Abstract
The study aims at evaluating the various drought indices for the humid, semi-arid and arid regions of India using conventional indices, such as rainfall anomaly index, departure analysis of rainfall and other indices such as Standard Precipitation Index (SPI) and Reconnaissance Drought Index (RDI) that were analyzed using the DrinC software. In SPI, arid region has seven drought years, whereas humid and semi-arid regions have four. In case of RDI, the humid and semi-arid regions have 11 drought years, whereas arid regions have 10 years. The difference in SPI and RDI was due to the fact that RDI considered potential evapotranspiration, and hence, correlation with plants would be better in case of RDI. Humid region showed a decreasing trend in initial value of RDI during the drought as compared to semiarid and arid regions and indicated possible climate change impact in these regions. Among all the indices, RDI was considered as an effective indicator because of implicit severity and high prediction matches with the actual drought years. SPI and RDI were found to be well correlated with respect to 3 months rainfall data and SPI values led to prediction of annual RDI. The results of our study established that this correlation could be used for developing disaster management plan well in advance to combat the drought consequences.
Similar content being viewed by others
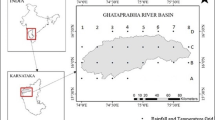
Avoid common mistakes on your manuscript.
1 Introduction
The frequent occurrence of drought due to climate change and global warming has become one of the significant global issues. It has been predicted to alter agriculture practices and land use land cover (LULC) more often than before. Intensified water cycle and extreme events, such as droughts and floods at the global scale have substantially changed the global climate and environment in the recent years (Beniston and Stephenson 2004), which increases under the influence of global warming (Dai 2011). Also, water deficit because of the temporal-spatial inconsistency between water supply and demand is expected to become harsh in case of warm climate (Huang et al. 2014; Raja et al. 2018). Recently, climate change has shown an increasing trend of drought incidences along with the recurrence rate (GoI 2016). Droughts, being a naturally occurring phenomenon, possess a tremendous effect on the physical, economic, social and political elements of the environment. Drought will be more frequent with severe issues under the following consequences: (a) increasing population at a faster rate, thus leading to increased demand of water; (b) limited or uncertain water supplies that are mainly due to the change in the climatic factors like increase in the temperature; and (c) extreme precipitation regimes (Smith and Katz 2013; Trenberth et al. 2015).
Drought occurs in all climatic regions. The concept to define drought varies with the subject of interest viz. meteorological, hydrological and agricultural droughts. A meteorological drought refers to the deficiency of rainfall, hydrological drought refers to the lack of fresh water availability or to maintain the ecosystem flows and agricultural drought that refers to the deficit in soil moisture to sustain crops and livestock, but ultimately all these lead to socio economic crisis. Drought is stood out from other natural disasters because it lacks a universal definition. Therefore, it must be defined based on the characteristics of each climatic regime and specific impact sectors. By considering all these, drought can be defined as the deficiency of water, thus severely affecting the potential crop production, runoff requirement of surface water bodies (streamflow), recharging of groundwater and reduction in environmental / ecosystem flows that causes water scarcity for human/livestock consumption and influences the entire renewable economic resources, and finally, results in a disaster for the entire mankind (Surendran et al. 2017a).
Different methods of drought assessment and several drought indices have been implemented worldwide with an objective of prediction and monitoring of droughts based on hydrological and meteorological data (Tsakiris et al. 2007, 2008, 2013; Otkin et al. 2015). Drought can be depicted in these three ways, i.e., severity of the incidence (used by calculating indices), period / duration (temporal) and spatial /areal extent.
The various climatic parameters for determining drought uses these drought indices for characterization of drought based on their severity, duration and frequency (Tsakiris et al. 2013). These drought indices can assist the researchers, policy makers, government officials and economists in the planning and decision-making process. The review of drought studies showed that no suitable single index is available universally and hence, it is necessary to consider more than one index. Some of the commonly used drought indices are as follows: PDSI (Palmer 1965), Deciles (Kinninmonth et al. 2000), standardized precipitation index (SPI) (McKee et al. 1993), vegetation condition index (Liu and Kogan 1996) and national rainfall index (Gommes and Petrassi 1994) with rainfall data input. A detailed review on these drought indices has been presented by many previous studies (Richard and Heim 2002; Tsakiris et al. 2007; Mishra and Singh 2010; Hayes et al. 2011; Sheffield et al. 2012; Stagge et al. 2017). Among these indices, SPI has been comprehensively used based on prolonged precipitation data in America (Hayes et al. 1999; Anctil et al. 2002), Asia (Min et al. 2003; Raziei et al. 2009), Africa (Ntale and Gan 2003; Mathieu and Richard 2003) and Europe (Tsakiris and Vangelis 2004; Nalbantis and Tsakiris 2009; Vasiliades et al. 2011).
The drought indices can be calculated manually or via software. In this study, the drought index calculator (DrinC) software has been used for this purpose (Tigkas et al. 2014). DrinC calculates the drought indices by considering all the factors through the flexible interface and provides a simple output. However, for getting a useful understanding of climatic states and drought pattern, the meteorological data needs to be of long-term datasets (at least 35 years). DrinC calculates the indices in the form of deciles, SPI, stream flow drought index (SDI) and reconnaissance drought index (RDI), which leads to the analysis of drought severity for the region. The software enables monitoring of drought, along with spatial distribution assessment and investigation of climatic as well as drought conditions.
The frequent occurrence of drought during the last two decades in the western United States, southeast Australia and northeast China (Sheffield and Wood 2008) has given testimony to the global warming forced extreme climate events. Most of the studies indicated an increase in the occurrence of drought. In the last 100 years, India faced worst droughts in the years 1917–18, 1965–66 and 1986–87, 2002, 2009 and 2012 and it has been more prone to drought which has enormous implications on all sectors (Anil and Indira 2007; GoI 2016). Indian Government is giving national priority to drought occurring due to erratic monsoons because such disaster has broader implications on the country’s economy. Areas, receiving very less annual rainfall, such the arid and semi-arid regions, are considered as areas prone to drought. In the absence of adequate water for irrigation, two third of the arable land is under rainfed farming in India. Another complicating factor in characterizing drought impacts in a vast country like India is that it varies on both spatial and temporal scales. With continuous droughts, once in every 5 to 7 years, the government is finding it difficult to handle the issues of the stakeholders mainly farmers and declaration of drought for crop insurance or subsidy linked issues. This issue is being observed across the world. Currently, different methods are followed by the Indian government to declare a drought, and hence, a universally accepted method, which suits to all climatic regions need to be developed in a country like India that covers diverse agro-ecological regions. However, if the farmer requires crop insurance money or subsidy for crop loss due to drought, then the district has to be declared as drought affected. Hence, in such case, the above mentioned universal drought calculation indices like RAI, departure analysis of rainfall and other indices such as SPI and RDI can be used. The primary objective of the present study is to use these drought indices for the three different climatic regions in India, such as humid, semi-arid and arid regions by DrinC model and conventional methods for characterization and analysis of drought and assess any indices is capturing the drought situation in all the three regions. These stations were selected in such a way that it represents the arid, semi-arid and humid tropics of India, because as discussed earlier with a single index it will be difficult to detect the intensity and severity of drought for the country as a whole.
2 Materials and Methods
2.1 Site Description
To evaluate drought, three different climatic regions over India (1 Northern India-arid; 2 and 3 from southern India-humid tropics, semi-arid) were selected. Details about the study sites are shown in Table 1 and Fig. 1. Mean Rainfall data observed in these regions are presented as Annexure I as supplementary data. The first region was the Kozhikode falls under humid tropical region of Kerala in Western Ghats region, which is one of the international ‘hot spots’ of biodiversity that is highly vulnerable to climate change. Kerala state is a primary producer of many of the commercial agricultural commodities in the country. Climatologically, it experiences a humid tropical climate, which is characterized by the presence of heavy rainfall, high relative humidity, abundant sunshine and high ambient temperature, and hence, researchers usually ignore the possibility of drought in such areas with high annual average rainfall of more than 3000 mm. The study area is observed to have an oppressive hot climatic condition between March and May end (35 °C), which is followed by the South-West monsoon till September end, and post-monsoon season between October and November. During December and January the temperature remains low, which is approximately 20 °C.
The second region is that of the Madurai falls under semi-arid region of Tamil Nadu and situated on the fertile and flat plain of the river Vaigai, in the northwest-southeast direction of Madurai. The hottest summer months are from March to July with the temperature reaching 42 °C at maximum and 26.3 °C as the minimum. The average annual rainfall received by the city is around 870 mm.
The third region is that of the Chandan, Jaisalmer falls under arid region of Rajasthan that lies in the Thar desert. It is the largest district of Rajasthan and third largest in the country in terms of area. The average annual rainfall received by the area is around 170 mm with summer temperatures reaching 48 °C at maximum and with an annual mean maximum temperature of 35.2 °C and a mean minimum of 17.7 °C.
2.2 Drought Indices
In the present study, drought indices based on precipitation and temperature were considered. Departure analysis of rainfall (DAR), rainfall anomaly index (RAI) and SPI needs only precipitation for the calculation, whereas RDI is the other type of drought index which requires precipitation as well as potential evapotranspiration. Calculation of drought indices, such as SPI and RDI were worked out using DrinC based on the log-normal method and gamma method both with a period of 12, 6 and 3 months.
2.2.1 Rainfall Anomaly Index (RAI)
RAI is a ranking procedure to assign degree to negative and positive precipitation anomalies, which is calculated using the Eq. (1).
Where, \( \overline{P} \) is the measured precipitation, \( \overline{E} \) is mean precipitation and is average of 10 extrema.
According to the magnitude, RAI is classified into nine classes which varies from extreme wet to extreme dry ranges.
2.2.2 Departure Analysis Rainfall (DAR)
Percentage departure in annual rainfall is calculated to understand the drought year by using the formula in Eq. (2) based on the value of departure DAR that is classified into five categories. This classification ranges from excess (<20%) rain to no rain condition (−100%)
Where, Xm is the mean annual rainfall from the annual rainfall series (Xi).
2.3 SPI
Calculation of SPI is based on long-term rainfall pattern or precipitation data. These data lead to a probability distribution, which are further transformed into a normal distribution, which results in low SPI for the respective location and desired period to be zero (McKee et al. 1993; Edwards and McKee 1997). SPI calculationis based on the number of SD that results in deviation of an observed value from the long-term mean, for a normally distributed random variable. Tigkas et al. 2014 described the comprehensive and stepwise method of determining SPI value. SPI values are positive, if greater than median precipitation and vice versa. The high negative value of SPI confirms severe drought in that particular area of study. The classification of drought conditions according to SPI has been categorized into seven classes, i.e., 2.0 or more: Extremely Wet; 1.5 to 1.99: Very Wet; 1.0 to 1.49: Moderately Wet; −0.99 to 0.99: Near Normal; −1.0 to −1.49: Moderately Dry; −1.5 to −1.99: Severely Dry −2 or less: Extremely Dry.
2.4 RDI
RDI has been used for characterization and monitoring of drought based on the water deficit data (Tsakiris and Vangelis 2005; Tsakiris et al. 2013; Vangelis et al. 2013; Tigkas et al. 2014). RDI is based on measuring the cumulative precipitation (P) and calculating the potential evapotranspiration (PET) values. The RDI’s initial value (αk) is calculated for the i-th year on a time basis of k (months) as mentioned below:
in which Pij and PETij are the rainfall / precipitation and PET of the j-th month of the i-th year and N is the total number of years of the available data. The values of αk follow both the gamma and the lognormal distributions in a wide range of locations and different time scales (Tigkas 2008; Tsakiris et al. 2007; Zarei et al. 2016; Zarei 2018) in a satisfactory manner.
By assuming that the log-normal distribution is applied, RDIst is calculated as follows (4).
in which y(i) is the ln (ak(i)), y is its arithmetic mean, and \( {\hat{\sigma}}_y \) is its standard deviation.
If the RDI values are positive, then it indicates the result is wet periods, whereas dry periods are indicated by negative RDI values when compared with the average climatic conditions of the interest area. Drought severity can be categorized as mild, moderate, severe and extreme classes, with corresponding boundary values of RDI as (−0.5 to −1.0), (−1.0 to −1.5), (−1.5 to −2.0) and (< −2.0).
3 Results and Discussion
Drought characteristics over humid, semiarid and arid regions were evaluated based on various drought indices. These indices measure how much precipitation for a given period has deviated from the normal mean or long-term average value.
3.1 Departure Analysis of Rainfall (DAR)
DAR refers to the identification of drought years which leads to finding out the extent of a deficit of annual rainfall. A drought year is the period when the total amount of annual rainfall over an area is deficient by more than 20% of its normal amount. Classification of rainfall based on departure from normal rainfall in annual scale is depicted in Table 2 and Fig. 2. Out of 34 years, in humid and semiarid regions, most of the years are coming under the normal category (i.e. 26 for humid and 21 for semiarid). While in case of the arid region the trend is different, where only 6 years are in the average normal condition balance years that are distributed among excess, deficit and small classes with 12, 15 and 5 years, respectively. Higher drought classes (No rain and scanty conditions) are nil in humid and semiarid regions for this period. Hence, the drought frequency is once in between 11 and 12 years for humid, once in nearly 5 years for semiarid and once in 1–2 years for arid region. So, the drought is predominant in arid region as expected and least for humid regions according to the departure analysis of rainfall. This is in contrast to the reality since in humid tropical regions only 3 years are falling under the deficit years (Surendran et al. 2017a, b). Hence, it is confirmed that the departure analysis of rainfall gives an indication about drought but its severity is not pronounced here and also some drought years are missed out.
3.2 Rainfall Anomaly Index (RAI)
The RAI showed a different picture when compared to DAR (Table 3 and Fig. 3a–c). In case of the humid region, 15 out of 34 years were under drought, while in the case of semiarid and arid regions it was 16 and 17 years, respectively. Considering the extremely dry category, the number of years was three in humid as well as semiarid regions but for the arid region only 1-year was under this category. This indicated a different picture and contradicting results than DAR. The difference might be due to facts that in RAI, the average of 10 extreme rainfalls was also considered in the calculation along with the annual average rainfalls. In the case of the arid region, the extreme rainfall years were less and hence only 1 year was under an extreme dry category. However, if we take the entire class of slightly dry to extremely dry, arid regions had more drought years.
3.3 Standardized Precipitation Index
Drought analysis by SPI over humid tropic, semiarid and arid regions over India was calculated and tabulated by using software DrinC (Table 4 and Fig. 4a-c). The dry years (Moderately dry, severely dry and extremely dry) were more in case of the arid region (7 years), but these were 4 years for semiarid and humid tropic regions. The critical thing to note was that extremely dry events were only present in semiarid regions and next higher class severely dry was more in the humid tropic region (3 years), which indicated a different scenario than the other two drought indices. This was in line with the findings of previous studies that showed SPI is the generally accepted and used index among the various drought monitoring indices.
3.4 RDI
The RDI is calculated based on three stages: Initial value of RDI (α12) normalized RDI (RDIn) and standardized RDI (RDIst). These may be computed for each month, seasons and hydrological year. RDI is based on the quantities of precipitation and potential evapotranspiration.
The ratio between mean annual rainfall and PET provides only a measure of humidity, or it gives a proper interpretation between different climatic regions. If the rainfall is much more than potential evapotranspiration the, then the area falls under humid tropics. For semiarid regions, it receives precipitation below potential evapotranspiration, but not hugely and if the precipitation is extremely below than potential evapotranspiration such climate is known as an arid region. This can be clearly understood from the initial RDI values for three geographical areas (Humid, Semiarid, and Arid) presented in Fig. 5. It can be observed that α12 seems to be higher for the humid area for the entire time series with an average value of 2.08 for the period of 1981–2014. The α12 value was less for semiarid and arid regions with averages 0.49 and 0.08, respectively. Humid region showed a decreasing trend in the initial value of RDI during the period 1981–2014 in comparison to semiarid and arid regions. In humid tropic regions, the humidity started to decrease towards the recent years, while for other two categories the slope showed small increment towards humidity which indicated that precipitation started to reduce the dominant effect of potential evapotranspiration. This showed the possible indication of a climatic change in the humid regions, where the temperature increases were witnessed with a reduction in rainfall during some seasons (Surendran et al. 2014, 2016, 2017a, b). However, this change was not much pronounced in the case of the other two regions. Even though there was a general perception that humid tropical region was not affected by drought or climate change events, but to our surprise, the results were different and undoubtedly this will give useful insights to the policy makers to understand the drought indices.
RDIst followed the similar procedure used for the calculation of SPI. However, it additionally calculated potential evapotranspiration apart from rainfall, and hence, gave better interpretation than SPI. Extreme drought years were observed in humid (2012) and semi-arid (2014) regions, whereas arid region, did not show any extreme drought years over this period of analysis (Table 5 and Fig. 6a to c). The results showed that humid and semi-arid region only had an extreme drought year as compared to arid regions, which was contradicting to many of the earlier studies and also a general perception that arid regions had more extremely dry years. Humid tropics and semi-arid tropical regions showed reduced incidence of rainfall along with the increase in temperature, which has been rightly observed with the RDI. This confirms the fact that RDI is a better drought indicator than all other methods. When computing all the drought classes together, the probability of getting a drought year was nearly 32% for humid tropic and semi arid regions while it was 29.4% for the arid region.
The linear trend also showed a decreasing trend for humid tropics (increasing drought intensity) and an increasing trend for arid regions (decreasing drought intensity). This implied that in the recent years, the numbers of drought years have increased. In another analysis of 110 years of data of Kozhikode (humid tropical) region showed indication of climate change in the recent years by wavelet analysis and reasons attributed for such events are largely due to anthropogenic trends (CWRDM 2017) and here in this study also with the data of three decades, from the Fig. 4a to c and 6a to c it is clear that extreme and severe classes were observed only after the year 2000, which falls in line with the reports of earlier study (Anil and Indira 2007; GoI 2016).
4 Correlation Analysis between RDI and SPI
SPI and RDI values were calculated from 1981 to 2014 for the different periods (Months of 3, 6, 9 and 12). The RDI values were correlated to the SPI values for all the three arid, semi-arid and humid regions of India and the linear regression line was produced between different categories (Fig. 7a to c), which showed RDI and SPI with significant correlation values (Table 6). This was done mainly to understand the pattern whether RDI can be predicted with precipitation data alone, which is considered to be a better indicator by which even stress on plants, can be understood.
4.1 Regression Analysis
The R2 value in the present study stated that annual SPI and RDI values were well-correlated and the linear regression was presented in Fig. 7a to c. This indicated that with precipitation (rainfall) data alone one could understand the RDI and drought years can be identified indirectly.
In the case of regression analysis, all different combinations, for instance, SPI 1st 3 months (Oct to Dec), 2nd 3 months, 3rd 3 months and 4th 3 months, 1st 6 months (Oct to Mar), 2nd 6 months (Apr to Sep) and 1st 9 months data were compared with the annual RDI values. Figure 7a to c explains better correlated values. The results showed that SPI with 1st 9 months, and annual rainfall data showed the better prediction of RDI with high R2 value in all the three regions. In humid tropical regions, SPI of 2nd 6 months (April to September) and 3rd 3 months (April to June) showed higher correlation with RDI. Hence, these rainfall data can be used for the prediction of RDI data and the possibility of drought. This can be understood from the Fig. 8a and b in which based on the regression model generated equation RDI has been predicted for selected years for SPI of 2nd 6 months and SPI of 1st 9 months and compared with the RDI observed values of that particular year. In the case of semi-arid regions, 1st 6 months (October to March) and 1st 3 months (October to December) showed a higher correlation with RDI, whereas in arid region 2nd 6 months (April to September) and 4th 3 months (July to September) showed the better prediction of RDI. The other combinations of SPI value did not show a higher correlation with RDI. The obtained results stated that RDI could be predicted with even 3 months of rainfall data for further years, RDI might be predicted before the year-end (Fig. 8a and b) and suitable policy decisions for drought can be made in advance, without waiting for the drought to happen.
SPI for different time scales were correlated with RDI (annual) for humid tropic, semiarid and arid climatic conditions over India. The correlation coefficient for different timescales (3, 6, 9 and 12 months) have been given Table 6. The maximum correlation coefficient was observed when correlation was done against 12 months data. From the values itself it was clear that the correlation coefficient increased with increase in the timescale. The results showed that for annual timescale it is more effective in predicting the RDI or in other words the effect of drought due to potential evapotranspiration will be more precise if we use an annual time scale. In case of shorter periods, usual dry and wet months were fluctuating over the periods. However, in some cases, mainly in arid regions, effect of short timescales also provided better correlation. It might be due to the significant impact of the parameter precipitation on the shorter timescale.
With the rainfall data alone, SPI can be calculated using DrinC and RDI can be found out for different time scales and drought preparedness plan can also be derived. Extensive range of scenarios may be developed for possible climate change situations and drought index of varying severity with different nomographs may also be devised using DrinC model. This can be compared with the regression equation showing a better fit, as stated by higher R2 value. These findings may be used for planning disaster management/preparedness plan to droughts and mitigate their effects on the activities in different sectors of the economy. Also, RDI can also be implemented as a climate index, suitable for identification of possible climatic trends in annual or seasonal basis. Statistically, significant trend is in support of reliable indicators of climate change that too on an annual basis (Tigkas et al. 2013). In addition to this for instance whether the humid region is tending to move towards an other climatic region may also be found out. In such case, DrinC leads to the evaluation of the possible future drought conditions along with climatic changes as well as leads to an assessment of the impact of drought on the economy; society and environment (e.g., crop production, drinking water availability, streamflow changes, etc.).
Drought analysis on a global scale has witnessed the use of SPI. The present study also confirmed that SPI can monitor both the short-term and long-term effects of the drought that is comparable among different locations due to its probabilistic characteristics. Even better prediction of RDI can also be calculated using the SPI for a small-time scale. This can help in the assessment of the potential impact of drought on agriculture since RDI considers potential evapotranspiration. Also, SPI can be computed on various time scales (Mishra and Singh 2010). Moreover, variations in SPI with different time scales can be associated with the deficiency in water in various hydrological cycle components (for e.g. soil moisture and streamflow) (Van Lanen et al. 2012; Mohammad et al. 2014).
5 Conclusions
For a country like India, identification of a suitable methodology for calculating drought index is vital for planning effective adaptation and mitigation strategies to overcome the drought situations. An attempt has been made to evaluate the applicability of several drought indices for arid, semi-arid and humid regions of India. The results showed that among several indices, SPI and RDI identified the drought situation in a more efficiently. RDI considers potential evapotranspiration, and hence, correlation with plants would be better as compared to SPI. RDI was found to be a better indicator than other methods since severity could be understood as identification of drought matches with the reality. Findings from our study could be used to understand the water availability for the whole year in advance with the help of 3 months SPI data, and thus, devising a strategy to combat droughts worldwide for efficient climate smart-agriculture, which indeed has broader implications on the global economy.
References
Anctil F, Larouche W, Viau AA (2002) Exploration of the standardized precipitation index with regional analysis. Can J Soil Sci 82(1):115–125
Anil KR, Indira H (2007) Multiple impacts of droughts and assessment of drought policy in major drought prone states in India. The Planning Commission, Government of India, New Delhi
Beniston M, Stephenson DB (2004) Extreme climatic events and their evolution under changing climatic conditions. Glob Planet Chang 44:1–9
CWRDM (2017) Annual Report of KSCSTE-CWRDM 2016–17. Published by CWRDM, Kozhikode, Kerala India
Dai AG (2011) Characteristics and trends in various forms of the Palmer drought severity index during 1900–2008. J Geophys Res 116:1–26
Edwards DC, McKee TB (1997) Characteristics of 20th century drought in the United States at multiple time scales. Climatol. Report number 97. Colorado State University, Fort Collins
GOI (2016) Manual for drought management. Department of Agriculture, Cooperation & Farmers Welfare Ministry of Agriculture & Farmers Welfare, Government of India, New Delhi
Gommes R, Petrassi F (1994) Rainfall variability and drought in sub-Saharan Africa since 1960. Agro-meteorol. Series working paper 9. Food and Agriculture Organization, Rome
Hayes MJ, Svoboda MD, Wilhite DA, Vanyarkho OV (1999) Monitoring the 1996 drought using the standardized precipitation index. Bull Am Meteorol Soc 80:429–438
Hayes M, Svoboda M, Wall N, Widhalm M (2011) The Lincoln declaration on drought indices: universal meteorological drought index recommended. Bull Am Meteorol Soc 92(4):485–488. https://doi.org/10.1175/2010BAMS3103.1
Huang SZ, Chang JX, Huang Q (2014) Spatio temporal changes and frequency analysis of drought in the Wei River Basin, China. Water Resour Manag 28(10):3095–3110
Kinninmonth WR, Voice ME, Beard GS, de Hoedt GC, Mullen CE (2000) Australian climate services for drought management. In: Wilhite DA (ed) Drought: a global assessment. Routledge, New York, pp 210–222
Liu WT, Kogan FN (1996) Monitoring regional drought using the vegetation condition index. Int J Remote Sens 17:2761–2782
Mathieu R, Richard Y (2003) Intensity and spatial extension of drought in South Africa at different time scales. Water SA 29(4):489–500
McKee TB, Doesken NJ, Kliest J (1993) The relationship of drought frequency and duration to time scales. Proceedings of the 8th Conference on Applied Climatology, Am Meteorol Soc, pp 179–184
Min SK, Kwon WT, Park EH, Choi Y (2003) Spatial and temporal comparisons of droughts over Korea with East Asia. Int J Climatol 23(2):223–233
Mishra AM, Singh VP (2010) A review of drought concepts. J Hydrol (Amst) 391:202–216
Mohammad RK, Majid V, Amin A (2014) Drought monitoring using a soil wetness deficit index (SWDI) derived from MODIS satellite data. Agric Water Manag 132:37–45
Nalbantis Ι, Tsakiris G (2009) Assessment of hydrological drought revisited. Water Resour Manag 23(5):881–897
Ntale HK, Gan T (2003) Drought indices and their application to East Africa. Int J Climatol 23:1335–1357
Otkin JA, Anderson MC, Hain C, Svoboda M (2015) Using temporal changes in drought indices to generate probabilistic drought intensification forecasts. J Hydrometeorol 16:88–105
Palmer WC (1965) Meteorological drought, research paper no. 45. US Department of Commerce Weather Bureau, Washington DC
Raja P, Singh N, Srinivas CV, Singhal M, Chauhan P, Singh M, Sinha NK (2018) Analyzing energy–water exchange dynamics in the Thar desert. Clim Dyn 50:3281–3300. https://doi.org/10.1007/s00382-017-3804-9
Raziei T, Sagfahian B, Paulo AA, Pereira LS, Bordi I (2009) Spatial patterns and temporal variability of drought in Western Iran. Water Resour Manag 23:439–455
Richard R, Heim JA (2002) Review of twentieth-century drought indices used in the United States. Am Meteorol Soc:1149–1166
Sheffield J, Wood EF (2008) Projected changes in drought occurrence under future global warming from multi-model, multi-scenario, IPCC AR4 simulations. Clim Dyn 13:79–105
Sheffield J, Wood EF, Roderick ML (2012) Little change in global drought over the past 60 years. Nature 491:435–438
Smith A, Katz R (2013) U.S. billion-dollar weather and climate disasters: data sources, trends, accuracy and biases. Nat Hazards. https://doi.org/10.1007/s11069-013-0566-5
Stagge JH, Kingston DG, Tallaksen LM, Hannah DM (2017) Observed drought indices show increasing divergence across Europe. Nat Sci Rep. https://doi.org/10.1038/s41598-017-14283-2
Surendran U, Sushanth CM, Mammen G, Joseph EJ (2014) Modeling the impacts of increase in temperature on irrigation water requirements in Palakkad district – a case study in humid tropical Kerala. J Water Clim Change 5:471–487
Surendran U, Sandeep O, Joseph EJ (2016) The impacts of magnetic treatment of irrigation water on plant, water and soil characteristics. Agric Water Manag 178:21–29
Surendran U, Sushanth CM, Mammen G, Joseph EJ (2017a) FAO-CROPWAT model-based estimation of crop water need and appraisal of water resources for sustainable water resource management: pilot study for Kollam district – humid tropical region of Kerala, India. Curr Sci 112:76–86
Surendran U, Kumar V, Ramasubramoniam S, Raja P (2017b) Development of drought indices for semi-arid region using drought indices calculator (DrinC) – a case study from Madurai District, a semi-arid region in India. Water Resour Manag 31:3593–3605. https://doi.org/10.1007/s11269-017-1687-5
Tigkas D (2008) Drought characterization and monitoring in regions of Greece. Eur Water 23(24):29–39
Tigkas D, Vangelis H, Tsakiris G (2013) The RDI as a composite climatic index. Eur Water 41:17–22
Tigkas D, Vangelis H, Tsakiris G (2014) DrinC: a software for drought analysis based on drought indices. Earth Sci Inf. https://doi.org/10.1007/s12145-014-0178-y
Trenberth KE, Fasullo JT, Shepherd TG (2015) Attribution of climate extreme events. Nat Clim Chang 5:725–730. https://doi.org/10.1038/nclimate2657
Tsakiris G, Vangelis H (2004) Towards a drought watch system based on spatial SPI. Water Resour Manag 18(1):1–12
Tsakiris G, Vangelis H (2005) Establishing a drought index incorporating evapotranspiration. Eur Water 9(10):3–11
Tsakiris G, Pangalou D, Vangelis H (2007) Regional drought assessment based on the reconnaissance drought index (RDI). Water Resour Manag 21(5):821–833
Tsakiris G, Nalbantis I, Pangalou D, Tigkas D, Vangelis H (2008) Drought meteorological monitoring network design for the reconnaissance drought index (RDI). In: Franco Lopez A (ed) Proceedings of the 1st international conference “drought management: scientific and technological innovations”. Zaragoza, Spain: Option Méditerranéennes, Series A, No. 80(12):57–62
Tsakiris G, Nalbantis I, Vangelis H, Verbeiren B, Huysmans M, Tychon B, Jacquemin I, Canters F, Vanderhaegen S, Engelen G, Poelmans L, De Becker P, Batelaan O (2013) A system-based paradigm of drought analysis for operational management. Water Resour Manag 27:5281–5297
Van Lanen HAJ, Wanders N, Tallaksen LM (2012) Hydrological drought across the world: impact of climate and physical catchment structure. Hydrol Earth Syst Sci Discuss 9:12145–12192
Vangelis H, Tigkas D, Tsakiris G (2013) The effect of PET method on reconnaissance drought index (RDI) calculation. J Arid Environ 88:130–140
Vasiliades L, Loukas A, Liberis N (2011) A water balance derived drought index for Pinios river basin, Greece. Water Resour Manag 25:1087–1101
Zarei AR (2018) Evaluation of drought condition in arid and semi- arid regions, using RDI index. Water Resour Manag 32(5):1689–1711. https://doi.org/10.1007/s11269-017-1898-9
Zarei AR, Moghimi MM, Mahmoudi MR (2016) Analysis of changes in spatial pattern of drought using RDI index in south of Iran. Water Resour Manag 30(11):3723–3743
Acknowledgments
The authors declare no conflict of interest related to the manuscript. The first two authors are thankful to the Executive Director of the Centre and The Head, Water Management (Agriculture) Division for providing the necessary support and encouragement for the smooth completion of this study. Other authors also wish to thank their respective Head of the institutions for their support during the study period. Besides the authors wish to thank the anonymous reviewers wholeheartedly for their critical comments and suggestions.
Author information
Authors and Affiliations
Corresponding author
Additional information
Publisher’s Note
Springer Nature remains neutral with regard to jurisdictional claims in published maps and institutional affiliations.
Electronic supplementary material
ESM 1
(DOCX 21 kb)
Rights and permissions
About this article
Cite this article
Surendran, U., Anagha, B., Raja, P. et al. Analysis of Drought from Humid, Semi-Arid and Arid Regions of India Using DrinC Model with Different Drought Indices. Water Resour Manage 33, 1521–1540 (2019). https://doi.org/10.1007/s11269-019-2188-5
Received:
Accepted:
Published:
Issue Date:
DOI: https://doi.org/10.1007/s11269-019-2188-5