Abstract
Ecologists often determine the relative importance of niche- and dispersal-based processes via variation partitioning based on species composition. Functional traits and their proxies of phylogeny are expected to increase the detection of niche-based processes and reduce the unexplained variation relative to species identity. We collected eight adult tree traits and phylogenetic data of 41 species and employed a phylogenetic fuzzy weighting method to address this issue in a 9-ha temperate forest dynamics plot. We used redundancy analysis to relate species, phylogenetic and functional compositions to environmental (soil resources and topography) and spatial variables. We also performed multi-scaled analyses on spatial variables by adding environment as the covariates to determine if functional traits increase the detection of niche-based processes at broad scales. The functional traits and intraspecific variation of the wood density among ontogenetic stages could dramatically increase the detection of niche-based processes and reduce the unexplained variation relative to species identity. Phylogenetic and functional compositions were mainly driven by total soil P and elevation, while species composition was weakly affected by multiple environmental variables. After controlling for the environment, a larger amount of the compositional variations in seed mass and maximum height were explained by finer-scaled spatial variables, indicating that dispersal processes may be important at fine spatial scales. Our results suggested that considering functional traits and their intraspecific variations could improve our understanding of ecological processes and increase our ability to predict the responses of plants to environmental change.
Similar content being viewed by others
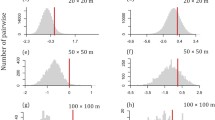
Avoid common mistakes on your manuscript.
Introduction
Determining the mechanisms that influence the variation in species composition among communities is a central question in community ecology. While niche- and dispersal-based processes have frequently been regarded as two dominant ecological processes, their relative importance to community assembly remains unclear. Variation partitioning based on a canonical analysis is used to distinguish these two processes by decomposing the variation in species composition among communities into environmental and spatial fractions (Borcard et al. 1992; Legendre et al. 2009). However, one problem with this method is that there is usually a large amount of unexplained variation, which potentially makes any conclusions about the ecological processes unreliable. These unexplained variations may reflect unmeasured environmental variables and stochastic processes (e.g., ecological drift) (Legendre et al. 2009; Liu et al. 2013). In the past few years, ecologists have attempted to solve this problem by improving the quality of independent variables, including measuring additional environmental properties (Chang et al. 2013; Jones et al. 2008) and developing more sophisticated spatial modeling techniques (e.g., principal coordinates of neighbor matrices (PCNM); Borcard and Legendre 2002), and by employing appropriate variation partitioning methods (Legendre et al. 2005, 2008; Tuomisto and Ruokolainen 2006). However, studies have rarely considered the effects of response variables, which usually use the species abundance matrix or eigenvectors generated from its distance matrix (e.g., Chang et al. 2013; Myers et al. 2013), on our ability of testing hypotheses about the mechanisms underlying the variation in community composition. Species niches are determined by their functional traits, which further influence their distributions along environmental gradients (McGill et al. 2006). Thus, the effects of niche-based processes on community assemblages are expected to be underestimated based on species identity-based response variables that do not consider species functional properties.
There is usually substantial functional redundancy in plant assemblages that may limit the discovery of ecological processes based on species identity. Previous studies have suggested that functional similarity is not the same as species similarity between communities (Fukami et al. 2005; Siefert et al. 2013; Swenson et al. 2012b). In the Barro Colorado Island forest dynamics plot and the forests of eastern North America, functional convergence was found across space and time although there was high species turnover between communities (Siefert et al. 2013; Swenson et al. 2012b). Similarly, Fukami et al. (2005) found converged functional composition but divergent species composition over time in experimental communities. Thus, quantifying functional traits may be more helpful than species identity to understanding assembly processes. In fact, early studies have suggested including species functional information in variation partitioning methods (Dray et al. 2012; Duarte et al. 2012; Legendre et al. 2009). For example, Legendre et al. (2009, p. 672) suggested that variation decomposition was likely altered when other biological (e.g., species traits) variables were included; Duarte et al. (2012, p. 959) also acknowledged that considering some relevant plant traits likely reduced the large amount of unexplained variation in the study.
In recent years, two studies have attempted to explore ecological processes by partitioning the variation of functional traits into environmental and spatial components (Siefert et al. 2013; Spasojevic et al. 2016). However, how functional traits can improve our understanding of ecological processes relative to species identity needs to be explored more deeply. Here, we test the hypothesis that functional traits have a better ability to detect ecological processes than species identity. We adopt the phylogenetic fuzzy weighting method, originally developed by Pillar and Duarte (2010), to generate a multivariate description of functional composition for a community (especially for single trait analyses), which may be compared with species composition more directly than the community-weighted mean trait values used by previous studies (Duarte et al. 2012; Siefert et al. 2013; Spasojevic et al. 2016). The functional response variables generated by the phylogenetic fuzzy weighting method have a data format and type that are consistent with those of species composition (i.e., abundance matrix). This functional composition is a fuzzy sets × sites matrix that is generated by weighting the species composition by the trait distances among species (Duarte et al. 2012). The fuzzy set describes the degree of phylogenetic/functional belonging to it for a species. A phylogenetic relationship can be regarded as a proxy of functional similarity among species for phylogenetically conserved traits (Jiang et al. 2018; Swenson 2013), and phylogenetic composition can thus be regarded as one of the trait-based response variables. Thus, quantifying phylogenetic composition can provide additional insight into the ecological processes for those potentially important and phylogenetically conserved, but unmeasured traits. One problem with functional traits is their considerable intraspecific variation, which can influence our understanding of the processes underlying community assembly (Jung et al. 2010; Messier et al. 2010; Spasojevic et al. 2016). In particular, intraspecific trait variation (ITV) among ontogenetic stages is an important variance component that has often been neglected in previous studies (Siefert et al. 2013; Spasojevic et al. 2016), which can potentially weaken our ability to use functional traits to infer ecological processes (Spasojevic et al. 2014).
Variation partitioning methods usually partition the variation of response variables (e.g., species composition) into a purely environmental fraction [a], a purely spatial fraction [c], a spatially structured environmental fraction [b] and an unexplained fraction [d]. In addition to the focus on reducing fraction [d], ecologists may obtain new insights into the niche- and dispersal-based processes by decomposing fraction [c] (Chang et al. 2013; Dray et al. 2012). The variation explained by fraction [c] at broad scales generally decreases when more environmental variables are included, whereas it increases at fine scales (Chang et al. 2013). Fine-scaled [c] likely indicates either niche-based processes due to missing environmental data at fine scales or dispersal-based processes (Dray et al. 2012). If the fine-scaled [c] indicates dispersal-based processes, the response variables related to some dispersal traits, such as seed mass (SM), may detect stronger dispersal-based processes, and thus a larger amount of variation may be explained by fraction [c] than by the other traits at fine spatial scales.
In this study, we aim to contrast the detection of ecological processes using species, phylogenetic and functional response variables via variation partitioning approaches in a 9-ha temperate old growth forest dynamics plot in northeastern China. We ask (1) whether trait-based response variables and the inclusion of their ITV [woody density (WD) in our study] among ontogenetic stages can increase the detection of niche-based processes and reduce fraction [d]; (2) whether response variables with dispersal-related traits can increase the detection of dispersal-based processes.
Materials and methods
Study site
This study was conducted in the Heilongjiang Liangshui National Natural Reserve in northeastern China (47°10′50″N, 128°53′20″E), which has a large area of originally mixed broadleaved-Korean pine forest. The mean annual temperature in this region is − 0.3 °C. The mean annual precipitation is 676 mm, which mostly occurs in the summer. We established a 9-ha (300 m × 300 m) forest dynamics plot in the reserve in 2005 following the methods of Condit (1998). We identified and measured all individuals with diameter at breast height (dbh) ≥ 1 cm within the plot. In 2010, we performed another census in the plot and documented 21,355 woody individuals belonging to 48 species, 34 genera and 20 families. The plot is relatively flat, with the elevation ranging from 425 to 508 m. In this study, we regarded species reaching a dbh > 10 cm as canopy species (28 species in total). Adult trees are individuals of canopy species with a dbh > 10.0 cm; sapling trees are individuals of canopy species with a dbh ≤ 5.0 cm; all trees are individuals of all 41 species with a dbh ≥ 1 cm (trait data are available for these species). Our analyses were mainly focused on adult trees at the 20 m × 20 m spatial scale.
Phylogenetic tree and trait collection
We constructed a phylogenetic tree using DNA sequences, including two plastid DNA genes (rbcL and matK) and one nuclear DNA gene (ITS) collected from GenBank and sequenced based on the standardized methods (Jiang et al. 2018; Kress et al. 2009). One species did not have any of the three sequences, and we collected an rbcL sequence from a congeneric species as a proxy. We aligned the rbcL and matK globally, the ITS sequences were aligned within orders or families, and all alignments were implemented using MUSCLE (Edgar 2004). We then combined the aligned rbcL, matK and multiple-ITS sequences into a supermatrix using the supermat function in the phylotools package in R-3.2.5 (R Core Team 2016). We constructed a maximum likelihood phylogeny using raxmlGui version 1.5b1 (Silvestro and Michalak 2011). We also used an order-level constraint tree constructed by Phylomatic (Webb and Donoghue 2005) to retain deep nodes a priori and make the topology of our phylogeny coincident with the APG III phylogeny (Kress et al. 2009; Muscarella et al. 2014). The maximum likelihood tree was finally calibrated by nonparametric rate-smoothing in r8s software to obtain the ultrametric phylogenetic tree (Sanderson 2003).
Eight traits, including the WD, SM, maximum height (Hmax), specific leaf area (SLA), leaf area (LA), leaf thickness (LT), leaf dry matter content (LDMC) and leaf phosphorus content (LPC), were collected in this study to indicate the major axes of the plant functional strategy (Jiang et al. 2018; Swenson et al. 2012a). WD is closely related to tree growth and mortality rates (Poorter et al. 2008). SM represents a trade-off among seed size, number and survival probability (Moles and Westoby 2006). Hmax is a major determinant of a species’ ability to compete for light (Moles et al. 2009). Both SM and Hmax can influence the dispersal ability of a plant (Siefert et al. 2015). LA has important consequences for the energy and water balance of a leaf and is tightly related to climate and allometric factors (Cornelissen et al. 2003; Peppe et al. 2011). The SLA, LDMC and LPC are core traits that represent the conservative vs. acquisitive trade-off along the leaf economics spectrum (Wright et al. 2004). LT plays an important role in determining the physical strength of leaves (Pérez-Harguindeguy et al. 2013). SM and Hmax were compiled from Seeds of the Woody Plants in China (State Forestry Administration 2001) and the Flora of China (Wu 1994–2009). Five adult individuals per species and 20 healthy and sun-exposed leaves per individual were collected to measure five leaf traits based on the standardized protocol (Pérez-Harguindeguy et al. 2013). To determine the effects of ITV among the ontogenetic stages on the detection of ecological processes, we measured the WD in both adult and sapling trees. For adults, three individuals were selected, and increment cores were generated (12 mm increment borer; 1.3 m off the ground) and divided into 1-cm segments. For the saplings and shrubs, 3–5 individuals were selected, and the main stems were cut off directly to generate a segment that was 10 cm in length and 1 cm in diameter. The fresh volumes of all segments were measured by water displacement, and they were oven-dried at 103 °C for 72 h. The WD was measured using the oven-dried mass divided by the fresh volume, and the adult WD was annulus weighed (Jiang et al. 2018).
Variables used in the variation partitioning
The environmental and spatial variables were generated as the descriptors, and the species, phylogenetic and functional compositions were generated as the response variables before variation partitioning. The topography (the proxy for potentially missing environmental factors) and soil were measured as the environmental variables in our study. The topography included the mean elevation, slope, sin (aspect), cos (aspect) and convexity. All variables were calculated based on the elevations of the four corners of each cell. These topographical variables are frequently used in forest dynamics plots, and detailed descriptions and formulas can be found in previous studies (Chang et al. 2013; Dray et al. 2012; Legendre et al. 2009). Ten soil variables (pH, total N, total P, available K, available N, available P, organic C, bulk density, soil moisture and mass water content) were generated using kriging interpolation based on the sample points in the plot. We employed systematic and random sampling approaches to collect soil samples based on the 20-m grid in our plot. Detailed soil sampling methods can be found in Shi et al. (2015). All topographical and soil variables (except for two aspect variables) were used to construct the third-degree polynomial equations to generate 39 variables (consisting of the original variables and their quadratic and cubic functions; Jones et al. 2008).
Principal coordinates of neighbor matrices eigenvectors were generated to describe the spatial variables and were then used as indirect proxies of dispersal-based processes (Borcard and Legendre 2002). These PCNM variables could describe the spatial structures at all spatial scales. First, we used the central coordinates of each cell to derive a Euclidean distance matrix. Second, a truncated matrix was generated by retaining and replacing the values in the distance matrix using a threshold (Legendre and Legendre 2012). The analysis was implemented by the pcnm function in the vegan package, and its default value was used as the threshold. Finally, PCNM variables were generated by performing principal coordinate analysis on the truncated distance matrix. A total of 156 PCNM variables with positive eigenvalues were generated in this study.
To contrast the effects of different response variables on the detection of ecological processes, we used 11 response variables, including species, phylogenetic and multivariable functional composition, as well as the functional compositions of WD, SM, Hmax, SLA, LA, LT, LDMC and LPC. The species abundance matrix was Hellinger-transformed to generate the species composition to ensure that our results were comparable to those of previous studies (Chang et al. 2013; De Cáceres et al. 2012; Legendre and Gallagher 2001). Instead of using the community-weighted mean trait values (Siefert et al. 2013; Spasojevic et al. 2016), we constructed trait dendrograms and employed the phylogenetic fuzzy weighting method developed by Pillar and Duarte (2010) to generate the functional composition (we also showed the variation partitioning results based on community-weighted mean trait values in Figs. S5–S6, which were similar with those based on phylogenetic fuzzy weighting method). The functional composition generated by dendrogram was easily comparable to the phylogenetic compositions generated by phylogenetic tree (Swenson et al. 2012a; Swenson 2014). First, we constructed a trait dendrogram for all of the traits together and individually for the eight traits using hierarchical clustering (Swenson et al. 2012a). For the multivariable trait dendrogram, principal component (PC) analysis was implemented a priori to reduce trait redundancy (the first five PCs for all 41 species explained 94.2% of the variation; the first four PCs for the 28 canopy species explained 91.6% of the variation; Swenson 2014). Second, the dendrogram was used to generate a trait distance matrix between species; then, this matrix was transformed to a similarity matrix between species. Third, this similarity matrix was standardized to make the sum of each column equal to one. Finally, this standardized matrix was multiplied by the species abundance matrix to generate a functional composition matrix (i.e., fuzzy sets × sites or P matrix in Duarte et al. 2016). This analysis was implemented by the matrix.p function in the PCPS package. The functional composition described the possibility of a species with a certain trait value to be present in a community. More detailed descriptions of the calculations and background for the phylogenetic fuzzy weighting approach can be found in a recent review by Duarte et al. (2016). We then generated the phylogenetic composition by replacing the dendrograms with a phylogenetic tree (Duarte et al. 2012). To be consistent among species, phylogenetic and functional compositions, the phylogenetic and functional compositions were also Hellinger-transformed prior to the variation partitioning analyses. All traits were log-transformed before analysis.
Statistical analyses
The environmental and spatial variables were used as the descriptors to explain the variations of species, phylogenetic and functional compositions using the varpart function in the vegan package. Before variation partitioning, the environmental and spatial variables needed to be filtered separately using forward selection programs to retain the variables that were significantly related to the response variables (Blanchet et al. 2008). To test the effects of ITV among ontogenetic stages on the detection of ecological processes, we focused our main analyses on the WD. We generated three WD values for each species in our analyses: the adult WD values, sapling WD values and mean WD values (the average of the adult and sapling values). We used these three values to perform variation partitioning analyses on both adult and sapling communities. When the adult WD values applied to adult communities or sapling WD values applied to sapling communities could reveal ecological processes better than the other two scenarios (e.g., sapling or mean WD values applied to adult communities), we could conclude that the ITV among ontogenetic stages was important and could not be ignored. In addition, we also applied all other adult traits to adult, sapling and all tree communities (Spasojevic et al. 2014). This substitution among different life histories based on adult traits has frequently been used in previous studies (Siefert et al. 2013; Yang et al. 2014). We performed these analyses to determine if applying adult trait values to only adult tree communities can infer ecological processes relative to species identity better than applying adult trait values to sapling and all tree communities.
To explore the difference in the variation explained by the PCNM variables at different scales for different traits, we performed a partial redundancy analysis by controlling for the environmental variables (Legendre and Legendre 2012). We grouped all 156 PCNMs into three categories of broad, medium and fine spatial scales: 1–49 were the broad-scaled PCNMs, 50–99 were the medium-scaled PCNMs, and 100–156 were the fine-scaled PCNMs. If finer-scaled PCNMs represented dispersal-based processes (Dray et al. 2012), we predicted that some traits relevant to dispersal, such as SM and Hmax, might have larger amounts of variation explained by spatial variables at finer spatial scales.
Results
For the adult trees, a larger amount of the variations of the phylogenetic, functional and individual trait compositions were explained by the pure environmental and shared environmental and spatial fractions ([a + b] fraction; except for LDMC), and these compositions had lower variation residuals ([d] fraction) than species composition (Fig. 1a). The ITV among the ontogenetic stages influenced the results of variation partitioning. First, a larger amount of variation in the WD composition was explained by the pure environmental and shared environmental and spatial fraction [a + b] when the composition of adult trees was analyzed using the adult traits and mean values (30.0 and 33.0%) than when the sapling trait values were used (12.5%) (Fig. 1a). An increased amount of the variation in the WD composition was explained by the fraction [a + b] when the adult traits, mean values and sapling traits were used to analyze the composition of the sapling trees (12.6, 28.7 and 35.2%; Fig. 1b). Second, the variations of the phylogenetic, functional and individual trait compositions explained by the environment [a + b] were similar or even lower than that of species composition when the sapling trees and all trees were analyzed based on adult traits, respectively (Figs. 1b and S1).
Proportional variation (adjusted R2 × 100%) in the effects of different response variables on the species composition of a adult and b sapling trees in a 9-ha temperate forest dynamics plot. The effects of purely environmental, purely spatial, and shared environmental and spatial fractions on response variable are indicated by shading, white and grey. Variables are the species (SPP), phylogenetic (Phy) and functional (Funt) composition, seed mass (SM), maximum height (H), specific leaf area (SLA), leaf area (LA), leaf thickness (LT), leaf dry matter content (LDMC) and leaf phosphorus content (LPC) based on adult traits for adult and sapling trees. For the wood density, we use adult traits (WD_A), sapling traits (WD_S) and mean values (WD_M) to analyze the adult and sapling trees. The dashed lines indicate the total amount of the variation in the species composition that is explained by the environmental and spatial fractions
More environmental variables with low explanatory powers influenced the species composition (9 variables), whereas fewer variables with high explanatory powers affected the phylogenetic, functional and individual trait compositions (3–6 variables) (Fig. 2). The total P and elevation were the main drivers of phylogenetic, functional and most individual trait compositions; however, the slope was the main driver for the composition of SM (Fig. 2). Across all response variables after forward selection, the distributions of the variation explained by the PCNMs were skewed to the right (Fig. S2). The explained variations of the phylogenetic, functional and individual traits compositions were higher than that of species composition across broad, medium and fine scales (Fig. S3). When topography and soil were added as covariates, the distributions of the variation explained by the PCNMs became flat (Fig. S4). The variations of the phylogenetic, functional and individual trait compositions explained by the PCNMs dramatically decreased and were generally lower relative to that of species composition at broad scales (Fig. 3a); however, the variations explained by the PCNMs were still higher than that of the species composition at medium and fine scales (Fig. 3). A larger number of PCNMs declined at broad scales than at fine scales (Figs. 3 and S2–S4). Consistent with the environmental variables, species composition was determined by multiple PCNMs, especially at broad scales (Figs. 3a, 3d and S3a). The compositions of SM and Hmax showed the largest amounts of variation explained by medium and fine-scaled PCNMs (Fig. 3d).
The variations (adjusted R2 × 100%) of the different response variables for adult trees that are explained by the principal coordinates of neighbor matrices (PCNMs; bars), and the number of PCNMs that is retained (open circles) after adding topography and soil as the covariates at a broad (1–49 PCNMs), b medium (50–99 PCNMs), c fine (100–156 PCNMs) and d both medium and fine scales. The response variables include the species (SPP), phylogenetic (Phy) and functional (Funt) composition, wood density (WD), seed mass (SM), maximum height (H), specific leaf area (SLA), leaf area (LA), leaf thickness (LT), leaf dry matter content (LDMC) and leaf phosphorus content (LPC). The dashed lines indicate the total amount of the variation in the species composition that is explained by the PCNMs after controlling for the environment
Discussion
In this 9-ha temperate forest dynamics plot, we found that functional traits could dramatically improve our understanding of niche- and dispersal-based processes over only considering species identity using a variation partitioning approach. However, without considering the ITV of the WD among the ontogenetic stages, we found that the ability of the functional traits to reflect ecological processes (especially for niche processes) declined. We also found that more environmental factors affected the species composition, whereas fewer environmental factors determined the trait-based response variables. The results suggest that species identity could depict multiple facets of a plant but might be a weaker descriptor for species distribution, while individual traits could depict one or certain facets of a plant but might be a stronger descriptor of species distribution. By decomposing fraction [c], we found that the niche-based processes influenced the community assemblages at broader scales, and dispersal processes might determine species distribution at finer scales.
Many studies have attempted to distinguish niche- and dispersal-based processes by partitioning the variation of species composition into environmental and spatial components (Chang et al. 2013; De Cáceres et al. 2012; Legendre et al. 2009; Myers et al. 2013; Qiao et al. 2015). However, few studies have used trait-based response variables instead of species composition to infer the relative importance of both processes (Siefert et al. 2013; Spasojevic et al. 2016), which were expected to increase our understanding of niche-based processes based on variation partitioning (Duarte et al. 2012; Legendre et al. 2009). To the best of our knowledge, this is the first study to simultaneously use multivariate species, phylogenetic and functional compositions as the response variables to systematically contrast their differences for inferring ecological processes via variation partitioning and integrate the ITV among the ontogenetic stages into analyses. We found that trait-based response variables (including phylogenetic composition) dramatically altered the results of variation partitioning and reduced the unexplained variation fraction [d], as predicted by previous studies (Duarte et al. 2012; Legendre et al. 2009). In addition, our results indicated that functional traits could improve our understanding of niche-based processes. First, the increased variation in the trait-based variables was explained by the environmental fraction [a + b] relative to species composition, which implied the determination of niche-based processes (Chang et al. 2013; Legendre et al. 2009). Specifically, we acknowledged that fraction [b] might also partly indicate dispersal processes (Chang et al. 2013). Second, a larger amount of the variation in the trait-based variables that was explained by broad-scaled PCNMs declined after controlling for the environment (Figs. 3a and S3a). Broad-scaled environmental control is generally regarded as a niche-based process (Dray et al. 2012). However, these conclusions strongly depended on whether we considered the ITV when the trait-based response variables were used. The ITV among the ontogenetic stages could influence our understanding of the rules of community assembly (Spasojevic et al. 2014). We found that functional traits could not reveal niche-based processes better than the species composition without considering the ITV of the WD, which was strongly influenced by the ontogeny of the trees (Hietz et al. 2017). On the other hand, only applying adult trait values to only adult tree communities can reveal ecological processes relative to species identity better than applying adult trait values to sapling and all tree communities. The result suggested that the substitution among different life histories based on adult traits, frequently used in previous studies (Siefert et al. 2013; Yang et al. 2014), might impede our understanding of ecological processes. Even so, we might have underestimated the importance of ITV in uncovering ecological processes because the ITV of the species along the environmental gradients was also important but was overlooked in this study (Spasojevic et al. 2016). This might explain why we found that a slightly larger amount of the variation in the WD was explained by the environment when the mean WD values were used to analyze adult tree communities than the adult WD values. In our study, the ITV of only the WD was measured, which would also underestimate the importance of the ITV. More ecologically important traits should be measured to explore the influence of ITV on ecological processes in the future.
Compared with trait-based response variables, species composition was influenced by more topographical and soil factors, but with low explanatory powers. The result was consistent for broad-scaled PCNM variables (Figs. S2a and S4a). This result indicated that species identity might be a more general descriptor of species characteristics, but would likely hide the relationship with the environment relative to functional traits, which were simple variables that directly responded to environmental gradients (McGill et al. 2006). Although individual traits had similar abilities to reveal ecological processes (similar variation partitioning results), the drivers of niche selection were different for different traits. In our study, leaf traits were filtered by the mean elevation and total P, while reproductive and dispersal traits were more strongly influenced by the slope. This result was not surprising because SM was not correlated with leaf traits among species (Table S1). Thus, analyses of individual traits could provide a deeper understanding of ecological processes and discover different environmental drivers that influence community assemblages and species distributions (Spasojevic and Suding 2012; Swenson and Enquist 2009).
It is generally hypothesized by ecologists that dispersal processes are the main ecological processes at fine spatial scales, and might be indicated by finer-scaled PCNM variables after controlling for environmental factors (Chang et al. 2013; Dray et al. 2012). However, the lack of environmental variables at fine spatial scales can also leave a large amount of the variation unexplained by environmental variables, but explained by the spatial variables. Here, we attempted to address this issue using a trait-based approach. After controlling for the environment, more of the variation in the traits relevant to dispersal was explained by the finer-scaled PCNMs (namely, SM and Hmax). Thus, our results implied that dispersal processes at least partly influenced the assembly of the communities at fine spatial scales. However, this suggestion does not indicate that niche-based processes are not important at these scales, but the demonstration of these processes requires precise environmental data at fine spatial scales.
Overall, our results indicated that both environmental (topography and soil) and spatial variables determined species, phylogenetic and functional compositions, which were consistent with previous studies conducted in other forest dynamics plots (Liu et al. 2013; Yang et al. 2014, 2015). For example, Liu et al. (2013) found that space, topography and soil could influence the spatial distribution of the functional structure in a subtropical forest. Similarly, Yang et al. (2014) showed that soil factors were important drivers of phylogenetic and functional structures in tropical plant assemblages. In addition to using phylogenetic or trait dispersion as the response variable, Yang et al. (2015) also found that environmental factors were more important drivers of phylogenetic and functional beta diversity than spatial factors. While these studies provided important knowledge about the effects of environmental and spatial factors on phylogenetic and trait patterns, they did not show their effects on phylogenetically and functionally compositional variables. Thus, it might be difficult to answer how functional traits could improve our understanding of ecological processes, as predicted by Legendre et al. (2009) and Duarte et al. (2012), and to determine what factors drove phylogenetic and functional beta diversity (i.e., compositional variation between communities) along environmental gradients. In addition, we also explored the importance of ITV of the WD when inferring ecological processes. We suggest that a future study should integrate ITV along environmental gradients across more traits.
Conclusion
We found that functional traits could reveal niche- and dispersal-based processes better than species composition, and the individual traits could more directly correlate to environmental axes. However, the ITV among ontogenetic stages played an important role in strengthening the relationship between traits and niche-based processes. Our study suggests that functional traits and their intraspecific variation should be embraced and integrated to explain ecological processes and predict the responses of species to environmental change.
References
Blanchet FG, Legendre P, Borcard D (2008) Forward selection of explanatory variables. Ecology 89:2623–2632. https://doi.org/10.1890/07-0986.1
Borcard D, Legendre P (2002) All-scale spatial analysis of ecological data by means of principal coordinates of neighbour matrices. Ecol Model 153:51–68. https://doi.org/10.1016/S0304-3800(01)00501-4
Borcard D, Legendre P, Drapeau P (1992) Partialling out the spatial component of ecological variation. Ecology 73:1045–1055. https://doi.org/10.2307/1940179
Chang L-W, Zelený D, Li C-F, Chiu S-T, Hsieh C-F (2013) Better environmental data may reverse conclusions about niche- and dispersal-based processes in community assembly. Ecology 94:2145–2151. https://doi.org/10.1890/12-2053.1
Condit R (1998) Tropical forest census plots. Springer, Berlin
Cornelissen JHC, Lavorel S, Garnier E, Diaz S, Buchmann N, Gurvich DE, Reich PB, ter Steege H, Morgan HD, van der Heijden MGA, Pausas JG, Poorter H (2003) A handbook of protocols for standardised and easy measurement of plant functional traits worldwide. Aust J Bot 51:335–380. https://doi.org/10.1071/BT02124
De Cáceres M, Legendre P, Valencia R, Cao M, Chang L-W, Chuyong G, Condit R, Hao Z, Hsieh C-F, Hubbell S, Kenfack D, Ma K, Mi X, Supardi Noor MN, Kassim AR, Ren H, Su S-H, Sun IF, Thomas D, Ye W, He F (2012) The variation of tree beta diversity across a global network of forest plots. Glob Ecol Biogeogr 21:1191–1202. https://doi.org/10.1111/j.1466-8238.2012.00770.x
Dray S, Pélissier R, Couteron P, Fortin MJ, Legendre P, Peres-Neto PR, Bellier E, Bivand R, Blanchet FG, De Cáceres M, Dufour AB, Heegaard E, Jombart T, Munoz F, Oksanen J, Thioulouse J, Wagner HH (2012) Community ecology in the age of multivariate multiscale spatial analysis. Ecol Monogr 82:257–275. https://doi.org/10.1890/11-1183.1
Duarte LDS, Debastiani VJ, Freitas AVL, Pillar VD, Peres-Neto P (2016) Dissecting phylogenetic fuzzy weighting: theory and application in metacommunity phylogenetics. Methods Ecol Evol 7:937–946. https://doi.org/10.1111/2041-210x.12547
Duarte LD, Prieto PV, Pillar VD (2012) Assessing spatial and environmental drivers of phylogenetic structure in Brazilian Araucaria forests. Ecography 35:952–960. https://doi.org/10.1111/j.1600-0587.2011.07193.x
Edgar RC (2004) MUSCLE: multiple sequence alignment with high accuracy and high throughput. Nucleic Acids Res 32:1792–1797. https://doi.org/10.1093/nar/gkh340
Fukami T, Martijn Bezemer T, Mortimer SR, Putten WH (2005) Species divergence and trait convergence in experimental plant community assembly. Ecol Lett 8:1283–1290. https://doi.org/10.1111/j.1461-0248.2005.00829.x
Hietz P, Rosner S, Hietz-Seifert U, Wright SJ (2017) Wood traits related to size and life history of trees in a Panamanian rainforest. New Phytol 213:170–180. https://doi.org/10.1111/nph.14123
Jiang F, Xun Y, Cai H, Jin G (2018) What factors potentially influence the ability of phylogenetic distance to predict trait dispersion in a temperate forest? Ecol Evol. https://doi.org/10.1002/ece3.3691 (in press)
Jones MM, Tuomisto H, Borcard D, Legendre P, Clark DB, Olivas PC (2008) Explaining variation in tropical plant community composition: influence of environmental and spatial data quality. Oecologia 155:593–604. https://doi.org/10.1007/s00442-007-0923-8
Jung V, Violle C, Mondy C, Hoffmann L, Muller S (2010) Intraspecific variability and trait-based community assembly. J Ecol 98:1134–1140. https://doi.org/10.1111/j.1365-2745.2010.01687.x
Kress WJ, Erickson DL, Jones FA, Swenson NG, Perez R, Sanjur O, Bermingham E (2009) Plant DNA barcodes and a community phylogeny of a tropical forest dynamics plot in Panama. Proc Natl Acad Sci USA 106:18621–18626. https://doi.org/10.1073/pnas.0909820106
Legendre P, Borcard D, Peres-Neto PR (2005) Analyzing beta diversity: partitioning the spatial variation of community composition data. Ecol Monogr 75:435–450. https://doi.org/10.1890/05-0549
Legendre P, Borcard D, Peres-Neto PR (2008) Analyzing or explaining beta diversity? Comment. Ecology 89:3238–3244. https://doi.org/10.1890/07-0272.1
Legendre P, Gallagher E (2001) Ecologically meaningful transformations for ordination of species data. Oecologia 129:271–280. https://doi.org/10.1007/s004420100716
Legendre P, Legendre LF (2012) Numerical ecology. Elsevier, Oxford
Legendre P, Mi X, Ren H, Ma K, Yu M, Sun I-F, He F (2009) Partitioning beta diversity in a subtropical broad-leaved forest of China. Ecology 90:663–674. https://doi.org/10.1890/07-1880.1
Liu X, Swenson NG, Zhang J, Ma K, Thompson K (2013) The environment and space, not phylogeny, determine trait dispersion in a subtropical forest. Funct Ecol 27:264–272. https://doi.org/10.1111/1365-2435.12018
McGill BJ, Enquist BJ, Weiher E, Westoby M (2006) Rebuilding community ecology from functional traits. Trends Ecol Evol 21:178–185. https://doi.org/10.1016/j.tree.2006.02.002
Messier J, McGill BJ, Lechowicz MJ (2010) How do traits vary across ecological scales? A case for trait-based ecology. Ecol Lett 13:838–848. https://doi.org/10.1111/j.1461-0248.2010.01476.x
Moles AT, Warton DI, Warman L, Swenson NG, Laffan SW, Zanne AE, Pitman A, Hemmings FA, Leishman MR (2009) Global patterns in plant height. J Ecol 97:923–932. https://doi.org/10.1111/j.1365-2745.2009.01526.x
Moles AT, Westoby M (2006) Seed size and plant strategy across the whole life cycle. Oikos 113:91–105. https://doi.org/10.1111/j.0030-1299.2006.14194.x
Muscarella R, Uriarte M, Erickson DL, Swenson NG, Zimmerman JK, Kress WJ (2014) A well-resolved phylogeny of the trees of Puerto Rico based on DNA barcode sequence data. PLoS One 9:e112843. https://doi.org/10.1371/journal.pone.0112843
Myers JA, Chase JM, Jimenez I, Jorgensen PM, Araujo-Murakami A, Paniagua-Zambrana N, Seidel R (2013) Beta-diversity in temperate and tropical forests reflects dissimilar mechanisms of community assembly. Ecol Lett 16:151–157. https://doi.org/10.1111/ele.12021
Peppe DJ, Royer DL, Cariglino B, Oliver SY, Newman S, Leight E, Enikolopov G, Fernandez-Burgos M, Herrera F, Adams JM, Correa E, Currano ED, Erickson JM, Hinojosa LF, Hoganson JW, Iglesias A, Jaramillo CA, Johnson KR, Jordan GJ, Kraft NJ, Lovelock EC, Lusk CH, Niinemets U, Penuelas J, Rapson G, Wing SL, Wright IJ (2011) Sensitivity of leaf size and shape to climate: global patterns and paleoclimatic applications. New Phytol 190:724–739. https://doi.org/10.1111/j.1469-8137.2010.03615.x
Pillar VD, Duarte L (2010) A framework for metacommunity analysis of phylogenetic structure. Ecol Lett 13:587–596. https://doi.org/10.1111/j.1461-0248.2010.01456.x
Poorter L, Wright SJ, Paz H, Ackerly DD, Condit R, Ibarra-Manríquez G, Harms KE, Licona JC, Martínez-Ramos M, Mazer SJ, Muller-Landau HC, Peña-Claros M, Webb CO, Wright IJ (2008) Are functional traits good predictors of demographic rates? Evidence from five Neotropical forests. Ecology 89:1908–1920. https://doi.org/10.1890/07-0207.1
Pérez-Harguindeguy N, Díaz S, Garnier E, Lavorel S, Poorter H, Jaureguiberry P, Bret-Harte MS, Cornwell WK, Craine JM, Gurvich DE, Urcelay C, Veneklaas EJ, Reich PB, Poorter L, Wright IJ, Ray P, Enrico L, Pausas JG, de Vos AC, Buchmann N, Funes G, Quétier F, Hodgson JG, Thompson K, Morgan HD, ter Steege H, Sack L, Blonder B, Poschlod P, Vaieretti MV, Conti G, Staver AC, Aquino S, Cornelissen JHC (2013) New handbook for standardised measurement of plant functional traits worldwide. Aust J Bot 61:167–234. https://doi.org/10.1071/bt12225
Qiao X, Li Q, Jiang Q, Lu J, Franklin S, Tang Z, Wang Q, Zhang J, Lu Z, Bao D, Guo Y, Liu H, Xu Y, Jiang M (2015) Beta diversity determinants in Badagongshan, a subtropical forest in central China. Sci Rep 5:17043. https://doi.org/10.1038/srep17043
R Core Team (2016) R: a language and environment for statistical computing. R Foundation for Statistical Computing, Vienna, Austria. https://www.R-project.org/
Sanderson MJ (2003) r8s: inferring absolute rates of molecular evolution and divergence times in the absence of a molecular clock. Bioinformatics 19:301–302. https://doi.org/10.1093/bioinformatics/19.2.301
Shi B, Gao W, Cai H, Jin G (2015) Spatial variation of soil respiration is linked to the forest structure and soil parameters in an old-growth mixed broadleaved-Korean pine forest in northeastern China. Plant Soil 400:263–274. https://doi.org/10.1007/s11104-015-2730-z
Siefert A, Lesser MR, Fridley JD (2015) How do climate and dispersal traits limit ranges of tree species along latitudinal and elevational gradients? Glob Ecol Biogeogr 24:581–593. https://doi.org/10.1111/geb.12287
Siefert A, Ravenscroft C, Weiser MD, Swenson NG (2013) Functional beta-diversity patterns reveal deterministic community assembly processes in eastern North American trees. Glob Ecol Biogeogr 22:682–691. https://doi.org/10.1111/geb.12030
Silvestro D, Michalak I (2011) raxmlGUI: a graphical front-end for RAxML. Org Divers Evol 12:335–337. https://doi.org/10.1007/s13127-011-0056-0
Spasojevic MJ, Suding KN (2012) Inferring community assembly mechanisms from functional diversity patterns: the importance of multiple assembly processes. J Ecol 100:652–661. https://doi.org/10.1111/j.1365-2745.2011.01945.x
Spasojevic MJ, Turner BL, Myers JA, Jones R (2016) When does intraspecific trait variation contribute to functional beta-diversity? J Ecol 104:487–496. https://doi.org/10.1111/1365-2745.12518
Spasojevic MJ, Yablon EA, Oberle B, Myers JA (2014) Ontogenetic trait variation influences tree community assembly across environmental gradients. Ecosphere 5:art129. https://doi.org/10.1890/es14-000159.1
State Forestry Administration (2001) Seeds of woody plants in China. China Forestry Publishing House, Beijing
Swenson NG (2013) The assembly of tropical tree communities—the advances and shortcomings of phylogenetic and functional trait analyses. Ecography 36:264–276. https://doi.org/10.1111/j.1600-0587.2012.00121.x
Swenson NG (2014) Functional and phylogenetic ecology in R. Springer, New York
Swenson NG, Enquist BJ (2009) Opposing assembly mechanisms in a neotropical dry forest: implications for phylogenetic and functional community ecology. Ecology 90:2161–2170. https://doi.org/10.1890/08-1025.1
Swenson NG, Erickson DL, Mi X, Bourg NA, Forero-Montaña J, Ge X, Howe R, Lake JK, Liu X, Ma K, Pei N, Thompson J, Uriarte M, Wolf A, Wright SJ, Ye W, Zhang J, Zimmerman JK, Kress WJ (2012a) Phylogenetic and functional alpha and beta diversity in temperate and tropical tree communities. Ecology 93:S112–S125. https://doi.org/10.1890/11-0402.1
Swenson NG, Stegen JC, Davies SJ, Erickson DL, Forero-Montaña J, Hurlbert AH, Kress WJ, Thompson J, Uriarte M, Wright SJ (2012b) Temporal turnover in the composition of tropical tree communities: functional determinism and phylogenetic stochasticity. Ecology 93:490–499. https://doi.org/10.1890/11-1180.1
Tuomisto H, Ruokolainen K (2006) Analyzing or explaining beta diversity? Understanding the targets of different methods of analysis. Ecology 87:2697–2708. 10.1890/0012-9658(2006)87[2697:aoebdu]2.0.co;2
Webb CO, Donoghue MJ (2005) Phylomatic: tree assembly for applied phylogenetics. Mol Ecol Notes 5:181–183. https://doi.org/10.1111/j.1471-8286.2004.00829.x
Wright IJ, Reich PB, Westoby M, Ackerly DD, Baruch Z, Bongers F, Cavender-Bares J, Chapin T, Cornelissen JH, Diemer M (2004) The worldwide leaf economics spectrum. Nature 428:821–827. https://doi.org/10.1038/nature02403
Wu ZY, Raven PH (1994–2009) Flora of China. Science Press & Missouri Botanical Garden Press, Beijing & St Louis
Yang J, Swenson NG, Zhang G, Ci X, Cao M, Sha L, Li J, Ferry Slik JW, Lin L (2015) Local-scale partitioning of functional and phylogenetic beta diversity in a tropical tree assemblage. Sci Rep 5:12731. https://doi.org/10.1038/srep12731
Yang J, Zhang G, Ci X, Swenson NG, Cao M, Sha L, Li J, Baskin CC, Slik JWF, Lin L, Poorter L (2014) Functional and phylogenetic assembly in a Chinese tropical tree community across size classes, spatial scales and habitats. Funct Ecol 28:520–529. https://doi.org/10.1111/1365-2435.12176
Acknowledgements
This study was financially supported by the National Natural Science Foundation of China (no. 31730015, National Natural Science Foundation of China), the Strategic Priority Research Program of the Chinese Academy of Sciences (XDPB0203, Chinese Academy of Sciences) and the Fundamental Research Funds for the Central Universities (2572017EA02, Northeast Forestry University). We thank the editor and two anonymous reviewers for the constructive comments that improved the quality of the manuscript. We also thank Dr. Leandro D.S. Duarte for the help and suggestions for the calculations of the phylogenetic fuzzy weighting approach, and Dr. Jinlong Zhang and Dr. Robert Muscarella for providing suggestions on the construction of the phylogenetic tree.
Author information
Authors and Affiliations
Contributions
FJ and GZJ conceived the idea. FJ, YHX and HYC collected the soil, topological and traits data. FJ analyzed the data. FJ and GZJ wrote the manuscript; other authors provided editorial advice.
Corresponding author
Additional information
Communicated by Katherine L. Gross.
Electronic supplementary material
Below is the link to the electronic supplementary material.
Rights and permissions
About this article
Cite this article
Jiang, F., Xun, Y., Cai, H. et al. Functional traits can improve our understanding of niche- and dispersal-based processes. Oecologia 186, 783–792 (2018). https://doi.org/10.1007/s00442-018-4060-3
Received:
Accepted:
Published:
Issue Date:
DOI: https://doi.org/10.1007/s00442-018-4060-3