Abstract
Key messages
Sixty-nine quantitative trait nucleotides conferring maize resistance to Gibberella ear rot were detected, including eighteen novel loci. Four candidate genes were predicted, and four kompetitive allele-specific PCR markers were developed.
Abstract
Maize Gibberella ear rot (GER), caused by Fusarium graminearum, is one of the most devastating diseases in maize-growing regions worldwide. Enhancing maize cultivar resistance to this disease requires a comprehensive understanding of the genetic basis of resistance to GER. In this study, 334 maize inbred lines were phenotyped for GER resistance in five environments and genotyped using the Affymetrix CGMB56K SNP Array, and a genome-wide association study of resistance to GER was performed using a 3V multi-locus random-SNP-effect mixed linear model. A total of 69 quantitative trait nucleotides (QTNs) conferring resistance to GER were detected, and all of them explained individually less than 10% of the phenotypic variation, suggesting that resistance to GER is controlled by multiple minor-effect genetic loci. A total of 348 genes located around the 200-kb genomic region of these 69 QTNs were identified, and four of them (Zm00001d029648, Zm00001d031449, Zm00001d006397, and Zm00001d053145) were considered candidate genes conferring susceptibility to GER based on gene expression patterns. Moreover, four kompetitive allele-specific PCR markers were developed based on the non-synonymous variation of these four candidate genes and validated in two genetic populations. This study provides useful genetic resources for improving resistance to GER in maize.
Similar content being viewed by others
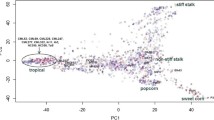
Avoid common mistakes on your manuscript.
Introduction
Maize ear rot is a prevalent fungal disease in maize-growing regions worldwide. It was first reported in Canada in 1923 and then identified in many other countries, including the USA, Mexico, Brazil, Germany, and China (Mesterházy et al. 2012). It can be caused by various pathogens but mostly by Fusarium verticillioides, F. graminearum, and Aspergillus flavus (Gaikpa and Miedaner 2019). The fungi infect maize ears, and then, the husk and kernel are covered with mildew, decreasing the yield and quality. Ear rot reduces output in maize by 5–10% typically and by more than 30% in severely affected areas. In addition, ear rot contaminates kernels with carcinogenic mycotoxins, particularly deoxynivalenol, zearalenone, and fumonisins, which are potential threats to human and animal health (Gaikpa and Miedaner 2019; Mesterházy et al. 2012). Chemical control methods can reduce maize ear rot severity to some extent, but are neither economical nor environmentally friendly (Andriolli et al. 2016). Therefore, genetic resistance is considered a better and more sustainable alternative to prevent yield loss and mycotoxin contamination (Akohoue and Miedaner 2022; Munkvold 2003).
Maize resistance to ear rot is quantitatively inherited in additive and epistatic manners, and highly influenced by the environment and genotype × environment interactions (Gaikpa and Miedaner 2019). A recent work has shown that knocking out ZmFER1, which encodes histidine-rich calcium binding protein, can improve resistance against Fusarium ear rot (FER) caused by F. verticillioides without agronomic penalty in maize (Liu et al. 2022). Two genes, ZmAuxRP1 and ZmWAX2, enhance maize resistance to FER by regulating the growth defense balance and cuticular wax deposition, respectively (Ma et al. 2023; Ye et al. 2019). However, there is limited report on identification of candidate genes for resistance or susceptibility to Gibberella ear rot (GER) caused by F. graminearum in maize.
Thus far, more than 100 quantitative trait loci (QTL) conferring maize resistance to GER have been identified in biparental segregating populations though linkage analysis (Akohoue and Miedaner 2022). For instance, Kebede et al. (2016) mapped 20 QTL conferring maize resistance to GER using a biparental population originating from a cross between Canadian resistant inbred line CO441 and public susceptible line B73. Galiano-Carneiro et al. (2021) identified four QTL conferring maize resistance to GER using six biparental populations originated from crosses between Brazilian resistant genotypes and European susceptible germplasm, and only one QTL, q1, was stable across test environments and populations. Zhou et al. (2021) detected 11 QTL conferring maize resistance to GER, including 5 stable QTL, using a recombinant inbred line (RIL) population originated from a cross between Chinese resistant parent DH4866 and susceptible line T877. Despite the potential genetic power of those QTL, due to their larger interval regions, small genetic effects, and environmental sensitivity, none of them has been successfully cloned (Akohoue and Miedaner 2022).
As a high-resolution gene mapping tool, genome-wide association study (GWAS) has been successfully applied to identify candidate genes conferring disease-resistance traits in maize, such as GST (Wisser et al. 2011), ZmFBL41 (Li et al. 2019), ZmWAX2 (Ma et al. 2023), and ZmBGLU17 (Liu et al. 2024). However, very limited studies have reported on the use of GWAS for maize resistance to GER until recently (Akohoue and Miedaner 2022). Han et al. (2018) failed to detect significant quantitative trait nucleotides (QTNs) associated with resistance to GER using unified mixed model with 130 dent and 114 flint lines from European maize breeding programs. Gaikpa et al. (2021) detected 8 significant QTNs associated with resistance to GER using FarmCPU model with 500 doubled haploid lines derived from two European maize landraces. Yuan et al. (2023) identified 69 and 16 significant QTNs associated with resistance to GER using general and mixed linear models, respectively, with 316 diverse inbred lines.
The statistical models used in previous researches are mainly under the framework of single-locus, which frequently uses the Bonferroni multiple test correction to reduce spurious associations, resulting in the elimination of some positive loci, in particular minor-effect loci (Zhang et al. 2019). As an alternative, several multi-locus GWAS models have been recommended that consider all marker information simultaneously and do not require a multiple test correction (Zhang et al. 2019). Recently, a novel multi-locus model named 3V multi-locus random-SNP-effect mixed linear model (3VmrMLM) was proposed by Li et al. (2022). This model first estimates potentially associated markers using single-marker genome-wide scanning and subsequently detects significant QTN using empirical Bayes and the likelihood ratio test in a multi-locus model; this undoubtedly improves its detection capability. The 3VmrMLM has been successfully applied in the detection of QTNs for complex quantitative traits in plants, such as seed tocopherol content in soybean (Yu et al. 2022), fiber-related traits in cotton (Han et al. 2023b), and seed germination under drought stress in rice (Yang et al. 2024).
To reveal novel QTNs and candidate genes conferring resistance to GER in maize, in this study, an association mapping (AM) population consisting of 334 maize inbred lines was genotyped using an Affymetrix CGMB56K SNP Array and phenotypically evaluated in five filed trials, and GWAS of resistance to GER was conducted using the 3VmrMLM. The study detected multiple novel GER resistance loci, and predicted four susceptibility candidate genes, which could be potentially used for GER resistance breeding program in the near future.
Materials and methods
Plant materials and field trials
The AM population used in this study consists of 334 diverse maize inbred lines, which were mainly collected from the USA and China (Table S1), grown in five environments in China: Nantong, Jiangsu Province (NT, 120° E, 31° N); Xinxiang, Henan Province (XX, 113° E, 35° N); and Sanya, Hainan Province (SY, 108° E, 18° N) in 2020, and NT and SY in 2021. Each field trial followed a randomized complete block design with two replicates. Each line was grown in single row, which was 3 m in length and spaced 0.6 m apart, thereby giving a planting density of 65,000 plants/ha. The agronomic management of the field experiments was the same in the five environments.
Phenotypic evaluation and data analysis
Artificial inoculation was conducted using an aggressive isolate of F. graminearum (strain F0609) according to the description in our previous study (Zhou et al. 2021). Briefly, the strain was cultured on potato dextrose agar medium for approximately 2 weeks, and then several agar plugs with fully grown mycelia were cultured in sterilized mung bean soup at 200 rpm for 2–3 d at 28 °C. The spore suspension was filtered, counted, and adjusted to a concentration of 1 × 106 spores/mL and supplemented with 0.001% of the surfactant Tween-20. For field inoculation, approximately 10 d after silk emergence, the primary ear of each plant was pierced at the base, middle, and top of a husk. Using a 10-cm-long needle, one to two kernels were pierced, but the spindle was not reached. Then 200 µL spore suspension was injected into each of the three wounds.
At the physiological maturity stage, inoculated ears were harvested, and the disease severity of each ear was scored using a 1–7 rating scale (Reid et al. 1994), where 1 = 0%, 2 = 1–3%, 3 = 4–10%, 4 = 11–25%, 5 = 26–50%, 6 = 51–75%, and 7 = 76–100% kernels showing visible disease symptoms over the entire cob.
The phenotypic data were analyzed using R version 4.02 for Windows (https://www.r-project.org/). Descriptive statistics and correlation analysis were conducted using describe function of the psych package and chart.Correlation function of the PerformanceAnalysis package, respectively. Analysis of variance (ANOVA) was performed using lmer function of the lme4 package, respectively. The broad-sense heritability (H2) was estimated as described by Knapp et al. (1985): individual environment H2 (%) = σ2g/(σ2g + σ2e/r) × 100% and multiple environments H2 (%) = σ2g/(σ2g + σ2ge/n + σ2e/nr) × 100%, where σ2g is the genotypic variance, σ2ge is the variance for the interaction of the genotype with the environment, σ2e is the error variance, n is the number of environments, and r is the number of replications. To minimize the effects of the environment, the best linear unbiased prediction (BLUP) for disease severity across the five environments was estimated using lmer function of the lme4 package for further analysis.
Genotyping, population structure, linkage disequilibrium, and relative kinship
Genotypes of the 334 maize inbred lines were evaluated using an Affymetrix CGMB56K SNP Array, which contains 56,000 single nucleotide polymorphisms (SNPs), made by China Golden Marker Biotech Co. (Beijing, China). After quality control, 32,853 SNPs with minor allele frequencies > 5% and missing data < 20% were used for subsequent analysis.
The population structure (Q) was performed using STRUCTURE 2.3 (Pritchard et al. 2000). The number of subpopulations (K) was set from 1 to 10, with five runs for each K value. Parameters were set to 100,000 burn-in periods and 100,000 Markov chain Monte Carlo replications in each run under the admixture model. The K value was estimated using the log likelihood of the data (LnP(D)) and an ad hoc statistic ΔK, based on the rate of change of LnP(D) between successive K values. Nei’s genetic distance was calculated and used to construct a neighbor-joining tree using MEGA-X (Kumar et al. 2018). The linkage disequilibrium (LD) parameter r2 between pairwise SNPs was calculated using PLINK (Purcell et al. 2007), in which the window size was set at 1000 kb, and r2 was set at 0.2. The relative kinship matrix (K) of the 334 maize inbred lines was computed using SPAGeDi 1.3 (Hardy and Vekemans 2002) with negative values between two individuals set to zero.
Genome-wide association study
GWAS was conducted using a compressed variance component mixed model in the 3VmrMLM package, and the method parameter was set to “Single_env”. The threshold of logarithm of odds (LOD) ≥ 3 (or P ≤ 0.0002) was selected as the significance threshold for association signals, and the remaining parameters were set to default values (Li et al. 2022). To confirm the efficiency of the 3VmrMLM, one widely used GWAS statistics model, mixed linear model controlling for population structure and kinship (MLM (Q + K)), was conducted using TASSEL 5.0 (Bradbury et al. 2007), and the threshold was also set at P ≤ 0.0002 (–log10(P) ≥ 3.70).
Candidate gene analysis
All potential candidate genes within 100 kb upstream and downstream of the detected QTNs were identified based on the B73 reference genome v4 (https://www.maizegdb.org/gbrowse). Gene annotation information was obtained from MaizeGDB (https://www.maizegdb.org) and NCBI (https://www.ncbi.nlm.nih.gov). Published gene expression data for maize resistance to GER were collected from Kebede et al. (2018) to identify the differently expressed genes (DEGs). The expression profiles of these DEGs were then validated in a susceptible inbred line T877 and a resistant inbred line DH4866, identified in our previous study (Zhou et al. 2021), using quantitative real-time PCR (qRT-PCR). Finally, genes that were differently expressed in both published gene expression data and the qRT-PCR assay were considered candidate genes for maize resistance to GER.
Quantitative real-time PCR analysis
Maize inbred lines T877 and DH4866 were grown in Nantong, Jiangsu Province, in 2022. The inoculation method was the same as those mentioned earlier for the phenotypic evaluation section. A control group was also evaluated under the same conditions and inoculated with ddH2O. The kernels closely surrounding the inoculation spots were collected from five individual plants, pooled at 1, 3, and 7 days after inoculation, and placed immediately in liquid nitrogen. Total RNA was extracted using Trizol reagent (Invitrogen, Carlsbad, CA, USA) and purified using an RNeasy Plant Mini Kit (Qiagen, Valencia, CA, USA).
RNA reverse transcription reactions were performed using MoScript™ RT111 Super Mix with dsDNase (two-step). qRT-PCR was performed with AceQ® qPCR SYBR® Green Master Mix (Q111-02/03) using an Applied Biosystems™ 7500 Real-Time PCR System. The maize β-tubulin served as an internal control to normalize the expression of each gene, and the relative gene expression was calculated using the 2−ΔΔCt method. Three biological and technical replicates were evaluated separately for each sample. The primers for qRT-PCR are listed in Table S2.
Molecular marker developed
Based on the resequencing information for resistant line DH4866 and susceptible line T877, the non-synonymous variations of the four candidate genes between the two lines were selected and converted into Kompetitive allele-specific PCR (KASP) markers. Then, the KASP markers were used to genotype the AM population used in this study and the RIL population used in a previous study (Zhou et al. 2021) to further confirm the association of the candidate genes with maize resistance to GER. A student’s t-test was used to determine significant differences between the two alleles. Genotyping was performed using the Douglas Scientific® Array Tape™ Platform. All KASP markers are described in Table S3.
Results
Population structure, linkage disequilibrium, and relative kinship
STRUCTURE software was used to calculate the Bayesian clustering from K = 1 to 10, with 5 independent runs for each K. The LnP(D) values increased as K increased from 1 to 10 without an obvious inflection point, and ΔK reached its peak at K = 5 (Fig. 1A), suggesting that this AM population could be divided into 5 subgroups. A neighbor-joining tree was constructed based on Nei’s genetic distance and showed five main clusters for this panel (Fig. 1B), consistent with the STRUCTURE result.
Population structure, linkage disequilibrium, and relative kinship analysis of 334 maize inbred lines. A Estimated ΔK and LnP(D) in the STRUTURE analysis. B Neighbor-joining tree of 334 maize inbred lines. C Linkage disequilibrium across 10 chromosomes. D Pairwise relative kinship of 334 maize inbred lines
The five subpopulations were designated P, Tangsipingtou (TSPT), BSSS, Lancaster (LAN), and Waxy (Table S1). Subgroup P, including 99 inbred lines, derived mainly from American hybrids 3382 and 78,599, contained the representative inbred lines Zheng58 and Qi319. Subgroup TSPT, including 51 inbred lines, derived mainly from Chinese maize landrace-Sipingtou, consisted mainly of inbred lines, such as Huangzaosi and Chang7-2. Subgroup BSSS was derived from the synthetic variety BSSS and included 76 inbred lines, its representative inbred line was B73. Subgroup LAN included 36 inbred lines, its representative inbred line was Mo17. Subgroup Waxy, included 72 inbred lines and belonged to waxy maize.
The average LD decay of the population was estimated at 100 kb, where the LD parameter (r2) dropped to half of its maximum value (Fig. 1C). Additionally, a lower level of genetic relatedness among the 334 inbred lines, as pairwise relative kinship values of 0 accounted for 63.86% of all kinship values, values from 0 to 0.2 accounted for 89.51%, and values greater than 0.5 accounted for only 3.10% (Fig. 1D).
Phenotypic variation and heritability
The descriptive statistics for resistance to GER in the AM population are presented in Table 1. Wide phenotypic variation among the 334 inbred lines was observed and ranged from 1.00 to 7.00 in five environments. The BLUPs of disease severity varied from 2.95 to 6.06, with an average of 4.27. Although a significantly positive correlation was observed among different environments, the correlation coefficients were relatively low (0.22–0.43). ANOVA showed significant differences between genotype, environment, and genotype × environment interactions, and the broad-sense heritability varied largely among all environments, from 65.09% in 21NT to 80.73% in 20SY. These results suggested that maize resistance to GER was mainly controlled by genetic factors, but was environmentally sensitive.
Based on the BLUP values across the five environments, 3 of 334 maize inbred lines showed resistance (disease severity below 3.0) (ID: 78, 129, and 208), and these have the potential to be valuable resources for maize GER resistance breeding. On the contrary, 41 inbred lines showed susceptible, exhibiting very high disease severity of 5.0 or higher. The remaining inbred lines were moderate (Table S1). There was a significant difference in resistance to GER among the five subpopulations. Waxy was the most susceptible, while BSSS was the most resistant (Fig. 2).
Genome-wide association study
A total of 69 QTNs were detected in five environments and BLUPs across all environments using the 3VmrMLM model, and each explained 1.62–7.04% of the phenotypic variation in resistance to GER (Fig. 3 and Table 2). However, only 13 QTNs were detected in five environments and BLUPs across all environments using MLM (Q + K) model (Table S4). Among the 69 QTNs, 13, 14, 7, 10, 12, and 13 QTNs were detected in 20NT, 20SY, 20XX, 21NT, 21SY and BLUP, explaining 34.33, 48.83, 33.97, 41.07, 43.35, and 42.73% of the phenotypic variation, respectively. However, none of these QTNs were detected in two or more environments. Similar results were obtained in previous GWASs of maize resistance to GER (Yuan et al. 2023) and FER (Yao et al. 2020). Referring to previous studies, 51 QTNs have been reported. Thus, the remaining 18 QTNs were considered novel (Table 2).
Manhattan (left) and quartile-quartile (right) plots of genome-wide association study results using the 3VmrMLM model. The six circles of the Manhattan plot from inside to outside show the results from Sanya in 2020 (20SY), Nantong in 2020 (20NT), Xinxiang in 2020 (20XX), Sanya in 2021 (21SY), Nantong in 2021 (21NT), and best linear unbiased prediction (BLUP)
Identification of candidate genes
According to the LD in this AM population (Fig. 1C), 348 potential candidate genes were identified 100 kb upstream and downstream of the 69 QTNs (Table S5). Based on the transcriptome data published by Kebede et al. (2018), four (Zm00001d029648, Zm00001d031449, Zm00001d006397, and Zm00001d053145) of them were considered DEGs (|fold change|≥ 5) (Fig. 4A). Among them, Zm00001d029648 encodes ent-copalyl diphosphate synthase, Zm00001d031449 encodes lipoxygenase 13, Zm00001d006397 encodes cytokinin-O-glucosyltransferase, and Zm00001d053145 encodes purple acid phosphatase. These candidate genes were significantly upregulated after inoculation with F. graminearum, and expressed at high levels in susceptible line B73 compared with resistant line CO441. The qRT-PCR assay showed that these four genes also were significantly induced by F. graminearum, and expressed at high levels in susceptible line T877 compared with resistant line DH4866 (Fig. 4B). Therefore, we hypothesize that these four genes may be involved in maize susceptibility to GER.
The expression level of four candidate genes in transcriptome data (A) and qRT-PCR assay (B). Sample names are displayed under the heat map, where 1d, 2d, 3d, and 7d represent 1, 2, 3, and 7 days after inoculation, respectively. The fold change/relative expression is the ratio of being treated with Fusarium graminearum versus water. * and ** represent a significance level of P < 0.05 and P < 0.01, respectively
Development and validation of molecular markers
For Zm00001d029648, the A/G (T877/DH4866) variation at the 1816th position in the coding sequencing region, which caused one amino acid substitution from aspartate to serine, was used as a target site to design KASP marker, K648. Significant differences of disease severity were observed between alleles AA and GG in the AM and RIL populations (Fig. 5A). GG was the resistance allele, and AA was the susceptibility allele. The disease severity of GG was 5.01 and 4.53% lower than that of AA in the AM and RIL populations, respectively.
The effect analysis of four KASP markers in the association mapping (AM) and recombinant inbred line (RIL) populations. A The genetic effect of K648 in the AM and RIL populations. B The genetic effect of K449 in the AM and RIL populations. C The genetic effect of K397 in the AM and RIL populations. D The genetic effect of K145 in the AM and RIL populations
For Zm00001d031449, the A/G (T877/DH4866) variation at the 455th position in the coding sequencing region, which caused one amino acid substitution from arginine to glutamine, was used as a target site to design KASP marker, K449. Significant differences of disease severity were observed between alleles AA and GG in the AM population, while no statistical difference was detected between alleles AA and GG in the RIL population (Fig. 5B). GG was the resistance allele, and AA was the susceptibility allele. The disease severity of GG was 7.78% lower than that of AA in the AM population.
For Zm00001d006397, the A/G (T877/DH4866) variation at the 137th position in the coding sequencing region, which caused one amino acid substitution from valine to alanine, was used as a target site to design KASP marker, K397. Significant differences of disease severity were observed between alleles AA and GG in the AM and RIL populations (Fig. 5C). GG was the resistance allele, and AA was susceptibility allele. The disease severity of GG was 3.60 and 5.59% lower than that of AA in AM and RIL populations, respectively.
For Zm00001d053145, the G/A (T877/DH4866) variation at the 395th position in the coding sequencing region, which caused one amino acid substitution from glycine to asparagine, was used as a target site to design KASP marker, K145. Significant differences of disease severity were observed between alleles AA and GG in the AM and RIL populations (Fig. 5D). AA was the resistance allele, and GG was the susceptibility allele. The disease severity of AA was 3.37 and 4.86% lower than that of GG in the AM and RIL populations, respectively.
Discussion
In this study, 69 QTNs were identified using the 3VmrMLM. Of these QTNs, 51 (74%; 51/69) co-localized with previously reported genetic loci conferring maize resistance to GER. Moreover, 10 QTNs (AX-86307484, AX-86281987, AX-86282886, AX-95647844, AX-86313662, AX-86299528, AX-86274115, AX-86318905, AX-86296412, and AX-86324800) were located in meta-QTL regions for maize ear rot, as estimated by Akohoue and Miedaner (2022). This suggested that the QTNs detected using the 3VmrMLM here were reliable, even though none of them could be detected in more than one environment or explained more than 10% of the phenotypic variance. More importantly, 18 novel QTNs conferring maize resistance to GER were identified in this study.
Combining genetic and transcriptome analysis is an effective approach to refining candidate genes for target traits. Yao et al. (2020) identified 21 candidate genes for maize resistance to FER by integrating GWAS and transcriptome analysis. Combining QTL mapping and transcriptome analysis, 5 and 81 candidate genes for maize resistance to GER and FER were identified, respectively (Cao et al. 2022; Kebede et al. 2018). In another study, 59 candidate genes for maize resistance to FER and/or GER were identified by combining meta-QTL and transcriptome analysis (Akohoue and Miedaner 2022). In this study, four candidate genes for maize susceptibility to GER, which could be significantly induced by F. graminearum, were identified by combining GWAS, transcriptomic data reported by Kebede et al. (2018), and qRT-PCR assay. These four genes were expressed at high levels in susceptible lines compared with resistant lines. TaHRC, a gene conferring Fusarium head blight susceptibility in wheat, could be significantly induced after inoculation with F. graminearum, and was more highly expressed in susceptible line than in resistance line (Su et al. 2019). ChSK1, a gene conferring quantitative susceptibility to maize southern leaf blight, was highly induced in susceptible line after C. heterostrophus infection, but less so in resistance line (Chen et al. 2023). Based on the above results, we speculate that these four candidate genes might be associated with maize susceptibility to GER.
Among these candidate genes, Zm00001d029648, corresponding to the previously confirmed gene An2, displayed higher transcript accumulation following fungal elicitation, and drove the production of non-volatile antibiotic families termed kauralexins and dolabralexins (Christensen et al. 2018; Harris et al. 2005; Mafu et al. 2018). In addition, the an2 mutant displayed increased susceptibility to FER (Christensen et al. 2018). Zm00001d031449, a lipoxygenase gene, was located in a meta-QTL for maize ear rot estimated by Akohoue and Miedaner (2022) and was speculated to be involved in regulating cell death in maize leaves (Barghahn et al. 2023). The expression level of maize lipoxygenase pathway genes can be induced after F. verticillioides inoculation (Maschietto et al. 2015). Two lipoxygenase genes, ZmLOX3 and ZmLOX12, have been confirmed to be involved in maize resistance to ear rot, and the lox3 mutant displayed increased resistance to FER but susceptibility to Aspergillus ear rot caused by Aspergillus flavus (Gao et al. 2009, 2007). The lox12 mutant exhibited higher susceptibility to FER (Christensen et al. 2014). Zm00001d006397 encodes cytokinin-O-glucosyltransferase, which is a key enzyme for regulating the level and function of cytokinin, and could be induced by heat stress (Han et al. 2023a). Cytokinin-mediated immunity or susceptibility has been studied extensively (Albrecht and Argueso 2017), and cytokinin-O-glucosyltransferase genes have a significant role in alleviating cytokinin-induced immunity in rice (Dauda et al. 2022). Zm00001d053145 encodes purple acid phosphatase, which has been suggested to generate reactive oxygen species as an immune response (Kaija et al. 2002), and it was induced under low phosphorus stress (Gonzalez-Munoz et al. 2015). In summary, these four genes could respond to biotic or abiotic stress, and the function of their protein is related to pathogen resistance. Thus, functional validation is worth in further study.
Apart from the four genes mentioned above, seven genes (Zm00001d031437, Zm00001d002312, Zm00001d002313, Zm00001d038534, Zm00001d018931, Zm00001d020533, and Zm00001d012550) contained leucine-rich repeat domain that is commonly existing in innate immune receptor proteins in plants (Padmanabhan et al. 2009), and two genes (Zm00001d031447 and Zm00001d039842) encoded the protein belonging to disease-resistance family proteins. These genes may also be involved in maize resistance to GER, although they were not differentially expressed in the transcriptome data published by Kebede et al. (2018). More experiments are needed to elucidate their functions.
Previous studies have demonstrated that non-synonymous mutations in genes can change their protein function and affect crop disease resistance. For example, a single base substitution results in a histidine-to-aspartic acid substitution in maize multiple disease-resistance gene GST, defining GST substrate specificity and biochemical activity (Wisser et al. 2011). In rice, a single base substitution results in a cysteine-to-arginine substitution in SPL36, causing spontaneous cell death and enhanced resistance to bacterial pathogens (Rao et al. 2021). In this study, the non-synonymous variations of four candidate genes were mined and converted into four KASP markers. The effects of these four markers were successfully validated in the AM and RIL populations (except for K449 in the RIL population). However, the effect of a single KASP marker could only reduce disease severity by less than 10%. These results further indicate that maize resistance to GER is controlled by polygenes with minor effects, as described in previous studies (Brauner et al. 2017; Kebede et al. 2016; Yuan et al. 2023; Zhou et al. 2021). Thus, it is better to pyramid these four resistance alleles in future maize disease breeding efforts.
Data availability
The datasets used in this study are available from the corresponding author upon reasonable request.
References
Akohoue F, Miedaner T (2022) Meta-analysis and co-expression analysis revealed stable QTL and candidate genes conferring resistances to Fusarium and Gibberella ear rots while reducing mycotoxin contamination in maize. Front Plant Sci 13:1050891
Albrecht T, Argueso CT (2017) Should I fight or should I grow now? The role of cytokinins in plant growth and immunity and in the growth-defence trade-off. Ann Bot 119:725–735
Andriolli CF, Casa RT, Kuhnem PR, Bogo A, Zancan RL, Reis EM (2016) Timing of fungicide application for the control of Gibberella ear rot of maize. Trop Plant Pathol 41:264–269
Barghahn S, Saridis G, Mantz M, Meyer U, Melluh JC, Misas Villamil JC, Huesgen PF, Doehlemann G (2023) Combination of transcriptomic, proteomic, and degradomic profiling reveals common and distinct patterns of pathogen-induced cell death in maize. Plant J 116:574–596
Bradbury PJ, Zhang Z, Kroon DE, Casstevens TM, Ramdoss Y, Buckler ES (2007) TASSEL: software for association mapping of complex traits in diverse samples. Bioinformatics 23:2633–2635
Brauner PC, Melchinger AE, Schrag TA, Utz HF, Schipprack W, Kessel B, Ouzunova M, Miedaner T (2017) Low validation rate of quantitative trait loci for Gibberella ear rot resistance in European maize. Theor Appl Genet 130:175–186
Cao A, de la Fuente M, Gesteiro N, Santiago R, Malvar RA, Butrón A (2022) Genomics and pathways involved in maize resistance to Fusarium ear rot and kernel contamination with fumonisins. Front Plant Sci 13:866478
Chen C, Zhao Y, Tabor G, Nian H, Phillips J, Wolters P, Yang Q, Balint-Kurti P (2023) A leucine-rich repeat receptor kinase gene confers quantitative susceptibility to maize southern leaf blight. New Phytol 238:1182–1197
Christensen SA, Nemchenko A, Park YS, Borrego E, Huang PC, Schmelz EA, Kunze S, Feussner I, Yalpani N, Meeley R, Kolomiets MV (2014) The novel monocot-specific 9-lipoxygenase ZmLOX12 is required to mount an effective jasmonate-mediated defense against Fusarium verticillioides in maize. Mol Plant-Microbe Interact 27:1263–1276
Christensen SA, Sims J, Vaughan MM, Hunter C, Block A, Willett D, Alborn HT, Huffaker A, Schmelz EA (2018) Commercial hybrids and mutant genotypes reveal complex protective roles for inducible terpenoid defenses in maize. J Exp Bot 69:1693–1705
Dauda WP, Shanmugam V, Tyagi A, Solanke AU, Kumar V, Krishnan SG, Bashyal BM, Aggarwal R (2022) Genome-wide identification and characterisation of cytokinin-O-glucosyltransferase (CGT) genes of rice specific to potential pathogens. Plants 11:917
Gaikpa DS, Miedaner T (2019) Genomics-assisted breeding for ear rot resistances and reduced mycotoxin contamination in maize: methods, advances and prospects. Theor Appl Genet 132:2721–2739
Gaikpa DS, Kessel B, Presterl T, Ouzunova M, Galiano-Carneiro AL, Mayer M, Melchinger AE, Schon CC, Miedaner T (2021) Exploiting genetic diversity in two European maize landraces for improving Gibberella ear rot resistance using genomic tools. Theor Appl Genet 134:793–805
Galiano-Carneiro AL, Kessel B, Presterl T, Gaikpa DS, Kistner MB, Miedaner T (2021) Multi-parent QTL mapping reveals stable QTL conferring resistance to Gibberella ear rot in maize. Euphytica 217:2
Gao XQ, Shim WB, Gobel C, Kunze S, Feussner I, Meeley R, Balint-Kurti P, Kolomiets M (2007) Disruption of a maize 9-lipoxygenase results in increased resistance to fungal pathogens and reduced levels of contamination with mycotoxin fumonisin. Mol Plant-Microbe Interact 20:922–933
Gao XQ, Brodhagen M, Isakeit T, Brown SH, Gobel C, Betran J, Feussner I, Keller NP, Kolomiets MV (2009) Inactivation of the lipoxygenase ZmLOX3 increases susceptibility of maize to Aspergillus spp. Mol Plant-Microbe Interact 22:222–231
Giomi GM, Kreff ED, Iglesias J, Fauguel CM, Fernandez M, Oviedo MS, Presello DA (2016) Quantitative trait loci for Fusarium and Gibberella ear rot resistance in Argentinian maize germplasm. Euphytica 211(3):287–294
Gonzalez-Munoz E, Avendano-Vazquez AO, Montes RA, de Folter S, Andres-Hernandez L, Abreu-Goodger C, Sawers RJ (2015) The maize (Zea mays ssp. mays var. B73) genome encodes 33 members of the purple acid phosphatase family. Front Plant Sci 6:341
Han S, Utz HF, Liu W, Schrag TA, Stange M, Wurschum T, Miedaner T, Bauer E, Schon CC, Melchinger AE (2016) Choice of models for QTL mapping with multiple families and design of the training set for prediction of Fusarium resistance traits in maize. Theor Appl Genet 129(2):431–444
Han S, Miedaner T, Utz HF, Schipprack W, Schrag TA, Melchinger AE (2018) Genomic prediction and GWAS of Gibberella ear rot resistance traits in dent and flint lines of a public maize breeding program. Euphytica 214:6
Han X, Zhang D, Hao H, Luo Y, Zhu Z, Kuai B (2023a) Transcriptomic analysis of three differentially senescing maize (Zea mays L.) inbred lines upon heat stress. Int J Mol Sci 24:9782
Han Z, Ke H, Li X, Peng R, Zhai D, Xu Y, Wu L, Wang W, Cui Y (2023b) Detection of epistasis interaction loci for fiber quality-related trait via 3VmrMLM in upland cotton. Front Plant Sci 14:1250161
Hardy OJ, Vekemans X (2002) SPAGeDi: a versatile computer program to analyse spatial genetic structure at the individual or population levels. Mol Ecol Notes 2:618–620
Harris LJ, Saparno A, Johnston A, Prisic S, Xu M, Allard S, Kathiresan A, Ouellet T, Peters RJ (2005) The maize An2 gene is induced by Fusarium attack and encodes an ent-copalyl diphosphate synthase. Plant Mol Biol 59:881–894
Kaija H, Alatalo SL, Halleen JM, Lindqvist Y, Schneider G, Vaananen HK, Vihko P (2002) Phosphatase and oxygen radical-generating activities of mammalian purple acid phosphatase are functionally independent. Biochem Biophys Res Commun 292:128–132
Kebede AZ, Woldemariam T, Reid LM, Harris LJ (2016) Quantitative trait loci mapping for Gibberella ear rot resistance and associated agronomic traits using genotyping-by-sequencing in maize. Theor Appl Genet 129:17–29
Kebede AZ, Johnston A, Schneiderman D, Bosnich W, Harris LJ (2018) Transcriptome profiling of two maize inbreds with distinct responses to Gibberella ear rot disease to identify candidate resistance genes. BMC Genom 19:131
Knapp SJ, Stroup WW, Ross WM (1985) Exact confidence intervals for heritability on a progeny mean basis1. Crop Sci 25:192–194
Kumar S, Stecher G, Li M, Knyaz C, Tamura K (2018) MEGA X: molecular evolutionary genetics analysis across computing platforms. Mol Biol Evol 35:1547–1549
Li N, Lin B, Wang H, Li X, Yang F, Ding X, Yan J, Chu Z (2019) Natural variation in ZmFBL41 confers banded leaf and sheath blight resistance in maize. Nat Genet 51:1540–1548
Li M, Zhang YW, Zhang ZC, Xiang Y, Liu MH, Zhou YH, Zuo JF, Zhang HQ, Chen Y, Zhang YM (2022) A compressed variance component mixed model for detecting QTNs and QTN-by-environment and QTN-by-QTN interactions in genome-wide association studies. Mol Plant 15:630–650
Liu C, Kong M, Zhu J, Qi X, Duan C, Xie C (2022) Engineering null mutants in ZmFER1 confers resistance to ear rot caused by Fusarium verticillioides in maize. Plant Biotechnol J 20:2045–2047
Liu C, He S, Chen J, Wang M, Li Z, Wei L, Chen Y, Du M, Liu D, Li C, An C, Bhadauria V, Lai J, Zhu W (2024) A dual-subcellular localized β-glucosidase confers pathogen and insect resistance without a yield penalty in maize. Plant Biotechnol J 22:1017–1032
Ma P, Liu E, Zhang Z, Li T, Zhou Z, Yao W, Chen J, Wu J, Xu Y, Zhang H (2023) Genetic variation in ZmWAX2 confers maize resistance to Fusarium verticillioides. Plant Biotechnol J 21:1812–1826
Mafu S, Ding Y, Murphy KM, Yaacoobi O, Addison JB, Wang Q, Shen Z, Briggs SP, Bohlmann J, Castro-Falcon G, Hughes CC, Betsiashvili M, Huffaker A, Schmelz EA, Zerbe P (2018) Discovery, biosynthesis and stress-related accumulation of dolabradiene-derived defenses in maize. Plant Physiol 176:2677–2690
Martin M, Miedaner T, Dhillon BS, Ufermann U, Kessel B, Ouzunova M, Schipprack W, Melchinger AE (2011) Colocalization of QTL for Gibberella ear rot resistance and low mycotoxin contamination in early European maize. Crop Sci 51(5):1935–1945
Martin M, Miedaner T, Schwegler DD, Kessel B, Ouzunova M, Dhillon BS, Schipprack W, Utz HF, Melchinger AE (2012) Comparative quantitative trait loci mapping for Gibberella ear rot resistance and reduced deoxynivalenol contamination across connected maize populations. Crop Sci 52(1):32–43
Maschietto V, Marocco A, Malachova A, Lanubile A (2015) Resistance to Fusarium verticillioides and fumonisin accumulation in maize inbred lines involves an earlier and enhanced expression of lipoxygenase (LOX) genes. J Plant Physiol 188:9–18
Mesterházy Á, Lemmens M, Reid LM (2012) Breeding for resistance to ear rots caused by Fusarium spp. in maize—a review. Plant Breed 131:1–19
Munkvold GP (2003) Cultural and genetic approaches to managing mycotoxins in maize. Annu Rev Phytopathol 41:99
Padmanabhan M, Cournoyer P, Dinesh-Kumar SP (2009) The leucine-rich repeat domain in plant innate immunity: a wealth of possibilities. Cell Microbiol 11:191–198
Pritchard JK, Stephens MJ, Donnelly PJ (2000) Inference of population structure using multilocus genotype data. Genetics 155:945–959
Purcell S, Neale B, Todd-Brown K, Thomas L, Ferreira MAR, Bender D, Maller J, Sklar P, de Bakker PIW, Daly MJ, Sham PC (2007) PLINK: a tool set for whole-genome association and population-based linkage analyses. Am J Hum Genet 81:559–575
Rao Y, Jiao R, Wang S, Wu X, Ye H, Pan C, Li S, Xin D, Zhou W, Dai G, Hu J, Ren D, Wang Y (2021) SPL36 encodes a receptor-like protein kinase that regulates programmed cell death and defense responses in rice. Rice 14:34
Reid LM, Mather DE, Bolton AT, Hamilton RI (1994) Evidence for a gene for silk resistance to Fusarium graminearum Schw. ear rot of maize. J Hered 85:118–121
Su Z, Bernardo A, Tian B, Chen H, Wang S, Ma H, Cai S, Liu D, Zhang D, Li T, Trick H, St Amand P, Yu J, Zhang Z, Bai G (2019) A deletion mutation in TaHRC confers Fhb1 resistance to Fusarium head blight in wheat. Nat Genet 51:1099–1105
Wen J, Shen Y, Xing Y, Wang Z, Han S, Li S, Yang C, Hao D, Zhang Y (2020) QTL mapping of resistance to Gibberella ear rot in maize. Mol Breed 40:94
Wisser RJ, Kolkman JM, Patzoldt ME, Holland JB, Yu J, Krakowsky M, Nelson RJ, Balint-Kurti PJ (2011) Multivariate analysis of maize disease resistances suggests a pleiotropic genetic basis and implicates a GST gene. Proc Nati Acad Sci USA 108:7339–7344
Xiang K, Zhang ZM, Reid LM, Zhu XY, Yuan GS, Pan GT (2010) A meta-analysis of QTL associated with ear rot resistance in maize. Maydica 55:281–290
Yang B, Chen S, Zheng Z, Zeng J, Liu J, Zhao H, Zheng Y (2024) Genome-wide association studies for rice seed germination under drought stress using 3VmrMLM. Food Energy Secur 13:e529
Yao L, Li Y, Ma C, Tong L, Du F, Xu M (2020) Combined genome-wide association study and transcriptome analysis reveal candidate genes for resistance to Fusarium ear rot in maize. J Integr Plant Biol 62:1535–1551
Ye J, Zhong T, Zhang D, Ma C, Wang L, Yao L, Zhang Q, Zhu M, Xu M (2019) The auxin-regulated protein ZmAuxRP1 coordinates the balance between root growth and stalk rot disease resistance in maize. Mol Plant 12:360–373
Yu K, Miao H, Liu H, Zhou J, Sui M, Zhan Y, Xia N, Zhao X, Han Y (2022) Genome-wide association studies reveal novel QTLs, QTL-by-environment interactions and their candidate genes for tocopherol content in soybean seed. Front Plant Sci 13:1026581
Yuan G, He D, Shi J, Li Y, Yang Y, Du J, Zou C, Ma L, Gao S, Pan G, Shen Y (2023) Genome-wide association study discovers novel germplasm resources and genetic loci with resistance to Gibberella ear rot caused by Fusarium graminearum. Phytopathology 113:1317–1324
Zhang YM, Jia ZY, Dunwell JM (2019) Editorial: the applications of new multi-locus GWAS methodologies in the genetic dissection of complex traits. Front Plant Sci 10:100
Zhou G, Li S, Ma L, Wang F, Jiang F, Sun Y, Ruan X, Cao Y, Wang Q, Zhang Y, Fan X, Gao X (2021) Mapping and validation of a stable quantitative trait locus conferring maize resistance to Gibberella ear rot. Plant Dis 105:1984–1991
Acknowledgements
We thank LetPub (www.letpub.com) for its linguistic assistance during the preparation of this manuscript. The bioinformatics analysis of this work was supported in part by the high-performance computing platform of Bioinformatics Center, Nanjing Agricultural University.
Funding
This study was supported by the Youth Talent Support Program of Jiangsu Association for Science and Technology (TJ-2023-052), the Key Research and Development Program of Jiangsu Province (BE2022343), the Seed Industry Revitalization Announcement and Leading Project of Jiangsu Province (JBGS[2021]054; JBGS[2021]002), the Natural Science Foundation of Nantong City (JC2023048), and Youth Doctoral Foundation of Jiangsu Yanjiang Institute Science (YJBS(2023)004).
Author information
Authors and Affiliations
Contributions
GZ and XG designed the experiment and drafted the manuscript; GZ and LM performed experiments and conducted data analysis; GZ, LM, CZ and FX performed phenotypic evaluation; GZ, YX, QW, DH and XG revised the manuscript. All authors have read and approved the final manuscript.
Corresponding authors
Ethics declarations
Conflict of interest
The authors declare no conflict of interest.
Ethical standards
The experiments were performed in compliance with the current laws of China.
Additional information
Communicated by Thomas Miedaner.
Publisher's Note
Springer Nature remains neutral with regard to jurisdictional claims in published maps and institutional affiliations.
Supplementary Information
Below is the link to the electronic supplementary material.
Rights and permissions
Springer Nature or its licensor (e.g. a society or other partner) holds exclusive rights to this article under a publishing agreement with the author(s) or other rightsholder(s); author self-archiving of the accepted manuscript version of this article is solely governed by the terms of such publishing agreement and applicable law.
About this article
Cite this article
Zhou, G., Ma, L., Zhao, C. et al. Genome-wide association study and molecular marker development for susceptibility to Gibberella ear rot in maize. Theor Appl Genet 137, 222 (2024). https://doi.org/10.1007/s00122-024-04711-z
Received:
Accepted:
Published:
DOI: https://doi.org/10.1007/s00122-024-04711-z