Abstract
India being a developing country, urban regions are often found to face extra congestions and excessive delays. With higher level of heterogeneity, lane following behaviour is hardly observed. Two-wheeler and motorized Three-wheeler driver accepts the minimum available lateral gap for overtaking. Complete right of way is utilized including shoulder (if available) for manoeuvres. Along with the longitudinal interaction, lateral interactions of vehicles are also observed on large scale, which mainly result in diminution of overall throughput of roadway. Hence, present study is attempted to study lateral placement of vehicles on urban arterial road. Lateral interaction being most intricate parameter and difficult to study from field, microscopic traffic simulation software VISSIM was used to develop various traffic volumes (From LOS-C to LOS-E). Simulation model was calibrated with data collected from field. Speed being the qualitative parameter, it was considered as prime parameter for model calibration. It has been observed that at moderate traffic flow, vehicles tend to maintain more gap even at low speed. But, at high flow level (LOS-E), this gap maintaining behaviour is very complex and fluctuating. Vehicles were observed to maintain abruptly varying lateral clearances at various percentile speeds. This variation was studied for speed and lateral clearance at 15th, 50th and 85th percentile values for combined data of all LOS volumes.
Access provided by Autonomous University of Puebla. Download conference paper PDF
Similar content being viewed by others
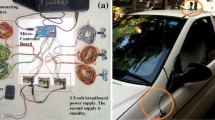
Keywords
1 Introduction
India being a developing country with population around 1.25 billion. The vehicular ownership is also increasing considerably each year. Sub-urban and urban regions are the places where most of the population is attracted. The Central Business District (CBD) zones of the city and the arterials connecting CBD’s to the other zones of city, face a large congestion during peak and even at off peak hours. Vehicles not only interact longitudinally, but also laterally to each other with variable speeds and acceleration. No lanes of traffic are observed, neither there is any lane discipline followed by the vehicles. In context to driving, lane discipline is when a driver keeps his/her vehicle within the road marking indicating lane unless changing lanes or direction [6]. Such lane following scenario is quite unseen in Indian traffic conditions. It is really a difficult task to maintain a lane with a maintained speed, as vehicles interaction leads the vehicles to change the longitudinal as well as lateral position with respect to others. This ultimately results into a poor lane behaviour with higher degree of heterogeneity. The speed of vehicle is likely to affect with regard to its lateral position on the roadway geometry. Lennie and Bunker [5] stated that the lateral position of vehicle is the distance between the carriageway centreline and the nearest edge of the vehicle. Highway {C}apacity {M}anual (2012) states that the lane widths less than 3.6 m reduce the travel speeds, and width larger than this value are not considered to increase speed above the base level. Whether the vehicle is in the centre lane, either curb side or the inner lane, the observed speed of vehicle is obviously dissimilar. Widespread range of physical dimensions, power to weight ratio, and other dynamic characteristics of vehicles lead to higher degree of heterogeneity, with mixed traffic. There exists a mixed traffic flow with no lane discipline. Hence the lateral movement of the vehicles are very high due to no lane discipline. Hence [6] studied the lateral movement of vehicles on straight road. Two wheelers and three wheelers are observed with the most reckless driving. Two wheelers accept the minimum available gap to overtake the other vehicles, by utilising the overall width of carriageway uses the same roadway space resulting in heterogeneous traffic. The bi-directional traffic on some roads utilises the same road space regardless of any physical segregation and occupies any lateral position on the road contingent on the availability of space. This forces the other vehicles to slower down their speeds. As observed, different types of vehicles share the same road space available without any lane discipline. Bunker et al. [1] showed that a maximum 7 s time gap between opposing vehicles influences drivers’ positioning of their vehicles. It was observed that the lateral positions of cars, utility vehicles, and semi-trailers are statistically different when opposed as compared to unopposed. Non-motorised vehicles share the same right of way along with the other passenger cars and heavy vehicles causing congestions and bottlenecks. Pal and Mallikarjuna [8] stated that drivers vary gaps based on the varying traffic conditions. The extent of vehicular interactions varies with the composition of traffic. As a result, causing delay and ultimately reduction in the capacity. Hence proper study of lateral placement of vehicles has become a challenging task, especially in developing countries like India.
Microsimulation is one of the techniques used to model the traffic and study its characteristics. Simulation is widely used tool in many industries for testing or inspecting the validity and reliability of the proposed methodology. Srikanth et al. [10] used the speed flow curves to calibrate the VISSIM model for multilane highways. Transportation industry is not an exception for the application of simulation technique as a use for problem solving. Microsimulation and macrosimulation tools are popular to study and inspect the traffic trends, behaviour and demand. Parking studies, feasibility studies at toll plaza, public transit stations for pedestrians and users, etc. are the applications of microsimulation modelling extensively used in transportation industry. Using computer-based simulation various traffic characteristics like speed, flow, density etc. can be studied. Though microsimulation is widely used to model homogeneous traffic in developed countries, it is also widely used in the developing countries in Asia to model the heterogeneous traffic. Manjunatha et al. [7] proposed a methodology to calibrate the mixed traffic using a microsimulation model. To model the heterogeneous traffic, non-lane following behaviour is not the only challenge, but the speed and acceleration characteristics of a number of vehicle categories, their power to weight ratio, dimensions, etc. are also to be taken into consideration. Hence to study the lateral behaviour of the vehicles, microsimulation software VISSIM is used in the present study. Kaur [4] modelled the heterogeneous traffic using VISSIM. The calibration of the selected study stretch is done using speed, flow, density curves and further the relation of speed with lateral clearance is compared. The coordinate data of vehicles from the VISSIM software is used to find out the lateral gaps between the different vehicle categories. VISSIM is the state-of-the-art multi-modal simulation software developed by PTV AG, Germany, popularly used to model both homogeneous and heterogeneous traffic. To reflect the field conditions with the simulation model, calibration is done for the selected data set. Drivers are forced to accept the gap as per the prevailing traffic conditions. Figure 1 outlines the site picture taken from the corridor, with a view of the study stretch.
Number of vehicle classes comprising different dimensions, speed and acceleration characteristics, and occupancy characterise the Indian traffic. Not only motorised but also non-motorised vehicles share the same right of way with other vehicles. These slow moving non-motorised vehicles followed by the reckless driving behaviour of three-wheelers and motorised two-wheelers force the passenger cars to slow down their speeds. Two-wheeler drivers accept the minimum available gap to surpass the vehicles, which also leads to diamond shaped queuing at intersections. Curb side bus stops are cause for the bus to wait until the passenger’s board/alight, which forms a bottleneck on the urban roadways. Roadside parking’s, frequent stopping of three-wheeler drivers at curb side, to board or alight more passengers for more fare, ultimately results into a mixed traffic condition with poor lane following behaviour. The degree of heterogeneity is hence observed more in case of developing countries than that of the developed countries, which are having proper lane following behaviour [9]. Hence to study such a complex and mixed traffic behaviour microsimulation technique might present a faithful outcome for obtaining the desired results. Hence, prior data is required to be collected for erecting successful simulation model with minimal errors.
2 Data Collection and Analysis
The study stretch considered was free from U-turning or crossing movements of vehicles, there was not any direct access for vehicles to enter, merge or diverge near the study stretch, the stretch was fairly straight and of uniform width throughout. The care of side friction, which is often observed in urban intercity roads was also taken in consideration that, there were no curb side parking’s at the stretch. These objectives were defined for selection of the study section; as pure interactions of the operating vehicles are to be studied. Also, the selected study stretch was free from gradient (firm) and curvature. The urban roads also often face problems like undesignated crossings of pedestrians. To encounter the issues related to pedestrians, as discussed in the previous section, the study stretch was selected near a foot-over bridge (FOB) facility for pedestrians.
By virtue of the FOB and divided roadway, the pedestrians were not observed to cross on the road section. Video graphic method of data collection was used to capture the wide-ranging variation of the vehicular interactions. Outer ring road from New Delhi was selected for the data collection purpose. Over the selected urban intercity arterial road, a 30 m section was marked for studying the interactions of vehicles. A wide lens high pixel resolution camera was used. The camera was placed on the edge of FOB in order to cover all three lanes (one direction) of traffic clearly. Figure 1 shows the Google image of the location of the study stretch. It is located at the Pamposh Enclave, greater Kailash, New Delhi. The collected data from the field was then analysed by Avidemux Software with high precision, having ten further parts (microseconds) of one second, for more accurate speed data of individual vehicles. The speed of 30-m study stretch was calculated for each class of vehicle, and this speed was considered as the average speed of particular vehicle class over the mid-block section of the arterial. The data was collected for 2 h duration. Out of various categories of vehicles were observed from the video, which were converted into five main categories for simplicity in the analysis. The categories of vehicles defined in the present study were namely two-wheeler (2W), three-wheeler (3W), small car (SC), big car (BC) and heavy vehicles (HV). The proportion and the average dimensions considered in the present study are as shown in Table 1. The dimensions for the set of vehicles which were observed while data collection was extracted from the official websites of respective motor vehicles. These dimensions were used to model the vehicles in the simulation model as well, in order to integrate more accuracy to vehicular dimensions from the simulation model and field.
The two-wheeler was composed of all motorised two-wheeler vehicles (excluding bi-cycle). Three-wheelers consists of motorised three-wheeler vehicles often observed in the urban regions. The car traffic was divided into two main classes. SC consists of sedan, prime-sedan, hatchback cars moderately having the engine capacity up to 1000–1200 horsepower. BCs consists of sport utility vehicles (SUVs) having more occupancy and engine capacity as compared to SCs. The length of BC vehicles is also comparatively more than those of SC. Lastly, buses, trucks, tempos, multi-axle vehicles and containers were converted into a single HV category of vehicles, as their speed and acceleration characteristics are nearly similar.
3 Capacity of Roadway Section
The capacity of a system can be defined as the number of vehicles, passengers or the like, can accommodate a system under the prevailing roadway and traffic conditions. Capacity is independent of demand. It is constant, irrespective of the total number of vehicles demanding the service. Dhamaniya and Chandra [3] stated that the lane capacity of was soundly related to the operating speed of the vehicles in the stream. In the present study, the capacity of roadway is calculated using the Greenshields model. Figure 2 refers the capacity of the study stretch and it was found to be 5957 veh./h and 6034 PCU/h.
Capacity of a roadway is a probabilistic approach and may vary with time and position. It is difficult to derive capacity analytically, hence in most of the practices, capacity is calculated from the field observed conditions.
4 Methodology for Calibration
The collected data was used as input for the simulation model development. The Calibration is progression to refine the model to replicate the observed data to an adequate level of accuracy (with negligible errors). The main aim of calibration is to satisfy the model objective to replicate the field conditions within the developed model. In the present study calibration was done by adjusting the driver’s behaviour parameters which are most important to modify the output of the model. VISSIM is by default a homogeneous lane-based traffic tool, developed by PTV-AG, Germany. It is based on the traffic conditions that observed in developed countries, especially from European and American continent. However, a heterogeneous non-lane-based traffic can also be modelled by a rigorous modification in drivers’ behaviour parameters. This flexibility of VISSIM has mainly achieved worldwide popularity for simulating the traffic. Many researchers used VISSIM to model the heterogeneous traffic, mainly from the developing countries including India. The flow chart for calibration is as shown in Fig. 3.
5 Calibration of Speed Data
Speed being the qualitative measure of traffic, it was considered as the primary parameter to calibrate for the simulation model being developed. Various percentile values of speed were calculated, and used as input for the desired speed distribution curve in the VISSIM interface, for each category of vehicle. The speed distribution of vehicles in the simulation model will replicate more precisely with the field data, if a greater number of percentile speed values are given to the speed distribution curve. Figure 5 shows the speed distribution for SC. The respective fractional values of cumulative frequency of speed were used to obtain the percentile value to be input in the desired speed distribution curve of vehicles in VISSIM, hence a separate statistical chi-squared test is not required.
The most basic and fundamental parameters of traffic are considered to be the speed, density and the volume. Hence, in the present study the comparison between the field observed and simulation results was done by associating the degree of similarity between the speed, density and volume of traffic of both. In the subsequent part of the study, the comparison of speed-flow and flow-density is also presented.
From Fig. 6a, b it can be observed that the results obtained from the simulation model are nearly matching with those of the field observed data values. This comparison was checked graphically, but to check for more accuracy and reliability of the simulation model results, (Analysis of Variance) ANOVA test for the three fundamental parameters speed, density and volume.
The ANOVA test was performed between the field observed data set, and the same data set obtained from the simulation model, for one-hour duration each. If there is any significant difference within the dataset is checked using the test. The Null Hypothesis was considered as “There is no significant difference between the two datasets”, and the Alternate Hypothesis was “There is significant difference between the two datasets”. Table 2 shows the results of ANOVA test.
From the ANOVA test results table, it can be seen that the P-value obtained for flow, density and speed is 0.3568, 0.8607 and 0.0884. All of these three P-values are greater than the P-critical value, which is 0.05 at 5 degrees of freedom. From this, it can be stated that there is no significant difference is observed between the field observed values and simulation model values of speed, flow and density. Hence, the model is said to represent the field observed vehicular traffic conditions (with negligible errors). As, the basic fundamental quantities of traffic, on which the overall performance of the roadway system can be judged with volume to capacity ratio, delay, etc. can be derived from these three basic quantities. Hence, these three quantities were considered for the model calibration purpose.
The calibrated simulation model was used to simulate the various volume levels for the study stretch. The vehicular volume ranging from LOS-C (moderately low traffic) to LOS-E (Dense Traffic). The volume at various LOS levels were calculated based on the V/C ratio criteria, as capacity value was already obtained for the study stretch.
To represent the volume or capacity of a system in context to number of vehicles per unit time cannot be an appropriate method of representation. As, the combined effect of 2W in numbers is completely different as compared to numbers of HV. Hence, in the present study the capacity of the study section is converted into equivalent number of PCU/h. The method proposed by [2] was used to find the PCU values of vehicles.
where, Vc, Vi is the speed of passenger car (SC in the present study) and subject vehicle respectively, and Ac, Ai is the projected rectangular area of the passenger car and subject vehicle, respectively. As SC is considered as the standard passenger vehicle, all other class of vehicles were converted into the equivalent number of SC. PCU of a vehicle type is very dynamic in nature and it has impact of all variables that affect the driving behaviour of the vehicles. Hence, the PCU for each class of vehicle was calculated and the overall throughput of traffic stream was measured in PCU/h. Based on the various volume levels, the Passenger car unit (PCU) values was calculated. The variation of PCU value at various LOS level was studied. Table 3 shows the variation of PCU with respect to volume and V/C ratio.
To check the reliability of the calculated PCU values for the developed simulation model, the observed composition of heterogeneous traffic was made run for one hour into the developed simulation model with the previous volumes, obtained from the various V/C ratios considered for the study. Further, this traffic was converted into the PCU/h traffic using the PCU values obtained for specified vehicle class at particular volume. The standard dimensions of field observed set of vehicles, those obtained from the official websites of the respective vehicles were used to model the dimensions of vehicles in VISSIM. Accompanying to this, for same volumes considered, the total vehicle input given to the simulation model was of only SC, i.e. the standard passenger vehicle considered for the study. The results obtained from the equivalent PCU/h volume and the SC only volume from the simulation were compared. The t-test was applied to the obtained results. The results obtained for one-hour simulation, i.e. for sixty data points were considered for the t-test. The t0 value obtained was 3.08 and the critical value was 5.73 which is greater than the t0, hence it proves that there is no significant difference between the two data sets. Hence the simulation model and the PCU values both are said to be valid and reliable, at specified volume levels. Figure 7 shows the comparison of the SC only data and PCU/h data of the volume, obtained at various volume levels.
Figure 7 describes about the similarity of the calibrated data of SC only traffic, with the equivalent mixed traffic in the simulation model. Which implies that the simulation model is capable of replicating the field conditions effectively with minimal errors.
6 Modelling Lateral Clearance of Vehicles
From the number of simulations runs with different random increment of seed for each simulation run, the average data was obtained, purposefully for more accurate results of analysis. The variation of vehicle specific speed with the lateral clearance maintained with adjacent vehicles was observed. All possible combinations of five class of vehicles with each other were extracted from the trajectory data. The coordinates of vehicles were obtained for every time step in simulation run. One second was divided into five parts (five-time steps per second) for more accurate results. Firstly, the centre to centre (c/c) distance of vehicles were extracted and then half of the widths of two adjacent vehicles was subtracted from the c/c lateral clearance between the vehicles. This lateral spacing (clearance) of vehicles was studied further. It was observed that, the lateral clearance of vehicles varied with respect to speed of vehicle, type of vehicle (size/dimensions/manoeuvrability), and the lane in which the vehicle is moving. Large variation of lateral clearance was observed among the vehicle pairs. It was observed from field that a vehicle moving adjacent to another vehicle, within a one-metre boundary has significant effect over its lateral movement, hence the range of lateral clearance values was selected from zero to one metre from the data set. The trajectory data obtained from the simulation run was completely in a scattered form, hence the outlying data (outliers) was removed from the analysis, as those data points would not make substantial difference to the results. As a vehicle within the lane is moving adjacent to another vehicle within one-metre distance laterally, then it is believed to have had an effect on the parallelly moving vehicle. This variation of speed of vehicle with the lateral clearance was captured with all pairs considering one vehicle pair at a time. For 2W as the subject vehicle, the results of speed versus (vs.) lateral clearance share as maintained with all categories of vehicles is plotted. Though the data points are scattered, the outliers were removed to obtain the results based on the data points which actually matters for the lateral clearance behaviour.
From Fig. 8a–e, it can be observed that the lateral clearance for the 2W varies significantly as the subject vehicle (vehicle adjacent to it) changes. For heavy vehicles, there is not much difference in the clearance maintained at various increasing speed levels, but in case of 2W and 3W these fluctuations are different. The cumulative frequency of the speed and lateral clearance together is represented in Fig. 9, considering 2W as standard vehicle.
Here, in Fig. 9, the term V-2w refers to the combined speed data of the 2W, while considered to calculate the lateral clearance with all vehicle classes, including 2W. Hence, V-2w implies the combined speed profile of 2W with all vehicles moving adjacent, and the other five curves of all vehicle classes represent the variation of lateral clearance of the specific vehicle with 2W as the subject (adjacent) vehicle. Figure 9 explains the variation of lateral clearance maintaining behaviour of all vehicles together, with their cumulative frequency distribution compared with the combined speed data of subject vehicle (2W).
7 Percentile Variation of Speed Versus Lateral Clearance
The field practices are however limited to minimum (15th), average (50th) and maximum (85th) speed limits of the vehicles. The overall data obtained from the simulation results of all three LOS levels (LOS-C, D and E) was combined together and a combined percentile variation was studied for the traffic stream. The design of the roadway is mainly based on these numbers, hence a typical percentile variation of percentile speed versus percentile lateral clearance for combined data is shown in Fig. 10.
In Fig. 10, the three percentile values mentioning the 15th, 50th and 85th percentile value, respectively for the three points in the figure from left to right. It has been observed that, as percentile speed increases, vehicles lean towards maintaining more distance with the vehicles moving adjacent to them. It was observed from field that, 2W drivers accept the minimum available gap (available lateral clearance between the adjacent vehicles) to overtake the vehicles, even at congested conditions. This heterogeneous behaviour of 2W was clearly captured. The clearance maintained by 2W with adjoining 2W was least, as compared to any other vehicle class moving adjoining with 2W. This lead 2W pair to have the least lateral clearance values at 50th and even at 85th percentile speeds which may be due to the smaller dimensions and better manoeuvrability characteristics of 2W. In contrast to this, the clearance maintained by HV was maximum at 50th and 85th percentile speed, as compared to all other vehicles. This is observed due to the large dimensions of HV. When a HV is operating at considerably higher speed, it was observed that the vehicles present on the roadway were maintaining larger gaps with HV and provides the right of way for HV to move. Psychologically, it can be stated that the large dimensions/size of heavy vehicles might be the reason behind this behaviour of other vehicles, and 2W is not an exception to this. Even, SC and BC have similar manoeuvrability and acceleration deceleration capabilities, but a BC is slightly superior to SC in case of engine capacity and size. The percentile speed values and the clearance maintained by BC with 2W were also observed to be more than SC by 22% at 15th percentile, and 25% at 50th percentile. This may be due to slight superiority characteristics of BC over SC in case of engine capacity and manoeuvrability. An increasing trend was observed between the speed and the lateral clearance of vehicles, with increase in speed of vehicles. Furthermore, it was observed that the clearance value surged with vehicle size ranging from 2W to HV.
8 Conclusions
The calibrated VISSIM model was used to simulate the various levels of traffic volume level. The capacity of the midblock section is obtained using the Greenshields’s model. The behaviour of individual vehicles is likely to change with the variation in the traffic stream, and it is very difficult to study this from field. The lateral behaviour being a very intricate and complex parameter, hence it was studied using the microsimulation technique. The reliability of the simulation model was checked by calibrating the speed, volume and density of the traffic stream. The speed data collected for each vehicle category was calibrated separately, for the simulation model to represent the actual field conditions more accurately. Also, the default drivers’ parameters in the VISSIM software were modified using trial and error method to replicate the volume and density that observed from field. The capacity of the urban arterial road selected for study was obtained as 5957 veh./h. (6034 PCU/h). The six-lane divided urban arterial road was observed to have V/C ratio as 0.91 and hence the Level of Service of the system was obtained as E. The PCU of vehicles was calculated at each volume level and their variation was studied with the V/C ratio. Further, the variation of speed of vehicles with lateral clearance with the vehicles in the traffic stream was studied. Five categories of vehicles, with one type as subject vehicle was considered. The percentile variation of speed with lateral clearance was also studied for 15th, 50th and 85th percentile values.
References
Bunker J, Parajuli A (2006) Examining lateral positions of cars and heavy vehicles on a two lane, two way motorway. Transp Eng Australia 10(2):129–139
Chandra S, Kumar U (2003) Effect of lane width on capacity under mixed traffic conditions in India. J Transp Eng 129(2):155–160
Dhamaniya A, Chandra S (2017) Influence of operating speed on capacity of urban arterial midblock sections. Int J Civ Eng 15(7):1053–1062
Kaur S (2015) Use of Vissim in heterogeneous traffic modelling—a review:453–454
Lennie S, Bunker J (2004) Using lateral position information as a measure of driver behaviour around MCVs:1–19
Mahapatra G, Maurya AK (2013) Study of vehicles lateral movement in non-lane discipline traffic stream on a straight road. Proc Soc Behav Sci 104:352–359
Manjunatha P, Vortisch P, Mathew T (2013) Methodology for the calibration of VISSIM in mixed traffic. In: Transportation research board 92nd annual meeting, p 11
Pal D, Mallikarjuna C (2016) Analysis of the effect of variable lateral gap maintaining behavior of vehicles on traffic flow modeling. Proc Eng 142:197–203
Siddharth SMP, Ramadurai G (2013) Calibration of VISSIM for Indian heterogeneous traffic conditions. Proc Soc Behav Sci 104:380–389
Srikanth S, Mehar A, Parihar A (2017) Calibration of Vissim model for multilane highways using speed flow curves. Stavební obzor Civ Eng J 26(3):303–314
Author information
Authors and Affiliations
Editor information
Editors and Affiliations
Rights and permissions
Copyright information
© 2022 The Author(s), under exclusive license to Springer Nature Singapore Pte Ltd.
About this paper
Cite this paper
Deshmukh, M., Dhamaniya, A. (2022). Lateral Placement of Vehicles Under Mixed Traffic in Indian Urban Scenario. In: Maurya, A.K., Maitra, B., Rastogi, R., Das, A. (eds) Proceedings of the Fifth International Conference of Transportation Research Group of India . Lecture Notes in Civil Engineering, vol 219. Springer, Singapore. https://doi.org/10.1007/978-981-16-8259-9_32
Download citation
DOI: https://doi.org/10.1007/978-981-16-8259-9_32
Published:
Publisher Name: Springer, Singapore
Print ISBN: 978-981-16-8258-2
Online ISBN: 978-981-16-8259-9
eBook Packages: EngineeringEngineering (R0)