Abstract
With climate change and the ever-drier climate, the issue of wildfires is becoming increasingly prominent, generating growing interest in the study of wildfires. The majority of the ongoing research is focused on surface wildland fuels with particular emphasis on dead and usually dry fuel. These insights are difficult to transpose to live fuels, particularly to crown fires. The flammability properties of dead and dry forest fuels are of little significance for understanding the onset and spread of crown fires. Hence, research regarding the flammability properties of fresh forest vegetation is very sparse. The same observation applies to crown fires, despite the fact that this type of wildfires is devastating, difficult to suppress, and usually having dramatic consequences. The aim of this paper is to determine how moisture dynamics of live crown samples (terminal parts of basal branches) of two coniferous species, Abies alba and Picea abies, influence their flammability properties. Experiments were performed in an adapted mass loss calorimeter with a custom-made sample holder in order to mimic the scenario of initiation of crown fires (surface to crown fire interface). Tests were performed with heat flux values of 50, 60, and 70 kW/m2 and with different moisture levels. At all heat flux values, the results show an increasing trend for the peak heat release rate when moisture content is reduced. A. alba samples reach higher peak release rates in comparison with P. abies samples. At heat fluxes of 50 kW/m2 and 60 kW/m2, fresh A. alba samples take longer to ignite than the P. abies samples. At the heat flux of 70 kW/m2, for the set of analyzed moisture contents, the ignition time interval for the A. alba samples is shorter than for the P. abies samples. The results of the principal component analysis (PCA) show that variables such as time to ignition (TTI), peak heat release rate (PHRR), and mean heat release rate (mean HRR) best describe the ignitability of the analyzed conifer samples.
Similar content being viewed by others
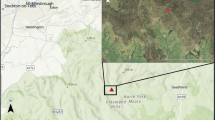
Avoid common mistakes on your manuscript.
1 Introduction
Wildland fires are a common component of many ecosystems, and every year they cause substantial losses in terms of human lives and damage to property and the environment. According to [1, 2], over the last decade, on average, more than 64,000 wildfires occurred annually. Brazil, Greece, and the USA were especially affected. Hundreds of thousands of wildfire outbreaks in Amazonia during the recent few years had a massive impact on the global ecosystem, with millions of acres of forests permanently destroyed [3]. Attica wildfires in 2018 with extreme fire behavior and hundred fatalities [4] are regarded as some of the deadliest wildland fires in Europe in recent history. During the 2018 California wildfires, more than 30,000 houses were burned (in the wildland-urban interface) with a death toll of almost 200 people [2]. It has become apparent that wildfires are occurring with increased frequency.
Numerous factors influence the frequency of occurrence of wildland fires. Some of the most cited include fuel density and fuel loading, structural characteristics of the fuels expressed as the surface area to volume ratio [5,6,7], chemical composition [8, 9], and the overall fuel arrangement. However, the dryness of wildland fuels (moisture level) could be delineated as the single most important factor [10]. With the constant increase of air temperature and elevated soil dryness over the past 20 years, the occurrence of forest fires has almost doubled, and the area affected by the fire has increased fivefold [11]. In the near future, owing to climate change, the worst is yet to come. According to the latest projections, the effects of climate change are expected to become more pronounced in the coming decades [12]. This is indicated by the Global Warming Scenario, which predicts a temperature increase from 2016 to 2035 in the range from 0.3°C to 0.7°C with a reduced precipitation rate [12]. Khabarov et al. even went one step further and “translated” the weather forecasts into a prediction of the burned area as a consequence of wildland fires [13]. They estimated the impacts of climate change under a “no adaptation” scenario for burned areas in Europe, using a Standalone Fire Model (SFM) based on a state-of-the-art large-scale forest fire modeling algorithm. They predicted a 200% increase in burned areas in Europe by 2090. For the study area included in the present paper, they provided even more pessimistic projections. Within the same scenario, they estimate that the Western Balkans and Eastern European countries will see a 150–560% increase in wildfires by 2090 [13].
Problems with wildland fires propelled international research, which has now become prolific and productive. However, most of the research interest is focused on surface wildland fires with particular emphasis on dry or wet dead fuels, neglecting the flammability properties of live vegetation [14,15,16,17,18]. This practice was probably adopted from early works on vegetation flammability where results for dead wet fuels were simply extrapolated to live fuels [19]. As opposed to surface fires, crown fires (where the fuel type is considerably different, comprising an enormous mass of live foliage, twigs, and branches) received relatively little attention in the scientific community [20]. This is probably due to the fact that this type of wildland fire is a relatively rare event. However, the consequences could be devastating [21]. Crown fires are of great intensity and are challenging for firefighters, requiring complex suppression tactics and having great potential to develop into megafires [20, 22].
According to Cruz [23], Molchanov [24] was the first researcher to scientifically determine the preconditions for the initiation of crown fires. Van Wagner [25, 26] provided the most notable contribution with the model that is now integrated into various wildland fire prediction systems, albeit with some modifications [27]. According to Wagner [25], the temperature under the base of the crown layer is the chief factor for tree crown ignition. Another one is the foliar moisture content. In the same paper, the author defined the relationship between the critical temperature for ignition, high above the line of fire, and fire intensity, and, inter alia, concluded that vertical spread from the surface fire will occur in the case when surface fire intensity exceeds a specified critical value [25]. Authors in [28] investigate the impact of water vapor, derived from the moisture content in vegetation on the combustion process via simulating an opposed diffusion flame and a laminar premixed flame with pyrolysis gases as the fuel and air as the oxidizer. The findings of this paper indicate that the water vapor from the moisture content in samples has an impact on the temperature distribution within both an opposed diffusion flame and a premixed laminar flame. Additionally, the study in [29] highlights the importance of including fuel moisture content as a parameter in flame models, especially for live wildland fuels. Work on the initiation of crown fires was further elaborated in [30]. The authors experimentally determined the relationship between the ignition temperature of branches at the bottom of the tree crown and its inherent moisture content. Alexander and Cruz, in an array of published articles [31,32,33,34,35,36,37,38,39,40], covered in depth the problem of crown fires, providing a number of useful insights. A summarized overview of recent research in the field and the models is provided in [27] and [20].
Virtually all the models for crown fire initiation from the literature incorporate fireline intensity. After the introduction by Bryam in 1959 [41], it gained considerable popularity in wildland fire research community. Fireline intensity is defined as heat released per unit time for each unit length of the firefront [42]. The main problem with fire intensity is that it does not incorporate the total energy (heat flux) that an exposed fuel object receives from fire [43]. In contrast, experiments performed under a constant heat flux do not have these shortcomings. That is why this approach is the de facto standard in the fire research community. Additionally, the measurement of fireline intensity on the field scale is inaccurate [42]. Quantification is not direct, yet it depends on other variables that are difficult or even impossible to measure, such as the mass of consumed fuel, rate of spread, etc. [42]. Finally, for crown fire models, the calculation of fireline intensity can even yield misleading results. Namely, in the Van Wanger crown fire model, fireline intensity (intensity threshold for transition from surface to crown fire) is directly proportional to canopy base height (CBH) (i.e., height from ground to the bottom of the crown) [26]. CBH is a very important parameter, which determines the probability of crowning from the approaching surface fire [27]. Values of CBH considerably differ between species and span from zero meters for mixed Douglas fir stands to five meters for Ponderosa pine (data from US northern Rocky Mountain stands [44]). Interestingly, for species with zero CBH, this model yields null for intensity threshold, which is obviously erroneous. Additionally, extensive research has been conducted on live fuels, with particular emphasis on shrub fuels (e.g., chaparral and mattoral) that contribute to the occurrence of crown fires. In [45], the logistic model was developed that serves as a valuable predictive tool for estimating fire spread in chaparral vegetation. This model incorporates various factors such as wind velocity, slope percent, moisture content, fuel loading, and species. In the context of chaparral forests, the crown base height plays a crucial role in reducing heat release rate and the rate of spread for both wind-driven and nonwind-driven fires [46]. Furthermore, research conducted in [47] reveals that wind velocity is the most influential factor in fire spread, significantly increasing fire intensity. In the presence of wind, flames become far more destructive compared to non-windy conditions [48].
Importance of surface to crown fire transition for conifers has been already addressed in literature [49], although modestly, but to our knowledge no study was focused on testing flammability parameters of terminal parts of branches. These parts of crown, especially for species with low crown base high (CBH) are the first tree elements that catch the fire from approaching surface fire flame front.
Flammability studies covering live fuels were predominately focused on either leaves/needles or twigs [50,51,52,53,54,55]. Nevertheless, there is little evidence of flammability testing of the parts or whole branches, as in the research in this paper. Exception are the studies by [56] where handy, in-field device for flammability testing of shoots was proposed. However, the methodology and presented “apparatus” don’t poses sufficient scientific rigor necessary to regard it as analytical instruments such as the cone calorimeter, mass loss calorimeter or fire propagation apparatus. This point was even raised in the manuscript itself.
A literature review of crown fire initiation indicates that the mechanisms are not fully understood, that some issues are overlooked and/or not adequately addressed, and that further work in this field is needed. This fact is what inspired the present research. Our suppositions are as follows: (a) terminal parts of basal branches of conifers, where the canopy base high is low, present the first elements of a crown that become affected by approaching surface fire line (an issue that is overlooked in the literature); (b) experiments in a laboratory setting should be performed with constant heat flux, as only this approach (as opposed to temperature measurement) reflects the real energy that exposed objects receive from fire and yields replicable and sound results; and (c) the moisture content and chemistry of the whole branches (not just leaves/needles) have a detrimental role in ignitibility.
These suppositions guided our research plan with the main objective to perform flammability testing of live crown fuel (from the most exposed basal branches) under different moisture level regimes. Experimental units (terminal parts of basal branches) were harvested from Picea abies and Abies alba stands. These species were selected because of their prevalence in Serbian coniferous forests and because of their very low CBH with basal branches literally touching the ground (Figure 1), at least on the stand edges (where the probability for surface-crown fire transition events is the highest). Flammability testing was performed using a custom-made installation comprising a mass loss calorimeter with a specially designed sample holder to minimize the instrumentation effect. Experiments were performed with a wide span of moisture levels to simulate seasonal moisture fluctuations. While the present research is focused on two conifer species, the upcoming experimental studies will include more species and expand terrestrial coverage, as well, to gather more information about the flammability characteristics of forest vegetation and to broaden knowledge about the phenomena of transition from surface to crown fires.
2 Materials and Methods
2.1 Study Area and Sampling Sites
Samples were collected from the Tara National Park in Western Serbia (between latitudes 43°43′13″ and 44°01′09″ N, and longitudes 19°13′51″ and 19°44′20″ E), whose total surface area is 24,991.82 ha at elevations between 200 m and 1591 m. The climate is humid continental with mild temperatures and rainy days in the spring and fall, warm and dry summers, and cold winters. The total annual precipitation ranges from 773 mm/m2 to 1038 mm/m2, with the maximum occurring from March to June [57]. July and August are the warmest months with average temperatures of 17.2°C, while January is the coldest with an average temperature of −2.1°C [58]. In August 2021, when the samples were collected, the average temperature was 18.4°C with total precipitation of 138.7 mm. The dominant conifers were fir (Abies alba) and spruce (Picea abies) forests.
Due to global climate change and increased mean daily temperatures, Tara National Park has been struck by a growing number of wildfires, the most devastating of which was the one in 2012, razing around 2000 ha of forest. The affected areas were at elevations from 900 m.a.s.l. to 1200 m.a.s.l. [59].
Sampling was performed on four locations, classified within the first wildfire vulnerability zone according to [59]. The GPS coordinates of the selected locations are given in Table 1. Collection sites were selected so that the trees were at least 100 m away from any road. The selected locations had minimal slopes.
2.2 Sample Preparation
The samples were taken by clipping live (green) terminal parts of basal tree branches. Individual trees were randomly selected, with a particular focus on trees located at the edge of the stand. This deliberate selection was made because the trees at the edge are the first to experience the “heat impact” from approaching surface fires. Samples from these trees were the subjects (experimental units) of our investigation. Young stands (less than 40 years old) were selected for harvesting. Branches were collected from the basal crown level from trees that were mature and of similar age. During the sampling, special attention was given to selecting similar samples with approximately the same length of 13 ± 0.5 cm (in order not to exceed the edges of the sample holder) and the diameter of primary/leading branch of 0.5 ± 0.2 cm. The number of samples collected from each location was between 35 and 40 branches. The collected samples were immediately packed in plastic bags and transported to the laboratory for combustion experiments. Samples were dried in a convective oven for different time periods so as to create a set of experimental units with a broad range of moisture contents. This method is straightforward and most frequently used in the literature [54, 60,61,62,63]. The main drawback is that a portion of the volatiles may evaporate during the drying. In order to maintain the fraction of volatiles lost from the samples as low as possible, the drying temperature was set to 60°C. All samples were measured with Radwag (AS 60/220.R2, PL) analytical weighing device before drying and then again after 4 h and 6 h spent in the oven. Additionally, a control group of five samples was created and dried up to a constant weight. This information was used to determine the mass of oven dry matter. The following formula was used to calculate the moisture content of the samples.
where FMC is the fuel moisture content, FM is the fresh mass of the sample, and DM is the dry mass of the sample. In this study, FMC pertains to the water content of the whole experimental unit—terminal parts of basal branches, comprising fresh branch wood and foliage (needles). With this protocol, experimental units with four levels of FMC were created and designated as follows: FMCfresh = 115 ± 5%; FMC4h = 70 ± 5%; FMC6h = 60 ± 5%; FMCdry = 0%.
2.3 Adapted Mass Loss Calorimeter
Flammability experiments of fuel samples can be performed on different scales: from the microscale, through the bench scale, to the full scale [64]. Obviously, full-scale or field experiments are the most useful as they provide invaluable insights about fire behavior on a real scale [64]. However, these studies are costly, both concerning the money and environmental impact, and overly intricate to conduct. On the other hand, if carefully planned, bench-scale experiments could provide useful data and help explain physical and chemical aspects at full scale [22, 65].
The experimental burnings for this study were performed in the Fire Protection Laboratory at the Faculty of Occupational Safety in Niš, University of Niš, Serbia, on a custom-made installation for material flammability testing. The installation consists of the mass loss calorimeter—MLC (Fire testing technology, UK) and a specially designed hood for extracting fire effluents during the combustion. The experimental installation is shown in Figure 2.
The hood and extraction system was designed according to ISO 5660 [66]. The flow of flue gases through the extraction system was made possible using a variable speed ventilator. The flow through the duct was set to the value of 0.035 m3/s, according to the requirements proposed by ISO 5660 [66].
In order to mimic the conditions during the real wildland fires as closely as possible, it was very important to minimize the effect of the installation/sample holder. In that respect, the standard sample holder for testing building materials was replaced with a specially designed sample holder, with porosity exceeding 80%. This design allowed a free flow of air through the sample holder, minimized the external effects (minimal mass of steel exposed to heating), and preserved the stability of the samples during the tests. The sample holder used during the experiments, together with the samples, is shown in Figure 3.
Airflow below the sample holder was measured before the experiments with a hot-wire anemometer (Testo 435, UK). The velocity below the empty sample holder was 0.1 ± 0.01 m/s.
Before the experiments, the conical heater was calibrated with a water-cooled Schmidt-Boelter flux meter. The flux meter was positioned at a distance of 25 mm from the heater, according to the value prescribed in ISO 5660 [66]. Calibration was performed for the values of 50, 60, and 70 kW/m2. The obtained heater values were in good agreement with the values provided in [67].
Thermopile was calibrated at each test heat flux using the calibration burner. Check gas with a known heating value (CH4, purity 99.95%) was used for calibration. Calibration was performed at 7 points—0.5, 0.75, 1, 2, 3, 4, and 5 kW.
Air temperature and humidity in the laboratory were measured using the temperature and relative humidity USB Data Logger (Lascar, UK). During the experiments, the temperature in the laboratory was 28 ± 2°C and the relative humidity was 57 ± 3%. These small fluctuations did not affect the obtained experimental results.
2.4 Experimental Design
We performed the full factorial experiment, consisting of all possible combinations of the factors’ levels. Factors used in experiments and specified levels of each factor are illustrated in the Table 2. Each experimental treatment (combination of factor levels effecting the response variable) was replicated three times.
From these factors and levels complete asymmetric factorial matrix was created with: one 4-level factor, one 3-level factor and one 2-level factor (41 × 31 × 21). This matrix contained 24 different experiment treatments and equal number of experimental treatment groups. Each experimental treatment group was created by three randomly selected experimental units, meaning that each experimental treatment was replicated three times. Overall of 24 × 3 = 72 experimental runs were performed. During each experimental run following response variables (flammability parameters of forest vegetation) were recorded: Time To Ignition (TTI), Heat Release Rate (HRR), Peak Heat Release Rate (PHRR), Average Effective Heat of Combustion (AEHC), Mass Loss Rate (MLR), and Total Heat Released (THR).
2.5 Statistical Analysis
First, assumption of normality of distributions was checked. For that purpose, Kolmogorov–Smirnov and Shapiro–Wilk test were used. Results indicated that several of distributions were not normal. For this reason, Kruskal–Wallis (KW) rank sum test, a nonparametric analogue of one-way ANOVA test, was used in order to test the differences in fire flammability parameters among the fuel types for different HF and FMC levels. While KW examines whether the group distributions are different, it doesn’t provide the specific clue which groups are dissimilar. For that reason, non-parametric post-hoc Dunn’s test, with Bonferroni correction was performed [68]. In order to investigate correlation between factors (HF, FMC and coniferous species) and response variables (flammability parameters: TTI, HRR, PHRR, AEHC, MLR and THR) Spearman’s correlation was used. Multivariate analysis of flammability across the species, due to strong collinearity between the flammability parameters, was performed with principal component analysis (PCA). In order to represent the results of PCA more illustratively biplots for both species were created. Finally, K-mean clustering was performed in order to confirm the results of PCA that experimental runs are grouped according to HF and MFC levels. For all tests the significance level was set at α = 0.05. Statistical analysis was performed using the R software (RStudio IDE).
3 Results
3.1 Differences in Fire Flammability Parameters Among the Fuel Types for Different HF and FMC Levels
Results of the KW test and non-parametric post-hoc Dunn’s test are reported in Tables 3 and 4. Outcomes of tests performed for treatments with fixed HF levels and varied FMC levels are reported in the last column of Tables 3 and 4 (shown in brown color). Similarly, results of tests performed for treatments with fixed FMC levels and varied HF levels are reported in the last row of each flammability parameter in Tables 3 and 4 (shown in blue color). Statistically significant results are shown in bold.
Treatments with constant HFs and varied FMC revealed significant differences in almost all flammability parameters, except for the average MLR and peak MLR for HF = 60 kW/m2 for A. alba and average MLR, PHRR for HF = 70 kW/ m2, peak MLR for HF = 60 kW/ m2 and THR for HF = 60 kW/ m2 for P. abies.
Figure 4 presents the TTI of the samples, for the three repetitions. The obtained results qualitatively indicate that moisture content in the samples is directly connected to TTI, while the heat flux value also contributes to sample TTI. Also, Figure 4 indicates that the TTI is shorter and within almost the same time range for samples with 60% moisture content and dry samples exposed to the HF at 50 kW/m2—from 22 ± 4 s to 17 ± 3 s for A. alba and from 35 ± 2 s to 28 ± 3 s for P. abies. Dry samples exhibited a more pronounced difference in TTI to the HF at 50 kW/m2, as opposed to HF at 60 and 70 kW/m2, where TTI is nearly equal—from 7 ± 1 s to 4 ± 1 s for A. alba samples, respectively, and from 11 ± 2 s to 4 ± 1 s for P. abies samples, respectively. For the fixed HF at 70 kW/m2, the TTI values for A. alba samples at different FMC levels have a narrower time range, from 4 ± 1 s to 20 ± 2 s, as opposed to the TTI for P. abies samples, with a time range from 4 ± 1 s to 31 ± 4 s.
For A. alba samples with FMC60%, the KW test showed no statistical significance of exposure to the HF at 50, 60, and 70 kW/m2 (Table 3, p = 0.063; p > 0.05). In every other case, as well as for P. abies samples, considerable statistical significance p < 0.05 was determined.
According to the results of post hoc Dunn’s test, the TTI values for dry groups of experimental units (FMCdry) were significantly lower than that for the groups with higher moisture content. This observation was valid for the whole range of applied HFs and for both coniferous species. For A. alba, the observed results for groups of experimental units with fixed levels of FMC115% and FMC70% were significantly different between all levels of HF. In relation to the fixed FMCdry level, both conifer species did not reveal significant differences for HF levels at 60 and 70 kW/m2. The same observation was noticed for the fixed FMC115% level with respect to P. abies.
Figure 5 visualizes the development of HRR for the analyzed A. alba and P. abies samples.
Combustion duration depends on the heat flux intensity and the FMC. The combustion stage for samples with the same moisture content will have different duration at varied HF intensities. For instance, for A. alba for the HF at 50 kW/m2, for all FMC levels, the duration of the combustion stage was 31, 25, 19, and 12 s, respectively. For P. abies samples, for HF at 70 kW/m2 combustion duration is 15, 11, 8, and 6 s for all FMC levels. Regarding the Mean HRR, the KW test for both conifer species reveals statistically significant results between fixed HF levels and different FMC levels. Similar results were observed across fixed FMC and different HF levels, except for FMC70% and FMC60% for A. alba, as well as FMC60% for P. abies, for which no statistical significance was observed.
The significant difference in the Mean HRR value of A. alba was found in experimental units exposed to HF at 50 kW/m2 and 60 kW/m2 between the dry groups and treatments with other ranges of applied FMC levels. In relation to the HF of 70 kW/m2, the only significant difference was observed for groups of experimental units with FMC115% and FMCdry levels. In most cases, the Mean HRR values of the dry groups of experimental units (FMCdry) for both conifer species were significantly higher related to the groups with higher moisture content. At the fixed FMC115% level, the groups of experimental units exposed to HF at 50 kW/m2 and 60 kW/m2 did not reveal significant differences from each other for both conifer species. Moreover, these groups demonstrated significantly lower values compared to the HF level of 70 kW/m2. The mean HRR values for the dry groups of experimental units (FMCdry) revealed significant differences among all levels of HF for A. alba, while the results for P. abies showed significant differences for the HF level at 60 kW/m2 compared with other HF levels.
The analysis of PHRR, shown in Figure 6, reveals a difference in PHRR in both sample groups. Therefore, A. alba samples with FMCdry for HF at 50, 60, and 70 kW/m2 have PHRR values 324 ± 3, 388 ± 7, and 276 ± 7 kW/m2, respectively, while dry P. abies samples have PHRR values 221 ± 7, 235 ± 6, and 172 ± 4 kW/m2, respectively. For the observed group of FMC levels in both sample groups, there is an increasing trend of PHRR values when exposed to all HF levels.
PHRR values for dry groups of experimental units were significantly higher than treatments with any other level of FMC, for A. alba. However, no statistically significant difference was observed for treatments with an HF value of 70 kW/m2 for P. abies, as well as for a combination of treatments involving fixed FMC levels and different levels of HF. Based on the KW test results reported in Tables 3 and 4, statistical significance was observed for all fixed HF levels in relation to different FMC levels (p < 0.05), except for P. abies samples for HF at 70 kW/m2 (p > 0.05). For P. abies samples, the results showed a narrow range of obtained PHRR values, from 159 kW/m2 to 172 kW/m2, as opposed to A. alba samples, whose range is considerably wider, from 188 kW/m2 to 276 kW/m2. In A. alba samples with FMC115% and FMC70% for different HF levels, the results were not statistically significant (p > 0.05), as opposed to the samples with FMC60% and FMCdry (p < 0.05). The significant difference observed for PHRR values was in the experimental units of A. alba for FMCdry between all levels of HF, as well for FMC60% between HF levels at 50 kW/m2 and 70 kW/m2.
Figure 7 illustrates the Average Effective Heat of Combustion (AEHC) in relation to the moisture content change in the samples and exposure to different HF. AEHC is determined as the ratio between THR during flaming combustion and the total mass of a burned sample.
As Tables 3 and 4 present, for the HF at 50 kW/m2 for FMC115 FMC70 and FMC60%, both sample groups have similar values, ranging from 6.91 to 8.17 for A. alba and from 6.16 to 6.97 for P. abies samples, whereby dry samples show a greater difference in the obtained AEHC.
For both conifer species KW test showed statistical significance results between fixed HF levels and different FMC levels (p < 0.05). However, for A. alba samples with fixed FMC70%, FMC60%, and FMCdry levels at different HF levels, the obtained AEHC results were not statistically significant (p > 0.05). The same applies to P. abies samples with fixed FMCdry.
In accordance with post hoc Dunn’s test, referring to both conifer species, the AEHC values for HF at 50 kW/m2 were not significantly different among the groups of experimental units with FMC115%, FMC70% and FMC60%. Additionally, the observed results for fixed HF levels at 60 and 70 kW/m2 regarding A. alba did not show significant differences between the group of experimental units with FMC70%, FMC60% and FMCdry. Significant differences regarding P. abies were found for fixed HF levels at 60 kW/m2 and 70 kW/m2 among groups of experimental units with FMC70% and FMCdry, as well for HF at 70 kW/m2 between FMC70% and FMC60%. For treatment with fixed FMC115% level and varied HF levels, the only significant difference was found in the experimental units exposed to HF at 60 kW/m2 compared to the other HF levels, for A. alba. Regarding P. abies, significant differences were revealed for the groups of experimental units with fixed FMC levels such as FMC115% and FMC60% exposed to HF at 60 kW/m2, as well for the FMC70% level exposed to HF at 50 kW/m2, related to other HF levels.
Observing the average MLR, results in Tables 3 and 4 don’t show consistent results between the average MLR and the moisture content in the samples, individually at different HF levels. In most cases, the MLR values for both conifer species did not show significant differences in mutual comparisons of FMC and HF levels (Tables 3, 4: p > 0.05). As presented in Tables 3 and 4, KW results for Peak MLR reviled statistically significant results between different FMC levels and fixed HF levels at 50 kW/m2 and 70 kW/m2 for A. alba, and 50 kW/m2 and 60 kW/m2 for P. abies. Additionally, a statistical significance was observed between different HF levels and fixed FMC levels, including FMC70%, FMC60%, and FMCdry in the case of A. alba, and similarly, for FMC115% and FMCdry regarding P. abies.
According to the results of post hoc Dunn’s test, the Peak MLR values of A. alba for the fixed HF at 50 kW/m2, significant differences were observed between the groups of experimental units with FMC70% and the FMC levels of FMC115% and FMC60%. Similar observations were found for the HF at 70 kW/m2, where the group of experimental units with FMC60% differ significantly from other FMC levels. According to all fixed FMC levels, regarding A. alba, significant differences observed were only for the groups of experimental units with FMC70% among all HF levels. For P. abies, the Peak MLR values for fixed HF at 60 kW/m2, the groups of experimental units with FMC60% showed no significant differences to other FMC levels. The same observation was found for fixed FMC115% level related to HF at 60 kW/m2. The dry group of experimental units (FMCdry) for both conifer species, revealed significant differences between HF at 50 kW/m2 and 60 kW/m2.
The THR values for the entire period of the experiment, for the P. abies species, show significantly higher values for the groups of experimental units with higher moisture content (FMC115% and FMC70%) than that for the groups with lower FMC levels (FMC60% and FMCdry). This observation is expressed primarily with the fixed HF at 70 kW/m2, where the group of experimental units with FMC70% differ significantly among other FMC levels, as well as for THR values during the burning period. Regarding the treatments with constant FMC levels (except for FMC115%), the THR values for the entire period of the experiment showed significant differences among the HF levels at 60 kW/m2 and 70 kW/m2, for P. abies. Also, concerning the THR values for burning period, the single significant difference observed was for the dry groups of experimental units (FMCdry) in P. abies, for HF at 60 kW/m2 in relation to other HF levels.
3.2 Correlation Analysis of Coniferous Species Between Flammability Parameters for Different HF and FMC Levels
The scatter plots of the obtained results are available in Appendix (see Figures 9and10), illustrating the interrelationship among the analyzed flammability parameters across different HF and FMC levels.
Significant correlations were found between FMC levels and various flammability parameters such as TTI, PHRR, Mean HRR and AEHC for both conifer species. Furthermore, a significant correlation was observed between the THR value for the burning period of A. alba and the THR value for the entire duration of the experiment for P. abies. Response variables that have a very strong negative correlation to FMC were PHRR (ρ = −0.95, p < 0.001), Mean HRR (ρ = −0.81, p < 0.001) and AEHC (ρ = −0.94, p < 0.001), for A. alba. Likewise, a highly significant negative correlation was observed between FMC and Mean HRR (ρ = −0.85, p < 0.001), PHRR (ρ = −0.76, p < 0.001) and AEHC (ρ = −0.61, p < 0.001), for P. abies. This indicates that as the moisture content decreases, the mentioned variables tend to increase, and vice versa. Strong positive correlation was found among FMC and TTI, for both conifer species (ρA.alba = 0.79, p < 0.001; ρP.abies = 0.77, p < 0.001). As expected, the ignition time decreases with decreasing moisture content of the samples. In terms of THR values, strong negative correlation between FMC and THR during the burning period (ρ = −0.74, p < 0.001) was noticed for A. alba, whereas a moderate positive correlation between FMC and THR for the entire duration of the experiment (ρ = 0.58, p < 0.001) was observed for P. abies. No significant correlations were found between FMC levels and MLR and Peak MLR for both conifer species (p < 0.05).
Significant correlations between HF and flammability parameters were discovered for both conifer species. Moderate negative correlations were found among HF levels and TTI for both conifer species (ρA.alba = −0.53, p < 0.001; ρP.abies = −0.59, p < 0.001). As foreseen, increasing the heat flux intensity cause decreases in the ignition time. No significant correlations between HF levels and PHRR, Mean HRR, AEHC and both THR values (burning and entire period) (p > 0.05) were detected for A. alba except for the MLR (ρ = 0.34, p < 0.05) and Peak MLR (ρ = 0.35, p < 0.05). Likewise, no significant correlations were found for P. abies between HF and Mean HRR, AEHC, Peak MLR and THR values for entire period of the experiment (p > 0.05), except for the PHRR (ρ = −0.33, p < 0.05) and MLR (ρ = −0.35, p < 0.05), in which a significant weak negative correlation was observed, as well as for the THR values for burning period, in which significant moderate positive correlation was observed (ρ = 0.49, p < 0.05).
In addition to the correlation for FMC and HF levels among flammability parameters, autocorrelation was also observed between response variables. Regarding TTI, very high negative correlations were found for PHRR (ρ = −0.82, p < 0.001) and Mean HRR (ρ = −0.81, p < 0.001), in relation to A. alba. In contrast, P. abies reports low negative correlation (ρ = −0.39, p < 0.05) and moderate negative correlation (ρ = −0.58, p < 0.001), respectively. This indicates that a higher PHRR value, as well as Mean HRR is reached with a shorter ignition time. These observations are more significant with regard to A. alba, unlike P. abies. Concerning the TTI and AEHC values, similar results were obtained for both conifer species, in which a high negative correlation was found (ρA.alba = −0.77, p < 0.001; ρP.abies = −0.63, p < 0.001). In relation to TTI and THR values for burning period, both conifer species reveal significant correlation, in which a high negative correlation was observed for A. alba (ρ = −0.74, p < 0.001), as well a moderate negative correlation was observed for P. abies (ρ = −0.51, p < 0.001). Moderate positive correlation was observed among TTI and THR values for entire period of the experiment, in relation to P. abies (ρ = 0.59, p < 0.001).
Weak but significant negative correlation was found between TTI and Peak MLR values for A. alba (ρ = −0.34, p < 0.001).
For both conifer species were observed a very high positive correlation between PHRR and Mean HRR (ρA.alba = 0.89, p < 0.001; ρP.abies = 0.88, p < 0.001), as well as AEHC values regarding the A. alba (ρ = 0.91, p < 0.001), while for P. abies, moderate positive correlation was found (ρ = 0.55, p < 0.001). Also, significant high positive correlation was detected for both conifer species between Mean HRR and AEHC values (ρA.alba = 0.73, p < 0.001; ρP.abies = 0.74, p < 0.001). Additionally, weak but significant negative correlation was found between AEHC and MLR values, as well as between MLR (ρ = −0.38, p < 0.05) and Peak MLR (ρ = −0.34, p < 0.05), regarding P. abies.
Concerning the THR values for burning period, very high positive correlation was found for Mean HRR values (ρ = 0.82, p < 0.001), and high positive correlation for PHRR (ρ = 0.77, p < 0.001) and AEHC values (ρ = 0.68, p < 0.001), for A. alba. Furthermore, a moderate positive correlation was observed between Peak MLR and THR for burning period for P. abies (ρ = 0.44, p < 0.001). With respect to THR for entire period of the experiment, weak but significant negative correlation was found for MLR values, for A. alba (ρ = −0.37, p < 0.05), and for AEHC for P. abies (ρ = −0.39, p < 0.05). Additionally, significant moderate negative correlation, between THR (for entire period of experiment) and Mean HRR (ρ = −0.42, p < 0.05) was noted for P. abies.
3.3 Results of PCA Analysis
Moisture content and heat flux are set as the explanatory variables, whose change is used to describe the changes in flammability parameters of coniferous species A. alba and P. abies. Figure 8 shows the biplot of Principal Component Analysis (PCA), which revealed that the first two dimensions represent 67.5% and 62% of the total variability in the data set. The vector loadings of the analyzed variables, given in Table 5, show approximately the same values and vector directions for both dimensions, except in the case of MLR and both THR variables.
On biplot it can be seen that there is a strong differentiation along the PC1, which is explained by Mean HRR, PHRR and AEHC in the positive sense, and an additional THR for the burning period regarding A. alba, and FMC and TTI in the negative sense, for both species. Dry samples are positioned in high PC1 values area which is characterized by higher values of Mean HRR, PHRR and AEHC and lower values of FMC and TTI. The subsamples containing some level of moisture are positioned in the negative PC1 values area and they are characterized by higher values of FMC and TTI and lower values of Mean HRR, PHRR and AEHC.
Along with PCA, K-mean cluster analysis was performed. The results of cluster analysis just confirmed what PCA has shown, i.e. there is a grouping according to moisture content. For both conifer species, one cluster contains entities with no moisture or low level (60%) of moisture. The second cluster is containing entities with higher levels of moisture.
4 Discussion
Transition from surface to crown fire depends on fuel characteristics, topology and weather conditions [69]. The most significant fuel characteristics are the type and state of tree species and especially crown profile [27]. Two analyzed species in this study (P. abies and A. alba) have specific crown profile with basal branches almost touching the ground. This feature makes them especially susceptible to catching fire from approaching surface fire front. With elevated distance between the canopy base and the ground (higher values of CBH), the chances of crowning are lower [69, 70].This has one additional, very important consequence. In the cases where CBH is high transition from surface to crown fire usually undergo through vertical pathway (ladder or bridge fuel) with convection as dominant heat transfer mechanism [27, 30, 71]. On the contrary, for species with very low CBH, where the foliage tissue is directly exposed to flame in close proximity, dominant mechanism of heat transfer is radiation. This was illustratively shown in [72,73,74]. These findings give reasonable justification to perform the experiments with instruments providing constant, predefined radiant HF levels. During the wildland fires radiant heat fluxes are in the range of 50–250 kW/m2 with the upper values for fully developed crown fires (not analyzed in this manuscript) [75,76,77]. In this study experiments were performed with mass loss calorimeter, previously calibrated to three predefined heat fluxes levels: 50, 60 and 70 kW/m2. To the best of our knowledge most of the studies in literature were performed with fluxes of 50 kW/m2 or lower [54, 60,61,62, 78,79,80,81,82], with only few exception [53].
At the beginning of each experiment, in the pre-ignition phase, after exposing the samples to heat flux, volatile compounds are separated, resulting in the formation of a flammable mixture. By applying the pilot igniter and after reaching the lower flammability limit, the samples are ignited, whereby the flame engulfs the entire surface of the samples. The structure of the sample holder allows a constant flow of oxygen into the combustion zone, which mixes with the pyrolysis gases. The delay period for volatiles to reach the ignition temperature prolongs the TTI of the samples. In addition, the water vapor generated obviously dilutes the concentrations of volatiles in the combustion zone and thus delays TTI [83]. Our experiments revealed, as was expected, that increase of HF level decreases the TTI. Observation was the same for both species. Fresh P. abies samples (FMC115%) had lower TTI when compared with A. alba samples with the same moisture level except for the HF level of 70 kW/m2. This difference was probably driven by the discrepancy in terpenes levels between the species. Terpenes are chemical compounds well known to be associated with flammability due to their relatively low flash point, low flammability limits and high gross calorific value [84,85,86]. In [87] authors showed the higher content of certain monoterpenes (especially α and β-pinene in control group) in P. abies in comparison to A. alba what directly supports our supposition. However, this trend was not observed for lower FMC levels. Possible explanation for these conflicting results may be that during the drying procedure (samples preparation phase) part of the high volatile terpenes evaporated uncontrollably and unevenly from the samples of both species. Hence, presented results, for lower FMC, should be interpreted with precaution. In real, terrain conditions there is seasonal pattern in FMC level which indirectly affects the content of terpenes in foliage. This phenomenon has been already studied and proved in Canadian and US forests (i.e., “spring dip” effect) [69].
We observed strong positive correlation between TTI and FMC for all HF levels. Similarly, McAllister et al. [77] showed connection between FMC and TTI. However, authors concluded that moisture content only explains 74–80% of the variability of the ignition time of live fuels and that the is dictated by the chemistry of fuel. This is in contrary to findings by [40] who identified only a weak effect of FMC on the ignition of canopy fuels by a surface fire in the range of 80 to 160 percent.
At the moment of ignition, the flame spreads over the sample, which increases the HRR, reaching PHRR, and then decreasing together with the sample mass during the smoldering stage. As expected, as the moisture content level in the samples decreases, it takes less time to reach PHRR. Trend of decreasing of PHRR with increased FMC values was clearly observed. The same held true for both tested tree species and for all three HF levels. This was expected as content of dry mass decreased with higher moisture contents.
FMC has a direct impact on the HRR and ignition time for both conifer species. As moisture content increases, the PHRR values have a negative correlation pattern. The results demonstrated in [88], showed that TTI for HF at 50 kW/m2 was significantly lower for live Pinaceae species, compared to our results. Contrary to the findings of [88] we find a significant difference between TTI for fresh and dry groups of experimental units, for both conifer species.
In contrast to what we anticipated both conifer species for the dry group of experimental units had PHRR values that were significantly lower for HF at 70 kW/m2 compared to other HFs. Another factor that additionally contributed to the PHRR value for HF at 70 kW/m2 was the moment of pilot igniter positioning in the gasification zone (just above the sample), whereby the pre-ignition stage and the combustion stage may occur almost simultaneously at higher heat fluxes, which necessarily influences the results. Moreover, with a shorter transition period from the combustion stage to the smoldering stage, the obtained PHRR values became lower.
A study by Madrigal et al. [89] which was performed under the similar experimental conditions, obtained PHRR results were broadly in line with our findings, for both conifer species (PHRRA.alba = 184 ± 5 kW/m2; PHRRP.abies = 120 ± 10 kW/m2). However, results obtained by Madrigal et al. [88] for PHRR for Pinaceae species were significantly higher compared to our results for HF at 50, 60 and 70 kW/m2, for both conifer species. The observed differences can be explained in the initial sample mass. Since the mass of our experimental units differs among different moisture contents, i.e., with a decrease in moisture, the mass also decreases, which can be expected with lower PHRR values. Similar observation was reached by the authors in [90]. The authors highlights the significance of considering not only the mass of foliage but also the size and structure of the samples, which are influenced by the characteristics of the species. However, regarding the effects of sample mass on the PHRR value, authors in [79] suggests considering whether the species behave as thermally thin, or thermally thick materials. Moreover, results in [91] show that the PHRR value is affected by the bulk density. The authors state that the peaks are wider with higher bulk density and vice versa, which leads that the burning process being more sustainable and longer [91]. In our case, the HRR curve has a sharp edge at the moment after the PHRR value is reached, which leads to the conclusion that our samples correspond to a smaller bulk density than the previous article. Furthermore, considering the PHRR values for dry groups of experimental units for both conifer species, the results are not in accordance with the analyzed Pinaceae species in [82, 88]. Obtained PHRR results in this manuscript are significantly lower compared to previous studies, except for dead fuel Pinus pinea, which is consistent with A. alba for HF at 60 kW/m2.
It is found that, in addition to heat flux, the remaining sample mass participating in combustion affects the PHRR value. With fresh samples, most of the water evaporates as early as the pre-ignition stage, which leads that the remaining sample mass during combustion is the smallest in fresh samples. After the pre-ignition and the combustion stages, when 80–90% of the sample mass has burned out, in the smoldering stage the remaining carbon burns out, whereby the MLR is significantly lower. The results of the numerical simulation in [92] indicate that the moisture content affects the pyrolysis process, as well as the ignition and combustion of the solid fuel in the gas phase. Observed results in present paper demonstrate that evaporation and pyrolysis occur at different times. Results demonstrated in [93], indicate that the mass of burnt fuel, bulk density and moisture content are important factors in fire spread through fuels with moisture content above 40%.
Samples exposed to heat radiation begin to decompose thermally and volatiles gradually begins to separate until they reach a specific MLR necessary for ignition. The MLR (or burning rate) increases until the PHRR is reached, which follows sample ignition, and then decreases during the smoldering phase. Authors in [91] observed that the Peak MLR values increase linearly with a decrease in bulk density. In our research, the Peak MLR values for dry group of experimental units, show statistical significance among all HF levels, and these results go beyond the values reported in [91].
One concern about our findings is that we did not take into account the remaining moisture content in the samples when evaluating the sample mass involved in combustion after the ignition. This does seem to depend on the remaining amount of moisture in samples which can be between 30 and 60% after ignition occurs. The ignition occurs at the top of the samples, where the evaporation of moisture first occurs from the surface, while the remaining amount of moisture is inside the samples [94].
Primarily mass loss occurs due to moisture release after exposing the samples to heater radiation. Regarding the average MLR values, samples with higher moisture content (FMC115% and FMC70%) are in good accordance with findings reported in [94] for groups of non-broadleaf species (Douglas fir, White fir). Authors in [94] found higher MLR values for non-broadleaf species than most broadleaf species, due to the higher surface-to-volume ratio, which can be considered as another factor that influences the MLR values.
Our results show no significant correlations between FMC levels and Average MLR values, as well as TTI and Average MLR values. Similar patterns have been identified for conifer species such as Lodgepole pine regarding the relationship between moisture content and MLR, as well as ignition time and MLR, where no statistical significance was observed. This is not the case for Douglas-fir where statistical significance was found between moisture content and MLR [95].
Both conifer species showed statistical significance for AEHC values for fixed HF values and different FMC levels. At different HF values, with a decrease in the moisture content in the samples, there is an increase in the AEHC value. Overall these findings are in accordance with findings reported by [96]. By comparing the results from [88], the AEHC values for species with low moisture content are similar to values obtained for dry groups of experimental units regarding A. alba (13.05–13.40 MJ/kg) and P. abies (10.30–13.66 MJ/kg). Additionally, our results report significantly lower AEHC values for groups with higher moisture content (5.15–6.91 MJ/kg for A. alba; 6.16–10.60 MJ/kg for P. abies) compared to live fuels in [88]. This can be explained that other factors such as local heat balance, fuel particle size and fuel porosity also contribute to AEHC results, besides moisture content [97]. Despite this, the AEHC results for P. abies with higher FMC level (FMC115%) were similar to AEHC values reported in [89]. Additionally, the difference in our results between samples with higher moisture content (fresh samples) and dry samples ties well with findings for the group of forest fuels reported in [79], as well for dry groups of experimental units with results reported in [81]. Regarding the THR values, both conifer species were not consistent with findings in [82]. Our results report significantly lower values for all experimental treatments compared to studies in [81, 82]. The probable explanation for these findings is that there was not enough remaining sample mass after ignition to contribute to the combustion process. The significant factors for achieving higher THR values, as well as PHRR, include the initial sample mass and moisture content [98].
5 Conclusion
In the present study flammability characteristics of terminal parts of two common coniferous species (A. alba and P. abies) were experimentally determined, depending on changes in moisture content at incident heat fluxes of 50, 60, and 70 kW/m2. The research was conducted using an adapted mass loss calorimeter configuration, whereby the examined samples were placed on a porous rectangular sample holder, representing the real, in field conditions. Additionally, as opposed to the conventional approach where just the foliage alone is tested, in our study flammability properties of the whole terminal parts of basal branches were determined yielding more reliable results.
For both species, the dependence between the level of moisture content and the value of the incident heat flux was determined. As the heat flux increases and the moisture content of the fuel decreases, the ignition time decreases. Due to the porous surface of the sample holder, the flow of fresh air in the combustion zone is increased, cooling and dissolving evolved volatiles, which consequently affects the delay in the ignition of the samples (higher TTI). Obtained values are higher than reported in the literature for flammability testing of coniferous foliage alone, indicating that experiments with conventional, non or slightly perforated, and aerated, sample holders could provide misleading results. The dependence of the level of moisture content and heat flux on mass loss rate (MLR) has not been detected. Upon the samples ignition, and reaching the PHRR, the rate of mass loss increased. A trend of increasing PHRR values with decreasing moisture content and heat flux was also observed. Depending on the moisture content and incident heat flux, the duration of the combustion phase varied. We didn’t notice an increase in PHRR values with elevated incident heat fluxes > 60 kW/m2 which can be attributed to the small mass of samples. AEHC indeed correlated with FMC and incident heat flux, however, this correlation weakened with elevated heat fluxes. With high incident heat flux (70 kW/m2) the process of thermal decomposition and extraction of volatiles happened very quickly (almost instantaneously). In some cases, devolatilization was so quick that flaming combustion even didn’t occur. This certainly affected obtained values of flammability parameters: TTI, HRR, PHRR, AEHC and THR.
Variables such as chemical composition, fats, resins, waxes, and terpenes also significantly affect the flammability of forest vegetation. However, these factors were not determined in this research. To overcome these limitations, future work will incorporate chemical analysis into the research plan. This additional analysis, building upon previous studies [99,100,101,102], will investigate the synergistic effects of fuel moisture content (FMC) and the chemical composition of forest fuels. The objective will be to determine the individual contributions of these factors to flammability and gain a deeper understanding of their combined influence. Additionally, further work will be also directed towards the improvement of the present flammability testing installation to even more realistically simulate real wildland fires events at bench scale.
References
Welcome to the Nation’s Logistical Support Center | National Interagency Fire Center. https://www.nifc.gov/ (Accessed 14 May 2022)
Largest wildfires of the decade—BatchGeo Blog. https://blog.batchgeo.com/largest-wildfires/ (Accessed 14 May 2022)
Yao JQ, Zhai HR, Tang XM, Gao XM, Yang XD (2020) Amazon fire monitoring and analysis based on multi-source remote sensing data. IOP Conf Ser. https://doi.org/10.1088/1755-1315/474/4/042025
Lagouvardos K, Kotroni V, Giannaros TM, Dafis S (2019) Meteorological conditions conducive to the rapid spread of the deadly wildfire in Eastern Attica, Greece. Bull Am Meteorol Soc 100(11):2137–2145. https://doi.org/10.1175/BAMS-D-18-0231.1
Ganteaume A, Jappiot M, Lampin C, Guijarro M, Hernando C (2013) Flammability of some ornamental species in wildland-urban interfaces in southeastern France: laboratory assessment at particle level. Environ Manage 52(2):467–480. https://doi.org/10.1007/s00267-013-0067-z
Simpson KJ et al (2016) Determinants of flammability in savanna grass species. J Ecol 104(1):138–148. https://doi.org/10.1111/1365-2745.12503
White RH, Zipperer WC (2010) Testing and classification of individual plants for fire behaviour: plant selection for the wildlandurban interface. Int J Wildl Fire 19(2):213–227. https://doi.org/10.1071/WF07128
Ormeño E et al (2009) The relationship between terpenes and flammability of leaf litter. For Ecol Manage 257(2):471–482. https://doi.org/10.1016/j.foreco.2008.09.019
Della Rocca G, Madrigal J, Marchi E, Michelozzi M, Moya B, Danti R (2017) Relevance of terpenoids on flammability of Mediterranean species: an experimental approach at a low radiant heat flux. IForest 10(5):766–775. https://doi.org/10.3832/ifor2327-010
Morgan Varner J, Kane JM, Kreye JK, Engber E (2015) The flammability of forest and woodland litter: a synthesis. Curr For Rep 1(2):91–99. https://doi.org/10.1007/s40725-015-0012-x
Badmaev N, Bazarov A (2020) Correlation analysis of terrestrial and satellite meteodata in the territory of the Republic of Buryatia (Eastern Siberia, Russian Federation) with forest fire statistics. Agric For Meteorol 297:108245. https://doi.org/10.1016/j.agrformet.2020.108245
Pachauri R, Meyer L (2014) Climate change 2014—synthesis report. Accessed 01 Mar 2021. http://ipcc.ch/pdf/assessment-report/ar5/syr/SYR_AR5_FINAL_full_wcover.pdf
Khabarov N et al (2016) Forest fires and adaptation options in Europe. Reg Environ Chang 16(1):21–30. https://doi.org/10.1007/s10113-014-0621-0
Matthews S (2014) Dead fuel moisture research: 1991–2012. Int J Wildl Fire 23(1):78–92. https://doi.org/10.1071/WF13005
Fernandes PM, Botelho HS, Rego FC, Loureiro C (2009) Empirical modelling of surface fire behaviour in maritime pine stands. Int J Wildl Fire 18(6):698. https://doi.org/10.1071/WF08023
Dimitrakopoulos AP, Papaioannou KK (2001) Flammability assessment of Mediterranean forest fuels. Fire Technol 37(2):143–152. https://doi.org/10.1023/A:1011641601076
Pyne Stephen J, Andrews PL, Laven RD (1996) Introduction to wildland fire. Wiley, New York
Verbesselt J, Fleck S, Coppin P (2002) Estimation of fuel moisture content towards fire risk assessment: a review. In: Viegas DX (ed) Forest fire research & wildland fire safety. Millpress, Rotterdam, pp 1–11
Finney MA, Cohen JD, McAllister SS, Jolly WM (2013) On the need for a theory of wildland fire spread. Int J Wildl Fire 22(1):25–36. https://doi.org/10.1071/WF11117
Xanthopoulos G, Athanasiou M (2020) Crown fire. Encyclopedia of wildfires and wildland-urban interface (WUI) fires. Springer, Cham, pp 1–15
Moreira F, Arianoutsou M, Corona P, las Heras JD (2012) Post-fire management and restoration of Southern European forests, vol 24. Springer, Dordrecht
Rodríguez y Silva F et al (2017) Assessment of crown fire initiation and spread models in Mediterranean conifer forests by using data from field and laboratory experiments. For Syst 26(2):1–14. https://doi.org/10.5424/fs/2017262-10652
Cruz MG (1999) Modeling the initiation and spread of crown fires. Dissertation, University of Montana
Molchanov V (1957) Conditions for the spread of crown fire in pine forest. Lesn Khozydystvo 10:50–63
Van Wagner CE (1977) Conditions for the start and spread of crown fire. Can J For Res 7(1):23–34. https://doi.org/10.1139/X77-004
Van Wagner CE (1993) Prediction of crown fire behavior in two stands of jack pine. Can J For Res 23(3):442–449. https://doi.org/10.1139/x93-062
Keane Robert E (2015) Wildland fuel fundamentals and applications. Springer, Cham
Ferguson SC, Dahale A, Shotorban B, Mahalingam S, Weise DR (2013) The role of moisture on combustion of pyrolysis gases in wildland fires. Combust Sci Technol 185(3):435–453. https://doi.org/10.1080/00102202.2012.726666
Weise DR et al (2018) Fire behavior in chaparral–evaluating flame models with laboratory data. Combust Flame 191:500–512. https://doi.org/10.1016/j.combustflame.2018.02.012
Xanthopoulos G, Wakimoto RH (1993) A time to ignition–temperature–moisture relationship for branches of three western conifers. Can J For Res 23(2):253–258. https://doi.org/10.1139/X93-034
Cruz MG, Alexander ME, Wakimoto RH (2003) Assessing canopy fuel stratum characteristics in crown fire prone fuel types of western North America. Int J Wildl Fire 12(1):39–50. https://doi.org/10.1071/WF02024
Cruz MG, Alexander ME, Wakimoto RH (2004) Modeling the likelihood of crown fire occurrence in conifer forest stands. For Sci 50(5):640–658
Cruz MG, Alexander ME, Wakimoto RH (2003) Assessing the probability of crown fire initiation based on fire danger indices. For Chron 79(5):976–983. https://doi.org/10.5558/tfc79976-5
Cruz MG, Alexander ME, Wakimoto RH (2005) Development and testing of models for predicting crown fire rate of spread in conifer forest stands. Can J For Res 35(7):1626–1639. https://doi.org/10.1139/x05-085
Alexander ME, Cruz MG (2012) Interdependencies between flame length and fireline intensity in predicting crown fire initiation and crown scorch height. Int J Wildl Fire 21(2):95–113. https://doi.org/10.1071/WF11001
Cruz MG, Alexander ME (2010) Assessing crown fire potential in coniferous forests of western North America: a critique of current approaches and recent simulation studies. Int J Wildl Fire 19(4):377–398. https://doi.org/10.1071/WF08132
Cruz MG, Alexander ME, Wakimoto RH (2002) Predicting crown fire behavior to support forest fire management decision-making. For Fire Res Wildl Fire Saf [Online]. http://nofc.cfs.nrcan.gc.ca/bookstore_pdfs/21122.pdf
Cruz M, Alexander M, Wakimoto RH (2003) Definition of a fire behavior model evaluation protocol: a case study application to crown fire behavior models. Fire Fuel Treat Ecol Restor [Online]. http://www.cnr.uidaho.edu/for433/secure/lessons/lesson02/cruzetal_2003_RMRS_proc.pdf
Alexander ME, Cruz MG (2011) Crown fire dynamics in conifer forests. In: Synthesis of knowledge of extreme fire behavior: volume I for fire managers. Department of Agriculture, Forest Service, Pacific Northwest Research Station, Portland, pp 107–144
Cruz MG, Butler BW, Alexander ME, Viegas DX (2006) Development and evaluation of a semi-physical crown fire initiation model. V International conference on forest fire research. https://doi.org/10.1016/j.foreco.2006.08.132
Byram GM (1959) Combustion of forest fuels. Forest fire: control and use. McGraw-Hill Book Company Inc, New York, pp 61–89
Morandini F, Perez-Ramirez Y, Tihay V, Santoni PA, Barboni T (2013) Radiant, convective and heat release characterization of vegetation fire. Int J Therm Sci 70:83–91. https://doi.org/10.1016/j.ijthermalsci.2013.03.011
Sullivan EA, Mcdonald AG (2014) Mathematical model and sensor development for measuring energy transfer from wildland fires. Int J Wildl Fire 23(7):995–1004. https://doi.org/10.1071/WF14016
Scott JH, Reinhardt ED (2005) Stereo photo guide for estimating canopy fuel characteristics in conifer stands. USDA For Serv 145:1–51
Weise DR, Zhou X, Sun L, Mahalingam S (2005) Fire spread in chaparral—‘Go or no-go?’ Int J Wildl Fire 14(1):99–106. https://doi.org/10.1071/WF04049
Cobian-Iñiguez J, Aminfar AH, Saha S, Awayan K, Weise DR, Princevac M (2022) The transition and spread of a chaparral crown fire: insights from laboratory scale wind tunnel experiments. J Combust 2022:1–13. https://doi.org/10.1155/2022/5630594
Weise DR, Koo E, Zhou X, Mahalingam S, Morandini F, Balbi JH (2016) Fire spread in chaparral—a comparison of laboratory data and model predictions in burning live fuels. Int J Wildl Fire 25(9):980–994. https://doi.org/10.1071/WF15177
Sanpakit C, Omodan S, Weise D, Princevac M (2015) Laboratory fire behavior measurements of chaparral crown fire. Univ Calif Riverside Undergrad Res J 9:123–129
Weise DR, Cobian-Iñiguez J, Princevac M (2018) Surface to crown transition. Encyclopedia of wildfires and wildland-urban interface (WUI) fires. Springer, Cham, pp 988–992
Leonelli L, Barboni T, Santoni PA, Quilichini Y, Coppalle A (2017) Characterization of aerosols emissions from the combustion of dead shrub twigs and leaves using a cone calorimeter. Fire Saf J 91:800–810. https://doi.org/10.1016/j.firesaf.2017.03.048
Ganteaume A, Jappiot M, Curt T, Lampin C, Borgniet L (2014) Flammability of litter sampled according to two different methods: comparison of results in laboratory experiments. Int J Wildl Fire 23(8):1061–1075. https://doi.org/10.1071/WF13045
Alam MA et al (2020) Shoot flammability is decoupled from leaf flammability, but controlled by leaf functional traits. J Ecol 108(2):641–653. https://doi.org/10.1111/1365-2745.13289
Simeoni A et al (2012) Flammability studies for wildland and wildland-urban interface fires applied to pine needles and solid polymers. Fire Saf J 54:203–217. https://doi.org/10.1016/j.firesaf.2012.08.005
Fateh T, Richard F, Batiot B, Rogaume T, Luche J, Zaida J (2016) Characterization of the burning behavior and gaseous emissions of pine needles in a cone calorimeter—FTIR apparatus. Fire Saf J 82:91–100. https://doi.org/10.1016/j.firesaf.2016.03.008
Romero B, Ganteaume A (2021) Effect of fire frequency on the flammability of two Mediterranean pines: link with needle terpene content. Plants 10(10):1–23. https://doi.org/10.3390/plants10102164
Jaureguiberry P, Bertone G, Díaz S (2011) Device for the standard measurement of shoot flammability in the field. Austral Ecol 36(7):821–829. https://doi.org/10.1111/j.1442-9993.2010.02222.x
Gigović L, Pourghasemi HR, Drobnjak S, Bai S (2019) Testing a new ensemble model based on SVM and random forest in forest fire susceptibility assessment and its mapping in Serbia’s Tara National Park. Forests 10(5):408. https://doi.org/10.3390/F10050408
Marković T, Radanović D, Nastasijević B, Antić-Mladenović S, Vasić V, Matković A (2019) Yield, quality and safety of yellow gentian roots produced under dry-farming conditions in various single basal fertilization and planting density models. Ind Crops Prod 132:236–244. https://doi.org/10.1016/j.indcrop.2019.02.027
Lode project—Loss data enhancement for DDR & CCA management. https://www.lodeproject.polimi.it/ (Accessed 08 Dec 2020)
Bartoli P, Simeoni A, Biteau H, Torero JL, Santoni PA (2011) Determination of the main parameters influencing forest fuel combustion dynamics. Fire Saf J 46(1–2):27–33. https://doi.org/10.1016/j.firesaf.2010.05.002
Schemel CF, Simeoni A, Biteau H, Rivera JD, Torero JL (2008) A calorimetric study of wildland fuels. Exp Therm Fluid Sci 32(7):1381–1389. https://doi.org/10.1016/j.expthermflusci.2007.11.011
Jervis FX, Rein G (2016) Experimental study on the burning behaviour of Pinus halepensis needles using small-scale fire calorimetry of live, aged and dead samples. Fire Mater 40(3):385–395. https://doi.org/10.1002/fam.2293
Santoni PA, Romagnoli E, Chiaramonti N, Barboni T (2015) Scale effects on the heat release rate, smoke production rate, and species yields for a vegetation bed. J Fire Sci 33(4):290–319. https://doi.org/10.1177/0734904115591176
Simeoni A (2013) Experimental understanding of wildland fires. Fire phenomena and the earth system. Wiley, Hoboken, pp 35–52
Finney MA, Cohen ABJD, Mcallister SS, Matt W (2013) On the need for a theory of wildland fire spread. Int J Wildland Fire. https://doi.org/10.1071/WF11117
ISO (2015) 5660-1. Reaction-to-fire tests – Heat release, smoke production and mass loss rate – Part 1: Heat release rate (cone calorimeter method) and smoke production rate (dynamic measurement)
Babrauskas V (1984) Development of the cone calorimeter—a bench-scale heat release rate apparatus based on oxygen consumption. Fire Mater 8(2):81–95. https://doi.org/10.1002/fam.810080206
McDaniel JK, Alexander HD, Siegert CM, Lashley MA (2020) Shifting tree species composition of upland oak forests alters leaf litter structure, moisture, and flammability. For Ecol Manage 482:118860. https://doi.org/10.1016/j.foreco.2020.118860
Werth PA et al (2011) Synthesis of knowledge of extreme fire behavior: volume I for fire managers. Department of Agriculture, Forest Service, Pacific Northwest Research Station, Portland
Molchanov V (1957) Conditions for the spread of crown fires in pine forests. Lesn Khoziaistvo 10(8):50–53
Keane RE (2019) Canopy fuel. In: Manzello SL (ed) Encyclopedia of wildfires and wildland-urban interface (WUI) fires. Springer, Cham, pp 1–12
Safdari MS, Amini E, Weise DR, Fletcher TH (2019) Comparison of pyrolysis of live wildland fuels heated by radiation vs convection. Fuel 268:117342. https://doi.org/10.1016/j.fuel.2020.117342
Safdari MS (2018) Characterization of pyrolysis products from fast pyrolysis of live and dead vegetation native. Dissertation, Brigham Young University
Safdari MS et al (2018) Characterization of pyrolysis products from fast pyrolysis of live and dead vegetation native to the Southern United States. Fuel 229:151–166. https://doi.org/10.1016/j.fuel.2018.04.166
Butler BW et al (2004) Measurements of radiant emissive power and temperatures in crown fires. Can J For Res 34(8):1577–1587. https://doi.org/10.1139/X04-060
Silvani X, Morandini F, Muzy J-F (2009) Wildfire spread experiments: fluctuations in thermal measurements. Int Commun Heat Mass Transf 36(9):887–892. https://doi.org/10.1016/j.icheatmasstransfer.2009.06.008
McAllister S, Grenfell I, Hadlow A, Jolly WM, Finney M, Cohen J (2012) Piloted ignition of live forest fuels. Fire Saf J 51:133–142. https://doi.org/10.1016/j.firesaf.2012.04.001
Lamorlette A, El Houssami M, Morvan D (2018) An improved non-equilibrium model for the ignition of living fuel. Int J Wildl Fire 27(1):29–41. https://doi.org/10.1071/WF17020
Weise DR, White RH, Beall FC, Etlinger M (2005) Use of the cone calorimeter to detect seasonal differences in selected combustion characteristics of ornamental vegetation. Int J Wildl Fire 14(3):321–338. https://doi.org/10.1071/WF04035
Blank RR, White RH, Ziska LH (2006) Combustion properties of Bromus tectorum L.: influence of ecotype and growth under four CO2 concentrations. Int J Wildl Fire 15(2):227–236. https://doi.org/10.1071/WF05055
Dibble AC, White RH, Lebow PK (2007) Combustion characteristics of north-eastern USA vegetation tested in the cone calorimeter: invasive versus non-invasive plants. Int J Wildl Fire 16(4):426–443. https://doi.org/10.1071/WF05103
Madrigal J, Hernando C, Guijarro M, DÃez C, Marino E, De Castro AJ (2009) Evaluation of forest fuel flammability and combustion properties with an adapted mass loss calorimeter device. J Fire Sci 27(4):323–342. https://doi.org/10.1177/0734904109102030
Ramadhan ML, Zarate S, Carrascal J, Osorio AF, Hidalgo JP (2021) Effect of fuel bed size and moisture on the flammability of Eucalyptus saligna leaves in cone calorimeter testing. Fire Saf J 120:103016. https://doi.org/10.1016/j.firesaf.2020.103016
Romero B, Fernandez C, Lecareux C, Ormeño E, Ganteaume A (2019) How terpene content affects fuel flammability of wildland–urban interface vegetation. Int J Wildl Fire 28(8):614–627. https://doi.org/10.1071/WF18210
Ormeño E et al (2009) The relationship between terpenes and flammability of leaf litter. For Ecol Manage 257(2):471–482. https://doi.org/10.1016/j.foreco.2008.09.019
Pausas JG, Alessio GA, Moreira B, Segarra-Moragues JG (2016) Secondary compounds enhance flammability in a Mediterranean plant. Oecologia 180(1):103–110. https://doi.org/10.1007/s00442-015-3454-8
Zamponi L, Michelozzi M, Capretti P (2007) Terpene response of Picea abies and Abies alba to infection with Heterobasidion s.l. For Pathol 37(4):243–250. https://doi.org/10.1111/j.1439-0329.2007.00493.x
Madrigal J, Guijarro M, Hernando C, Díez C, Marino E (2011) Effective heat of combustion for flaming combustion of mediterranean forest fuels. Fire Technol 47(2):461–474. https://doi.org/10.1007/s10694-010-0165-x
Madrigal J, Hernando C, Guijarro M (2013) A new bench-scale methodology for evaluating the flammability of live forest fuels. J Fire Sci 31(2):131–142. https://doi.org/10.1177/0734904112458244
Etlinger MG, Beall FC (2004) Development of a laboratory protocol for fire performance of landscape plants. Int J Wildl Fire 13(4):479–488. https://doi.org/10.1071/WF04039
El Houssami M et al (2016) Experimental and numerical studies characterizing the burning dynamics of wildland fuels. Combust Flame 168:113–126. https://doi.org/10.1016/j.combustflame.2016.04.004
Yashwanth BL, Shotorban B, Mahalingam S, Lautenberger CW, Weise DR (2016) A numerical investigation of the influence of radiation and moisture content on pyrolysis and ignition of a leaf-like fuel element. Combust Flame 163:301–316. https://doi.org/10.1016/j.combustflame.2015.10.006
Dahale A, Ferguson S, Shotorban B, Mahalingam S (2013) Effects of distribution of bulk density and moisture content on shrub fires. Int J Wildl Fire 22(5):625–641. https://doi.org/10.1071/WF12040
Pickett BM, Isackson C, Wunder R, Fletcher TH, Butler BW, Weise DR (2010) Experimental measurements during combustion of moist individual foliage samples. Int J Wildl Fire 19(2):153–162. https://doi.org/10.1071/WF07121
McAllister S, Weise DR (2017) Effects of season on ignition of live wildland fuels using the forced ignition and flame spread test apparatus. Combust Sci Technol 189(2):231–247. https://doi.org/10.1080/00102202.2016.1206086
Babrauskas V (2006) Effective heat of combustion for flaming combustion of conifers. Can J For Res 36(3):659–663. https://doi.org/10.1139/x05-253
Possell M, Bell TL (2013) The influence of fuel moisture content on the combustion of Eucalyptus foliage. Int J Wildl Fire 22(3):343–352. https://doi.org/10.1071/WF12077
White RH, Weise DR, Frommer S (1996) Preliminary evaluation of the flammability of native and ornamental plants with the cone calorimeter. In: Proceedings of the international conference on fire safety, pp. 256–265
Matt FJ, Dietenberger MA, Weise DR (2020) Summative and ultimate analysis of live leaves from Southern U.S. forest plants for use in fire modeling. Energy Fuels 34(4):4703–4720. https://doi.org/10.1021/acs.energyfuels.9b04107
Jolly WM et al (2012) Relationships between moisture, chemistry, and ignition of Pinus contorta needles during the early stages of mountain pine beetle attack. For Ecol Manage 269:52–59. https://doi.org/10.1016/j.foreco.2011.12.022
Boardman CR, Dietenberger MA, Weise DR (2021) Specific heat capacity of wildland foliar fuels to 434°C. Fuel 292:120396. https://doi.org/10.1016/j.fuel.2021.120396
McAllister S, Weise DR (2017) Effects of season on ignition of live wildland fuels using the forced ignition and flame spread test apparatus. Combust Sci Technol 189(2):231–247. https://doi.org/10.1080/00102202.2016.1206086
Acknowledgements
The manuscript is part of the collaboration within the EU COST Action CA18135 FIRElinks.
Funding
This work was financially supported by the Ministry of Education, Science and Technological Development of the Republic of Serbia, [Grant No. 451-03-68/2022-14/ 200148].
Author information
Authors and Affiliations
Corresponding author
Ethics declarations
Conflict of interests
The authors declare no conflict of interest.
Additional information
Publisher's Note
Springer Nature remains neutral with regard to jurisdictional claims in published maps and institutional affiliations.
Appendix
Appendix
1.1 Scatter Plots for Illustrating the Interrelationship Between Flammability Parameters Across Different Heat Flux and FMC Levels
Rights and permissions
Springer Nature or its licensor (e.g. a society or other partner) holds exclusive rights to this article under a publishing agreement with the author(s) or other rightsholder(s); author self-archiving of the accepted manuscript version of this article is solely governed by the terms of such publishing agreement and applicable law.
About this article
Cite this article
Mišić, N., Protić, M., Cerdà, A. et al. Transition from Surface to Crown Fires: Effects of Moisture Content. Fire Technol 60, 669–700 (2024). https://doi.org/10.1007/s10694-023-01525-1
Received:
Accepted:
Published:
Issue Date:
DOI: https://doi.org/10.1007/s10694-023-01525-1