very dissimilar values (Anselin and Bera 1998; Chi and Zhu 2008). We use Moran’s I, one of the most widely used spatial autocorrelation statistics (Anselin 1995; Getis 2007; Ord and Getis 1995). There are two forms of Moran’s I. The Global Moran’s I is a measure describing the overall spatial relationship across all geographic units for the entire study area. Therefore, there is only one value derived for the entire study area. The global Moran’s I is calculated from the following formula (Chakravorty et al. 2003; Li and Zhang 2011; Moran 1948):
where \( \overline{x} = \frac{1}{\text{n}}\sum\nolimits_{i = 1}^{n} {x_{i} } \), x i and x j are values of pollutant x in two geographic units (i.e., counties) i and j, w ij is the weight coefficient between counties i and j (which is defined in the spatial weight matrix), and n is the total number of geographic units (i.e., counties). The computation of Moran’s I index is achieved in GeoDA, a free spatial data analysis software package that was initially developed by the Spatial Analysis Laboratory of the University of Illinois at Urbana-Champaign under the direction of Luc Anselin.
The spatial weights matrix formed by weight coefficients is an integral part of spatial modeling. It is defined as the formal expression of spatial dependence between observations (Anselin 1988). This paper adopts a commonly used strategy to determine the spatial weight w ij , namely, spatial linkage based on border sharing. A binary weight matrices are constructed to determine w ij , that is, let w ij = 1 if geographic units i and j are adjacent to each other; otherwise w ij = 0 (Kim et al. 2009; Porter and Purser 2010).
The statistical significance of Moran’s I can be tested using Z statistics by comparing calculated Moran’s I from Eq. (1) and the expected value of Moran’s I, E(I) (Chakravorty et al. 2003; Li and Zhang 2011). Standardized Z statistics and E(I) are respectively expressed as
and
where wi and wj are separately the sum of the elements of ith and jth row of spatial matrix. The values of global Moran’s I range from −1 to 1.
The global Moran’s I is a global index that represents the spatial autocorrelation of an entire study area as a single value. Spatial autocorrelation may differ in accordance with each location, especially when the study area is relatively large. The second form of Moran’s I, local Moran’s I, which is also often called local indicator of spatial association (LISA), is a local indicator of variations in the study area. It is a measure designed to describe the heterogeneity of spatial association across different geographic units within the study area (Chakravorty et al. 2003; Chi and Zhu 2008). Local Moran’s I is defined as
where the operation of summing j is limited to the surrounding areas of i. As shown in Eq. (5), the local Moran’s I decomposes the global Moran’s I into the contribution of each location. Similar to global Moran’s I, a high (positive) value of local Moran’s I means the association of similar values whereas a low (negative) value means the association of dissimilar values (Kim et al. 2009; Li and Zhang 2011).
3.2 Spatial distribution of environmental pollutants
The distribution of SO2 and COD emissions is estimated for 2,392 countiesFootnote 2 in China. The results of global Moran’s I are summarized in Table 1. The statistical significance of the Moran’s I values are tested using both z test and p values. As shown in the table, the high Z scores and low p values suggest that Moran’s I values are highly significant statistically for both SO2 and COD (at 0.01 significant level) and there seems to be statistically significant spatial autocorrelation effect in both 2000 and 2010. During 2000–2010, Moran’s I increased for both SO2 and COD (especially for the former), indicating a tendency of increased concentration of SO2 and COD emissions in China.
To visually explore spatial autocorrelation, we create Moran scatter plots. The Moran scatter plot illustrates the relationship between the values of the chosen attribute at a given location and the average value of the same attribute at neighboring locations (Anselin 1996). The four quadrants of the scatter plot correspond to four types of local spatial autocorrelation. Positive spatial autocorrelation are shown in quadrant I (high–high type, or HH, indicating high values surrounded by high values, and quadrant III (low–low type, or LL, indicating low values surrounded by low values); negative spatial autocorrelation are displayed in quadrant II (low–high type, or LH, indicating low values surrounded by high values) and quadrant IV (high-low, or HL, indicating high values surrounded by low values).
Figures 1 and 2 respectively present local Moran scatter plots of SO2 and COD emissions in 2000 and 2010. For both SO2 and COD emissions, most counties are found in quadrants I and III, suggesting a fairly high degree of positive spatial autocorrelation.
The values of both the global Moran’s I and the Moran scatter plots show a fairly strong spatial autocorrelation at the national level, revealing spatial concentration of SO2 and COD emissions. To detect the local variation in spatial autocorrelation and local patterns of spatial association, we conducted local Moran’s I analysis. The univariate LISA maps in Figs. 3 and 4 include the counties for which the local Moran’s I statistics are significant at the 0.05 level. The positive and significant value of local Moran’s I indicates spatial clustering of similar values (counties in the high–high and low–low groups or regions), whereas a negative and significant value indicates spatial clustering of dissimilar values (counties in the low–high and high-low groups or regions).
In order to have a better understanding of the spatial clustering in LISA maps, the brief introduction to the geography of industries that emit more pollutant is necessary. In 2010, the top three industries of SO2 emissions are electric power industry, ferrous metal (steel, iron etc.) industry, nonmetal mineral industry, which emit the 73 % of total SO2 emissions in China (MEP 2011a). Moreover, petroleum industry and coal mining industries also have higher SO2 emissions than others. In China, the ferrous metal and nonmetal industries mainly distribute in Shandong, Hebei, Henan and Liaoning provinces, and coal mining industry mainly spread in Shanxi, Neimeng and Liaoning provinces. Shandong province is the largest SO2 emission province, which account for 7.4 % of total SO2 emissions in China, and then are Neimeng, Henan and Shanxi provinces (MEP 2011a).
For COD emissions, in 2010, the largest three industries of COD emissions are paper industry, agricultural and sideline products industry, chemical products manufacturing, which have the 51.8 % of total COD emissions in China (MEP 2011a). These industries distribute mainly in Shandong, Henan and Hebei provinces in north of China, and in Guangdong, Jiangsu, Zhejiang, Fujian and Guangxi provinces in South of China. Guangxi is the top COD emissions province that has the 7.6 % of total emissions in China, and then are Shandong, Henan provinces (MEP 2011a).
By comparing the LISA maps of 2000 and 2010 (Figs. 3, 4), we would find that for SO2 emissions, HH regions became more prominent when the number of counties increased from 67 to 76. Meanwhile, the number of counties in the LL clusters decreased from 430 to 362. This finding confirms increased degree of pollutant concentration and deterioration in environmental protection in China. The HH clusters of SO2 emissions can be classified into three categories. The first category is located in heavy industrial regions, where high pollution industries are concentrated. In 2000, major clusters in this category are located in Liaoning province (including industrial cities Fushun, Tieling, Jinzhou, etc.) and central Shandong province (located in Zibo and surrounding areas, a traditional petroleum industrial region in China where other high-pollution industries such as porcelain and steel industries are also important). In 2010, while the HH cluster central Shandong remains, the HH clusters in Liaoning diminished due to decreased industrial activities in those areas. New HH clusters emerged in eastern Heibei province, especially in the Tangshan region where Caifeidian Industrial Zone—a national industrial park—is located, there is agglomeration of steel, chemical and electric industries, including the Capital Iron and Steel Group, or Shougang, which was relocated from Beijing prior to the 2008 Beijing Olympic Games.
The second category of HH clusters is located in the regions that are rich in mineral resources and where mining industries are important. In 2000, one of major HH cluster of this kind was located in central Shanxi province, the capital of China’s coal mining industry. Another major HH cluster of counties are in the Ordos city in central Inner Mongolia where there are also many coal mines on China’s largest coal reserve. By 2010, the HH cluster in central Mongolia became much bigger. Another HH cluster in this category emerged that includes two counties—Xishui and Pingba—in western Guizhou province, where coal industry is important for local and provincial economies. The third category of HH clusters is situated in the major cities and surrounding suburbs areas. The HH cluster in southern Jiangsu province, for example, includes counties and county-level cities that are part of highly-urbanized Suzhou–Wuxi–Changzhou city region, which has literally been connected to the Shanghai metropolis. In addition, counties in the HH clusters in southwestern Chongqing, and in Guangzhou and Huizhou Cities of Guangdong province, are all close to major urban areas and rapidly industrializing with high population densities.
For the LL clusters of counties in SO2 emissions, they are mostly located in regions with high altitude (mountainous landscape), sparse population, and undeveloped economy, especially western parts of the country. The largest LL cluster includes 177 counties—nearly half of LL type of counties—and is centered in Qinghai-Tibet Plateau, extending from southern Xinjiang and Qinghai province in the north to the northern half of Yunnan province in the south, from entire Tibet in the west and the western half of Sichuan province (by the western edge of Sichuan Basin) in the east. Another major LL cluster area is found in the Loess plateau, covering southwestern Gansu province and central and southwestern Shanxi province in the Qingling Mountain region. Other LL clusters are located in the Tarim Desert of Xinjiang province, Sanjiang Plain of Heilongjiang provinces, and the border counties between Jiangxi and Fujian provinces, between Jiangxi and Hubei provinces, and between Jiangxi and Anhui provinces, most of which are also mountainous counties.
The LISA maps of COD emissions also reveal a tendency of increased concentration, with the number of counties in the HH clusters increasing from 99 in 2000 to 119 in 2010. Meanwhile, the number of counties belonging to the LL clusters decreased from 464 to 426 counties. For both years, there is a clear east–west divide on the maps: the LL clusters counties in COD emissions are mainly located in western part of China, whereas the HH clusters are mostly in the east, divided by a line, if to draw one, that seems to coincide well with the famous “Hu Huanyong Line”.Footnote 3 Overall, most counties in the HH category are located in economically developed and highly populated regions. Still, two types of HH clusters can be identified based on geographic location. The first type of HH clusters includes about 70 % of all HH counties, which are mostly located in major city regions, major urban areas (such as provincial capitals) and their surrounding regions. These areas include Pearl River Delta metropolitan region, the Greater Yangze River Delta metropolitan region, Changsha–Zhuzhou–Xiangtan city group in Hunan province, Chengdu city region in Sichuan province, Xi’an-Xianyang city region in Shaanxi province, Urumqi city area in Xinjiang, and urban areas in some other provinces such as Liaoning, Heilongjiang and Shandong.
The second type of HH clusters includes those counties in industrial regions with agglomeration of industries of high COD emissions. One of such clusters is located in in southern Guangxi Autonomous Region. Including over 30 counties, this region has a very high concentration of industries that have high COD emissions, such as sugar manufacturing, nonferrous metal industry and paper industry. In 2010, another cluster of similar kind emerged in eastern Yunnan province. That area is the national epicenter of tobacco production in China.
Similar to LL clusters of SO2 emissions, the largest LL cluster of COD emissions covers the entire Tibet, almost all counties in Qinghai province, counties in southern part of Gansu province, western half of Sichuan province and northern half of Yunnan province. East to this LL cluster is Sichuan Basin, which is surrounded by this the largest LL cluster in the west and north and some other clusters in the east. This interesting pattern illustrates the association of COD emission with economic development and population distribution in relation to physical environment (generally speaking high COD emissions in economically developed, highly populated areas that are relatively flat and low-laying and low COD emissions in those mountainous areas with sparse population). Other LL clusters of COD emissions are scattered Inner Mongolia, Loess Plateau, and Xinjiang. Compared to 2000, however, the number and scope of LL clusters in 2010 became smaller.
To conclude, both SO2 and COD emissions appear to correlate with economic development, population density and industrial agglomeration. Economically developed areas with high population densities, are characterized by both of high COD and SO2 emissions. Mountainous regions with sparse population, such as Qinhai-Tibet Plateau, Qinling Mountain, Wushan Mountain, Loss Plateau, Inner Mongolia, etc., have remarkably low SO2 emissions and low COD emissions. We will investigate the specific relationships between the SO2/COD emissions and other variables including level of economic development, population density, and industrial structure.
4 Investigating the driving forces of pollutant emissions: a spatial econometric analysis
4.1 Spatial econometric models and specifications
In this section, we will use regression models to investigate the driving forces of pollutant emissions in China. When spatial autocorrelation exists, which has been proved to be the case in this study in the previous section, using OLS models to estimate the regression of the spatial data would lead to bias or invalid results (Anselin 1988). Spatial regression models take spatial autocorrelation into consideration. Two basic models attempt to model spatial dependence, the spatial lag model (SEM) and the spatial error model (SLM) (Anselin 1988; Tsutsumi and Seya 2009). While SLM allows for the observed value of nearby observations, SEM deals specifically with spatial autocorrelation in the error terms (Chi and Zhu 2008; Maddison 2006). In SLM, explanatory variables include a spatial lag for the dependent variable as well as a set of exogenous variables. The expression for the spatial lag model is:
where Y is a vector of dependent variables, X is the matrix of explanatory variables, W is the spatial weight matrix, ρ is the spatial autoregressive parameter, β is a vector of regression coefficients, and ε is the vector of independent disturbance terms.
The standard spatial model with autoregressive disturbances represents an alternative form of spatial dependence. A SEM is given by:
where λ is the spatial autoregressive coefficient, X is the matrix of explanatory variables, W is the spatial weight matrix, β is the regression coefficients, ε is the spatially autoregressive error term, and μ is the vector of independent disturbance term representing normal distribution.
Based on the analysis of LISA maps in the previous section, we make the following three hypotheses that applicable to China: (1) emissions of SO2 and COD have a statistically significant relationship with GDP, and SO2 is more sensitive to economic development than COD; (2) emissions of SO2 and COD have a positive correlation with population density, as high population density is often associated with more business activities and more energy consumption, and thus indicates more pollutant emission; (3) industrial structure also has significant impacts on pollutant emissions. We use proportion of tertiary industry, instead of the proportion of secondary industry, as an explanatory variable, because not all the secondary industry have the high SO2/COD emissions, but tertiary activities are generally thought to be less energy intensive and generate less pollutant than the industries in the secondary sector. Therefore, we believe that use of “tertiary activities” is better than using the proportion of secondary industries when the effects of industrial structure on pollutant emissions are discussed.
In order to test the above hypotheses and evaluate the impacts of those variables, we propose regression models using pollutant emissions as dependent variable and influencing factors as independent variables. As the spatial interdependence has to be taken into account when estimating the models proposed, we use the two spatial econometrics models (SLM and SEM) to estimate. The basic form of the econometric model is:
where y is total SO2 or COD emissions of counties in 2010. GDP represents per capita GDP, PEO represents population density as measured in person per square kilometer, and INDU denotes a variable of industrial structure as measured by the proportion of tertiary industry.
The data of SO2 and COD emissions are collected from a variety of sources, including Annual Statistical Report on Environment of China (MEP 2011a), Reports on Environmental Quality in China (MEP 2011b), etc. Data on GDP, population density and industry structure are collected from National Statistical Yearbook (NBSC 2011b), China Statistical Yearbook for Regional Economy (Comprehensive Department, NBSC 2011), and China County Statistical Yearbook (NBSC 2011a), etc.
Table 2 presents descriptive statistics of data and variables. We would like to make a note that SO2 is mainly contained in exhaust gas, while COD exists in waste water. Exhaust gas and waste water come from both industrial and domestic sources
Some previous studies used double logarithmic of pollutant emissions and GDP in the regression model and proved that there was significant relationship between variables in double logarithmic form (Bimonte 2002; Hettige et al. 2000; Kahuthu 2006; Kheder and Zugravu 2012). Logarithmic transformation can reduce the heteroskedasticity effect. In order to figure out whether or not logarithmic transformation is necessary for our dataset, we conduct a spatial association test to decide the variable form. We use bivariate Moran’s I, which is a measure of the correlation of one variable with another variable in space, to estimate the spatial association of two variables in different forms: original form, single logarithmic transformation, and double logarithmic transformation. The results of bivariate Moran’s I are presented in Table 3.
The result of bivariate Moran’s I demonstrate that, double logarithmic form of SO2, COD emissions and GDP, PEO; and single logarithmic form of SO2, COD emissions and INDU (logarithmic form of SO2 and COD emission, original form of INDU) have the most significant spatial autocorrelation. Thus, for the dependent variable (SO2 or COD emission), we use its logarithmic transformation. We also use logarithmic transformation for independent variables GDP and PEO and use the original form of variable INDU in the regression model (Eq. 8).
4.2 Results
We first estimated the model using OLS, and then compare estimation results with those from SEM and SLM. Lagrange multiplier tests are conducted to help identify whether the spatial autocorrelation is due to spatial lag or error and help decide whether SEM or SLM is a better model with robust estimation. If both tests for spatial error and spatial lag are significant, higher test statistics implies a better model.
In order to assess the impacts of explanatory variables, we introduce three models by adding one explanatory variable each time: while there is one explanatory variable (GDP) in the first model, variables PEO and INDU in logarithmic form are respectively added in the second and third models. By doing this, we can test the robustness of the models by comparing the coefficients of each model and determine the best-fit one. The OLS results show that, when incorporating more explanatory variables, the correlation between independent and dependent variables becomes stronger (Table 4).
The OLS results also demonstrate that both SLM and SEM are significant for the three models. For both SLM and SEM, the p value for the initial LM test is 0.000, and their LM values—Lagrange Multiplier (lag) and Lagrange Multiplier (error)—are high and close (Table 4). We further compare the robust forms of the test statistics (robust LM values) in order to determine which one—SLM or SEM—is more robust. The results indicate that for SO2 emissions, both robust Lagrange Multipliers—Robust LM (lag) and Robust LM (error) in Table 4—are significant at the 0.01 significance level. Nonetheless, p value for SEM is smaller than p value for SLM. For COD emissions p value for SEM is also smaller than p value for SLM. Moreover, whereas the robust LM for SEM is significant at the 0.01 significance level for all the three models, the robust LM for SLM is significant only at the 0.05 significance level for Model 2 and Model 3. These results indicate that that the role of spatial correlation is stronger in the error model compared to the lag model. Thus, we chose SEM over SLM to estimate the three models (Model 1, Model 2 and Model 3).
The result of Lagrange multiplier tests from OLS could help decide whether SEM or SLM is a better model with robust estimation. Nevertheless, it has been concluded from the literature that From the previous study, we know that because spatial autocorrelation is not formally incorporated into OLS, the use of OLS may yield biased estimates. Moran’s I statistic of pollutant emissions have demonstrated the spatial heterogeneity of the data, so the regression model should be based on the SLM or SEM models via maximum likelihood estimation, rather than OLS estimation. The results of SLM and SEM are respectively listed in Tables 5 and 6.
Based on the values of log likelihood (LogL), AIC and SC in Tables 5 and 6, the fit improves considerably when the spatial lag variable or LAMBDA is added to the model. The results show that the fit of the regression models are improved when spatial dependence effect is taken into account. Comparing the results between spatial lag and spatial error, the LogL, AIC and SC are more significant in spatial error, indicating that the changes of pollutant emissions in one county would affect its neighbors through explanatory variables. Thus, as explained earlier, SEM is chosen over SLM to estimate the three models (Model 1, Model 2 and Model 3).
Model 1 is used to test the relationship between pollutant emissions and economy development. The results in Table 6 provide evidences that support a positive correlation between per capita GDP and pollutant (both SO2 and COD) emissions. In the SEM model, the regression coefficients between SO2 emissions and per capita GDP and between COD emissions and per capita GDP are both significant at the 1 % level. This tells that the counties with higher levels of economic development are more likely to have more pollutant emissions and higher environmental risk. In addition, comparing the coefficients of two pollutant emissions, we could find that SO2 emissions are subject to greater influence of economic development. These findings support the first hypothesis.
Model 2 extends the specifications of Model 1 by adding the variable of population density. The regression results of the SEM models suggest that population density has significant and positive effects on SO2 emissions and COD emissions, which provides support for the second hypothesis. Compared to Model 1, both LogL and AIC increase in Model 2 (Table 6). In Model 2, the regression coefficients of per capita GDP with SO2 emissions and the COD emissions respectively fall to 0.7340 and 0.3942. The regression coefficients of population density are respectively 0.4820 for SO2 and 0.4244 for COD, implying noticeable impacts of population density on pollutant emissions. Economic development (measured by per capita GDP) appears to be a more important factor influencing SO2 emissions and spatial spillovers than population density. For COD emissions, however, population density has greater influence than the economic development. Population concentration contributes much to the clustering of COD emissions. These results support hypothesis 2.
Model 3 incorporates another independent variable, INDU, which is a variable of industrial structure as measured by the proportion of tertiary industry. The estimation results show that all the three explanatory variables (LnGDP, LnPEO, and INDU) have a significant relationship with both SO2 emissions and COD emissions, with regression coefficients that are significant at the 0.01 significance level. In Model 3, the regression coefficients of LnGDP and LnPEO are significant at the 0.01 significance level for both SO2and COD. The regression coefficient of INDU is −0.0054 for SO2 emissions, which is significant at the 0.05 significance level. This confirms that the development of tertiary industry generally helps decrease SO2 emissions in a county, and thus has positive effect on air pollutant control. This result supports with hypothesis 3. Nevertheless, for COD emissions, the regression coefficient of INDU is 0.0040 at the 0.05 significant level, meaning that the higher percent of tertiary industry does not help decrease COD emissions. Thus, this result does not provide evidence to support the assumption in hypothesis 3 as the sign of the regression coefficient was expected to be negative.
Anyhow, when comparing the three models, all the goodness-of-fit measures suggest that Model 3 provides the best fit. Among the three explanatory variables in Model 3, the level of economic development as measured in per capita GDP is a leading factor that affects SO2 emission, followed by population density. The results from the SEM model reveal that on the county level in China, an increase of 1 % in per capita GDP could possibly lead to an average increase of 0.72 % in SO2 emissions; an increase of 1 % in population density could result in an average increase in SO2 emission by 0.50 %. Meanwhile, our results confirm that a shift in industrial structure towards a service economy may help reduce SO2 emissions. Many regions with high SO2 emissions are concentrated with heavy industries with relatively low share of service industries. These estimation results suggest that economic growth of counties, especially the development of heavy industries, may lead to remarkable increase in SO2 emissions, and thus aggravate air pollution. Due to spatial spillover effects, economic growth also leads to the spatial concentration of SO2 emissions. Regions with rich mineral resources or concentrated heavy industries, in particular, are where the HH clusters of SO2 emissions are found.
For COD emissions, the primary explanatory variable is LnPEO, and the secondary is LnGDP, both are significant at the 0.01 significance level. The results from the SEM analysis show that an increase of 1 % in population density would causes an increase of 0.41 % in COD emissions, whereas an increase of 1 % in per capita GDP is associated with an increase of 0.40 % in COD emissions. However, the finding on the impact of industrial structure—the association of higher percentage of tertiary industry with increase in COD emissions—contradicts hypothesis 3. The seemingly surprising result, we suspect, may be due to the influence of the other two variables. As pointed out before, the leading effect that influences COD emissions is population density. Regions with high population density are often highly urbanized areas with high level of economic development. For those regions, tertiary industries, or services, usually take a high share in the economy. In other words, the impact of variable INDU might be overshadowed or even distorted by the other two variables of population density and economic development.
The results of Model 3 show that autoregressive parameters—ρ in the spatial lag model (Table 5) and λ in the spatial error model (Table 6)—are positive and statistically significant (at the 1 % level). These results confirm significant spatial dependence and strong spillover effects. In SEM model, λ is respectively 0.6240 and 0.5621 for SO2 and COD, which means that an increase of 1 % in one county’s SO2 emission or COD emissions would lead to an increase of 0.62 % in SO2 emission or 0.56 % increase in COD emissions of its neighbors. It demonstrates that the pollutant emissions have strong spillover effects among neighboring counties, the up and down of pollutant emissions in one county could result in corresponding changes in neighbor counties. The spillover effects in pollutant emissions can occur through the agglomeration effects of economic development and population concentration. The spillover effects, or “demonstration effects”, also take place when a locality’s economic and/or environmental policies are imitated followed by its neighbors. In China, similar to the national target of economic growth, the national target of total pollutant emission control each year is decomposed at the provincial and sub-provincial levels. Provinces and counties are evaluated on their achievement in economic growth and controlling pollutant emission. Theoretically, this system of evaluation of government performance could lead to competition among provinces and counties in controlling pollution while developing the economy. For instance, if one county implements strict environmental protection measures, or invests in technology to reduce the pollutant emissions, neighboring counties might follow and take similar measures to strengthen environmental protection. In the opposite case, if a county has very weak or reluctant environmental protection program or bureaucracy with stimulating industrial development and attracting investment as its top priority, its neighbors may also take similar measures in order not to be left behind in economic growth.
5 Conclusions and discussions
This study provides strong evidence of spatial autocorrelation of major pollutant emissions at county level in China. The significant values of both global Moran’s I and local Moran’s I, for instance, point to fairly strong pattern of spatial clustering of pollutant emissions. This research has identified several types of HH and LL clusters for both SO2 and COD emissions. We find that overall both SO2 and COD emissions appear to correlate with economic development, population density, mineral resources, and industrial agglomeration. Economically developed areas with high population densities, especially the Pearl River Delta and Yangtze River Delta regions, are generally characterized by both of high COD and SO2 emissions. Mountainous regions with sparse population, such as Qinhai-Tibet Plateau, Qinling Mountain, Wushan Mountain, Loss Plateau, Inner Mongolia, etc., have remarkably low SO2 emissions and low COD emissions.
Our further analysis using spatial econometric models confirm that economic development, population density and industrial structure are driving forces of SO2 emissions and COD emissions in China. The model (Model 3) that includes all the three explanatory variables has a better fit than models (Model 1 and Model 2) that only include fewer of these variables. Comparing spatial regression models with OLS, the advantages of SLM and SEM over OLS are shown as they effectively reveal the existence and significance of spatial dependence. The SEM, in particular, is chosen over SLM as the role of spatial correlation is stronger in the error model compared to the lag model. The results from this study conform with the literature that spatial autocorrelation and geographic dependence should be considered in the analysis of spatial data. The paper demonstrates that the inclusion of spatial interaction in regression models could increase the accuracy of estimation results when analyzing the effect of explanatory variables on pollutant emissions.
The estimation results of the SEM model show that the economic development does play a crucial role in SO2 emissions, and population concentration also has a significant impact. Moreover, we find that the growing tertiary industry in the economy helps decrease SO2 emissions. Thus, it is noteworthy that in China at current stage of economic development, rapid economic growth, especially the development of heavy industries and resource-oriented industries would aggravate air pollution and worsen environmental degradation. The policy implications from these findings are that environmental policies should consider regional characteristics and differences instead of applying the similar policy to all the regions. Meanwhile, environmental policies should take into spatial spillover effects into consideration and pay particular attention to some special areas. Special guidelines or rules, for instance, may need to be enforced in industrial areas where heavy industries, such as Caofeidian in Tangshan of Hebei province and Zibo in Shandong province, though a more discreet approach is needed. More environmental protection efforts should be made in regions where mining or other resource-oriented industries concentrate, such as Ordos in Inner Mongolia and eastern Guizhou where air pollution has already been very serious. A comprehensive pollution control responsibility system need to be formulated and implemented more effective, in which localities are held responsible for pollution they generate and they are not affected by their neighbors.
For COD emissions, the leading influencing factor is population concentration, and economic development also has significant impact. However, the finding on the role of industrial structure—the association of higher percentage of tertiary industry with increase in COD emissions—contradicts hypothesis 3. We suspect that this surprising result may be due to strong influence of the other two variables on COD emissions. The HH clusters of COD emissions are mostly located in mega city-regions such as Pearl River Delta, and Yangtze River Delta, Changsha–Zhuzhou–Xiangtan city group, as well as provincial capitals represented by Nanjing, Chengdu and Xi’an. The policy implication is that strict water environmental protection policies need be taken implemented in those highly urbanized and heavily populated areas where tremendous waste water discharge. Governments need to take measures to slow down the expansion and sprawl of cities, encourage residents and industries to conserve water, and invest more in technologies and facilities to treat waste water and improvement sewage treatment efficiency.
We want to highlight again that the spatial autocorrelation of SO2 and COD emissions has significantly strong relationship with the economic development. The eastern region of China, which is economically advanced and heavily populated, has lower environmental quality and more severe environmental problems. On the other hand, western China has an undeveloped economy and lower pollutant emissions. Therefore, at the national level, the government should encourage some heavy industries and high energy-consumption industries in the east to be relocated to the west. This will certainly help address the persistent and rising gap between the east and the west in economic development. To be sure, some measures (e.g., new technologies) need to be taken to avoid a simple transfer of pollution from east to west. On the regional level, this study finds that high pollutant emissions are found not only in major urban areas, but also in many counties and small cities on the fringe of those major urban areas. A regional plan of urban development should be developed and adopted in those areas to coordinate industrial development and urban growth, and to prevent environmental quality from deteriorating in those areas.
Notes
China had been implementing 5-year plans that outline social and economic development initiatives. From 2006, the name of the 11th 5-year program was changed to “guideline” in order to more accurately reflect China’s transition from a planned economy to a socialist market economy, or “socialism with Chinese characteristics”.
In this paper, “counties” refer to county-level administrative units, which are mostly counties, but also include autonomous counties and county-level cities.
“Hu Huanyong Line”, also known as “Aihui-Tenchong Line”, is an imaginary line proposed by Chinese population geographer Hu Huanyong in 1935 by connecting two places—Aihui, Heilongjiang Province in the northeast and Tengchong, Yunnan Province in the southwest. West of the line, land and population respectively accounted for 57 and 4 % of the national total in 1935 (about 57 and 6 % in today), while east of the line, land and population respectively accounted for 43 and 96 % of the national total in 1935 (about 43 and 94 % in today). For more description of the concept, see Naughton (2007).
References
Anselin L (1988) Spatial econometrics: methods and models. Kluwer Academic Publishers, Dordrecht
Anselin L (1995) Local indicators of spatial association: LISA. Geogr Anal 27(2):93–115
Anselin L (1996) The Moran scatterplot as an ESDA tool to assess local instability in spatial association. In: Fischer M, Scholten H, Unwin D (eds) Spatial analytical perspectives on GIS. Taylor and Francis, London, pp 111–125
Anselin L (2007) Spatial econometrics in RSUE: retrospect and prospect. Reg Sci Urban Econ 37:450–456
Anselin L, Bera A (1998) Spatial dependence in linear regression models with an introduction to spatial econometrics. In: Ullah A, Giles DE (eds) Handbook of applied economic statistics. Marcel Dekker, New York, pp 237–289
Beckerman W (1992) Economic growth and the environment: whose growth? Whose environment? World Dev 20(4):481–496
Bimonte S (2002) Information access, income distribution, and the environmental Kuznets curve. Ecol Econ 41:145–156
Brajer V, Mead RW, Xiao F (2011) Searching for an environmental Kuznets curve in China’s air pollution. China Econ Rev 22:383–397
Brülhart M, Sbergami F (2009) Agglomeration and growth: cross-country evidence. J Urban Econ 65:48–63
Chakravorty S, Koo J, Lall SV (2003) Metropolitan industrial clusters: patterns and progresses. World Bank Policy Research Working Paper 3073, pp 1–35
Chi G, Zhu J (2008) Spatial regression models for demographic analysis. Popul Res Policy Rev 27:17–42
Chowdhury RR, Moran EF (2012) Turning the curve: a critical review of Kuznets approaches. Appl Geogr 32:3–11
Comprehensive Department, National Bureau of Statistics of China (NBSC) (2011) China statistical yearbook for regional economy 2011. China Statistical Press, Beijing
Dasgupta S, Laplante B, Wang H, Wheeler D (2002) Confronting the environmental Kuznets curve. J Econ Perspect 16(1):147–168
Dinda S (2004) Environmental Kuznets curve hypothesis: a survey. Ecol Econ 49:431–455
Drucker J, Feser E (2012) Regional industrial structure and agglomeration economies: an analysis of productivity in three manufacturing industries. Reg Sci Urban Econ 42:1–14
Franklin RS, Ruth M (2012) Growing up and cleaning up: the environmental Kuznets curve redux. Appl Geogr 32:29–39
Gangadharan L, Valenzuela MR (2001) Interrelationships between income, health and the environment: extending the environmental Kuznets curve hypothesis. Ecol Econ 36:513–531
Getis A (2007) Reflections on spatial autocorrelation. Reg Sci Urban Econ 37:491–496
Grossman GM, Krueger AB (1991) Environmental impacts of a North American free trade agreement. National Bureau of Economic Research Working Paper 3914, Cambridge
Grossmann GM, Krueger AB (1995) Economic growth and the environment. Q J Econ 110(2):353–377
He J, Wang H (2012) Economic structure, development policy and environmental quality: an empirical analysis of environmental Kuznets curves with Chinese municipal data. Ecol Econ 76:49–59
He C, Huang Z, Ye X (2013) Spatial heterogeneity of economic development and industrial pollution in urban China. Stoch Environ Res Risk Assess. doi:10.1007/s00477-013-0736-8
Hettige H, Mani M, Wheeler D (2000) Industrial pollution in economic development: the environmental Kuznets curve revisited. J Dev Econ 62:445–476
John A, Pecchenino R (1994) An overlapping generations model of growth and the environment. Econ J 104:1393–1410
Kahuthu A (2006) Economic growth and environmental degradation in a global context. Environ Dev Sustain 8:55–68
Kheder SB, Zugravu N (2012) Environmental regulation and French firms location abroad: an economic geography model in an international comparative study. Ecol Econ 77:48–61
Kim CW, Phipps TT, Anselin L (2003) Measuring the benefits of air quality improvement: a spatial hedonic approach. Environ Econ Manag 45:24–39
Kim Y, Byun Y, Kim Y, Eo Y (2009) Detection of Cochlodinium polykrikoides red tide based on two-stage filtering using MODIS data. Desalination 249:1171–1179
Koop G, McKitrick R, Tole S (2010) Air pollution, economic activity and respiratory illness: evidence from Canadian cities, 1974–1994. Environ Model Softw 25:873–885
Krugman P (1998) Space: the final frontier. J Econ Perspect 12(2):161–174
Li R, Zhang H (2008) An empirical analysis on the regional discrepancy and tendency of EKC in China (1981–2004). J Xi’an Jiaotong Univ (Soc Sci) 28:35–43
Li C, Zhang Z (2011) Spatial statistics analysis of regional environmental pollution in China. Energy Procedia 5:163–168
Lindmark M (2002) An EKC-pattern in historical perspective: carbon dioxide emissions, technology, fuel prices and growth in Sweden, 1870–1997. Ecol Econ 42:333–347
Maddison D (2006) Environmental Kuznets curves: a spatial econometric approach. J Environ Econ Manag 51(2):218–230
McPherson MA, Nieswiadomy ML (2005) Environmental Kuznets curve: threatened species and spatial effects. Ecol Econ 55:395–407
Ministry of Environmental Protection of the People’s Republic of China (MEP) (2010) Report on the State of the Environment in China, Beijing
Ministry of Environmental Protection of the People’s Republic of China (MEP) (2011a) Annual Statistical Report on Environment of China 2010. China Environmental Science Press, Beijing
Ministry of Environmental Protection of the People’s Republic of China (MEP) (2011b) Reports on Environmental Quality in China 2006–2010. China Environmental Science Press, Beijing
Moran PAP (1948) The interpretation of statistical maps. J R Stat Soc B 10(2):243–251
National Bureau of Statistics of China (NBSC) (2011a) China county statistical yearbook 2011. China Statistical Press, Beijing
National Bureau of Statistics of China (NBSC) (2011b) China statistical yearbook 2011. China Statistical Press, Beijing
Naughton B (2007) The Chinese economy: transitions and growth. MIT Press, Cambridge
Ord JK, Getis A (1995) Local spatial autocorrelation statistics: distributional issues and an application. Geogr Anal 27(4):286–306
Patacchini E (2008) Local analysis of economic disparities in Italy: a spatial statistics approach. Stat Methods Appl 17:85–112
Porter JR, Purser CW (2010) Social disorganization, marriage, and reported crime: a spatial econometrics examination of family formation and criminal offending. J Crim Justice 38:942–950
Qi Z, Ye X, Zhang H, Yu Z (2013) Land fragmentation and variation of ecosystem services in the context of rapid urbanization: the case of Taizhou city, China. Stoch Environ Res Risk Assess. doi:10.1007/s00477-013-0721-2
Roca J, Padilla E, Farré M, Galletto V (2001) Economic growth and atmospheric pollution in Spain: discussing the environmental Kuznets curve hypothesis. Ecol Econ 39:85–99
Shafik N, Bandyopadhyay S (1992) Economic growth and environmental quality: time series and cross-country evidence. Background paper for the World Development Report 1992. World Bank, Washington, DC
Shen J (2006) A simultaneous estimation of environmental Kuznets curve: evidence from China. China Econ Rev 17:383–394
Stern DI (2000) Applying recent developments in time series econometrics to the spatial domain. Prof Geogr 52(1):37–49
Stern DI (2004) The rise and fall of the environmental Kuznets curve. World Dev 32(8):1419–1439
Su Z, Hu R, Lin S (2009) Spatial econometric analysis of Kuznets’ relationship between environmental quality and economic growth. Geogr Res 28(2):303–310
Suri V, Chapman D (1998) Economic growth, trade and energy: implications for the environmental Kuznets curve. Ecol Econ 25:195–208
Tamazian A, Rao BB (2010) Do economic, financial and institutional developments matter for environmental degradation? Evidence from transitional economies. Energy Econ 32:137–145
Taylor MS, Copeland BR (2004) Trade, growth and the environment. J Econ Lit 42(1):7–71
Tol RSJ, Pacala SW, Socolow RH (2009) Understanding long-term energy use and carbon dioxide emissions in the USA. J Policy Model 31:425–445
Tsutsumi M, Seya H (2009) Hedonic approaches based on spatial econometrics and spatial statistics: application to evaluation of project benefits. J Geogr Syst 11:357–380
Vehmas J, Luukkanen J, Kaivo-oja J (2007) Linking analyses and environmental Kuznets curves for aggregated material flows in the EU. J Clean Prod 15:1662–1673
Wu K, Ye X, Qi Z, Zhang H (2012) Impacts of land use/land cover change and socioeconomic development on regional ecosystem services: the case of fast-growing Hangzhou metropolitan area, China. Cities 31:276–284
Ying LG (2005) From physical to general spaces: a spatial econometric analysis of cross-country economic growth and institutions. Ann Reg Sci 39(2):393–418
Yue W, Liu Y, Fan P, Ye X, Wu C (2012) Assessing spatial pattern of urban thermal environment in Shanghai, China. Stoch Environ Res Risk Assess 26:899–911
Author information
Authors and Affiliations
Corresponding author
Rights and permissions
About this article
Cite this article
Li, Q., Song, J., Wang, E. et al. Economic growth and pollutant emissions in China: a spatial econometric analysis. Stoch Environ Res Risk Assess 28, 429–442 (2014). https://doi.org/10.1007/s00477-013-0762-6
Published:
Issue Date:
DOI: https://doi.org/10.1007/s00477-013-0762-6