Abstract
Non-perennial rivers have attracted significant attention due to the progressive increase in water demand and pollution, river engineering side effects and climate change. The present study investigates the interannual, seasonal and spatial variability of nine water quality variables (pH, colour, turbidity, biochemical oxygen demand, total phosphorus, total inorganic nitrogen, chlorophyll-a, total solids and thermotolerant coliforms) from 93 monitoring river sites distributed over 11 watersheds in the highly populated Brazilian semiarid region. Shapiro–Wilk and Kruskal–Wallis tests were applied for assessing data normality and statistical differences, respectively. Additionally, principal component analysis (PCA) was performed for comparison between temporal and spatial data sets, while the Standardized Precipitation Index (SPI) was used to characterize meteorological drought. The results revealed that spatial variability is more evident than temporal variability. In the temporal scale, the interannual variability is more relevant than the seasonal one. The discharge of wastewater seems to attenuate a seasonal hydrological effect on water quality. There is a deterioration of water quality in most watersheds in the drier years, even in the rainy season. This is especially for colour, total phosphorus, total inorganic nitrogen and thermotolerant coliforms. The abrupt transition from dry to wet years also played an important role in changes in river water quality. This study provides new insights for the understanding of the water quality patterns in non-perennial rivers. In addition, we go on to identify important aspects for the management of these water sources, such as the need for wastewater discharge guidelines within scope of each watershed, and restrictions on the use of soil and water in the driest periods.
Similar content being viewed by others
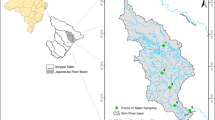
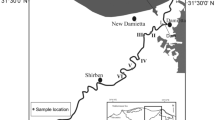
Explore related subjects
Discover the latest articles, news and stories from top researchers in related subjects.Avoid common mistakes on your manuscript.
1 Introduction
Semiarid rivers typically present low discharge rates due to the disconnectivity between the upstream and downstream areas, and the main river courses and floodplains or adjacent riverside areas (Grill et al., 2019; Rubel & Kottek, 2010). Additionally, there is vertical discontinuity to the groundwater and temporal discontinuity due to rainfall seasonality and recurrent droughts (Costa et al., 2013; Grill et al., 2019). These rivers characterized by hydrological discontinuity are non-perennial, and can be found in the north and southeast of the African continent, in the southwest of North America, in the central region of Asia, in Australia and in the northeast of South America (Messager et al., 2021). Considering that the non-perennial watercourses have a substantial and growing share of the global river hydrological network (currently > 50%) due to the progressive increase in water demand, river engineering side effects and climate change (Datry et al., 2018; Gamvroudis et al., 2017; Grill et al., 2019), a better understanding of their hydrology and biochemical processes is of utmost importance for improving integrated water resources management, not only in drylands.
The intermittent and temporary mechanisms of the river flow discontinuity are very pronounced in drought-hit regions, such as the State of Ceará in the Brazilian semiarid, where a high-density reservoir network has been built to overcome water scarcity during drought occurrences (Costa et al., 2021; Lima Neto et al., 2011; Mamede et al., 2012, 2018). Although the reservoir network delays the beginning of the hydrological drought, it confers more discontinuity in longitudinal dimension as the recovering of river flow after dry years is also delayed, which is also accentuated by the anthropic water demand (van Oel et al., 2018).
Previous water quality studies in the Brazilian semiarid have focused on surface water reservoirs (e.g. Fraga et al., 2020; Lorenzi et al., 2018; Mesquita et al., 2020; Moura et al., 2019; Nascimento Filho et al., 2019; Raulino et al., 2021; Rocha & Lima Neto, 2021a, b; Wiegand et al., 2021). Compared to surface reservoirs, the river data is much scarcer. Moreover, the specific knowledge on the river quality is limited to a few publications (Cruz et al., 2019; Lima et al., 2018; Oliveira Filho & Lima Neto, 2018). This hampers any attempt of quantifying the vulnerabilities of the river ecosystems to droughts, urban interventions and land use changes, which potentially affect both spatially and temporally the water quality in tropical semiarid watersheds in Brazil. This study aims to highlight the main seasonal, interannual and spatial changes related to the river water quality and their man drivers at management scale, because this knowledge can support decision-making regarding the use of water and land, sanitation and water pollution control, which are fundamental for human health and biodiversity in aquatic ecosystems (Dantas et al., 2020).
The main objective of our study is to assess the river water quality at seasonal, interannual and spatial scales from 93 monitoring river sites distributed over 11 watersheds in the State of Ceará, Brazilian semiarid. The specific objectives are (1) to evaluate the statistical significance of interannual and seasonal water quality differences; (2) to compare the water quality variability among the selected watersheds; and (3) to infer the dominant environmental and anthropogenic processes that drive the variations of the water quality at the watershed scale. Multiple site water quality monitoring data over the years in similar climatic regions can provide robust insights into the main factors that affect river water quality at the watershed scale, considering seasonal and annual time scales. With such data, for example, we would be able to identify the most polluted watersheds, the seasonal effects on the changes in water quality, and to estimate interannual trends to improve water management.
2 Material and Methods
2.1 Study Area
The study site consists of eleven watersheds or hydrosystems covering a total area of 142,380 km2 in the State of Ceará, Brazil (Fig. 1). The hydrosystems are called Acaraú—AC, Banabuiú—BN, Coreaú—CO, Alto Jaguaribe—AJ, Médio Jaguaribe—MJ, Baixo Jaguaribe—BJ, Litoral—LT, Metropolitanas—MT, Salgado—SL and Sertões de Crateús—SC. Based on data from the information system of the Ceará Geosocioeconomic Information System (Ipece, 2021), the MT watersheds have a highest demographic density (273 inhab km−2) followed by the SL (79 inhab km−2), BJ (55 inhab km−2), AC (42 inhab km−2), CR (40 inhab km−2), LT (40 inhab km−2), CO (35 inhab km−2), MJ (35 inhab km−2), AJ (26 inhab km−2), BN (26 inhab km−2) and SC (16 inhab km−2). Considering all watersheds, this value corresponds to 62 inhab km−2. Approximately 78% of the population of the State of Ceará lives in urban areas and 22% in rural areas. The highest rate of urbanization occurs in the hydrographic region of metropolitan basins (MT). The percentages of urban areas in the hydrographic regions are different, ranging from 0.2% in the MJ and SC and to 3.6% in the MT.
The climate is tropical semiarid, where the annual rainfall is about 800 mm, while the potential evapotranspiration is about 2200 mm, according to the data provided by the Foundation of Meteorology and Water Resources of the State of Ceará—FUNCEME (Ceará, 2018a; Funceme, 2019). During the study period (2013–2018), the average annual rainfall was 635 mm, which was relatively lower than the above-mentioned climatological normal (800 mm). The annual rainfall distribution for each watershed is shown in Fig. 2. The large and medium-sized rivers usually flow from February to June due to regular or above-average rainy seasons, while they dry naturally out during the other months (Costa et al., 2021). Interannually, there is a high variability of streamflow discharges with a coefficient of variation generally above 1.0 (Güntner & Bronstert, 2004). The construction of a large number of reservoirs (> 28,000) and land use changes in the watersheds also enhance the variability of the river flow (Souza Filho, 2018). Among the more than 28,000, there are reservoirs with a capacity of less than 0.5 hm3 up to reservoirs with more than 750 hm3 (Ceará, 2018b). Moreover, a total of 155 are considered strategic reservoirs, as they have regional importance in water storage (Ceará, 2018b). The largest reservoir in the state of Ceará has a storage capacity of 6700 hm3 and is located in the Jaguaribe River, one of the longest among non-perennial rivers in the world (Castro et al., 2020).
2.2 Data Set
2.2.1 Water Quality Data
River water quality data were made available by the Environmental Agency of the State of Ceará (SEMACE). The monitoring variables included pH, colour (true), turbidity, total inorganic nitrogen—TIN (nitrate, nitrite and ammonia), biochemical oxygen demand—BOD, total phosphorus—TP, chlorophyll-a, total solids—TS and thermotolerant coliforms—TC. The monitoring sites are detailed in Table 1 and Fig. 1. The monitoring samples were carried out quarterly and considered strategic areas for qualitative assessment of the river network. However, in a few periods, some river sites became inaccessible or totally dried, hindering a more homogeneous sampling. Thus, there is missing data in 2013 and 2014 for some hydrosystems, but a complete quarterly data set for 2015–2018. Water sampling and laboratory analysis followed standard methods published by the National Water Agency (Brazil, 2011). These water quality variables have been used in Brazil to assess the waterbody quality conditions. So, waters, which are classified as class II (see class limits in Table 2), can be used for human supply after conventional treatment (coagulation, flocculation, sedimentation, filtration and disinfection) and to protect aquatic ecosystems (Brazil, 2005). We assumed that at least 90% of samples must be within the class II limits, in order to classify the waterbody as class II (based on Sabiá, 2008).
2.2.2 Precipitation Data
The monthly watershed precipitation was obtained from FUNCEME, considering a 30-year historical series (1988–2018). In the study area, the precipitation has been monitored by 575 rainfall stations. The monthly watershed values are provided by FUNCEME based on these stations and using the Thiessen polygon method (Thiessen, 1911).
2.3 Precipitation Time Series Analysis
We defined the rainy season (Gregory, 1979). So, if it rains a month more than the monthly rainfall median, this month belongs to the rainy season. For each hydrographic region, a monthly rainfall median was calculated to classify the months as being part of the rainy season. Consequently, each hydrographic region presented a minimum monthly rainfall: AC (32 mm), BJ (32 mm), BN (36 mm), CO (35 mm), CR (31 mm), LT (30 mm), MT (55 mm), SC (22 mm) and SL (43 mm).
The occurrence of droughts for each watershed was assessed transforming the monthly precipitation to the 12-month Standardized Precipitation Index (SPI-12) (McKee et al., 1993) using the MDM (Meteorological Drought Monitoring) software (Salehnia et al., 2017). The SPI is an index used worldwide for drought identification (Stagge et al., 2015). A drought occurs when the SPI values are continually negative and reach − 1.00 or less (McKee et al., 1993). The SPI value categories are presented in Table 3.
2.4 Water Quality Statistical Analysis
Firstly, the data sets of each watershed were separated year-by-year from 2013 to 2018 (interannual scale), and by rainy and dry seasons considering all years together (seasonal scale). Then, the Shapiro–Wilk test (Royston, 1982; Shapiro & Wilk, 1965) was applied to assess the data normality for the interannual and seasonal data sets for each watershed. As the majority of the data (64%) did not fit a normal distribution, the Kruskal–Wallis test (Walpole et al., 1998) was applied to evaluate the statistically significant difference between the aforementioned data sets. The statistical tests were carried out considering the complete data and excluding the outliers. After obtaining the p values and contrasting them with the test hypotheses at a 95% confidence level, the percentage of the data set that did not present significant statistical difference at seasonal and interannual scales was calculated. The software R (version 3.5.3) was used to construct the graphs and perform the above-mentioned statistical analysis.
Finally, PCA was carried out in Past 3.0. Standardized data matrices were generated for each data set. The matrix columns correspond to the water quality variables (see Sect. 2.2.1). In this sense, 20 PCAs were performed, i.e. one PCA for the whole data, two PCAs for seasonal separation (dry and rainy), six PCAs for interannual separation (2013–2018) and eleven PCAs for spatial separation (among the watersheds). This procedure was used to assess in which data set the reduction in dimensionality can explain the greater variability of the data after orthogonal linear transformation for principal components (PC), as well as to verify which variables most influence this variability (Ojok et al., 2017). Hence, eigenvalues and factor loadings were evaluated, considering that (1) the PCA method selects PCs with eigenvalues greater than one (> 1.0) and the eigenvalue indicates the amount of variance explained by the PC (Kaiser, 1960, Braeken and Assen, 2017); and (2) the factor loading explains the importance of original variables to a particular component and it is classified as strong (> 0.75), moderate (0.75–0.50) and weak (0.50–0.30) (Liu et al., 2003; Olsen et al., 2012).
3 Results and Discussion
3.1 SPI Values and Occurrence of Dry Years
Figure 3 shows the SPI-12 from 2013 to 2018, indicating different trends among the studied watersheds (see Fig. 1). In the AC and CR watersheds, the SPI average remained negative between 2013 and 2017. In the BN, CO, MJ and LT watersheds, this occurred between 2014 and 2016. For AJ, SL and SC watersheds, the mean annual values of negative SPI were identified during the periods 2015–2017, 2015–2016 and 2014, 2016–2017, respectively. In MT, all years remained with positive SPI, while for BJ, the opposite occurred. Overall, the dry years occurred mainly from 2013 to 2017, while 2018 was characterized by an increase in rainfall. It is important to highlight that a rainy 2018 did not imply the return of normal river flow conditions due to the cumulative effect of the previous long meteorological drought. The SPI-12 results are consistent with those identified in other semiarid regions with a predominance of occurrences in the near normal range, reaching frequently moderately dry, severely dry and extremely dry stages (Shadeed, 2012; Suliman et al., 2020; Surendran et al., 2019). Our results are also in agreement with Brito et al. (2017), who found more severe droughts in the Northeast of Brazil between 2011 and 2016 (baseline: 1981–2016).
Twelve-month Standardized Precipitation Index (SPI) for each study watershed from 2013 to 2018 (baseline period: 1988–2018). Each bar on the plots corresponds to the monthly SPI value, highlighting the beginning of each year evaluated (ex: Jan-14). The dry period is identified for the negative SPI values that reach the lines in red tones
3.2 Water Quality Patterns
Table 4 presents the percentage of the samples of each river water quality variable that can be classified as class II (Brazil, 2005). The lowest percentages of agreement to the evaluated standards were TP, followed by colour (true), BOD, total dissolved solids, TC, ammonia, chlorophyll-a, pH, turbidity, nitrite and nitrate. Moreover, taking into account the sum of the percentages of each variable of each hydrosystem, the watershed with the lowest water quality was MT, followed by SC, BN, SL, BJ, CR, AJ, LT, CO, AC and MJ.
The variations in the pH values indicated that the river waters were in general in the neutral range (6 to 9) (see Figs. 4 and 5). However, increased alkalinity was found downstream of a system of stabilization ponds for sewage treatment and in the vicinity of an urban area of the SC watershed. This effect may be driven by algal activity that can raise pH to values above 9.5 in the warmest periods of the year (Kgopa et al., 2018). Consistently, in the dry season (Fig. 4), the pH values tended to be higher, as observed by Andem et al. (2014), except for BN. The MJ and MT watersheds had significant pH differences between seasons and years. The highest pH changes in MJ occurred between 2014 and 2015 (Fig. 5), when an extremely dry phase took place, which might have driven the pH peak by reducing the river flow, consequently, increasing the phytoplankton activity. Despite not having an expressive dry phase from 2013 to 2018, the MT watershed was influenced by intense urban occupation and industrial activities, which can affect the pH stability in the rivers (Bhadrecha et al., 2016). In general, the river waters presented a strong “buffering capacity” to seasonal and interannual climatic variations, most likely due to alkalinity (Ojok et al., 2017). Turbidity rarely exceeded the permitted limit for national classification (100 NTU). In addition to the values shown in Fig. 4, there were outliers of up to 1000 NT in AJ, CO and MT. Turbidity increased mainly in the rainy season of the moist years, which can be justified by the carriage of sediment in this period (Medeiros et al., 2010). Similar results were also found by Ahmadi and Moradkhani (2019) in other climatic zones. Another effect, which may be associated with the low turbidity in the study area, would be the presence of a high-density reservoir network (Mamede et al., 2012). Since the small and micro-sized reservoirs are usually empty at the beginning of the rainy season, they work as a sediment trap, retaining a significant fraction of the sediment yield in the watershed (Lima Neto et al., 2011; Mamede et al., 2018). Fast flow, however, especially after prolonged droughts, favoured the occurrence of the outliers. Interannually, statistical differences occurred for AC and AJ, probably generated by the increase in rainfall in 2017 and 2018, being more evident for AJ.
Nevertheless, colour exceeded the permitted values for national classification (75 uH) in most cases. The highest colour values occurred in drier years, but with a predominance of increase in the rainy season for most watersheds, consistently with the results of Ahipathy and Puttaiah (2006) and Anhwange et al. (2012). After a prolonged dry period, the effect of re-mobilization and transport of accumulated sediments and organic materials in the river basin to the outlet lead to the increase of colour values. The highest peaks were found in the SC watershed, which also presented the driest conditions during the study period. The statistical differences for the BN and CO watershed occurred because BN had one of the lowest accumulated precipitation, while CO presented the highest amount of accumulated rainfall during the study period, unfolding two extremes of hydroclimatic conditions that can influence changes in the colour of the river waters.
According to the World Health Organization (WHO, 2017), pH, turbidity and colour are acceptability aspects for drinking water and important operating parameters of water quality, although they are not directly related to health. The recommended pH for water consumption should range from 6.5 to 8.5. Turbidity above 4 NTU is already visible to human vision, while and generally a colour of up to 15 uH is accepted. It is noteworthy that the values of the national legislation for water class II are based on the conservation of water resources and the assumption that the water will be treated before being consumed for drinking.
According to the national water quality standards, BOD must be below 5 mg L−1. BOD is not a health-based guideline for safe drinking water recommended by the WHO (WHO, 2011). However, rivers are globally considered polluted when they present BOD above 5 mg L−1 and may be related to waters that affect the health of the population as it is related to the high concentration of organic matter, solids and pathogens (Wen et al., 2017). The percentages of BOD in any river basin did not show more than 90% agreement with waters classified as class II. The maximum BOD values (Fig. 6) were observed for MT (129.00 mg L−1), SL (96.40 mg L−1) and AJ (83.90 mg L−1). The highest concentrations of BOD occurred in the dry season and in the urbanized river stretches that received sanitary effluent discharges in MT, SL, SC and BJ watersheds. In fact, the impact of human activities on water quality is more noticeable in the dry season (Ahipathy & Puttaiah, 2006; Andem et al., 2014; Moyel & Hussain, 2015; Yu et al., 2016). On the other hand, the effect of untreated wastewater release into the rivers on the deterioration of water quality is widely discussed in the literature (e.g. Blanco et al., 2019; Dutta et al., 2018; Wang et al., 2015), which point out that the supply of organic matter and nutrients act in the depletion of dissolved oxygen and in the ecosystem imbalance, causing the death of fish and stimulating the growth of phytoplankton. These occurrences are further facilitated by the low speeds of a large part of the semiarid river stretches and, in some periods, the non-flow river conditions. The MJ watershed presented, on the other hand, the best water quality conditions for BOD in the river waters. Its monitoring sites are downstream of the largest reservoir in Ceará and close to a pumping station for water supply. These sites also drain very low-population density areas, which are less susceptible to high organic loads. Nevertheless, there was only a statistically significant difference for AC and MT at seasonal scale. The MT watershed had BOD concentrations higher than AC. Most likely, rainfall in MT generated a dilution effect on BOD concentration. Thus, watersheds with lower water quality had small seasonal variation, except for extreme precipitation.
Eutrophic waters were observed in urban areas and/or in river stretches that receive excessively wastewater discharge, as also seen for high colour and BOD concentrations. Some watersheds (MT, SL and SC) were evidently more eutrophic, with respect to total phosphorus—TP (see Figs. 6 and 7). The CO and AJ watersheds presented TP increase in the rainy season, which was also found in other areas by Cruz et al. (2019) and Dalu et al. (2019). However, TP in the MT watershed presented an increase in the dry season, similarly to the results reported by Meter et al. (2019). According to Bowes et al. (2012) reported in the UK, if there is a predominance of punctual discharges, the phosphorus load decreases with the increase in river flow due to the dilution effect, while in the case of predominant diffuse sources, an increase in the load proportional to the flow is expected, due to the release of phosphorus retained in the sediment (Mainstone & Parr, 2002). Interannually, significant differences (without outliers) were found for a large number of watersheds (AC, AJ, MJ, BJ, LT, MT and SL). More frequent interannual TP variations were also reported by McKee et al. (2000). Our results indicate that in the drier years, there is an increase in the concentration of TP, which can be associated with the above-mentioned effect of dominant punctual discharges, as suggested by Bowes et al. (2012). According to data from the National Sanitation Information System, sewage collection coverage in the state of Ceará is only 26% (Brazil, 2019). The highest percentages of sewage coverage are concentrated in urban areas, and range from 4 (in MJ) to 38% (in MT). The improvement of technologies to tertiary level could attenuate effluent concentrations from point sources in these water sources, as also verified by Wang et al. (2015).
Regarding the total organic nitrogen—TIN (nitrate, nitrite and ammonia), the ammonia concentrations exceeded the national standards for seven watersheds (BN, CO, CR, BJ, MT, SL and SC). The significant ammoniacal fraction (Pearson correlation coefficient between TIN and ammonia of 0.97) is an indication of significant wastewater discharges in the watersheds (Wang et al., 2015). Moreover, a positive Pearson correlation coefficient of 0.70 was identified between BOD and ammonia. Seasonal and interannual differences are presented in Figs. 6 and 7, respectively. Significant seasonal differences were found only for the AC, CO and SC watersheds, with increased TIN in the rainy season (Fig. 8). The increase in nitrogen concentrations in the rainy season may be related to the (re-) suspension of organic matter accumulated in the sediment or carried by runoff. Seasonal differences depend on the balance among river water pollution, nitrogen degradation (especially in the dry season) and nitrogen input in the rainy season. It seems that the former, which maintain a continuous high nitrogen source throughout the year, is much more relevant than the latter two, causing no significant seasonal differences in most of the watersheds.
The difficulty of comparing nutrient levels at seasonal scale was also discussed by Reisinger et al. (2016) and Ramírez et al. (2018), who associated the increase in nutrients in the transition between periods of low to high flows. Interannually (Fig. 9), statistical differences were found in the AC, CO, AJ, MJ, BJ and SL watersheds. In these watersheds, the interannual difference was characterized by an increase in 2015, 2016 and 2017 (relatively drier years) and a reduction in 2018 (relatively moist year). In CO, the statistical difference occurred mainly due to the decrease in nitrogen in 2018, the wettest year. Some outliers were observed in the rainy years. Note that floods and droughts alter the dynamics of inorganic nitrogen fractions (Xue et al., 2016). Moreover, the increase in nutrient concentration in the rainy season could also have been influenced by sediment erosion. The shallow soils of the Brazilian semiarid region are susceptible to erosion in high rainfall events (Medeiros et al., 2016).
The concentration of chlorophyll-a was characteristic of eutrophic waters, as reported by Pacheco and Lima Neto (2017) and Nguyen et al. (2019). This can be attributed to the growth of algae, the low-flow conditions in the dry periods and the irrigation return flow, as reported by Rocha Junior et al. (2018). Chlorophyll-a increase in the dry season was probably a result of increased water temperature and reduced water flow rate, which potentially favoured phytoplankton growth (Nguyen et al., 2019; Onwuteaka & Choko, 2018). All watersheds showed significant differences between years. The increase in chlorophyll-a in the dry season of the wetter years may also be related to the availability of nutrients. Wu et al. (2016) found that the variability of chlorophyll-a concentration was related to different types of sediment input to the rivers, which may be an explanation for the present results. Additionally, according to Jarvie et al. (2006), during rainy season, particulate phosphorus represents the large portion that flows into the river. However, the reactive phosphorus associated with the consequences of algae growth occurs mainly during drier periods.
The percentages of TDS that did not present more than 90% agreement with freshwater class II were mainly related to the watersheds under coastal influence (CR, BJ, LT and MT). TDS levels above about 600 mg L−1 make freshwater increasingly unpalatable (WHO, 2021). Moreover, high total solid (TS) (Fig. 9) concentrations (> 1000 mg L−1) were observed in monitoring sites (22 in total) located in the municipalities (7 in total) with a relevant estuarine zone. The estuarine zone in Brazil covers localities facing the sea or within 50 km from the coast to the interior (Brazil, 2015). A main environmental impact of the estuarine zone in the semiarid northeast of Brazil is shrimp farming (Lacerda et al., 2021). Significant seasonal differences of TS were only found for the coastal CO and MT watersheds, increasing in the dry season, which has been associated with an increase in the concentration of organic matter (Laraque et al., 2013; Hassan et al., 2017). However, high TS concentration was also observed in the rainy season due to domestic effluents and surface runoff from the cultivated fields, as reported by Andem et al. (2014) and Vaishali and Punita (2013). The interannual differences were only significant for three coastal watersheds (AC, CR and MT) and two countryside watersheds (AJ, BN), but with more frequent outliers in the drier years, especially for the MT watershed. Also, in the drier years, the highest values were found for the watersheds that showed significant statistical difference.
The watersheds that presented more microbiological risks for drinking water were MT and SL. In MT, more than 60% of the TC values are above the recommended limit (Brazil, 2005). In SL, two of the monitoring sites have all TC values equal to or greater than national standard. It is noteworthy that these limits are for raw water. For drinking water, these waters are well above what is allowed, as there must be no coliforms in any 100 mL of sample (WHO, 2021). Thus, they must be treated if they are used for human supply. The rainfall-runoff events have a double effect for river water TC concentration: the dilution of TC in the river and the carrying of sediments and waste into the river network. A relevant effect of the latter effect could have produced an increase in TC for the study watersheds in the rainy season, similar to the findings of Anhwange et al. (2012) and Nguyen et al. (2016). The AJ and SL watersheds had a higher median in the dry season, as reported by Reder et al. (2015). However, significant seasonal differences were found only in CO and SC watersheds. At interannual scale, significant differences were produced by a massive increase of TC in some drier years for AC, CR and MJ. In the AJ watershed, a very high concentration of TC was observed not only in the driest year (2015), but also in the wettest one (2018). Uncertainties about the variation in the concentration of coliforms in rivers are related to the versatile metabolism of microorganisms in terms of their multiplication rate and limitations in their counting in laboratory tests (Marques et al., 2019). Additionally, the watersheds with influence of the seawater showed lower TC values, except for those with densely populated cities, such as MT. This can be explained by the decrease of coliforms with the increase in salinity and dilution due to marine water inflow (Liu & Chan, 2015).
In summary, considering all watersheds, interannual variability was more relevant than seasonal variability, except for pH, turbidity and TIN (see Table 5) for complete data. However, by removing the outliers from the analysis, interannual variability also became predominant for turbidity and TIN. In this sense, the continuous release of wastewater can undermine the natural seasonal effects of variations in water quality. Thus, interannual variations become more evident due to the significant change in the amount of rainfall in this temporal scale.
Figure 10 summarizes the spatio-temporal variability of river water quality. The illustrated arrows indicate dry (reddish) or wet (bluish) conditions. An extremely complex behaviour of water quality was observed. While some variables increased in the dry periods, both at seasonal and interannual scales (pH, BOD and TS), other variables increased in the rainy periods (turbidity). On the other hand, some variables increased in the wet season but decreased in wet years (colour, TP, TIN and TC), while the opposite occurred with other variables (chlorophyll-a). The spatial differences in water quality variation are more significant; thus, actions to improve water quality as well as guidelines for the release of effluents should be thought of locally. Another important aspect is the interannual influence; in the rainy season of the driest years, the water quality has a significant deterioration, and the population should be alerted in this period about the uses of water, whether for potability, irrigation or bathing. Seasonal differences, especially in the more anthropogenic affected watersheds, are less noticeable, so the rainy season does not always represent a dilution of pollutants and an improvement in water quality. Reservoirs have a strong influence on changes in water quality, especially on turbidity, due to sediment retention. Water quality variability is greater for the dry season, for dry years and for drier regions, as seen from the PCA data.
3.3 PCA and Spatio-temporal Variability of Water Quality
The plots for the eigenvalues of the two first principal components for each data set are shown in Fig. 11. The larger the eigenvalues, the better the principal components of linear transformation can explain the variance of the data. Separation by data set showed eigenvalues greater than the PCA for complete data (Fig. 11a). The dry season (D) had the two first principal components that better explain the variance in data than those from the rainy season (R) (Fig. 11b). On the interannual scale, this occurred for 2014 (Fig. 11c). On the spatial scale, two watersheds are highlighted (SC and SL) (Fig. 11d).
Eigenvalue plots for two first principal components for each PCA. a Complete data. b Seasonal separation (R—data in the rainy season and D—data in the dry season). c Annual separation. d Spatial separation (Acaraú—AC, Banabuiú—BN, Coreaú—CO, Alto Jaguaribe—AJ, Médio Jaguaribe—MJ, Baixo Jaguaribe—BJ, Litoral—LT, Metropolitanas—MT, Salgado—SL and Sertões de Crateús—SC)
The factor loadings represent the relative importance of the projections of the original variables (Ojok et al., 2017). Table 6 shows the percentage of the variance explained of the PCAs and the more representative factor loadings. The eigenvalues are representative up to the fourth or fifth component. The ammonia and BOD variables showed the highest loadings in the first component of the most performed PCAs, with moderate (0.50–0.75) and weak importance (0.30–0.50). This is common under conditions driven by domestic wastewater and agricultural effluents, as reported by Lap et al. (2021).
The results indicate the complexity of the data variation, where the eigenvalues were representative up to the fourth or fifth component (see Table 6). Only the PCAs performed for the interannual and spatial separation were able to represent more than 70% of the data variance, which is an important factor in choosing the variables of the quality determinants (Tripathi & Singal, 2019; Razmkhah et al., 2010). The lower eigenvalues in the rainy season, when compared to those in the dry season, indicated the variability generated by the occurrence of rainfall over the river basin, as also reported by Ojok et al. (2017). This variability provided by the wetter season occurs mainly in the first rains after the dry period.
The data sets of the drier years from 2013 to 2017 provided the highest eigenvalues in the first two PCs. So, the drier years showed a higher percentage of the variance of the PCs. This may indicate that the drier years offered more definitive characteristics in relation to the water quality variables. When the severity of the drought has intensified from 2015 to 2017, the water quality gradually showed a higher variance with more outliers. Thus, the effects of the rainy season in a drier year can generate annual variability in the water quality, which justifies the reduction of the explanation by the eigenvalues in the PCs. In 2018, this variability becomes even more evident due to the less expressiveness of the PCA, as it is characterized as a year of transition with relatively wetter patterns.
Regarding the spatial scale, the SC watershed is an inland area, typically drier and strongly impacted by wastewater discharges. The same characteristics can be drawn for the SL watershed, although it showed milder periods of drought. The MT watershed is influenced by coastal rains that could provide greater variability in water quality. These meteorological effects are even stronger in the AC and LT watersheds, whose water quality was in better conditions when compared to other watersheds.
The BOD, ammonia and TP variables were the most important in the first principal component in MT and SL watersheds. These watersheds have in common the high population density and impacts of urban activities on the water resources, similar to the trends identified by Singh et al. (2005) and Qin et al. (2020).
4 Conclusions
We investigated river water quality patterns at watershed scale in relation to the drought occurrences and the anthropogenic effects in a large semiarid region, which differ from those typically found in other climate zones. This work provides a synthesis that advances the knowledge of the complex influence of climate, hydrology and anthropogenic effects on the river water quality in drylands, which may help improve the integrated water resources management. The main findings of this study are as follows:
Spatial variability was more relevant than interannual ones, followed by seasonal variability. This was found by applying statistical tests, inter-hydrosystem comparison and the PCA analysis. The processes that may have driven this finding were (a) the continuous discharge of effluents throughout the seasons that undermined the seasonal hydrological effects, and (b) the combination of a prolonged drought and moist rainfall years that governed the streamflow regimes. The transition from dry to moist years had a relevant role on the river water quality changes.
The driest years, even during their rainy season, affected water quality negatively. This was mainly for TP, NIT, TC, deteriorating water quality for drink water and bathing. This may indicate the need for stricter guidelines for the discharge of wastewater in dry periods.
We also observed that while pH, BOD and TS increased in the dry periods, both at seasonal and interannual scales, turbidity increased in the rainy periods. On the other hand, colour, TP, TIN and TC increased in the rainy season but decreased in wet years, while the opposite occurred with chlorophyll-a.
Anthropogenic impacts on water sources can end up inhibiting the natural effects of rains on the dilution of pollutants, revealing different patterns between hydrographic regions. Planning for mitigating actions to control nutrient release in this region is urgent, especially given the concentrations of total phosphorus, whether in the implementation of tertiary-level wastewater treatment systems, or in the control of land use. The urban watersheds (MT, SL, SC and AJ) presented the highest concentration of organic matter and nutrients. The rivers, which flow through urban areas, were the most polluted ones, principally due to sanitary effluents.
It is noteworthy that a severe drought occurred in the region during the study period, and the existence of some outliers in the data may be a result of favoured extreme conditions. Investigating these patterns with a longer time series may reveal new patterns for long-term wet years. The findings of this work may be basis for water quality modelling studies not only for the study area, but also for regions with similar climate, socio-economic and land use conditions. They may help to define initial and boundary conditions, as well as model structure. In addition, investigations may be carried out regarding depollution measures, based on what is presented in this research.
Data Availability
Data will be made available on reasonable request.
Code Availability
Not applicable.
References
Ahipathy, M. V., & Puttaiah, E. T. (2006). Ecological characteristics of Vrishabhavathy River in Bangalore (India). Environmental Geology, 49(1), 1217–2122. https://doi.org/10.1007/s00254-005-0166-0
Ahmadi, B., & Moradkhani, H. (2019). Revisiting hydrological drought propagation and recovery considering water quantity and quality. Hydrological Processes, 33(10), 1492–1505. https://doi.org/10.1002/hyp.13417
Andem, A. B., Okorafor, K. A., Udofia, U. U., & Oku, E. E. (2014). Seasonal variation in limnological characteristics of the upper, middle and lower reaches of Calabar River, Southern Nigeria. International Journal of Biological Sciences, 1(2), 52–60.
Anhwange, B. A., Agbaji, E. B., & Gimba, E. C. (2012). Impact assessment of human activities and seasonal variation on River Benue, within Makurdi Metropolis. International Journal of Science and Technology., 2(5), 248–254.
Bhadrecha, M. H., Khatri, N., & Tyagi, S. (2016). Rapid integrated water quality evaluation of Mahisagar river using benthic macroinvertebrates. Environmental Monitoring and Assessment, 188(4), 254. https://doi.org/10.1007/s10661-016-5256-9
Blanco, M., Rizzi, J., Fernandes, D., Colin, N., Maceda-Veiga, A., & Porte, C. (2019). Assessing the impact of waste water effluents on native fish species from a semi-arid region, NE Spain. Science of the Total Environment, 654, 218–225. https://doi.org/10.1016/j.scitotenv.2018.11.115
Bowes, M. J., Gozzard, E., Johnson, A. C., et al. (2012). Spatial and temporal changes in chlorophyll-a concentrations in the River Thames basin, UK: Are phosphorus concentrations beginning to limit phytoplankton biomass? Science of the Total Environment, 426, 45–55. https://doi.org/10.1016/j.scitotenv.2012.02.056
Braeken, J., & van Assen, M. A. L. M. (2017). An empirical Kaiser criterion. Psychological Methods, 22(3), 450–466. https://doi.org/10.1037/met0000074
Brazil. (2005). CONAMA Resolution º 357. Provides for the classification of water bodies and environmental guidelines for their classification, as well as establishing the conditions and standards for the release of wastewaters, and other measures. Brasília, Brazil.
Brazil. (2011). Resolution 724/2011. Establishes standardized procedures for the collection and preservation of surface water samples for monitoring purposes. National Water Agency (ANA). Brasília , Brazil.
Brazil. (2015). National Coastal Management Plan. Ministry of the Environment, 1, 23.
Brazil. (2019). National Sanitation Information System. http://www.snis.gov.br/.
Brito, S. S. B., Cunha, A. P. M. A., Cunningham, C. C., et al. (2017). Frequency, duration and severity of drought in the Semiarid Northeast Brazil region. International Journal of Climatology, 38(2), 517–529. https://doi.org/10.1002/joc.5225
Castro, A., Costa, A. T., Carneiro Neto, J. A., Morais, J. S. D., & Claudino-Sales, V. (2020). Scientific expedition to the upper Jaguaribe River (Ceará): Identification of the source of possibly largest temporary river in the world. Caderno De Geografia, 30(63), 2020. https://doi.org/10.5752/p.2318-2962.2020v30n63p956
Ceará. (2018a). PLANERH - Chapter 6 - Concentrated waterbalance. https://www.srh.ce.gov.br/planerh/.
Ceará. (2018b). PLANERH. Chapter 7 - Water infrastructure. https://www.srh.ce.gov.br/planerh/.
Costa, A. C., Foerster, S., Araújo, J. C., et al. (2013). Analysis of channel transmission losses in a dryland river reach in north-eastern Brazil using streamflow series, groundwater level series and multi-temporal satellite data. Hydrological Processes, 27(7), 1046–1060. https://doi.org/10.1002/hyp.9243
Costa, A. C., Estácio, A. B. S., Souza Filho, F. A., & Lima Neto, I. E. (2021). Monthly and seasonal streamflow forecasting of large dryland catchments in Brazil. Journal of Arid Land, 13(3), 205–223. https://doi.org/10.1007/s00000-021-0097-x
Cruz, M. A. S., Gonçalves, A. A., Aragão, R., et al. (2019). Spatial and seasonal variability of the water quality characteristics of a river in Northeast Brazil. Environmental Earth Sciences, 78, 68. https://doi.org/10.1007/s12665-019-8087-5
Dalu, T., Wasserman, R. J., Magoro, M. L., et al. (2019). River nutrient water and sediment measurements inform on nutrient retention, with implications for eutrophication. Science of the Total Environment., 684, 296–302. https://doi.org/10.1016/j.scitotenv.2019.05.167
Dantas, J. C., Silva, R. M., & Santos, C. A. G.(2020). Drought impacts, social organization, and public policies in northeastern Brazil: A case study of the upper Paraíba river basin. Environmental Monitoring and Assessment, 192(5), 192–317. https://doi.org/10.1007/s10661-020-8219-0.
Datry, T., Foulquier, A., Corti, R., et al. (2018). A global analysis of terrestrial plant litter dynamics in non-perennial waterways. Nature Geoscience, 11, 497–503. https://doi.org/10.1038/s41561-018-0134-4
Dutta, S., Dwivedi, A., Kumar, M., et al. (2018). Use of water quality index and multivariate statistical techniques for the assessment of spatial variations in water quality of a small river. Environmental Monitoring and Assessment, 190(12), 718. https://doi.org/10.1007/s10661-018-7100-x
Fraga, R. F., Rocha, S. M. G., & Lima Neto, I. E. (2020). Impact of flow conditions on coliform dynamics in an urban lake in the Brazilian semiarid. Urban Water Journal, 17(1), 43–53. https://doi.org/10.1080/1573062X.2020.1734948
Funceme. (2019). Foundation of Meteorology and Water Resources of the State of Ceará (FUNCEME). Rain calendar. https://funceme.br/app-calendario/.
Gamvroudis, C., Dokou, Z., Nikolaidis, N. P., et al. (2017). Impacts of surface and groundwater variability response to future climate change scenarios in a large Mediterranean watershed. Environmental Earth Sciences, 76, 385. https://doi.org/10.1007/s12665-017-6721-7
Grill, G., Lehner, B., Thieme, M., et al. (2019). Mapping the word’s free-flowing rivers. Nature, 569, 215–221. https://doi.org/10.1038/s41586-019-1111-9
Güntner, A., & Bronstert, A. (2004). Representation of landscape variability and lateral redistribution processes for large-scale hydrological modelling in semi-arid areas. Journal of Hydrology, 297(1), 136–161. https://doi.org/10.1016/j.jhydrol.2004.04.008
Gregory, S. (1979). The definition of wet and dry periods for discrete regional units. Weather, 34, 363–369.
Hassan, T., Parveen, S., Bhat, B. N., & Ahmad, U. (2017). Seasonal variations in water quality parameters of River Yamuna, India. International Journal of Current Microbiology and Applied Sciences, 6(5), 694–712. https://doi.org/10.20546/ijcmas.2017.605.079.
Ipece. (2021). Ceará Geosocioeconomic Information System. Ceará Institute for Research and Socioeconomic Strategy. http://ipecedata.ipece.ce.gov.br/ipece-data-web/.
Jarvie, H. P., Neal, C., & Withers, P. J. A. (2006). Sewage-effluent phosphorus: A greater risk to river eutrophication than agricultural phosphorus? Science of the Total Environment, 360(1–3), 246–253. https://doi.org/10.1016/j.scitotenv.2005.08.038
Kaiser, H. F. (1960). The application on electronic computers to factor analysis. Educational and Psychological Measurement, 20(1), 141–151. https://doi.org/10.1177/001316446002000116
Kgopa, P. M., Mashela, P. W., & Manyevere, A. (2018). Suitability of treated wastewater with respect to pH, electrical conductivity, selected cations and sodium adsorption ratio for irrigation in a semi-arid region. Water SA, 44(4), 551–556. https://doi.org/10.4314/wsa.v44i4.04
Lacerda, L. D., Ward, R. D., Godoy, M. D. P., Meireles, A. J. A., Borges, R., & Ferreira, A. C. (2021). 20-years cumulative impact from shrimp farming on mangroves of Northeast Brazil. Frontiers in Forests and Global Change, 4, 653096. https://doi.org/10.3389//ffgc.2021.653096
Lap, B. Q., Nam, N. H., & Ahn, B. T. K. (2021). Monitoring water quality in Lien Son irrigation system of Vietnam and identification of potential pollution sources by using multivariate analysis. Water, Air and Soil Pollution, 232, 187. https://doi.org/10.1007/s11270-021-05137-9
Laraque, A., Moquet, J., Alkattan, R., et al. (2013). Seasonal variability of total dissolved fluxes and origin of major dissolved elements within a large tropical river: The Orinoco, Venezuela. Journal of South American Earth Sciences, 44, 4–17. https://doi.org/10.1016/j.jsames.2012.12.011
Lima Neto, I. E., Wiegand, M. C., & Araújo, J. C. (2011). Sediment redistribution due to a dense reservoir network in a large semi-arid Brazilian basin. Hydrological Sciences Journal, 56(2), 319–333. https://doi.org/10.1080/02626667.2011.553616
Lima, B. P., Mamede, G. L., & Lima Neto, I. E. (2018). Monitoring and modelling water quality in a semiarid basin. Engenharia Sanitaria e Ambiental, 23(1), 125–135. https://doi.org/10.1590/s1413-41522018167115
Liu, W., & Chan, W. (2015). Assessment of the climate change impacts on fecal coliform contamination in a tidal estuarine system. Environmental Monitoring and Assessment, 187(12), 728. https://doi.org/10.1007/s10661-015-4959-7
Liu, C., Lin, K., & Kuo, Y. (2003). Application of factor analysis in the assessment of groundwater quality in a blackfoot disease area in Taiwan. Science of the Total Environment, 313, 77–89. https://doi.org/10.1016/S0048-9697(02)00683-6
Lorenzi, A. S., Cordeiro-Araújo, M. K., Chia, M. A., & Bittencourt-Oliveira, M. C. (2018). Cyanotoxin contamination of semiarid drinking water supply reservoirs. Environmental Earth Sciences, 77, 595. https://doi.org/10.1007/s12665-018-7774-y.
Mainstone, C. P., & Parr, W. (2002). Phosphorus in rivers - Ecology and management. Science of the Total Environment, 282–283, 25–47. https://doi.org/10.1016/s0048-9697(01)00937-8
Mamede, G. L., Araújo, N. A. M., Schneider, C. M., et al. (2012). Overspill avalanching in a dense reservoir network. PNAS, 109(19), 7191–7195. https://doi.org/10.1073/pnas.1200398109
Mamede, G. L., Guentner, A., Medeiros, P. H. A., et al. (2018). Modeling the effect of multiple reservoirs on water and sediment dynamics in a semiarid catchment in Brazil. Journal of Hydrologic Engineering, 23(12). https://doi.org/10.1061/(asce)he.1943-5584.0001701.
Marques, L.O. A., Taffarello, D., Calijuri, M. C., et al. (2019). Phosphorus and thermotolerant coliforms’ loads in Brazilian watersheds with limited data: Considerations on the integrated analysis of water quality and quantity. Brazilian Journal of Water Resources, 24(3). https://doi.org/10.1590/2318-0331.241920170137.
McKee, T. B., Doesken, N. J., & Kleist, J. (1993). The relationship of drought frequency and duration to time scales. Eighth Conference on Applied Climatology, Anaheim, California, 1(1), 17–22.
McKee, L., Eyre, B., & Hossain, S. (2000). Intra- and interannual export of nitrogen and phosphorus in the subtropical Richmond River catchment. Australia. Hydrological Processes, 14(10), 1787–1809. https://doi.org/10.1002/1099-1085(200007)14:103.0.co;2-z
Messager, M. L., Lehner, B., Cockburn, C., et al. (2021). Global prevalence of non-perennial rivers and streams. Nature, 594, 391–397. https://doi.org/10.1038/s41586-021-03565-5
Medeiros, P. H. A., Guentner, A., Francke, T., et al. (2010). Modelling spatio-temporal patterns of sediment yield and connectivity in a semi-arid catchment with the WASA-SED model. Hydrological Sciences Journal, 55(4), 638–648. https://doi.org/10.1080/02626661003780409
Medeiros, P. R. P., Cavalcante, G. H., & Brandini, N. (2016). Inter-annual variability on the water quality in the Lower São Francisco River (NE-Brazil). Acta Limnologica Brasiliensia, 28,. https://doi.org/10.1590/s2179-975x3515
Mesquita, J. B. F., Lima Neto, I. E., Raabe, A., & Araújo, J. C. (2020). The influence of hydroclimatic conditions and water quality on evaporation rates of a tropical lake. Journal of Hydrology, 590. https://doi.org/10.1016/j.jhydrol.2020.125456.
Meter, K. J. V., Chowdhury, S., Byrnes, D. K., et al. (2019). Biogeochemical asynchrony: Ecosystem drivers of seasonal concentration regimes across the great lakes basin. Limnology and Oceanography, 65(4), 848–862. https://doi.org/10.1002/lno.11353
Moura, D. D., Lima Neto, I. E., Clemente, A., et al. (2019). Modeling phosphorus exchange between bottom sediment and water in tropical semiarid reservoirs. Chemosphere, 240,. https://doi.org/10.1016/j.chemosphere.2019.125686
Moyel, M. S., & Hussain, N. A. (2015). Water quality assessment of the Shatt al-Arab River, Southern Iraq. Journal of Coastal Life Medicine, 3(6). https://doi.org/10.12980/jclm.3.2015j5-26.
Nascimento Filho, S. L., França, E. J., De Júnior, M. M., et al. (2019). Interactions between benthic microalgae, nutrients and benthic macroinvertebrates in reservoirs from the semi-arid Neotropical region. Fundamental and Applied Limnology, 192(3), 237–254. https://doi.org/10.1127/fal/2019/1180
Nguyen, H. T. M., Le, Q. T. P., Garnier, J., et al. (2016). Seasonal variability of faecal indicator bacteria numbers and die-off rates in the Red River basin, North Viet Nam, 6, 21644. Scientific Reports. https://doi.org/10.1038/srep21644
Nguyen, T. T. N., Némery, J., Gratiot, N., et al. (2019). Nutrient dynamics and eutrophication assessment in the tropical river system of Saigon – Dongnai (southern Vietnam), 653, 370–383. Science of the Total Environment. https://doi.org/10.1016/j.scitotenv.2018.10.319
Ojok, W., Wasswa, J., & Ntambi, E. (2017). Assessment of seasonal variation in water quality in River Rwizi using multivariate statistical techniques, Mbarara Municipality, Uganda. Journal of Water Resource and Protection, 9, 83–97. https://doi.org/10.4236/jwarp.2017.91007
Olsen, R. L., Chappell, R. W., & Loftis, J. C. (2012). Water quality sample collection, data treatment and results presentation for principal components analysis – Literature review and Illinois River watershed case study. Water Research, 46(9), 3110–3122. https://doi.org/10.1016/j.watres.2012.03.028
Oliveira Filho, A. A., & Lima Neto, I. E. (2018). Water quality modeling at Poti river in Teresina (Piauí, Brazil). Engenharia Sanitaria e Ambiental, 23(1). https://doi.org/10.1590/s1413-41522017142354.
Onwuteaka, J., & Choko, P. (2018). Phytoplankton community response to seasonal changes in chlorophyll a and nitrate-phosphate concentrations in a tidal Blackwater River in Niger Delta. Annual Research & Review in Biology, 21(6). https://doi.org/10.9734/arrb/2017/38456.
Pacheco, C. H. A., & Lima Neto, I. E. (2017). Effect of artificial circulation on the removal kinetics of cyanobacteria in a hypereutrophic shallow lake. Journal of Environmental Engineering, 143(12). https://doi.org/10.1061/(asce)ee.1943-7870.0001289.
Qin, G., Liu, J., Xu, S., et al. (2020). Water quality assessment and pollution source apportionment in a highly regulated river of Northeast China. Environmental Monitoring and Assessment, 192(7), 446. https://doi.org/10.1007/s10661-020-08404-0
Raulino, J. B., Silveira, C. S., & Lima Neto, I. E. (2021). Assessment of climate change impacts on hydrology and water quality of large semiarid reservoirs in Brazil. Hydrological Sciences Journal, 66(8), 1321–1336. https://doi.org/10.1080/02626667.2021.1933491
Razmkhak, H., Abrishamchi, A., & Torkian, A. (2010). Evaluation of spatial and temporal variation in water quality by pattern recognition techniques: A case study on Jajrood river (Tehran, Iran). Journal of Environmental Management, 91(4), 852–860. https://doi.org/10.1016/j.jenvman.2009.11.001
Ramírez, A. K., Salcedo, M. Á., Sánchez, A. J., et al. (2018). Relationship among physicochemical conditions, chlorophyll-a concentration, and water level in a tropical river–floodplain system. International Journal of Environmental Science and Technology, 16, 3869–3876. https://doi.org/10.1007/s13762-018-2127-7
Reder, K., Flörke, M., & Alcamo, J. (2015). Modeling historical fecal coliform loadings to large European rivers and resulting in-stream concentrations. Environmental Modelling & Software, 63, 251–263. https://doi.org/10.1016/j.envsoft.2014.10.001
Reisinger, A. J., Tank, J. L., & Dee, M. M. (2016). Regional and seasonal variation in nutrient limitation of river biofilms. Freshwater Science, 35(2). https://doi.org/10.1086/685829.
Rocha Junior, C. A. N., Costa, M. R. A., Menezes, R. F., et al. (2018). Water volume reduction increases eutrophication risk in tropical semi-arid reservoirs. Acta Limnologica Brasiliensia, 30,. https://doi.org/10.1590/s2179-975x2117
Rocha, M. J. D., & Lima Neto, I. E. (2021a). Modeling flow-related phosphorus inputs to tropical semiarid reservoirs. Journal of Environmental Management, 295, 113123. https://doi.org/10.1016/j.jenvman.2021.113123
Rocha, M. J. D., & Lima Neto, I. E. (2021b). Phosphorus mass balance and input lad estimation from the wet and dry periods in tropical semiarid reservoirs. Environmental Science and Pollution Research International. https://doi.org/10.1007/s11356-021-16251-w.10.1007/s11356-021-16251-w
Royston, P. (1982). An extension of Shapiro and Wilk’s W test for normality to large samples. Applied Statistics, 31(2), 115–124. https://doi.org/10.2307/2347973
Rubel, F., & Kottek, M. (2010). Observed and projected climate shifts 1901–2100 depicted by world maps of the Köppen-Geiger climate classification. Meteorologische Zeitschrift, 19(2), 135–141. https://doi.org/10.1127/0941-2948/2010/0430
Sabiá, R. J. (2008). Study of the pollutant emission pattern for framing of intermittent rivers: Case study of Salgado river, CE. 2008. 112 F; Thesis. Federal University of Ceará.
Salehnia, N., Alizadeh, A., Sanaeinejad, H., et al. (2017). Estimation of meteorological drought indices based on AgMERRA precipitation data and station-observed precipitation data. Journal of Arid Land, 9(6), 797–809. https://doi.org/10.1007/s40333-017-0070-y
Shadeed, S. (2012). Spatio-temporal drought analysis in arid and semi-arid regions: A case study from Palestine. Arabian Journal for Science and Engineering, 38(9). https://doi.org/10.1007/s13369-012-0504-y.
Shapiro, S. S., & Wilk, M. B. (1965). An analysis of variance test for normality (complete samples). Biometrika, 52(3/4), 591–611.
Singh, K. P., Malik, A., & Sinha, S. (2005). Water quality assessment and apportionment of pollution sources of Gomti River (India) using multivariate statistical techniques: A case study. Analytica Chimica Acta, 538(1), 355–374. https://doi.org/10.1016/j.aca.2005.02.006
Souza Filho, F. A. (2018). Water resources: Ceará 2050 together thinking or future. Fortaleza - CE, 2018, 94p.
Stagge, J. H., Tallaksen, L. M., Gudmundsson, L., et al. (2015). Candidate distributions for climatological drought indices (SPI and SPEI). International Journal of Climatology, 35(13), 4027–4040. https://doi.org/10.1002/joc.4267
Suliman, A. H. A., Awchi, T. A., Al-Mola, M., et al. (2020). Evaluation of remotely sensed precipitation sources for drought assessment in Semi-Arid Iraq. Atmospheric Research, 242, 105007. https://doi.org/10.1016/j.atmosres.2020.105007
Surendran, U., Anagha, B., Raja, P., et al. (2019). Analysis of drought from humid, semi-arid and arid regions of India using DrinC model with different drought indices. Water Resources Management, 33, 1521–1540. https://doi.org/10.1007/s11269-019-2188-5
Thiessen, A. H. (1911). Precipitation averages for large areas. Monthly Weather Review, 39, 1082–1084. https://doi.org/10.1175/1520-0493(1911)39%3c1082b:PAFLA%3e2.0.CO;2
Tripathi, M., & Singal, S. K. (2019). Use of principal component analysis for parameter selection for development of a novel Water Quality Index: A case study of river Ganga India. Ecological Indicators, 96(1), 430–436. https://doi.org/10.1016/j.ecolind.2018.09.025
Vaishali, P., & Punita, P. (2013). Assessment of seasonal variation in water quality of river Mini, at Sindrot Vadodara. International Journal of Environmental Sciences, 3, 1424. https://doi.org/10.6088/ijes.2013030500013
Van Oel, P. R., Martins, E. S. P. R., Costa, A. C., et al. (2018). Diagnosing drought using the downstreamness concept: The effect of reservoir networks on drought evolution. Hydrological Sciences Journal, 63(7), 979–990. https://doi.org/10.1080/02626667.2018.1470632
Walpole, R. E., Myers, R. H., & Myers, S. L. (1998). Probability and statistics and scientists. 6 ed. 739p.
Wang, Y., Liu, C., & Lee, J. (2015). Differentiating the spatiotemporal distribution of natural and anthropogenic processes on river water–quality variation using a self-organizing map with factor analysis. Archives of Environmental Contamination and Toxicology, 69(2), 254–263. https://doi.org/10.1007/s00244-015-0167-2
Wen, Y., Schoups, G., & Giesen, N. V. (2017). Organic pollution of rivers: Combined threats of urbanization, livestock farming and global climate change. Scientific Reports. https://doi.org/10.1038/srep43289
World Health Organization (WHO). (2011). Guidelines for drinking-water quality: Fourth edition incorporating the first addendum, WHO Library Cataloguing-in-Publication Data.
World Health Organization (WHO). (2017). Guidelines for drinking-water quality: Fourth edition incorporating the first addendum, WHO Library Cataloguing-in-Publication Data.
Wiegand, M. C., Nascimento, A. T. P., Costa, A. C., et al. (2021). Trophic state changes of semi-arid reservoirs as a function of the hydro-climatic variability. Journal of Arid Environments, 184, 104321. https://doi.org/10.1016/j.jaridenv.2020.104321
Wu, X., Duan, H., Bi, N., et al. (2016). Interannual and seasonal variation of chlorophyll-a off the Yellow River Mouth (1997–2012): Dominance of river inputs and coastal dynamics. Estuarine, Coastal and Shelf Science, 183, 402–412. https://doi.org/10.1016/j.ecss.2016.08.038
Xue, Y., Song, J., Zhang, Y., et al. (2016). Nitrate pollution and preliminary source identification of surface water in a semi-arid river basin, using isotopic and hydrochemical approaches. Water, 8(8). https://doi.org/10.3390/w8080328.
Yu, S., Xu, Z., Wu, W., et al. (2016). Effect of land use types on stream water quality under seasonal variation and topographic characteristics in the Wei River Basin, China. Ecological Indicators, 60, 202–212. https://doi.org/10.1016/j.ecolind.2015.06.029
Acknowledgements
The authors are very thankful for the data and support provided by the Environmental Agency of the State of Ceará (SEMACE), and for the helpful comments from Prof. Fernando J. A. da Silva (UFC) and Prof. Cristovão V. S. Fernandes (UFPR). The second author thanks the Brazilian National Council for Scientific and Technological Development (CNPq) for the post-doc scholarship (155814/2018-4).
Funding
This study received financial support from the Brazilian National Council for Scientific and Technological Development—CNPq (Research Grant 155814/2018–4) and Ceará State Research Foundation – FUNCAP (Research Grant PNE-0112–00042.01.00/16).
Author information
Authors and Affiliations
Corresponding author
Ethics declarations
Ethics Approval
Not applicable.
Consent to Participate
Not applicable.
Consent for Publication
Not applicable.
Competing Interests
The authors declare no competing interests.
Additional Declarations for Articles in Life Sciences Journals that Report the Results of Studies Involving Humans and/or Animals
Not applicable.
Additional information
Publisher's Note
Springer Nature remains neutral with regard to jurisdictional claims in published maps and institutional affiliations.
Rights and permissions
About this article
Cite this article
Freire, L.L., Costa, A.C. & Lima Neto, I.E. Spatio-temporal Patterns of River Water Quality in the Semiarid Northeastern Brazil. Water Air Soil Pollut 232, 452 (2021). https://doi.org/10.1007/s11270-021-05406-7
Received:
Accepted:
Published:
DOI: https://doi.org/10.1007/s11270-021-05406-7