Abstract
To investigate the diagnostic efficiency of Raman spectroscopy for the diagnosis of breast cancer, we searched PubMed, Web of Science, Cochrane Library, and Embase for articles published from the database establishment to May 20, 2022. Pooled sensitivity, specificity, diagnostic odds ratio, and area under the receiver pooled operating characteristic curve were derived for the included studies as outcome measures. The methodological quality was assessed according to the questionnaires and criteria suggested by the Diagnostic Accuracy Research Quality Assessment-2 tool. Sixteen studies were included in this meta-analysis. The pooled sensitivity and specificity of Raman spectroscopy for breast cancer diagnosis were 0.97 (95% CI, [0.92–0.99]) and 0.96 (95% CI, [0.91–0.98]). The diagnostic odds ratio was 720.89 (95% CI, [135.73–3828.88]) and the area under the curve of summary receiver operating characteristic curves was 0.99 (95% CI, [0.98–1]). Subgroup analysis revealed that all subgroup types in our analysis, including different races, sample types, diagnostic algorithms, number of spectra, instrument types, and laser wavelengths, turned out to have a sensitivity and specificity greater than 0.9. Significant heterogeneity was found between studies. Deeks’ funnel plot demonstrated that publication bias was acceptable. This meta-analysis suggests that Raman spectroscopy may be an effective and accurate tool to differentiate breast cancer from normal breast tissue, which will help us diagnose and treat breast cancer.
Similar content being viewed by others
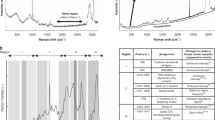
Explore related subjects
Discover the latest articles, news and stories from top researchers in related subjects.Avoid common mistakes on your manuscript.
Introduction
According to statistics, the number of new breast cancer (BC) patients in 2020 has reached 2.26 million, which indicates that BC has become the highest incidence of cancer [1]. Mammography and ultrasonography are currently the main methods of screening for BC. Although highly effective in reducing BC mortality due to early diagnosis and treatment, mammography still suffers from some limitations, such as lower sensitivity in screening patients with dense breasts [2], frequent false positive alarm [3], and ionizing radiation risk. Correspondingly, ultrasonography is safe for pregnant women because it does not use ionizing radiation and performs better than mammography for dense breasts. However, it is not sensitive to microcalcifications and the detection rate is dependent on the experience level of the examining physician. Therefore, the development of a rapid, non-invasive, convenient, and sensitive method for BC detection is imminently required in clinical practice.
As a powerful spectroscopic technique, Raman spectroscopy (RS) has been widely used in biological detection [4, 5] based on the principle of providing molecular information about chemical bonds related to molecular vibration and rotation, which helps detect tiny changes in the structure of biomolecules composed of lipids, proteins, and nucleic acids during the development of cancer [6,7,8]. In terms of preliminary findings, RS showed high accuracy in diagnosing cancers such as bladder [9], kidney [10], and skin cancer [11], with both sensitivity and specificity exceeding 0.9. In addition, RS has relatively loose requirements on the morphology of the tested samples, such as solid tissue strips, pathological tissue sections, and even liquid samples that can be used for Raman analysis [12, 13].
Surface-enhanced Raman spectroscopy (SERS) is a technique for enhancing the Raman signal of biomolecules by using precious metal nanoparticles (gold, silver, and copper) as substrates. The electromagnetic mechanism (EM) and chemical effect (CT) of metal nanoparticles are central to the principle [14]. The EM is caused primarily by the coupling of the incident electromagnetic field in the gap between nanoscale metallic materials and can be explained as a contribution to the enhancement of the incident field and the enhancement of the Stokes scattering of molecular re-emission at a specific point on the surface where the sample is located. The CT mechanism is primarily derived from nanoscale metal particle structure and charge transfer between molecules, which is accomplished through the formation of new analyte-metal surface complexes. These two processes take place simultaneously and work together to increase the Raman spectrum intensity [15]. SERS is currently widely used in the detection of biological fluids such as serum [16], tears [17], and urine [18] because it can generate significantly enhanced Raman signals, even at the single-molecule level in some cases [19].
Given the benefits of RS, some studies on its use in the diagnosis of breast cancer have been reported [20, 21]. However, the outcomes of different studies differ. This can be explained by the fact that different studies have different sample sizes and diagnostic algorithms. As a result, we conducted this meta-analysis to determine the clinical value of RS to comprehensively analyze the exact effect of RS in diagnosing BC.
Methods
Literature search
We searched the relevant articles published in PubMed, Embase, Web of Science, and Cochrane Library from the establishment of the database to May 20, 2022. The search terms were as follows: (1) [(“Breast cancer” OR “Breast tumor” OR “Breast neoplasm” OR “Mammary cancer”) AND (“Raman spectroscopy” OR “RS” OR “efficacy” OR “sensitivity” OR “specificity”)]. No language or study type restrictions were applied when conducting the initial literature search.
Inclusion criteria
Studies according to all of the following criteria were included: (1) studies involved RS of two parts of normal breast tissue and BC. (2) BC samples in the studies were derived from patients confirmed by pathology or purchased standard BC cell lines. (3) Studies provided true positives (TP), true negatives (TN), false positives (FP), and false negatives (FN) directly or indirectly to construct a 2 × 2 table. (4) The studies were reported in original articles.
Exclusion criteria
The following exclusion criteria were applied: (1) research involving non-human subjects. (2) Other types of study: review articles, letters, case reports, and comments. (3) Patient and data overlapping studies.
Data extraction
Two investigators independently extracted data, and differences were resolved by consensus. Overall, a total of 6 important diagnostic efficiency-related parameters were extracted, including diagnostic sensitivity, specificity, TP, TN, FP, and FN. In addition, methodological and technical data reflecting the baseline characteristics of the studies such as first author name, publication year, geographic location, number of patients, number of spectra, sample type, diagnostic algorithm, and laser wavelength were also carefully extracted.
Literature quality assessment
The standard quality assessment of each study is based on the tool Quality Assessment of Diagnostic Accuracy Studies (QUADAS-2) [22]. It consists of four parts: patient selection, index test, reference standard, flow, and time. The levels of bias risk and applicability concerns were rated as low risk, high risk, and unclear risk. The QUADAS‑2 assessment was performed by Review Manager 5.3
Statistical analysis
The accuracy of RS in diagnosing BC was assessed by pooling TP, TN, FP, and FN data to calculate sensitivity, specificity, positive likelihood ratio (PLR), negative likelihood ratio (NLR) values, corresponding 95% confidence intervals (CI), and diagnostic odds ratio (DOR). Moreover, summary receiver operator characteristics (SROC) [23] were generated to assess the relationship between sensitivity and specificity, and the area under the curve (AUC) was calculated to determine the overall performance of RS. Diagnostic tools are considered excellent when the AUC value is more significant than 0.8. To further explore potential sources of heterogeneity, the inconsistency index (I2) statistic and chi-square test were used for subgroup analysis [24]. I2 > 50% and P-value < 0.05 were considered significant for heterogeneity meanwhile a random‑effects model was applied. We also conducted Deeks’ funnel plot asymmetry test to investigate publication bias [25]. All the above statistical analyses were performed using Stata 16.0.
Results
Study selection
The study screening procedure is presented in a PRISMA flowchart (Fig. 1). After an initial literature search, a total of 2798 articles were identified, which were reduced to 712 after the removal of duplicates. Then, 656 articles were excluded by a manual screening of the titles and abstracts, and 40 articles were removed by reading the full text and reviewing the data. Finally, sixteen articles [26,27,28,29,30,31,32,33,34,35,36,37,38,39,40,41] were enrolled in this meta-analysis according to the inclusion criteria and exclusion criteria. Since the experimental sample population origins were distinct from each other, 2 studies conducted by the same author were both included [31, 32].
Study characteristics
A total of 58,144 spectra from 16 articles were included in this meta-analysis. Most studies were conducted in China (n = 7), Pakistan (n = 3), and the USA (n = 3). Others were conducted in South Korea (n = 1), Japan (n = 1), and the UK (n = 1). Sample types were breast tissue (n = 9), standard cell lines (n = 1), serum (n = 4), whole blood (n = 1), and saliva (n = 1). Among the 16 recruited studies, 11 studies used RS, 4 studies used SERS, and 1 study used Raman confocal spectroscopy (RCS). All of the above studies were performed in vitro with pathological diagnosis as the gold standard. The detailed information of each study we included is shown in Table 1.
Assessment of study quality and publication bias
The assessment of the risk of bias and concerns about the suitability of the included studies according to the QUADAS-2 tool [42] are shown in Fig. 2. Deeks’ tests for publication bias yielded p values of 0.53 which revealed that no significant publication bias was shown in the pooled analysis of the included studies (Fig. 3).
Overall analysis
We measured overall diagnostic accuracy by calculating sensitivity, specificity, PLR, NLR, and diagnostic odds ratio (DOR). The pooled sensitivity and specificity of RS were 0.97 (95% CI, 0.92–0.99) and 0.96 (95% CI, 0.91–0.98), respectively (Fig. 4). The pooled PLR and NLR were 21.98 (95% CI, 10.08–47.96) and 0.03 (95% CI, 0.01–0.09), respectively (Fig. 5). The DOR of RS demonstrated high accuracy (721; [95% CI, 136–3829]). The AUC of the SROC curve was 0.99 (95% CI, 0.98–1) (Fig. 6). Heterogeneity was significant across all pooled studies (I2 > 50%, p < 0.05).
Subgroup analysis
To investigate the effects of different races, sample types, instrument types, numbers of spectra, diode laser wavelengths, and diagnostic algorithms on the accuracy of Raman spectroscopy in distinguishing BC, we performed relevant subgroup analyses, whose results are shown in Table 2. The subgroup of Asian samples, serum samples, PCA algorithm, the numbers of sample spectra more than 200, laser = 785 nm, and RS showed extremely high DOR (1093.98; [95% CI, 133.31–8977.81]), (4247.05; [95% CI, 236.48–76,273.26]), (281,444.30; [95% CI, 293.11–2,700,000]), (2027.29; [95% CI, 196.92–20,871.04]), 779.76 (102.65–5923.19), and (841.31; [95% CI, 89.20–7934.57]), respectively. Moreover, we found that the subgroup of diagnostic algorithm based on PCA outperformed other subgroups in various evaluation indicators, with sensitivity being 1 (95% CI, 0.93–1.00), specificity being 0.98 (95% CI, 0.93–1.00), PLR being 61.60 (95% CI, 14.14–268.46), and NLR being 0 (95% CI, 0.00–0.08).
Discussion
RS has been extensively researched as a new technology that has become widely used in the biomedical field in recent years [42,43,44]. By analyzing 58,144 spectra from 16 studies, we confirmed the superiority and high diagnostic efficiency of RS in diagnosing BC by combining recent findings to systematically investigate the diagnostic performance of RS for BC.
We discovered that the general pooled diagnostic sensitivity and specificity of RS for BC were 0.97 (95% CI, 0.92–0.99) and 0.96 (95% CI, 0.91–0.98), respectively. Both the sensitivity and specificity were over 0.9, indicating that RS had a high identification of BC samples and can distinguish them from normal samples respectively with a low omission diagnostic rate. Furthermore, the random-effects model yielded a pooled DOR of 720.89 (95% CI, 135.73–3828.88). Since a DOR exceeding 1 indicates a high discriminative effect and the discriminative effect increases with the DOR value, the DOR of RS in diagnosing BC has a reliable diagnostic effect. In the SROC curve analysis, the AUC was 0.99 (95% CI, 0.98–1), suggesting an excellent performance for detecting breast cancer samples by using RS. Diagnostic efficiency is considered excellent according to the SROC's standard grading system.
A series of subgroup analyses were performed to further clarify the optimal conditions for the diagnosis of BC by RS. According to the subgroup analysis results, all subgroups performed well, with sensitivity and specificity greater than 0.9. Despite having a lower DOR than the Asian group, the PLR and NLR were comparable in both groups, indicating that RS is capable of screening for BC in all races. Furthermore, our study discovered that RS performed better in serum samples than in breast tissue samples, possibly due to interference from normal breast tissue in breast cancer biopsy samples. Numerous normal spectra can still be collected in a single malignant tissue in our included studies using breast tissue as samples because operators frequently collect dozens of spectra in a single sample, which are thought to be homogeneous. These misclassified spectra are the primary cause of the low diagnostic performance of breast samples. At the same time, the extremely high DOR of the serum sample group also suggests that serum samples may one day be used for BC screening for RS. In terms of sample size for diagnostic results, the pooled DOR decreased significantly when studies with fewer than 200 spectra were included in the subgroup. The explanation for this could be that the multivariate analysis algorithm’s training set was created with mistakes due to the short number of samples, resulting in poor performance in detecting BC and normal tissue. In addition, with the innovation and development of Raman spectroscopy for tumor diagnosis, technologies such as Raman spectroscopy identification and diagnosis based on machine learning are emerging. Our subgroup analysis revealed that RS in conjunction with the PCA algorithm had an excellent diagnostic effect, with a DOR of 28,144.30. Due to a lack of data for the other diagnostic algorithms included, we were unable to analyze them. Besides, subgroup analysis of both the RS and SERS instruments was performed to clarify the impact of different Raman instruments on the experimental results. We discovered that both types of Raman instruments achieved good sensitivity and specificity, despite the fact that the DOR values in the RS group were higher than in the SERS group (841.31 vs 495.98), but this may be due to the significantly smaller number of spectra in the SERS group, and cannot be used to justify RS being better than SERS. It is worth noting that the adsorption of molecules on metal colloidal particles and rough metal surfaces in the SERS principle can increase the spectral intensity of the sample by 10^4–10^6 times, making it suitable for detecting BC in combination with serum samples. We already know that the fourth power of the excitation wavelength has an inverse relationship with the Raman scattering efficiency. It is crucial to select the proper excitation wavelength in order to maximize scattering effectiveness while minimizing fluorescence interference. Our findings indicate that the DOR at wavelength = 785 nm is comparable to the overall DOR, but we were unable to investigate wavelengths above and below 785 nm due to a lack of data. Overall, based on the findings of our subgroup analysis, we have reason to believe that RS combined with the PCA algorithm using serum samples will become an effective method for BC screening in the future.
The inelastic scattering of photons from molecular surfaces causes Raman scattering, and the scattering spectrum is influenced by the energy exchange between the sample molecules and the photons. The sample’s biological makeup can be determined by examining the relative position and strength of each distinctive peak in the Raman spectrum [45]. When compared to normal breast tissue, BC tissue’s lipid and carotenoid contents reduced due to the development of cancer, while its protein content considerably rose. As a result, it was discovered that while the peaks at 853 cm−1 (protein) were greatly enhanced, the peaks at 719 cm−1 (lipid) and 1159 cm−1 (carotenoid) were significantly reduced in RS [46]. RS distinguishes between BC and normal breast tissue in this manner.
RS has the potential to distinguish different stages of BC development in addition to serving as a screening tool for the disease. Han et al. [27] used RS to accurately distinguish four types of breast tissue (normal breast tissue, atypical ductal hyperplasia (ADH), ductal carcinoma in situ (DCIS), and invasive ductal carcinoma (IDC)). The experimental results revealed that the spectrum of the ADH sample had a significant decrease in the peaks representing lipid characteristic peaks (1300 cm−1 represents CH3 deformation frequencies in lipid and 1656 cm−1 represents the C–C stretch of phospholipids) and a significant increase in the peaks representing DNA (1096 cm−1) and protein characteristic peaks (1267 cm−1 represents the C-N stretching mode of protein). Although the overall accuracy could be improved, it is still clinically useful for ADH and DCIS diagnosis. According to Wellings and Jensen’s [47] model of breast cancer development, normal cells in the terminal ductal lobular unit first develop into AH, then DCIS, and finally IDC. Early detection and intervention in the AH stage of breast cancer can reduce the occurrence of cancer [48]. As a result, detecting breast AH by RS is critical for protecting women’s health. RS enables the identification and discovery of cancer cells at an early stage by quantifying changes in the chemical structure and content of substances in breast tissue, which is currently unattainable by other cancer screening methods. We hope to improve the diagnostic performance of RS in the future, making it the primary method of early screening for BC.
Although studies on the application of RS in vivo were excluded due to a lack of TN, TP, FN, and FP, which are required to calculate sensitivity, specificity, and so on, their role in the diagnosis and treatment of BC during surgery cannot be underestimated. Lizio et al. [49]. have developed a novel technique for measuring BC specimens on an intraoperative timescale (20 min) for rapid assessment of BC during surgery. Wen et al. [50] used an AU nanostar-based photoacoustic, surface-enhanced Raman spectroscopy, and thermosurgical probe to create a “three-in-one” therapeutic nanoprobe for residual microtumors in orthotopic BC. Following the treatment strategy for residual microcarcinoma, mouse experiments confirmed that the tumor did not recur after residual tumor eradication. These results show that RS can be employed in clinical settings, particularly given its quick and non-destructive advantages, which can be used to identify tumor boundaries and even remove any remaining cancer following surgery.
The present meta-analysis also has several limitations. First, despite our subgroup analysis, our study’s fundamental drawback is significant heterogeneity, which cannot be minimized. Second, the patient size in each study was small, and the number of spectra varied greatly among the included studies, potentially influencing the results. Third, due to a lack of available data, we were unable to conduct a meta-analysis of various breast cancer pathological subtypes, which may have impacted the accuracy of our findings. We cannot demonstrate that our results accurately separate BC of different subtypes because BC types are complex and diverse. Fourth, standard procedures and protocols for RS diagnosis have not been established, making it difficult to standardize procedures for RS.
Conclusion
As an emerging optical diagnostic technique, RS has great potential in detecting malignant breast lesions. At the same time, it has the advantages of non-invasiveness, real-time, and ease of use. However, before considering real-time use in clinical settings, larger sample size studies are required to determine whether RS can distinguish between different BC subtypes. Meanwhile, the RS’s performance must be further examined and normalized.
Data availability
The data that support the findings of this study are available on request from the corresponding author. The data are not publicly available due to privacy or ethical restrictions.
References
Sung H, Ferlay J, Siegel RL, Laversanne M, Soerjomataram I, Jemal A, Bray F. Global Cancer Statistics 2020: GLOBOCAN estimates of incidence and mortality worldwide for 36 cancers in 185 countries. CA: a cancer journal for clinicians. 2021;71(3):209–249. https://doi.org/10.3322/caac.21660
Kolb TM, Lichy J, Newhouse JH. Comparison of the performance of screening mammography, physical examination, and breast US and evaluation of factors that influence them: an analysis of 27,825 patient evaluations. Radiology. 2002;225(1):165–75. https://doi.org/10.1148/radiol.2251011667.
Berquin A. Douleur chronique : quelques repères pour mieux comprendre et agir. Ann Phys Rehab Med. 2013;56:https://doi.org/10.1016/j.rehab.2013.07.210
Sattlecker M, Bessant C, Smith J, Stone N. Investigation of support vector machines and Raman spectroscopy for lymph node diagnostics. Analyst. 2010;135(5):895–901. https://doi.org/10.1039/b920229c.
Sathyavathi R, Saha A, Soares JS, Spegazzini N, McGee S, Rao Dasari R, Fitzmaurice M, Barman I. Raman spectroscopic sensing of carbonate intercalation in breast microcalcifications at stereotactic biopsy. Sci Rep. 2015;5:9907. https://doi.org/10.1038/srep09907.
Zheng C, Jia HY, Liu LY, Wang Q, Jiang HC, Teng LS, Geng CZ, Jin F, Tang LL, Zhang JG, Wang X, Wang S, Alejandro FE, Wang F, Yu LX, Zhou F, Xiang YJ, Huang SY, Fu QY, Zhang Q, Gao Z, Ma ZB, Li L, Fan ZM, Yu ZG. Molecular fingerprint of precancerous lesions in breast atypical hyperplasia. J Int Med Res. 2020;48(6):300060520931616. https://doi.org/10.1177/0300060520931616.
Kopec M, Imiela A, Abramczyk H. Monitoring glycosylation metabolism in brain and breast cancer by Raman imaging. Sci Rep. 2019;9(1):166. https://doi.org/10.1038/s41598-018-36622-7.
Kopeć M, Abramczyk H. Angiogenesis - a crucial step in breast cancer growth, progression and dissemination by Raman imaging. Spectrochim Acta Part A Mol Biomol Spectrosc. 2018;198:338–45. https://doi.org/10.1016/j.saa.2018.02.058.
Jin H, Lin T, Han P, Yao Y, Zheng D, Hao J, Hu Y, Zeng R. Efficacy of Raman spectroscopy in the diagnosis of bladder cancer: a systematic review and meta-analysis. Medicine. 2019;98(47): e18066. https://doi.org/10.1097/md.0000000000018066.
Jin H, He X, Zhou H, Zhang M, Tang Q, Lin L, Hao J, Zeng R. Efficacy of Raman spectroscopy in the diagnosis of kidney cancer: a systematic review and meta-analysis. Medicine. 2020;99(27): e20933. https://doi.org/10.1097/md.0000000000020933.
Zhang J, Fan Y, Song Y, Xu J. Accuracy of Raman spectroscopy for differentiating skin cancer from normal tissue. Medicine. 2018;97(34): e12022. https://doi.org/10.1097/md.0000000000012022.
Hagan S, Martin E, Enríquez-de-Salamanca A. Tear fluid biomarkers in ocular and systemic disease: potential use for predictive, preventive and personalised medicine. EPMA J. 2016;7(1):15. https://doi.org/10.1186/s13167-016-0065-3.
Lee S, Kim JK. Label-free Raman spectroscopic techniques with morphological and optical characterization for cancer cell analysis. Adv Exp Med Biol. 2021;1310:385–99. https://doi.org/10.1007/978-981-33-6064-8_14.
Blanco-Formoso M, Alvarez-Puebla RA. Cancer diagnosis through SERS and other related techniques. Int J Mol Sci. 2020;21 (6). https://doi.org/10.3390/ijms21062253
Ma N, Zhang XY, Fan W, Guo S, Zhang Y, Liu Y, Chen L, Jung YM. SERS study of Ag/FeS/4-MBA interface based on the SPR effect. Spectrochim Acta Part A Mol Biomol Spectrosc. 2019;219:147–53. https://doi.org/10.1016/j.saa.2019.04.005.
Gao N, Wang Q, Tang J, Yao S, Li H, Yue X, Fu J, Zhong F, Wang T, Wang J. Non-invasive SERS serum detection technology combined with multivariate statistical algorithm for simultaneous screening of cervical cancer and breast cancer. Anal Bioanal Chem. 2021;413(19):4775–84. https://doi.org/10.1007/s00216-021-03431-3.
Cennamo G, Montorio D, Morra VB, Criscuolo C, Lanzillo R, Salvatore E, Camerlingo C, Lisitskiy M, Delfino I, Portaccio M, Lepore M. Surface-enhanced Raman spectroscopy of tears: toward a diagnostic tool for neurodegenerative disease identification. J Biomed Opt. 2020;25(8):1–12. https://doi.org/10.1117/1.Jbo.25.8.087002.
Markina NE, Zakharevich AM, Markin AV. Determination of methotrexate in spiked human urine using SERS-active sorbent. Anal Bioanal Chem. 2020;412(28):7757–66. https://doi.org/10.1007/s00216-020-02932-x.
Wang Z, Zong S, Wu L, Zhu D, Cui Y. SERS-activated platforms for immunoassay: probes, encoding methods, and applications. Chem Rev. 2017;117(12):7910–63. https://doi.org/10.1021/acs.chemrev.7b00027.
Zheng C, Liang L, Xu S, Zhang H, Hu C, Bi L, Fan Z, Han B, Xu W. The use of Au@SiO2 shell-isolated nanoparticle-enhanced Raman spectroscopy for human breast cancer detection. Anal Bioanal Chem. 2014;406(22):5425–32. https://doi.org/10.1007/s00216-014-7967-5.
Shang LW, Ma DY, Fu JJ, Lu YF, Zhao Y, Xu XY, Yin JH. Fluorescence imaging and Raman spectroscopy applied for the accurate diagnosis of breast cancer with deep learning algorithms. Biomed Opt Express. 2020;11(7):3673–83. https://doi.org/10.1364/boe.394772.
Schueler S, Schuetz GM, Dewey M. The revised QUADAS-2 tool. Annals of internal medicine. 2012;156 (4):323; author reply 323–324. https://doi.org/10.7326/0003-4819-156-4-201202210-00018
Moses LE, Shapiro D, Littenberg B. Combining independent studies of a diagnostic test into a summary ROC curve: data-analytic approaches and some additional considerations. Stat Med. 1993;12(14):1293–316. https://doi.org/10.1002/sim.4780121403.
Higgins JP, Thompson SG, Deeks JJ, Altman DG. Measuring inconsistency in meta-analyses BMJ (Clinical research ed). 2003;327(7414):557–60. https://doi.org/10.1136/bmj.327.7414.557.
Begg CB, Mazumdar M. Operating characteristics of a rank correlation test for publication bias. Biometrics. 1994;50(4):1088–101.
Haka AS, Volynskaya Z, Gardecki JA, Nazemi J, Shenk R, Wang N, Dasari RR, Fitzmaurice M, Feld MS. Diagnosing breast cancer using Raman spectroscopy: prospective analysis. J Biomed Opt. 2009;14(5): 054023. https://doi.org/10.1117/1.3247154.
Han B, Du Y, Fu T, Fan Z, Xu S, Hu C, Bi L, Gao T, Zhang H, Xu W. Differences and relationships between normal and atypical ductal hyperplasia, ductal carcinoma in situ, and invasive ductal carcinoma tissues in the breast based on raman spectroscopy. Appl Spectrosc. 2017;71(2):300–7. https://doi.org/10.1177/0003702816681009.
Lazaro-Pacheco D, Shaaban AM, Titiloye NA, Rehman S, Rehman IU. Elucidating the chemical and structural composition of breast cancer using Raman micro-spectroscopy. EXCLI J. 2021;20:1118–32. https://doi.org/10.17179/excli2021-3962.
Ma D, Shang L, Tang J, Bao Y, Fu J, Yin J. Classifying breast cancer tissue by Raman spectroscopy with one-dimensional convolutional neural network. Spectrochim Acta Part A Mol Biomol Spectrosc. 2021;256: 119732. https://doi.org/10.1016/j.saa.2021.119732.
Lin D, Wang Y, Wang T, Zhu Y, Lin X, Lin Y, Feng S. Metabolite profiling of human blood by surface-enhanced Raman spectroscopy for surgery assessment and tumor screening in breast cancer. Anal Bioanal Chem. 2020;412(7):1611–8. https://doi.org/10.1007/s00216-020-02391-4.
Nargis HF, Nawaz H, Ditta A, Mahmood T, Majeed MI, Rashid N, Muddassar M, Bhatti HN, Saleem M, Jilani K, Bonnier F, Byrne HJ. Raman spectroscopy of blood plasma samples from breast cancer patients at different stages. Spectrochim Acta Part A Mol Biomol Spectrosc. 2019;222: 117210. https://doi.org/10.1016/j.saa.2019.117210.
Nargis HF, Nawaz H, Bhatti HN, Jilani K, Saleem M. Comparison of surface enhanced Raman spectroscopy and Raman spectroscopy for the detection of breast cancer based on serum samples. Spectrochim Acta Part A Mol Biomol Spectrosc. 2021;246: 119034. https://doi.org/10.1016/j.saa.2020.119034.
Zhang H, Wang X, Ding R, Shen L, Gao P, Xu H, Xiu C, Zhang H, Song D, Han B. Characterization and imaging of surgical specimens of invasive breast cancer and normal breast tissues with the application of Raman spectral mapping: a feasibility study and comparison with randomized single-point detection method. Oncol Lett. 2020;20(3):2969–76. https://doi.org/10.3892/ol.2020.11804.
Iwasaki K, Araki A, Krishna CM, Maruyama R, Yamamoto T, Noothalapati H. Identification of molecular basis for objective discrimination of breast cancer cells (MCF-7) from normal human mammary epithelial cells by Raman microspectroscopy and multivariate curve resolution analysis. Int J Mol Sci. 2021;22 (2). https://doi.org/10.3390/ijms22020800
Bilal M, Bilal M, Tabassum S, Saleem M, Mahmood H, Sarwar U, Bangush H, Munir F, Aslam Zia M, Ahmed M, Shahzada S, Ullah Khan E. Optical screening of female breast cancer from whole blood using Raman spectroscopy. Appl Spectrosc. 2017;71(5):1004–13. https://doi.org/10.1177/0003702816667516.
Li Q, Li W, Zhang J, Xu Z. An improved k-nearest neighbour method to diagnose breast cancer. Analyst. 2018;143(12):2807–11. https://doi.org/10.1039/c8an00189h.
Koya SK, Brusatori M, Yurgelevic S, Huang C, Werner CW, Kast RE, Shanley J, Sherman M, Honn KV, Maddipati KR, Auner GW. Accurate identification of breast cancer margins in microenvironments of ex-vivo basal and luminal breast cancer tissues using Raman spectroscopy. Prostaglandins Other Lipid Mediat. 2020;151: 106475. https://doi.org/10.1016/j.prostaglandins.2020.106475.
Feng S, Huang S, Lin D, Chen G, Xu Y, Li Y, Huang Z, Pan J, Chen R, Zeng H. Surface-enhanced Raman spectroscopy of saliva proteins for the noninvasive differentiation of benign and malignant breast tumors. Int J Nanomed. 2015;10:537–47. https://doi.org/10.2147/ijn.S71811.
Majumder SK, Keller MD, Boulos FI, Kelley MC, Mahadevan-Jansen A. Comparison of autofluorescence, diffuse reflectance, and Raman spectroscopy for breast tissue discrimination. J Biomed Opt. 2008;13(5): 054009. https://doi.org/10.1117/1.2975962.
Kim S, Kim W, Bang A, Song JY, Shin JH, Choi S. Label-free breast cancer detection using fiber probe-based Raman spectrochemical biomarker-dominated profiles extracted from a mixture analysis algorithm. Analytical methods : advancing methods and applications. 2021;13(29):3249–55. https://doi.org/10.1039/d1ay00491c.
Lin Y, Gao J, Tang S, Zhao X, Zheng M, Gong W, Xie S, Gao S, Yu Y, Lin J. Label-free diagnosis of breast cancer based on serum protein purification assisted surface-enhanced Raman spectroscopy. Spectrochim Acta Part A Mol Biomol Spectrosc. 2021;263: 120234. https://doi.org/10.1016/j.saa.2021.120234.
Giamougiannis P, Silva RVO, Freitas DLD, Lima KMG, Anagnostopoulos A, Angelopoulos G, Naik R, Wood NJ, Martin-Hirsch PL, Martin FL. Raman spectroscopy of blood and urine liquid biopsies for ovarian cancer diagnosis: identification of chemotherapy effects. J Biophotonics. 2021;14(11): e202100195. https://doi.org/10.1002/jbio.202100195.
Zhao J, Zeng H, Kalia S, Lui H. Using Raman spectroscopy to detect and diagnose skin cancer in vivo. Dermatol Clin. 2017;35(4):495–504. https://doi.org/10.1016/j.det.2017.06.010.
Lyng FM, Traynor D, Ramos IR, Bonnier F, Byrne HJ. Raman spectroscopy for screening and diagnosis of cervical cancer. Anal Bioanal Chem. 2015;407(27):8279–89. https://doi.org/10.1007/s00216-015-8946-1.
Downes A, Elfick A. Raman spectroscopy and related techniques in biomedicine. Sensors (Basel, Switzerland). 2010;10(3):1871–89. https://doi.org/10.3390/s100301871.
Rahman K. Studies on free radicals, antioxidants, and co-factors. Clin Interv Aging. 2007;2(2):219–36.
Wellings SR, Jensen HM. On the origin and progression of ductal carcinoma in the human breast. J Natl Cancer Inst. 1973;50(5):1111–8. https://doi.org/10.1093/jnci/50.5.1111.
Kader T, Hill P, Rakha EA, Campbell IG, Gorringe KL. Atypical ductal hyperplasia: update on diagnosis, management, and molecular landscape. Breast cancer research : BCR. 2018;20(1):39. https://doi.org/10.1186/s13058-018-0967-1.
Lizio MG, Liao Z, Shipp DW, Boitor R, Mihai R, Sharp JS, Russell M, Khout H, Rakha EA, Notingher I. Combined total internal reflection AF spectral-imaging and Raman spectroscopy for fast assessment of surgical margins during breast cancer surgery. Biomed Opt Express. 2021;12(2):940–54. https://doi.org/10.1364/boe.411648.
Wen Y, Truong VX, Li M. Real-time intraoperative surface-enhanced Raman spectroscopy-guided thermosurgical eradication of residual microtumors in orthotopic breast cancer. Nano Lett. 2021;21(7):3066–74. https://doi.org/10.1021/acs.nanolett.1c00204.
Acknowledgements
We express our thanks to Prof. Yong Yu from the Department of Ultrasound in Shandong Provincial Hospital who was involved in the revision of our manuscript.
Funding
The work was supported by the Natural Science Foundation of Shandong Province under Grant [ZR2021QH047] and the Clinical Science and Technology Innovation Development Program of Jinan (grant number 202134036).
Author information
Authors and Affiliations
Contributions
Mei-Huan Wang and Qian Wang conceived the idea for the article. Mei-Huan Wang and Xiao Liu conducted data collection and analysis. Qian Wang managed and drafted the manuscript. Hua-Wei Zhang approved the final version of the manuscript. All authors contributed to the article and approved the submitted version.
Corresponding authors
Ethics declarations
Ethics approval and consent to participate
Not applicable.
Consent for publication
Written informed consent for publication of their clinical details and clinical images was obtained from the patient.
Conflict of interest
The authors declare no competing interests.
Additional information
Publisher's note
Springer Nature remains neutral with regard to jurisdictional claims in published maps and institutional affiliations.
Rights and permissions
Springer Nature or its licensor holds exclusive rights to this article under a publishing agreement with the author(s) or other rightsholder(s); author self-archiving of the accepted manuscript version of this article is solely governed by the terms of such publishing agreement and applicable law.
About this article
Cite this article
Wang, MH., Liu, X., Wang, Q. et al. Diagnosis accuracy of Raman spectroscopy in the diagnosis of breast cancer: a meta-analysis. Anal Bioanal Chem 414, 7911–7922 (2022). https://doi.org/10.1007/s00216-022-04326-7
Received:
Revised:
Accepted:
Published:
Issue Date:
DOI: https://doi.org/10.1007/s00216-022-04326-7