Abstract
This study investigates the changes in the marginal cost, revenue, and profit efficiencies after a series of deals by US acquiring banks for the period from 1992 to 2007 using the nonparametric data envelopment analysis (DEA) method and the market reaction model. All the efficiency measures show increases in the first two deals but show significant decreases in the next two to three deals and substantial efficiency increases for the last two acquisitions. The efficiency losers are those that engage in just four mergers. Banks that undertake six to seven acquisitions recover their earlier efficiency losses to achieve a net 12.5% cumulative profit efficiency. The results of the market reaction model show that acquirers lose the most by the time they announce their third and fourth deals, 25.8% and 23.9% respectively, while targets gain the most when they acquire their fourth deal, 34.5%. The efficiency dynamics results show consistency with both the managerial hubris theory, where efficiency gains occur in early deals (first and second), and the learning theory where efficiency measures form a U-shape curve. These results show that the theories are complementary rather than contradictory. Moreover, frequent acquirers tend to be highly profitable, but most importantly they are externally attracted to finding targets with specific characteristics like a relatively small size, high percentage of operating assets, and a high cost efficiency.
Similar content being viewed by others
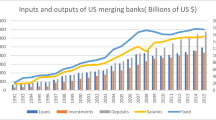
Avoid common mistakes on your manuscript.
1 Introduction
The empirical studies on corporate finance have extensively explored acquisitions as a strategic event. The majority of these studies consistently show negative returns for the acquirers and positive returns for the acquisition. (Asquith 1983; Agrawal et al. 1992; Loughran and Vijh 1997; Rau and Vermaelen 1998; Moeller et al. 2005; Al-khasawneh 2006). Acquisitions within the US banking industry have occurred in waves due, in part, to regulatory reforms and technology changes. The two primary regulatory influences behind the fifth acquisition wave (1993–2003) were the enactment of the 1994 Riegle–Neal Interstate Banking and Branching Efficiency Act and the 1999 Graham–Leach–Bliley Act. The Riegle–Neal Act removed the remaining geographic restrictions on branch banking. The Graham–Leach–Bliley Act repealed the Glass–Steagall Act of 1933 and allowed commercial banks to engage in activities such as investment banking. The result of these regulatory changes was a surge in bank acquisitions that sharply reduced the number of operating banks but led to an increase in the total number of branch banks. According to Berger et al. (2004), the period from 1995 to 2000 involved the largest number and highest value of banks acquired over any 5-year period. Wang (2003) finds that the average size of banking organizations increased by more than 35% with a total value of $1.4 trillion in the 1990s. Alexandridis et al. (2012) indicate that the brief sixth acquisition wave (2003–2007) emerged about three years after the burst of the technology bubble that put an end to the fifth acquisition wave that saw a total value of more than $1 trillion spent on deals within the US. Alexandridis et al. (2012) argue that the main characteristics of the sixth acquisition wave were easier access to liquidity and more fair-valued acquisitions that led to more rational acquisition decisions. In addition, cash deals were more common, and targets received lower premiums. The sixth acquisition wave ended with the 2007 economic crisis during which most acquisitions targeted failed banks with the assistance of the FDIC. More than at any other time, bank acquirers in the fifth and sixth waves practiced the serial acquisition of multiple targets.
Several theories in the corporate finance literature explain well the motives behind and the consequences of frequent acquisitions. The most widely studied hypotheses are the learning and the managerial hubris theories. The basic idea of the learning hypothesis is that the more acquisitions successfully undertaken, the more the acquirers learn from prior experience. Therefore, the performance of the acquirer rises with the number of accomplished acquisition deals (Aktas et al. 2011, 2013; Fuller et al. 2002; Hayward and Hambrick 1997). According to this theory, acquirers learn how to select a suitable target and the best time to acquire the target, and how to efficiently reallocate the acquired assets and then merge them with the acquirer’s assets. The learning theory has a concave function; a U-shaped relation between experience and the acquirers' stock performance during the announcement period (Hayward 2002; Haleblian and Finkelstein 1999). The managerial hubris theory, on the hand, states that managers do serial acquisitions because of increasing overconfidence, especially if the very early acquisitions increase shareholder value (Billett and Qian 2008; Malmendier and Tate 2008; Hayward and Hambrick 1997). The hubris theory holds when the serial acquirer’s cumulative abnormal return declines from deal to deal.
Previous studies of acquisitions have adopted the market-oriented methodology to study the short-term performance of frequent acquirers and have given no attention to the tracking of the operating performance in the longer term (Aktas et al. 2011; Cai et al. 2011; Ismail 2008; Fuller et al. 2002; Loderer and Martin 1990; Schipper and Thompson 1983). However, market reaction studies try to capture the short-term market effect and are hence unsuited for the strategic nature of the acquisition decision. The use of market reaction methods shows what the market learns and thinks about managers and not what managers learn or think. Accordingly, managers who favor acquisitions and become habituated to performing them should be judged with solid internal measures of performance. A market reaction method is a short-term approach that cannot test the learning theory because learning requires time.
There do not appear to be any published studies that have examined the serial acquirers in the US banking industry, an industry with more stakeholders than any other. This study is an attempt to fill this gap in the literature by tracking the marginal deal-to-deal operating performance of serial acquirers using the nonparametric data envelopment analysis (DEA). We use the DEA to calculate the profit, revenue, and cost efficiency scores to track the marginal, deal-to deal efficiency changes. Moreover, the sample period covers the period from 1992 to 2007 that was a time of extensive deregulation with two acquisition waves. The large number and frequency of acquisitions during these two waves provides an opportunity to study the behavior of serial acquirers. Furthermore, and because of the scarcity of literature that study the market reaction of the US banking sector acquisitions solely, the market model is used to analyze the stock market reaction of the acquirers, and targets using several short-term and long-term event windows while controlling for the number of merger deals.Footnote 1 Finally, we also investigate the determinants of the likelihood that acquirers will perform additional deals.
The rest of this study is organized as follows: Sect. 2 presents the method used in estimating the profit, revenue, and cost efficiency scores. Section 3 provides the sample and descriptions of the data and the proxies. Section 4 gives the results, the conclusion, and policy implications.
2 Method
2.1 The nonparametric data envelopment analysis
Charnes et al. (1978) coined the term “data envelopment analysis” (DEA). Since then, a multitude of works have applied and extended the DEA. The DEA constructs a frontier based on the sample data rather than using an assumed production function. This nonparametric approach shows how a specific decision-making unit (DMU) operates relative to other DMUs by providing a benchmark for the best-practice technology based on the DMUs in the sample. Several reasons justify the use of the DEA in this paper. First, the DEA is widely used to measure the efficiency scores in the banking industry, given the homogeneity of the input–output structure of banks (Berger and Humphrey 1992; Elyasiani and Mehdian 1992; Isik and Hassan 2003; Al-Khasawneh and Essaddam 2012; Al-Khasawneh 2013; Halkos et al. 2016; Fung and Pecha 2019; and McKee and Kagan 2018). Second, the number of observations is small during some years in my sample period, and the DEA is a suitable method for analyzing limited observations. Third, unlike with a parametric approach in which analysts have to assume a functional form and to calculate its parameters, there is no need to specify a form for the production function when using the DEA. Banks are compared to each other and the best banks make the efficient frontier. Fourth, and from the empirical point of view, most studies that have used both the stochastic frontier analysis (SFA) and the DEA have found that both approaches preserve the efficiency rankings of the DMUs (see Isik and Hassan 2002, 2003; Al-Sharkas et al. 2008).
However, the DEA requires some assumptions. In this paper, the output-oriented DEA is assumed, mainly because banks have more control over their policy for granting loans than that for accepting deposits. In addition, it adopts the intermediation approach (Sealey and Lindley 1977; Avkiran 2011; Charles et al. 2018) where financial institutions are considered as brokers who transform inputs into profitable outputs. The selected inputs and outputs and their consequent prices are the most widely used in the related literature that analyzes the efficiency of the banking industry (Das and Ghosh 2009; Belanès et al. 2015; Ray and Das 2010; Sahoo et al. 2014; Dong et al. 2016; Prior et al. 2019). These inputs, outputs, and their prices represent a very high percentages of banking activities that are well-suited to the intermediation DEA approach adopted in this paper.
Furthermore, this paper assumes the variable returns to scale (VRS) (Banker et al. 1984) in which the frontier changes over time due to technological progress, financial crises, industry concentration, and financial deregulation (Isik and Hassan 2003). Practically, the DEA scores are estimated by using one joint frontier that includes all DMUs for the whole study period. This procedure enables us to track any efficiency frontier changes over time and satisfy the number of inputs and outputs according to Cooper et al. (2006) rule of thumb that requires the number of DMUs to be greater than three times the total number of input and output variables on which the DEA scores are estimated.
2.1.1 Estimation of cost efficiency
The nonparametric cost efficiency can be estimated by summing the input prices rather than the output quantities. Consider n DMUs, where each DMU uses m inputs to produce s outputs. Then, the general form of the cost minimization problem is:
Here, pi is a vector of input prices for the jth DMU, and \(x_{*}^{i}\) is the cost minimization vector of input quantities for the jth DMU given the input prices and output levels.
The first constraint places a restriction on the input side that requires the use of inputs in a linear combination at the efficient frontier to be less than or equal to the use of the inputs by the ith bank. The second constraint shows that the observed outputs of the DMUj must be less than or equal to a linear combination of outputs, \({x}_{i}^{*}\), of the DMUs forming the efficient frontier. The third constraint assures the feasibility of the solution. The fourth constraint imposes the variable return to scale (VRS) assumption. Accordingly, the cost efficiency (CE) of each DMU can be obtained as follows:
where its value equals one for the DMUs that lie on the efficient frontier. The cost efficiency scores range from zero to one.
2.1.2 Estimation of revenue efficiency
Using the same considerations as in the previous subsection, revenue efficiency (RE) can be obtained for each DMU. The revenue-maximization problem maximizes the vector of output quantities, \({y}^{*}\), in the first step. Then, the revenue-maximization problem is calculated as follows:
Here, \({q}_{r}\) is a vector of output prices for the jth DMU, and \({y}_{r}^{*}\) is the maximization vector of output quantities for the DMUs that forms the efficient frontier. The first constraint indicates that the use of the inputs in a linear combination of efficient DMUs must be less than or equal to the use of the inputs of the jth DMU. The second constraint shows that the observed outputs of the jth DMU must be less than or equal to the linear combination of the DMUs that forms the efficient frontier. The last two constraints are defined in the previous subsection. After solving the above problem, we can obtain the revenue efficiency as follows:
where \({\sum }_{r=1}^{s}{q}_{r}{y}_{r}\) is the observed actual revenue of the DMU, and \({\sum }_{r=1}^{s}{q}_{r}{y}^{*}\) is the virtual efficiency profit that could be achieved if the DMU were situated on the efficient frontier. The value of the profit efficiency scores will always range from zero to one.
2.1.3 Estimation of profit efficiency
Summing the cost and revenue efficiencies generates the profit-efficiency concept, which seeks to minimize costs and maximize revenue simultaneously. Unlike cost and revenue efficiencies, the profit efficiency (PE) is obtained by allowing inputs and outputs to vary. The profit-maximization problem can be written as follows:
Here, the first constraint indicates that the use of the inputs in a linear combination of efficient DMUs must be less than or equal to the use of inputs of the jth DMU. The second constraint shows that the observed outputs of the jth DMU must be less than or equal to the linear combination of the DMUs that forms the efficient frontier. However, the two constraints in this problem are solved simultaneously. The third constraint is imposed to assure that the revenue maximization and cost minimization are both achieved. This constraint requires that the inputs of the jth DMU must be greater than or equal to the output of the DMUs on the efficient frontier, and it indicates that the output of the jth DMU must be less than or equal to the outputs of the DMUs on the efficient frontier. This constraint is important because it is only possible to maximize profit efficiency by minimizing costs. In this case, profit maximization is equivalent to cost minimization. The same argument is valid for the revenue efficiency. Finally, the profit efficiency can be obtained using the following equation:
where \({\sum }_{r=1}^{s}{q}_{r }{y}_{r}-{\sum }_{i=1}^{m}{p}_{i }{x}_{i}\) represents the observed profitability of DMUi. This value can be negative for the DMUs with losses. By contrast, \({\sum }_{r=1}^{s}{q}_{r }{y}_{r}^{*}-{\sum }_{i=1}^{m}{p}_{i }{x}_{i}^{*}\) represents the virtual profitability that can be achieved if the DMU is located on the efficient frontier. Accordingly, the profit efficiency values must lie in the range of (−α, 1).
2.2 Method used to evaluate the market reaction
To test the market reaction to frequent acquisition announcements, the event study method (Dodd and Warner 1983) is used to analyze the market reaction to the acquirer following the acquisition announcements of sequential deals. The market model is estimated over a 300-day period ending 51 days before the announcement of the acquisition. The CRSP value weighted index is used as a market portfolio return:
where \({R}_{it}\) represents the abnormal returns to bank stock i at time t, \({R}_{it}\) represents the actual returns to bank stock i at time t, \({a}_{i}\) is the ordinary least squares (OLS) estimate of the intercept in the estimated market model, \({b}_{i}\) is the OLS estimate of the slope coefficient for the market in the model that reflects the change in the market return relative to the return for bank i, and \({R}_{mt}\) represents the actual returns to a market portfolio of bank stocks at time t. Furthermore, the cumulative abnormal returns (CARs) are applied using five event windows for their calculation: (− 1,1) is one day before the acquisition announcement to one day after the acquisition announcement, (− 15,15), (− 30,30), (− 120,120), (− 160,160), and (− 200,200) according to the following formula:
2.3 Method to study determinants of likelihood of making more deals
To test the variable that may enhance the likelihood of acquirers engaging in more than one acquisition, we selected a set of widely used variables in the M&A literature (Doukas and Zhang 2014; Shams and Gunasekarage 2016; Beccalli and Frantz 2016; Harp et al. 2020; Al-Khasawneh et al. 2020). The selected variables consider the size of targets and acquirers, the geographic dispersion between the acquirer and the target, the method of payment used to accomplish the merger deal, the profitability of targets and acquirers, the ability of both parties to convert their deposits into performing assets, and the efficiency variables of targets and acquirers. The size is represented in two variables: size (dummy) equals one for large acquirers (if the total assets of the acquirer is above the third quartile of the sample banks) and zero otherwise. The other size variable is the target’s relative size (REL) measured by the ratio of the target’s total assets to the acquirer’s total assets. (GEO) is a dummy variable that represent the geographic dispersion between the acquirer and the target and equals one for interstate and zero for intrastate acquisitions. (STOCK) represents the percentage of stock financing and measures the payment used to finance the merger deal. The easy to observe profitability is represented by the return on assets of acquirers (AROA) and targets (TROA). The ratio of loans, leases, and investments to total deposits (LLID) measures the ability of the bank to create operating assets using the total deposits. (ALLID) and (TLLID) represent the ratio of acquirers and targets, respectively. We uniquely include the efficiency variables used in the efficiency dynamics section to test the explanatory power of these variables. (APROF) and (TPROF) represent the acquirers’ and targets’ profit efficiency, respectively. (AREV) and (TREV) represent the acquirers’ and targets’ revenue efficiency, respectively. (ACOST) and (TCOST) represent the acquirers’ and targets’ cost efficiency, respectively. Table 1 presents the summary statistics for the above-mentioned variables.
The robust heteroscedastic OLS and the Probit regression are used to investigate the determinants of the likelihood of doing more deals.Footnote 2 The dependent variable is a binary variable that equals zero if the bank made one deal during the period and equals one if it made more than one deal. This conditional probability model measures the likelihood that a bank makes more deals given that it has already made one deal. The OLS is given by:
where Y = 1 if a bank made more than one deal, and Y = 0 if it made just one deal. b is a vector of coefficients, and X’ is the transpose of a vector of the independent variables defined above. Since the predicted probability of an OLS is not bounded by zero and one, we also used the Probit model:
where ϕ is the cdf of the standard normal distribution. Moreover, the Hausman specification test is used to test for endogeneity in the OLS models.
3 Sample characteristics
The sample of US banking acquirers comes from Thomson Reuters’ Datastream. The sample includes all friendly, accomplished acquisitions in which both the target and the acquirer are commercial banks during the period from 1992 to 2007, totaling 253 banks. The ownership of the target is 100% transferred to the acquirer. However, the number of banks and the frequencies in the results section are less than that in the initial sample mainly because some banks acquired more than one target in a single year (sometimes as much as six acquisitions a year). Consequently, we consider the earliest number of acquisitions to calculate the change in efficiency. For example, if a bank has made five acquisitions so far, and it then accomplishes four more acquisitions in that year, then the ninth acquisition is treated as the sixth acquisition rather than the ninth one. Because the DEA is a benchmarking statistical technique, the sample includes not only acquiring banks, but also the universe of US banks for the whole study period to get more solid efficiency estimates.
Table 2 and Fig. 1 represent the total number of friendly acquisition deals, and the value of those deals. Figure 1 graphically illustrates the fifth and the sixth acquisition waves. The table shows that the fifth acquisition wave accounts for 72.4% of the deals, and 64% of the total value of all deals over the 1992–2007 period, whereas the sixth acquisition wave counts for 27.6% of the deals and 36% of the total value. However, the fifth acquisition wave lasts for 11 years, while the sixth acquisition wave only lasts for 5 years that makes the average annual values of the fifth and the sixth acquisition waves $US 46,455 and $US 57,368 respectively. Table 3 presents the number of deals and the percentages for each wave and the cumulative percentages and cumulative numbers of deals across acquisitions. As the table shows, the number of banks with more than nine acquisitions is low. Therefore, this study considers nine and less acquisitions, which is 81.5% of the total sample size, due to the limited number of acquirers with a higher number of acquisitions. The table indicates that the percentage of banks with one acquisition is 22.5%, and the percentage of those engaged in two or more account for 77.5%. The acquirers that made four or more acquisitions account for more than 50% of the total number of deals. The last column of Table 3 shows the cumulative percentages for each number of acquisitions. The table also shows that 253 banks conducted 605 deals during the sample period, which is an average of more than two acquisitions for each bank.
Table 4 represents the inputs, outputs, and their proxy prices for the universe of US banks (columns 1 through 5). Figure 2 illustrates the time trend of the deposits and loans as core inputs and outputs of conventional banks. The figure clearly shows the growing trends in both deposits and loans, and it also shows the improvement in the loans to deposits ratio over the sample period from 60% in 1992 to around 84% in 2007. This growing ratio indicates the improved efficiency in using resources to create more loans.
Columns 6 through 10 in Table 4 present the proxy prices of the inputs and outputs, and Fig. 3 does so graphically. The figure shows a downward trend in loan prices over time from around 16% in 1992 to 8% in 2007. The price of deposits, on the other hand, shows clear stability over time going from 3.9% in 1992 to 3.2% in 2007. Consequently, the interest margin ratio (column 11) (interest on loans–interest of deposits) decreased substantially from 12.6% in 1992 to 4.5% in 2007. This trend shows that the increase in loans created a huge supply that caused their prices to decrease.
Although we use a non-parametric approach to estimate the efficiencies, the accuracies of our estimates are robust to the presence of endogeneity (correlation between inputs and efficiencies). Many studies have examined the issue of endogeneity in a parametric approach, and they have proposed several methods to deal with it (Schlotter et al. 2011). However, few studies have examined the issue of endogeneity in the non-parametric DEA (Bifulco and Bretschneider 2001, 2003; Orme and Smith 1996; Ruggiero 2005). The most comprehensive study is from Cordero et al. (2015) who use a Monte Carlo simulation to test the accuracy of DEA estimates in the presence of endogeneity. Their findings indicate that DEA estimates are robust to low and negative correlations (rho < 0.20), less robust (but no severely biased) to medium positive correlations (rho = 0.4), and might be severely biased when there are high correlations (rho = 0.8) between inputs and efficiencies.Footnote 3 Following Cordero et al. (2015), we construct and present the inputs-efficiencies matrix in the Appendix. The matrix indicates low, negative correlation coefficients between the inputs and efficiency estimates that means our calculated efficiencies are robust to the presence of endogeneity.
4 Results and conclusions
4.1 DEA results
Tables 5, 6 and 7 present the results for the revenue, cost, and profit efficiency changes of serial acquirers. Each table is organized to show the marginal changes in deal-to-deal efficiencies along with the number of acquisitions. The end columns of each table represent the summation of deal-to-deal changes in efficiency (sum by rows, titled cumulative change), and the summation of each deal series (sum by columns, titled the cumulative change of a deal). The diagonal line represents the efficiency change of the last acquisition each acquirer accomplished.
Table 5 shows the revenue and deal-to-deal changes in efficiency for up to seven deals. The table indicates that the second and third acquisitions have positive efficiency changes. The only exceptions are the acquirers with only two acquisitions that have changes of − 0.04 in efficiency. The negative changes start to increase after the fourth acquisition and continue until the seventh deal. The last row of the table presents the cumulative change in each acquisition series (presented in Fig. 4). Figure 4 indicates positive efficiency changes for the first four deals, where the fourth deal has the only significant increase (3.6%), while the fifth and sixth deals have significantly negative efficiency changes. But, year seven indicates a significant increase in efficiency of 10%. The last column of the table represents the cumulative marginal deal-to-deal changes. The column shows that the first four deals maintain a positive increase, deal five through seven show negative marginal deal-to-deal efficiency but with a decreasing trend (from − 20.2 to − 6.6% for the fifth and seventh deal respectively).
Table 6 represents the cost efficiency results. Unlike the revenue efficiency results, the table shows more negative changes especially for the early four deals. Positive values start after the fifth deal and continue to be more common for the sixth and seventh deals.
The cumulative change for each acquisition series (last row, presented in Fig. 5) shows that the cost efficiency keeps decreasing for the first five deals and reaches a minimum by the fifth deal with a cumulative loss of − 48.3%. The sixth and seventh deals have substantial cost efficiency changes but the cumulative deal-to-deal changes remain negative. This sign means that the acquirers start learning how to be more cost efficient after the fifth deal, but they are still unable to regain their lost cost efficiency before any engagement in acquisitions. The last column of the table shows that the cumulative marginal changes in cost efficiency across all acquisition series are all negative and the maximum efficiency loss occurs after the fourth and the fifth deals, − 50.4% and − 39.0% respectively, except for the seventh deal that has a 2.5% efficiency gain.
Profit efficiency changes combine both the revenue and cost efficiencies. Those changes are presented in Table 7. The cumulative changes for each acquisition series of the table are summarized in the bottom row of the table (illustrated in Fig. 6). The results show that the profit efficiency increases after the first four deals but decreases by − 31.3% after the fifth deal. However, the most substantial positive changes occur at the seventh deal, which is 12.1%. The last column of the table indicates the same results, where the fifth deals causes a loss of 40.4% across all acquisition sequences, after which clear gains in the profit efficiency occur.
Table 8, supplemented by Fig. 7, summarizes the diagonal component of Tables 4, 5 and 6. This table shows the marginal change in the last deal after which acquirers exercise no more acquisitions, that is, the acquirer does not exercise what it learned from its previous acquisitions.
The value of this last deal’s efficiency effect is that this is the deal where the acquirers will give up or stop acquiring more targets because of either stopping the efficiency loss when the acquirer can handle no more efficiency losses or because of being satisfied with the efficiency enhancement when the acquirer achieves the targeted efficiency levels. The results show that the fifth and the sixth deals are the most destructive with regards to the revenue efficiency at − 23.8% and − 11.6%, respectively. Other deals still show a negative but insignificant trend, with the third deal as the only exception. Cost efficiency decreases after the third deal by − 10% (1 year earlier than the revenue efficiency reduction) but starts to increase to 2.5% by the seventh deal. The profit efficiency results show that there is a significant decrease after the second deal of − 3.9% (driven by the cost efficiency) and a significant decrease following the fifth and sixth deals of − 41.6% and 10.9% respectively that are driven by the revenue efficiency. Following the seventh deal, acquirers have an insignificant negative loss in profit efficiency of − 3.4%.
Summing up, the above results show that early acquisitions (one to three) have positive efficiency changes, the fourth and fifth acquisitions have the most severe efficiency losses, while the sixth and seventh deals have fast and significant efficiency gains. As mentioned earlier, this paper is not intended to test any of the existing related theories, but the results indicate that the first three acquisitions positively enhance the acquirer’s revenue efficiency and decrease the cost efficiency with lower rates than the revenue efficiency gains, but the cost efficiency loss reaches its maximum by the fourth deal. The results are consistent with the managerial hubris theory. The loss is followed by efficiency gains afterward that reflect a trend that is consistent with the managerial learning theory.
4.2 The market reaction results
In this subsection, the stock market reaction to the acquirers, and targets are represented using several short-term and long-term event windows while controlling for the deal series. However, the long-term market reaction is given more weight when representing the acquirer, while the short-term windows are given more weight for targets given that they are delisted shortly after the acquisition.
Table 9 represents the acquirers’ CARs and the t-statistics. The table indicates that the short-term windows (− 1 to 1) and (− 15, 15) have the most significant reactions. The table shows that in the short run, the more frequent the acquisitions, the greater the acquirer’s loss in stock price. The table shows that the first deal causes an average loss of 1.62% and reaches 2.99% in reaction to the sixth deal. However, there are acquisitions series with no significant market reaction (fourth, fifth, eighth, and ninth). This lack may indicate that the market gets used to the frequent acquisitions, but acquirers keep surprising it from time to time. In the long run, only the third and fourth deals have significant losses with an average of 25.8% (− 200 to 200) and 23.6% (− 160 to 160) respectively. These results show that the market starts reacting differently for serial acquirers with three to four acquisitions in the long run rather than in the short run.
Table 10 represents the targets’ CARs when acquired by acquirers with different levels of experience. Consistent with the acquisition literature, targets have significant positive returns for most event windows. However, the table shows that the t-statistics decrease as the number of acquisitions increase but are significant for the short run windows and for most of the long-run event windows. The table shows that targets with four acquisitions have the highest market return of 22.8%, 29.2%, and 34.5% for the three short-term event windows respectively. Further, the table shows that in the long run some returns lose significance, and the superiority of the fourth deal is no more the case. The sixth, seventh, and eighth deals for targets are now the ones with higher returns with a range from 42.7 to 50%.
The table shows that the targets in four, five, eight, and nine acquisitions have significant loses in their returns in the long run. This result coincides with the acquirer’s market reaction for the same number of deals. Again, this result indicates that the market does not only treat frequent acquirers in a different way but also their selected targets by reacting with less fervor. These results show that not only the number of deals affect the acquirer’s returns but also the target’s returns as well. This finding is new to the acquisition literature.
4.3 Likelihood of making more deals results
The results of the OLS and the Probit regression are presented in this subsection. The intended objective is to test the likelihood that acquirers will perform more than one acquisition by testing for the possible endogeneity in both models. Table 11 presents the results. The dependent variable is a binary variable that equals zero if an acquirer made one deal during the period and equals one if it made more than one deal. However, the results of both models are identical. Consistent with Doukas and Zhang (2014) and Shams and Gunasekarage (2016), the results show that the probability of acquiring more than one target is negatively related to the relative size, and vice versa. Practically, this result means that frequent acquirers tend to merge with smaller targets, while the banks with one merger tend to merge with larger acquirers. The table also shows that the acquirer’s ROA has a positive effect on the probability of more acquisitions and this probability increases if the target is successful in converting its deposits into performing assets as represented by TLLID. Consistent with Beccalli and Frantz (2016), this result indicates that the highly profitable acquirers’ tendency to acquire more targets is conditioned on finding targets with high performing holdings of assets. The higher significance of TLLID than AROA shows that the target’s operating assets to deposits ratio is a stricter condition for multiple acquisitions than the profitability of acquirers. However, a high TLLID ratio indicates that a shortage of liquidity makes this ratio dually informative, once it delivers a positive indication of a high ability to generate performing assets, and another by indicating increasing liquidity risk. Weak, but strong are characteristics make acquirers favor more acquisitions. Efficiency wise, the table shows that revenue efficient targets that are driven by their cost rather than their revenue efficiency increase the probability of attracting experienced acquirers. The results of this subsection indicate that relatively small targets with a low liquidity ratio and a high cost efficiency are more likely to be acquired by experienced acquirers.
To detect if the regression models contain endogeneity, we used the Hausman test for endogeneity. We run a 2SLS under the null hypothesis that the LLID is exogenous. The Hausman test compares the OLS and six estimates to check for significant differences. If significant differences are indicated, then the regressor is endogenous. If there are no significant differences, then the regressor is exogenous. We use the ratio of earning assets to total assets as the instrumental variable because it is relatively highly correlated with LLID but less correlated with the dependent variable. Since the p values of the Hausman specification test (HT) are relatively high, there is no evidence of endogeneity.
5 Conclusions
The acquisition literature presents two widely tested hypotheses: the managerial hubris and the learning hubris hypothesis. However, most of the literature has used market reaction methods to study the consequences of serial acquisitions on stocks prices.
This study attempts to fill the gap in this literature in several ways. First, the sample only comprises banks, which are screened out from any samples in the acquisition literature. Second, the sample period (1992–2007), which covers the fifth and sixth acquisition waves by US banks after historic deregulation. Third, and most importantly, this study uses the DEA to track the changes in deal-to-deal revenue, cost, and profit efficiencies, and consequently, is able to identify the acquisitions that maximize or minimize the gains or losses in efficiency.
The results show that banks that acquire fewer than three targets or more than five targets have the most efficiency-enhancing deals, while the fourth and fifth acquisitions are the most efficiency-destroying deals. The results also show that the losses in cost efficiency start earlier than the losses in revenue efficiency by one year. This finding can be explained by the fact the acquirers pay in advance for the targets, while restructuring delays revenues. Accordingly, the results support both the managerial hubris hypothesis, where managers gain more confidence because of the increasing efficiency gains following their early deals and the learning hypothesis, where after a few efficiency-destroying deals they could be wiser in selecting targets. The results, generally, show zigzag efficiency trends that indicates acquirers should not engage in no more than three deals, or less than five.
This study further analyzes the market reaction to a serial acquirer and their targets by using short- and long-term event windows. The results show that acquirers’ stock returns lose the least in the short run after the third and fourth deals but lose most in the long run for the same number of acquisitions. The market reaction to targets shows that the fourth acquisition has the highest market return for the three short-term event windows, while a higher number of acquisitions has higher returns in the long run. The inverse returns for acquirers and targets seem not only to be applicable for the cumulative abnormal returns, but also for the number of acquisitions and the event windows. This study gives evidence that the third and fourth acquisitions are the ones that destroy the acquirer’s efficiency and market returns the most.
Finally, the results of the multivariate regression models indicate that experienced acquirers are not defined by their lust to acquire, but rather they are highly selective when choosing their targets where they favor relatively small targets with a high percentage of performing assets, low-liquid targets, with high cost efficiencies.
Notes
This paper was not initially intended to test any of the existing theories on acquisitions, but the findings show a sort of consistency with the managerial hubris and learning theories that I find favorable to present.
We also ran a Logit regression, but only present the Probit results because it provides better goodness of fit, as measured by the McFadden's Pseudo R-squared.
Cordero et al (2015) assume a trans-log production function, but they emphasize that their results are similar when assuming a Cobb–Douglas function.
References
Agrawal A, Jaffe JF, Mandelker GN (1992) The post-merger performance of acquiring firms: a re-examination of an anomaly. J Finance 47:1605–1621. https://doi.org/10.1111/j.1540-6261.1992.tb04674.x
Aktas N, Bodt ED, Roll R (2011) Serial acquirer bidding: an empirical test of the learning hypothesis. J Corp Finance 17:18–32. https://doi.org/10.1016/j.jcorpfin.2010.07.002
Aktas N, Bodt ED, Roll R (2013) Learning from repetitive acquisitions: evidence from the time between deals. J Financ Econ 108:99–117. https://doi.org/10.1016/j.jfineco.2012.10.010
Alexandridis G, Mavrovitis CF, Travlos NG (2012) How have M&As changed? Evidence from the sixth merger wave. Eur J Finance 18:663–688. https://doi.org/10.1080/1351847x.2011.628401
Al-khasawneh JA (2006) Bank efficiency dynamics and market reaction around merger announcement. Dissertation, University of New Orleans
Al-Khasawneh JA (2013) Pairwise X-efficiency combinations of merging banks: analysis of the fifth merger wave. Rev Quant Finance Account 41:1–28. https://doi.org/10.1007/s11156-012-0298-8
Al-Khasawneh JA, Essaddam N (2012) Market reaction to the merger announcements of US banks: a non-parametric X-efficiency framework. Glob Finance J 23:167–183. https://doi.org/10.1016/j.gfj.2012.10.003
Al-Khasawneh JA, Benameur K, Shariff MZ, Khiyar KA (2020) Methods of payment in US banks’ acquisition: efficiency perspectives. Appl Econ 52:1487–1501. https://doi.org/10.1080/00036846.2019.1676389
Al-Sharkas AA, Hassan MK, Lawrence S (2008) The impact of mergers and acquisitions on the efficiency of the US banking industry: further evidence. J Bus Finance Account 35:50–70. https://doi.org/10.1111/j.1468-5957.2007.02059.x
Asquith P (1983) Merger bids, uncertainty, and stockholder returns. J Financ Econ 11:51–83. https://doi.org/10.1016/0304-405x(83)90005-3
Avkiran NK (2011) Association of DEA super-efficiency estimates with financial ratios: investigating the case for Chinese banks. Omega 39:323–334. https://doi.org/10.1016/j.omega.2010.08.001
Banker RD, Charnes A, Cooper WW (1984) Some models for estimating technical and scale inefficiencies in data envelopment analysis. Manag Sci 30:1078–1092. https://doi.org/10.1287/mnsc.30.9.1078
Beccalli E, Frantz P (2016) Why are some banks recapitalized and others taken over? J Int Financ Mark Inst Money 45:79–95. https://doi.org/10.1016/j.intfin.2016.07.001
Belanès A, Ftiti Z, Regaïeg R (2015) What can we learn about Islamic banks efficiency under the subprime crisis? Evidence from GCC Region. Pac-Basin Finance J 33:81–92. https://doi.org/10.1016/j.pacfin.2015.02.012
Berger AN, Humphrey DB (1992) Megamergers in banking and the use of cost efficiency as an antitrust defense. Antitrust Bull 37:541–600. https://doi.org/10.1177/0003603x9203700302
Berger AN, Buch CM, Delong G, Deyoung R (2004) Exporting financial institutions management via foreign direct investment mergers and acquisitions. J Int Money Finance 23:333–366. https://doi.org/10.1016/j.jimonfin.2004.01.002
Bifulco R, Bretschneider S (2001) Estimating school efficiency: a comparison of methods using simulated data. Econ Educ Rev 20:417–429. https://doi.org/10.1016/s0272-7757(00)00025-x
Bifulco R, Bretschneider S (2003) Response to comment on estimating school efficiency. Econ Educ Rev 22:635–638. https://doi.org/10.1016/s0272-7757(03)00044-x
Billett MT, Qian Y (2008) Are overconfident CEOs born or made? Evidence of self-attribution bias from frequent acquirers. Manag Sci 54:1037–1051. https://doi.org/10.1287/mnsc.1070.0830
Cai J, Song MH, Walkling RA (2011) Anticipation, acquisitions, and bidder returns: industry shocks and the transfer of information across rivals. Rev Financ Stud 24:2242–2285. https://doi.org/10.1093/rfs/hhr035
Charles V, Tsolas IE, Gherman T (2018) Satisficing data envelopment analysis: a Bayesian approach for peer mining in the banking sector. Ann Oper Res 269:81–102. https://doi.org/10.1007/s10479-017-2552-x
Charnes A, Cooper W, Rhodes E (1978) Measuring the efficiency of decision making units. Eur J Oper Res 2:429–444. https://doi.org/10.1016/0377-2217(78)90138-8
Cooper WW, Seiford LM, Tone K (2006) Introduction to data envelopment analysis and its uses: with DEA-solver software and references. Springer, New York
Cordero JM, Santín D, Sicilia G (2015) Testing the accuracy of DEA estimates under endogeneity through a Monte Carlo simulation. Eur J Oper Res 244:511–518. https://doi.org/10.1016/j.ejor.2015.01.015
Das A, Ghosh S (2009) Financial deregulation and profit efficiency: a nonparametric analysis of Indian banks. J Econ Bus 61:509–528. https://doi.org/10.1016/j.jeconbus.2009.07.003
Dodd P, Warner JB (1983) On corporate governance: a study of proxy contests. J Financ Econ 11:401–438. https://doi.org/10.1016/0304-405x(83)90018-1
Dong Y, Firth M, Hou W, Yang W (2016) Evaluating the performance of Chinese commercial banks: a comparative analysis of different types of banks. Eur J Oper Res 252:280–295. https://doi.org/10.1016/j.ejor.2015.12.038
Doukas JA, Zhang W (2014) Envy-motivated merger waves. Eur Financ Manag 22:63–119. https://doi.org/10.1111/eufm.12045
Elyasiani E, Mehdian S (1992) Productive efficiency performance of minority and nonminority-owned banks: a nonparametric approach. J Bank Finance 16:933–948. https://doi.org/10.1016/0378-4266(92)90033-v
Fuller K, Netter J, Stegemoller M (2002) What do returns to acquiring firms tell us? Evidence from firms that make many acquisitions. J Finance 57:1763–1793. https://doi.org/10.1111/1540-6261.00477
Fung JKH, Pecha D (2019) The efficiency of compensation contracting in China: do better CEOs get better paid? Rev Quant Finance Account 53:749–772. https://doi.org/10.1007/s11156-018-0765-y
Haleblian J, Finkelstein S (1999) The influence of organizational acquisition experience on acquisition performance: a behavioral learning perspective. Adm Sci Q 44:29–56. https://doi.org/10.2307/2667030
Halkos GE, Matousek R, Tzeremes NG (2016) Pre-evaluating technical efficiency gains from possible mergers and acquisitions: evidence from Japanese regional banks. Rev Quant Finance Account 46:47–77. https://doi.org/10.1007/s11156-014-0461-5
Harp NL, Kim KH, Oler DK (2020) A bold move or biting off more than they can chew: examining the performance of small acquirers. Rev Quant Finance Account. https://doi.org/10.1007/s11156-020-00893-x
Hayward MLA (2002) When do firms learn from their acquisition experience? Evidence from 1990 to 1995. Strateg Manag J 23:21–39. https://doi.org/10.1002/smj.207
Hayward MLA, Hambrick DC (1997) Explaining the premiums paid for large acquisitions: evidence of CEO Hubris. Adm Sci Q 42:103–127. https://doi.org/10.2307/2393810
Isik I, Hassan M (2002) Technical, scale and allocative efficiencies of Turkish banking industry. J Bank Finance 26:719–766. https://doi.org/10.1016/s0378-4266(01)00167-4
Isik I, Hassan MK (2003) Financial deregulation and total factor productivity change: an empirical study of Turkish commercial banks. J Bank Finance 27:1455–1485. https://doi.org/10.1016/s0378-4266(02)00288-1
Ismail A (2008) Which acquirers gain more, single or multiple? Recent evidence from the USA market. Glob Finance J 19:72–84. https://doi.org/10.1016/j.gfj.2008.01.002
Loderer C, Martin K (1990) Corporate acquisitions by listed firms: the experience of a comprehensive sample. Financ Manag 19:17–33. https://doi.org/10.2307/3665607
Loughran T, Vijh AM (1997) Do long-term shareholders benefit from corporate acquisitions? J Financ 52:1765–1790. https://doi.org/10.1111/j.1540-6261.1997.tb02741.x
Malmendier U, Tate G (2008) Who makes acquisitions? CEO overconfidence and the market’s reaction. J Financ Econ 89:20–43. https://doi.org/10.1016/j.jfineco.2007.07.002
Mckee G, Kagan A (2018) Community bank structure an x-efficiency approach. Rev Quant Finance Account 51:19–41. https://doi.org/10.1007/s11156-017-0662-9
Moeller SB, Schlingemann FP, Stulz RM (2005) Wealth destruction on a massive scale? A study of acquiring-firm returns in the recent merger wave. J Finance 60:757–782. https://doi.org/10.1111/j.1540-6261.2005.00745.x
Orme C, Smith P (1996) The potential for endogeneity bias in data envelopment analysis. J Oper Res Soc 47:73–83. https://doi.org/10.1057/jors.1996.7
Prior D, Tortosa-Ausina E, García-Alcober MP, Illueca M (2019) Profit efficiency and earnings quality: evidence from the Spanish banking industry. J Prod Anal 51:153–174. https://doi.org/10.1007/s11123-019-00553-w
Rau PR, Vermaelen T (1998) Glamour, value and the post-acquisition performance of acquiring firms. J Financ Econ 49:223–253. https://doi.org/10.1016/s0304-405x(98)00023-3
Ray SC, Das A (2010) Distribution of cost and profit efficiency: evidence from Indian banking. Eur J Oper Res 201:297–307. https://doi.org/10.1016/j.ejor.2009.02.030
Ruggiero J (2005) Impact assessment of input omission on DEA. Int J Inf Technol Decis Mak 04:359–368. https://doi.org/10.1142/s021962200500160x
Sahoo BK, Mehdiloozad M, Tone K (2014) Cost, revenue and profit efficiency measurement in DEA: a directional distance function approach. Eur J Oper Res 237:921–931. https://doi.org/10.1016/j.ejor.2014.02.017
Schipper K, Thompson R (1983) Evidence on the capitalized value of merger activity for acquiring firms. J Financ Econ 11:85–119. https://doi.org/10.1016/0304-405x(83)90006-5
Schlotter M, Schwerdt G, Woessmann L (2011) Econometric methods for causal evaluation of education policies and practices: a non-technical guide. Educ Econ 19:109–137. https://doi.org/10.1080/09645292.2010.511821
Sealey CW, Lindley JT (1977) Inputs, outputs, and a theory of production and cost at depository financial institutions. J Finance 32:1251–1266. https://doi.org/10.1111/j.1540-6261.1977.tb03324.x
Shams SM, Gunasekarage A (2016) Operating performance following corporate acquisitions: does the organisational form of the target matter? J Contemp Account Econ 12:1–14. https://doi.org/10.1016/j.jcae.2016.02.001
Wang JC (2003) Merger-related cost savings in the production of bank services. SSRN Electron J. https://doi.org/10.2139/ssrn.648108
Author information
Authors and Affiliations
Corresponding author
Additional information
Publisher's Note
Springer Nature remains neutral with regard to jurisdictional claims in published maps and institutional affiliations.
Appendix 1
Rights and permissions
About this article
Cite this article
Al-Khasawneh, J.A., Sanchez, B.A. Deal-to-deal marginal efficiency dynamics of serial US banking acquirers. Rev Quant Finan Acc 57, 1283–1308 (2021). https://doi.org/10.1007/s11156-021-00978-1
Accepted:
Published:
Issue Date:
DOI: https://doi.org/10.1007/s11156-021-00978-1