Abstract
Diamond burnishing is a surface modification method aimed at improvements in the surface integrity (SI) and operating behavior of metal components. A cost-effective optimization approach for increasing the fatigue strength of diamond-burnished steel components has been developed. The basic idea is that the fatigue strength can be controlled by controlling some of the SI characteristics (surface micro-hardness, hardened-layer depth and roughness) whose measurements are not time-consuming and expensive. Thus, a multi-objective optimization task was set and solved using the weight vector method. The governing factors were the diamond radius and burnishing force. The resulting fatigue limit differed from the maximum fatigue limit by a mere 0.44%, which proves the effectiveness of the proposed approach. The results obtained for the fatigue limit are explained by means of an X-ray analysis of the introduced residual stresses and an analysis of the microstructures of the surface and subsurface layers. It has been established that a greater depth of the affected zone coupled with a smaller gradient in the alteration of the microstructure in depth provides larger fatigue strength.
Similar content being viewed by others
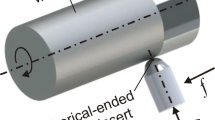
Avoid common mistakes on your manuscript.
1 Introduction
Surface modification technologies are effective tools for increasing the fatigue strength of metal components. One such technology is mechanical surface treatment (MST), which is based on severe plastic deformation, and one of the methods used to implement MST is diamond burnishing (DB). In general, DB is perceived of as a typical smooth burnishing process. However, using an appropriate combination of process governing factors, DB can also achieve deep burnishing and thus significantly increase the fatigue strength [1]. The direct approach to optimizing the DB process requires a planned experiment and subsequent regression analysis to obtain a combination of governing factors that can provide maximum fatigue strength. The fatigue strength for each experimental point in the experimental design is obtained via time-consuming and expensive fatigue tests (Wöhler’s curves or accelerated methods) requiring special equipment and trained operators. The indirect assessment of fatigue strength via introduced residual stresses is also an expensive and time-consuming experiment: A reliable method for measuring residual stresses, such as the X-ray diffraction technique, requires expensive equipment and a highly skilled operator.
Fatigue strength increases due to DB’s severe plastic deformation (significant cold work) of the surface and subsurface layers. Residual compressive stresses are introduced, and the surface micro-hardness increases significantly. The physical carrier of this macro-effect is the modified microstructure produced by the strain hardening. Strain hardening (coldwork) is measured through the surface micro-hardness and the depth of the hardened layer. Obviously, a correlation exists between the surface micro-hardness and the fatigue strength of the diamond-burnished component. There is no information in the literature concerning how this correlation is related to DB although the singular relationships between the micro-hardness and fatigue strength and DB have been investigated by many authors. The surface hardness [2,3,4,5,6,7] and micro-hardness distributions [8] at a depth from the surface due to roller [2,3,4,5] and ball [6,7,8] burnishing were investigated for non-ferrous materials [2, 4, 5, 8] and steels [3, 6, 7]. In the last two decades, a number of studies [9,10,11,12,13,14,15,16,17,18,19,20,21,22,23,24,25,26,27] have focused on the effect of slide burnishing (SB), and, in particular, DB, on the micro-hardness of both non-ferrous metals and alloys as well as steels. The effect of DB on the fatigue strength of steel components was investigated by Aliev and Aslanov [28] for stainless steel, Korzynski et al. [22] for 42CrMo4 steel, Korzynski et al. [29] for 42CrMo4 and 41Cr4 steels with chromium coatings, Maximov et al. [30] for 42Cr4 steel, Maximov et al. [31] for 37Cr4 steel, Swirad [32] for 40HM steel, Maximov et al. [24] for AISI 316Ti steel and Maximov et al. [1, 33] for 41Cr4 steel. However, the correlations between the fatigue strength and the micro-hardness obtained in these studies have not been investigated.
Different combinations of the magnitudes of the governing factors for DB lead to different complex sets of surface layer qualities, known collectively as the surface integrity (SI). A direct correlation exists between the SI and the fatigue strength of a treated metal component, thus allowing the fatigue strength to be controlled by some of the SI characteristics whose measurements are not time-consuming and expensive. For instance, the measurement of the micro-hardness is significantly more affordable and significantly less expensive than an X-ray stress analysis and fatigue tests. It is commonly claimed that fatigue strength increases with the increase in surface micro-hardness by means of the burnishing technologies. However, the achievement of excessively great surface micro-hardness is associated with a significant increase in the density of the dislocations in the surface layer, which leads to the introduction of micro-defects. Therefore, the relationship between micro-hardness and the fatigue strength is not synonymous. On this basis, a cost-effective optimization approach has been developed in this study in order to achieve fatigue strength very close to the maximum.
2 Materials and methods
The material used was 41Cr4 steel. This steel is a typical representative of the medium-carbon, low-alloy steels and used widely in engineering practice. The steel was received in the form of \( \varphi 20\;{\text{mm}} \) bar stock with lengths of 3000 mm. The chemical composition (in wt%), which we established, was as follows: C—0.41, Si—0.25, Mn—0.71, Cr—0.93, P—0.012, S—0.012, Cu—0.28, Ni—0.09, Al—0.024, Ti—0.022, Mo—0.015 and Fe—balance. Tensile and fatigue tests were carried out in our “Testing of Metals” laboratory. The average mechanical characteristics were: Young’s modulus E = 2 × 105 MPa, yield limit \( \sigma_{0.2} = 789\;{\text{MPa}} \), ultimate stress \( \sigma_{u} = 986\;{\text{MPa}} \), elongation \( A_{5} = 10.3\% \), transverse contraction \( z_{t} = 26\% \) and fatigue limit \( \sigma_{ - 1} = 440\;{\text{MPa}} \).
The micro-hardness measurements were conducted on a \( {\text{ZHV}}\mu \) micro-tester. The basic specimens had diameters of 12 mm, as they were consistent with the fact that a layer of material was removed from the bars (“as received”) via turning in order to obtain fatigued specimens. The micro-hardness specimens were manufactured on a CNC T200 lathe using a special burnishing device mounted on the tool post of the lathe (Fig. 1). A polycrystalline diamond tool with a spherical tip was supported elastically within the device. The required burnishing force was set by deforming an axial spring with linear behavior situated within the device. The deforming diamond insert was brought into contact with the specimen at its centerline and normal to the surface being treated. The device was then fed into the specimen an additional 0.05 mm to allow the diamond tool to become disengaged from the stop in the device. The latter was then fed along the surface of the rotating specimen to produce a burnished surface.
Four workpieces were used to produce the micro-hardness specimens. Each workpiece was designed for DB and consisted of four cylindrical surfaces with different burnishing forces but identical diamond insert radii, as shown in Fig. 1. The initial workpieces had a length of 160 mm and diameter of 20 mm. Each workpiece was clamped to one side with the chuck and supported on the other side. Turning and DB were conducted in one clamping process to minimize the concentric run-out in burnishing. The turning was conducted from end to end for each workpiece using a DNMG 50608 – RF carbide cutting insert. The length treated via DB using one and the same combination of governing factors was 20 mm. DB was implemented with a constant feed rate of \( f = 0.05\;{\text{mm/rev}} \) and burnishing velocity of \( v = 100\;{\text{m/min}} \) but with different combinations of diamond radii and burnishing forces. Finally, four micro-hardness specimens were cut from each workpiece for a total of 16 specimens.
For each cylindrical specimen (experimental point from the experimental design), the surface micro-hardness was measured along six equidistant-to-one-another generatrixes belonging to the cylindrical surface. Ten measurements were taken for each generatrix for a total of 60 measurements per specimen. For each specimen, a statistical graph of the measurements was made, and the scattering interval and the clustering center (median) were found. The result corresponding to the median was accepted as the final result for the surface micro-hardness of the corresponding specimen.
For each specimen, the depth of the hardened layer was determined by following the algorithm as follows:
-
1.
One of the two face surfaces of each specimen is polished;
-
2.
The micro-hardness is measured along eight equally spaced radial directions to a depth of 1 mm from the surface in 0.05 mm increments;
-
3.
For each incremental depth from the surface, the arithmetic mean of the results of the eight measurements is calculated; for each specimen, the measured surface micro-hardness is added to the resulting database;
-
4.
The resulting database for the micro-hardness distribution for each specimen is visualized in the “micro-hardness–depth of hardened-layer” coordinate system;
-
5.
For each specimen, the arithmetic mean of the micro-hardness is calculated at a depth from the surface in the 0.3–1-mm interval. This arithmetic mean is the initial micro-hardness;
-
6.
For each specimen, the hardened-layer depth is defined by the intersection of the initial micro-hardness and the HV = HV(depth) graphics.
An experiment was conducted in order to obtain the surface micro-hardness and the depth of the hardened layer. The basic DB parameters are the sphere radius r of the deforming diamond insert, burnishing force Fb, feed rate f and burnishing velocity v. In our previous studies on DB, it was established that the radius and burnishing force have the strongest effect on the SI and fatigue performance of the processed component. Therefore, the governing factors in the present study were the radius and burnishing force. The feed rate and the burnishing velocity were chosen as \( f = 0.05\;{\text{mm/rev}} \) and \( v \le 100\;{\text{m/min}} \). Higher velocities cause more heat to be generated, and higher temperatures in the contact between the diamond insert and the surface being treated lead to the so-called softening effect, which reduce the beneficial effects of the compressive residual stresses (RS) on the surface and subsurface layers [24]. The number of passes, working scheme and use of lubricant are additional parameters. In the present study, one pass and the lubricant Hacut 795-H were used. The levels of the governing factors are shown in Table 1. The objective functions were the surface micro-hardness YHV and the depth of the hardened layer Yd. The experimental points for the experimental design are shown in Table 2. The desired functions YHV = YHV({X}) and Yd = Yd({X}) were obtained via regression analyses, where {X} = [x1, x2]T is the vector of the governing factors.
In our previous study [1, 33], regression models for the roughness and rotating bending fatigue limit of hourglass-shaped fatigue specimens were established. The specimens were made of the same batch 41Cr4 steel (\( \varphi 20\;{\text{mm}} \)) and were also diamond burnished with different combinations of radii and burnishing forces according to Tables 1 and 2. The feed rate and burnishing velocity were, respectively, \( f = 0.05\;{\text{mm/rev}} \) and \( v \le 100\;{\text{m/min}}. \) The minimum diameter of the fatigue specimen was 7.5 mm. The objective functions \( Y_{{\sigma_{ - 1} }} \) (fatigue limit) and \( Y_{\text{Ra}} \) (roughness) depending on the radius and burnishing force in coded form were found via regression analyses.
In order to obtain the \( \hbox{max} Y_{{\sigma_{ - 1} }} = \hbox{max} Y_{{\sigma_{ - 1} }} \left( {Y_{\text{HV}} } \right) \) function, the following computational procedure was developed and used:
-
1.
The micro-hardness interval \( Y_{\text{HV,min}} \le Y_{1} \le Y_{\text{HV,max}} \) is divided into k subintervals, where \( Y_{\text{HV,max}} = Y_{\text{HV,min}} + k\Delta Y_{1} \) (Fig. 2);
-
2.
In the vicinity of each value \( Y_{{{\text{HV}},i}} \), a micro-interval \( \left( {\left( {Y_{{{\text{HV}},i}} - \varepsilon } \right)\;\left( {Y_{{{\text{HV}},i}} + \varepsilon } \right)} \right) \) is defined, where \( \varepsilon \) is a small (in comparison with the measured surface micro-hardness) positive number, for example, in the interval (0.1, 5);
-
3.
The one-objective optimization tasks are solved with regard to \( Y_{{\sigma_{ - 1} }} \) for a defined limit of \( Y_{\text{HV}} \), i.e.,
$$ \begin{aligned} & Y_{{\sigma_{ - 1} ,i}} \left( {X_{i}^{*} } \right) = \hbox{max} Y_{{\sigma_{ - 1} ,i}} > Y_{{\sigma_{ - 1} }} \left( X \right) \\ & \left( {Y_{{{\text{HV}},i}} - \varepsilon } \right) \le Y_{\text{HV}} (\{ X\} ) \le \left( {Y_{{{\text{HV}},i}} + \varepsilon } \right) \\ & \left\{ {X_{i}^{*} } \right\} = \left[ {x_{1}^{(i)*} x_{2}^{(i)*} } \right]^{\text{T}} \in \varGamma ,\quad \forall i = 1,2, \ldots ,k, \\ \end{aligned} $$where \( \left\{ {X_{i}^{*} } \right\} \) is the vector of the optimal governing factors for the ith iteration and \( \varGamma \) is the governing factor space.
-
4.
The magnitude \( Y_{{{\text{HV}},i}} (X_{i}^{*} ) \) of the \( Y_{\text{HV}} (X) \) function is calculated.
Thus, a tabular function for k pairs \( Y_{{{\text{HV}},i}} \left( {X_{i}^{*} } \right),\;\hbox{max} Y_{{\sigma_{ - 1} ,i}} \left( {X_{i}^{*} } \right) \) was obtained.
In an analogous manner, the tabulated forms of the \( \hbox{max} Y_{{\sigma_{ - 1} }} = \hbox{max} Y_{{\sigma_{ - 1} }} \left( {Y_{d} } \right) \) and \( \hbox{max} Y_{{\sigma_{ - 1} }} = \hbox{max} Y_{{\sigma_{ - 1} }} \left( {Y_{\text{Ra}} } \right) \) functions were found.
The RSs in the surface and subsurface layers of the burnished specimens were measured using the X-ray diffraction technique. To analyze the stress gradient under the specimen’s surface, the material layers were gradually removed by electrolytic polishing. Diffraction measurements were made using a vertical θ/θ X’Pert PRO MPD diffractometer with a pin-hole collimator of 1.0 × 1.0 mm2 in the primary beam. The positioning of the measured specimen used a six-degree-of-freedom system and laser triangulation with an accuracy of approximately 5 μm. The effective penetration depth of the CrKα radiation into the investigated steel was approximately 4 μm, which is why a biaxial stressed state was adopted, and the \( \sin^{2} \psi \) method was implemented to estimate the residual stresses using the least squares fitting procedure. The measured diffraction profiles of the α-Fe {211} planes had maximums at \( 2\theta \approx 156.4^{ \circ } \) for filtered CrKα radiation. The diffraction profiles were fitted using the Pearson VII function, and the lattice strains were calculated. The X-ray elastic constants, \( s_{1} = 1.25 \times 10^{ - 6} \;{\text{MPa}}^{ - 1} \) and \( 1/2\;s_{2} = 5.76 \times 10^{ - 6} \;{\text{MPa}}^{ - 1} \), were used in Hooke’s law.
The analysis of the microstructures of the surface and subsurface layers was conducted in accordance with ISO 6507-1 and ISO 6508-1 using an NEOPHOT 2 microscope. The enlargements that were used were in interval between 100 × and 1000×. Figure 3 shows the investigated zones of each specimen. Any zone with a formed texture due to DB was denoted as an “affected zone.”
3 Results and Discussion
The measured surface micro-hardness for all 16 experimental points is depicted in Fig. 4. The outcomes for surface micro-hardness and the depth of the hardened layer, which were subjected to regression analyses, are outlined in Tables 3 and 4. Regression analyses were conducted using QStatLab software [34]. Since the governing factors had four levels, the regression models were chosen to be polynomials of degree no larger than three. In order to perform the correct statistical analyses, the number of coefficients in the polynomials could not exceed the number of experimental points, i.e., 16. The following models were obtained for the two objective functions:
The rotating bending fatigue limit and the roughness, which depended on the radius and burnishing force in coded form, were found to be [1]:
The above equations were obtained on the basis of planned experiments and subsequent regression analyzes. Graphical visualizations of Eqs. (1)–(4) are shown in Fig. 5. Obviously, the four functions have extremum (maximum or minimum) in the governing factor space \( \varGamma \). One-objective optimization of the objective functions (1)-(4) was conducted by means of QStatLab software and involved using a genetic algorithm:
where \( \left\{ {X_{j}^{*} } \right\} \) are the vectors of the optimal values of the governing factors under the corresponding optimization criterion: maximum fatigue limit, minimal roughness, maximum surface micro-hardness and maximum depth of the hardened layer.
Figure 6 shows the optimal values of the objective functions and the corresponding optimal values of the governing factors. It is important to note that the extremums of the four objective functions are obtained for different combinations of magnitudes of the governing factors, respectively, different vectors \( \left\{ {X_{j}^{*} } \right\} \). Detailed information on the optimization is provided in Table 5.
The main objective of this study is to develop a cost-effective optimization approach for obtaining the maximum fatigue limits of diamond-burnished components using objective functions consisting of SI properties. Thus, the need to perform costly and time-consuming fatigue tests is eliminated. For this purpose, it is necessary to investigate the \( \hbox{max} Y_{{\sigma_{ - 1} }} = \hbox{max} Y_{{\sigma_{ - 1} }} \left( {\text{Ra}} \right) \), \( \hbox{max} Y_{{\sigma_{ - 1} }} = \hbox{max} Y_{{\sigma_{ - 1} }} \left( {Y_{\text{HV}} } \right) \) and \( \hbox{max} Y_{{\sigma_{ - 1} }} = \hbox{max} Y_{{\sigma_{ - 1} }} \left( {Y_{d} } \right) \) dependencies. Figure 7 shows the graphical visualizations of these functions, which were obtained via the algorithm described in Materials and Methods section. Figure 7a shows that minimizing the roughness leads to minimizing the fatigue limit. It is known that an increase in the surface micro-hardness leads an increased fatigue limit. However, Fig. 7b shows that this relationship is not linear. The rotating bending fatigue limit reaches its maximum value before the maximum value of the micro-hardness is reached. In other words, the relationship between the two functions is not synonymous. The most likely reason for this result is that the achievement of maximum micro-hardness is associated with an increase in the density of dislocations, which introduces defects in the surface layers. Figure 7c shows that reaching the maximum fatigue limit corresponds to the depth of the hardened layer, which is less than the maximum depth. Obviously, the same fatigue limit value can be achieved with a different depth of the hardened layer due to the different gradient of the micro-hardness in depth. Figure 7 shows that to achieve a fatigue limit close to the maximum, DB must be implemented with magnitudes of governing factors that simultaneously increase the roughness and reduce the surface micro-hardness and the depth of the hardened layer in comparison with their extreme values. Therefore, multi-objective optimization is necessary, as the vector of the objective functions must include \( Y_{\text{Ra}} \), \( Y_{\text{HV}} \) and \( Y_{d} \). Since the corresponding optimal values \( \left\{ {X_{\text{Ra}}^{*} } \right\} \), \( \left\{ {X_{\text{HV}}^{*} } \right\} \) and \( \left\{ {X_{d}^{*} } \right\} \) of the governing factors of these functions are to be found, the weigh vector method [1] is the most appropriate optimization method. In this case, it is necessary to find the center of gravity of three points with coordinates, respectively, \( \left\{ {X_{\text{Ra}}^{*} } \right\} \), \( \left\{ {X_{\text{HV}}^{*} } \right\} \) and \( \left\{ {X_{d}^{*} } \right\} \):
The outcomes from the multi-objective optimization are listed in Table 5. The result obtained for the rotating bending fatigue limit is 538 MPa. This result differs from the maximum fatigue limit (\( \hbox{max} Y_{{\sigma_{ - 1} }} = 540.4\;{\text{MPa}} \)) by 0.44%, which proves the effectiveness of the proposed approach.
The results obtained for the fatigue limit, shown in Table 5, can be explained by the combination of two effects (macro- and micro-effects) which DB produces. The essence of the macro-effect is the creation of compressive RS in the superficial and subsurface layers. This stress retards the formation and growth of fatigue macro-cracks and thus increases the fatigue limits of the diamond-burnished components. The micro-effect is the modification of the microstructures of the surface and subsurface layers in terms of grain refinement, homogenization and material pore reduction. Such microstructure is characterized by increased plasticity and fatigue crack resistance. The fatigue limit depends on the combination of these two effects. To evaluate the two effects, X-ray diffraction and microstructure analyses were performed in this study. For this purpose, four groups of cylindrical specimens with diameters of 20 mm and machined lengths of 30 mm (two samples in each group) were subjected to DB with the following magnitudes of the governing factors: \( \left\{ {X_{{\sigma_{ - 1} }}^{*} } \right\} \), \( \left\{ {X_{\text{Ra}}^{*} } \right\} \), \( \left\{ {X_{\text{HV}}^{*} } \right\} \) and \( \left\{ {X_{d}^{*} } \right\} \), respectively. One sample from each group was subjected to an X-ray stress analysis after DB, and the second specimen was subjected to an analysis of the microstructures of the surface and subsurface layers.
The residual axial and hoop stress distributions, obtained via X-ray stress analyses, are shown in Fig. 8. For brevity, the four specimens are denoted as follows: specimen A—burnished with \( \left\{ {X_{{\sigma_{ - 1} }}^{*} } \right\} \), specimen B—burnished with \( \left\{ {X_{\text{Ra}}^{*} } \right\} \), specimen C—burnished with \( \left\{ {X_{\text{HV}}^{*} } \right\} \) and specimen D—burnished with \( \left\{ {X_{d}^{*} } \right\} \). Both surface residual stresses (RSs) show the same trend: They are the smallest in absolute value for specimen A. The axial RS of this specimen is smaller in absolute value by 700 and 750 MPa, respectively, than the axial RS of the D and C specimens. It is important to note that specimen B, treated via smoothing DB, also has an axial RS larger in absolute value than that for specimen A. The surface hoop RSs of the three samples B, C and D are practically the same: approximately − 600 MPa, while, for specimen A, they are almost two times smaller in absolute value. It is clear that these surface RSs, both manifestations of the macro-effect, are unable to predict fatigue behavior. It can be assumed that specimens C and D are characterized by a huge gradient of the equivalent plastic strain in depth, which also predetermines such a gradient for the RS in depth. The excessive surface plastic strain is obviously the reason for the introduction of multiple surface defects via DB, which these defects subsequently reducing the fatigue limit.
Taking into account the experimental results for the fatigue limit, the graphical dependencies in Fig. 8 give rise to the following comments:
-
Although the measured surface RSs of the B, C and D specimens are significantly larger in absolute value than that of sample A, this increase in surface RS does not lead to an improvement in the fatigue limit (Table 5). To the contrary, excessively large surface RSs are the result of excessive surface plastic deformation producing local surface defects and caused by DB conducted with inappropriate magnitudes of the governing factors.
-
The presence of huge gradients of both RS in depth (up to 0.03–0.04 mm) also implies such a gradient for the physical–mechanical state of the surface and subsurface layers. Hence, there is reason to believe that high-density dislocation configurations are formed in these layers, which are the physical basis for the nucleation of micro-cracks.
-
The residual stress distribution, depicted in Fig. 8, is an initial characteristic of the stressed states of the surface and subsurface layers immediately after DB. The diamond-burnished component, subjected to a cyclic load, undergoes residual stress relaxation, whose material carrier is the evolution in the microstructures of these layers. Obviously, the residual stress relaxation rate depends on the initial residual stress distribution. On the basis of the experimental results for the fatigue limit, it can be assumed that the presence of RS values which are excessively large in absolute value and have large gradients in depth increases the residual stress relaxation rate.
The outcomes from the analyses of the microstructures are visualized in Fig. 9. In the cross section, the fibrous structure (texture) of specimen A has a depth of up to 100 μm (Fig. 9a). The depth of the refined structure is up to 15 μm, as the refinement in A is relatively coarse compared to that in the rest of specimens. In the longitudinal section (Fig. 3), granular sorbite predominates in the zone with the refined structure (Fig. 9a). For specimen B, a fibrous structure with a depth of up to 20 μm is observed in cross section (Fig. 9b). The structure is strongly refined, as the mechanical mixture is sorbite. The refinement is more pronounced compared to the refinement observed in specimen A. The structural alteration is characterized by a significant gradient in the radial direction (from fine to coarse grains), which explains the significant gradient of the observed residual stresses. In longitudinal section, the refinement of the surface layer is investigated. The depth of the structural changes is about 30 μm, and the depth of the structural alterations in the longitudinal section is about 30 μm. A refined sorbite structure is observed and more dispersed compared to that of specimen A. For specimen C, a fibrous structure with a depth of up to 80 μm is observed in cross section (Fig. 9c). Up to 25–30 μm of the fibrous structure (texture) consists of a strongly refined sorbite–troostite and gradates in ferrite–pearlite structure. The structural alterations are characterized by a significant gradient in the radial direction from fine to coarse grains, as in specimen B but down to a significantly greater depth. The depth of the structural changes in the longitudinal section is about 80 μm. Two zones are observed: Z.1—a strongly refined sorbite–troostite grainy structure up to 20 μm in depth, which is more dispersed compared to that of specimen B, and Z.2—a refined sorbite grainy structure from 20 to 80 μm in depth. For specimen D, a fibrous structure with a depth of up to 150 μm is observed in cross section (Fig. 9d). Up to 10–15 μm of the texture is troostite and strongly refined. This zone is characterized by a large gradient of change in the microstructure in depth. The transitional zone, where the texture is ferrite–sorbite with an insignificant share of the ferrite, is shown via an interrupted line. The fibers in this zone are almost equidistant from the external cylindrical surface. The depth of the structural changes in the longitudinal section is greater than 150 μm. Up to 25 μm in refined sorbite structure is observed.
On the basis of the observed microstructures of the four specimens, the following comments can be made:
-
The depth of the affected zone (zone with fibrous structure–texture) is smallest for specimen B. Simultaneously, a significant gradient in the microstructure in the radial direction (from fine to coarse grains) is observed. These characteristics of the microstructure, i.e., thin affected layer and significant gradient, are reflected in residual compressive stresses with very large surface gradients (Fig. 8), which, in turn, lead to low fatigue strength.
-
The observed alteration in the microstructure of specimen A, due to DB, is oppose that observed in specimen B. The formed texture has a depth of 100 μm, and the gradient of alteration in depth is significantly smaller. These characteristics of the microstructure produce a significantly smaller gradient of the residual axial stresses and an almost zero gradient of the residual hoop stresses. As a result, based on these two characteristics of the modified microstructure, i.e., significant depth of the affected zone and minimal gradient, the fatigue strength of specimen A is the greatest.
-
With respect to the observed microstructure characteristics, the other two specimens take on intermediate positions between specimen A and specimen B. Regardless of the significant depth of the affected zone (80–150 μm), the gradients of alteration in depth of the microstructures found in C and D are larger than that for specimen B. These characteristics lead to very large surface gradients of both axial and hoop residual stresses: For both specimens (C and D), the surface residual stresses are practically equal. As a result, the fatigue strengths of the two specimens are significantly greater than that of specimen B but smaller than that of specimen A.
4 Conclusions
The following basic conclusions can be drawn:
-
A cost-effective optimization approach for increasing the fatigue strength of diamond-burnished steel components has been developed. The basic idea is that the fatigue strength can be controlled via some of the SI characteristics (surface micro-hardness, hardened-layer depth and roughness) whose measurements are not time-consuming and expensive. Thus, a multi-objective optimization task was set and solved using the weight vector method. The rotating bending fatigue limit so obtained differs from the maximum fatigue limit by 0.44%, which proves the effectiveness of the proposed approach.
-
The results obtained for the fatigue limit are explained by means of an X-ray analysis of the introduced residual stresses and an analysis of the microstructures of the surface and subsurface layers. The presence of significant compressive residual stresses (hoop and axial), but with a smaller gradient in depth, decreases the residual stress relaxation rate and thus increases the fatigue limit. It has been established that a larger depth of the affected zone, coupled with a smaller gradient of alteration of the microstructure in depth, provides greater fatigue strength.
Abbreviations
- A 5 :
-
Elongation
- E :
-
Young’s modulus
- f :
-
Feed rate
- F b :
-
Burnishing force
- HV:
-
Micro-hardness
- r :
-
Diamond radius
- Ra :
-
Surface roughness
- s i :
-
X-ray elastic constants
- v :
-
Burnishing velocity
- x i :
-
Variable in coded form
- \( \tilde{x}_{i} \) :
-
Variable in natural form
- {X}:
-
Vector of the governing factors (variables)
- \( \left\{ {X_{j}^{*} } \right\} \) :
-
Vector of the optimal values of the governing factors (variables)
- Y i :
-
Objective function
- z t :
-
Transverse contraction
- \( \varepsilon \) :
-
Small positive number
- \( \varGamma \) :
-
Governing factor space
- \( \sigma_{ - 1} \) :
-
Fatigue limit
- \( \sigma_{0.2} \) :
-
Yield limit
- \( \sigma_{\text{u}} \) :
-
Ultimate stress
References
Maximov JT, Duncheva GV, Anchev AP, Dunchev VP (2020) Smoothing, deep or mixed diamond burnishing of low-alloy steel components—optimization procedures. Int J Adv Manuf Technol 106:1917–1929
Hassan AM, Al-Wahhab OMA (1998) Surface characteristics of some roller burnished non-ferrous components. Mater Manuf Process 13(4):505–515
Nguyen TT, Le XB (2018) Optimization of interior roller burnishing process for improving surface quality. Mater Manuf Process 33(11):1233–1241
Ovali I, Akkurt A (2011) Comparison of burnishing process with other methods of hole surface finishing processes applied on brass materials. Mater Manuf Process 26:1064–1072
Shankar E, Prabu SB, Kumar TS, Stalin John MR (2018) Investigation of TiAlN coated roller burnishing on Al-(B4C)p MMC workpiece material. Mater Manuf Process. https://doi.org/10.1080/10426914.2018.1453160
Rao DS, Hebbar HS, Komaraiah M (2007) Surface hardening of high-strength low alloy steels (HSLA) dual-phase steels by ball burnishing using factorial design. Mater Manuf Process 22:825–829
Rao DS, Hebbar HS, Komaraiah M, Kempaiah UN (2008) Investigations on the effect of ball burnishing parameters on Surface Hardness and wear resistance of HSLA dual-phase steels. Mater Manuf Process 23:295–302
Travieso-Rodriguez JA, Gomes-Gras G, Jorba-Piero J, Carrillo F, Dessein G, Alexis J, Gonzalez-Rojas HA (2015) Experimental study on the mechanical effects of the vibration-assisted ball-burnishing process. Mater Manuf Process 30(12):1490–1497
Bednarski P, Bialo D, Brostow W, Czechowski K, Polowski W, Rusek P, Tobola D (2013) Improvement of tribological properties of matrix composites by means of slide burnishing. Mater Sci 19(4):367–372
Buldum BB, Cagan SC (2018) Study of ball burnishing process on the surface roughness and microhardness of AZ91D alloy. Exp Tech 42(2):233–241
Czechowski K, Tobola D (2017) Slide finishing burnishing of metal alloys and metal matrix composites. Mechanik NR 7:1–3
Gharbi F, Sghaier S, Hamdi H, Benameur T (2012) Ductility improvement of aluminum 1050A rolled sheet by a newly designed ball burnishing tool device. Int J Adv Manuf Technol 60(1–4):87–99
Luo H, Liu J, Wang L, Zhong Q (2006) The effect of burnishing parameters on burnishing force and surface microhardness. Int J Adv Manuf Technol 28(7–8):707–713
Maximov JT, Anchev AP, Duncheva GV, Ganev N, Selimov KF (2017) Influence of the process parameters on the surface roughness, micro-hardness, and residual stresses in slide burnishing of high-strength aluminum alloys. J Braz Soc Mech Sci Eng 39(8):3067–3078
Tanaka H, Ishii W, Yanagi K (2011) Optimal burnishing conditions and mechanical properties of surface layer by surface modification effect induced of applying burnishing process to stainless steel and aluminum alloy. J Jpn Soc Technol Plast 52(605):726–730 (in Japanese)
Teimouri R, Amini S, Bami AB (2018) Evaluation of optimized surface properties and residual stress in ultrasonic assisted ball burnishing of AA6061-T6. Measurement 116:129–139
Boguslaev VA, Yatsenko VK, Yakovlev VG, Stepanova LP, Pukhalskaya GV (2008) The effect of diamond burnishing on structure and properties of detonation-gas coatings on gas-turbine engine parts. Metal Sci Heat Treat 50(1–2):44–48
Brostow W, Czechowski K, Polowski W, Rusek P, Tobola D, Wronska I (2013) Slide diamond burnishing of tool steels with adhesive coatings and diffusion layers. Mater Res Innov 17(4):269–277
Hamadache H, Laouar L, Zeghib NE, Chaoui K (2006) Characteristics of Rb40 steel superficial layer under ball and roller burnishing. J Mater Process Technol 180(1–3):130–136
Hamadache H, Zemouri Z, Laouar L, Dominiak S (2014) Improvement of surface conditions of 36CrNiMo6 steel by ball burnishing process. J Mech Sci Technol 28(4):1491–1498
Huuki J, Laakso SVA (2013) Integrity of surface finished with ultrasonic burnishing. Proc IMechE Part B J Eng Manuf 227(1):45–53
Korzynski M, Lubas J, Swirad S, Dudek K (2011) Surface layer characteristics due to slide diamond burnishing with a cylindrical-ended tool. J Mater Process Technol 211:84–94
Lobanowski J, Ossowska A (2006) Influence of burnishing on stress corrosion cracking susceptibility of duplex steel. J Achiev Mater Manuf Eng 19(1):46–52
Maximov JT, Duncheva GV, Anchev AP, Ganev N, Amudjev IM, Dunchev VP (2018) Effect of slide burnishing method on the surface integrity of AISI 316Ti chromium-nickel steel. J Braz Soc Mech Sci Eng 40:194. https://doi.org/10.1007/s40430-018-1135-3
Sachin B, Narendranath S, Chakradhar D (2018) Effect of cryogenic diamond burnishing on residual stress and microhardness of 17–4 PH stainless steel. Mater Today 5(9):18393–18399
Sachin B, Narendranath S, Chakradhar D (2018) Experimental evaluation of diamond burnishing for sustainable manufacturing. Mater Res Express 5(10):106514
Saldana-Robles A, Plascencia-Mora H, Aguilera-Gomez E, Saldana-Robles A, Marquez-Herrera A, Diosdado-De la Pena JA (2018) Influence of ball-burnishing on roughness, hardness and corrosion resistance of AISI 1045 steel. Surf Coat Technol 339:191–198
Aliev KT, Aslanov TI (1979) The influence of diamond burnishing on the fatigue strength and wear resistance of the shafts of petroleum chains. Chem Petrol Eng 15(6):459–461
Korzynski M, Pacana A, Cwanek J (2009) Fatigue strength of chromium coated elements and possibility of its improvement with slide diamond burnishing. Surf Coat Technol 203:1670–1676
Maximov JT, Duncheva GV, Anchev AP, Dunchev VP (2019) Crack resistance enhancement of joint bar holes by slide diamond burnishing using new tool equipment. Int J Adv Manuf Technol 102:3151–3164
Maximov JT, Duncheva GV, Anchev AP, Ganev N, Dunchev VP (2019) Effect of cyclic loading on fatigue performance of slide burnishing components made of low-alloy medium carbon steel. Fatigue Fract Eng Mater Struct 42(6):1414–1425
Swirad S (2007) The effect of burnishing parameters on steel fatigue strength. Nonconventional Technol Rev 1:113–118
Maximov JT, Duncheva GV, Anchev AP, Dunchev VP, Ichkova MD (2020) Improvement in fatigue strength of 41Cr4 steel through slide diamond burnishing. J Braz Soc Mech Sci Eng 42:197. https://doi.org/10.1007/s40430-020-02276-8
Vuchkov IN, Vuchkov II (2009) QStatLab Professional, V. 5.5 – Statistical Quality Control Software. User’s Manuel, Sofia
Acknowledgment
This work was supported by the European Regional Development Fund within the OP “Science and Education for Smart Growth 2014-2020,” Project CoC “Smart Mechatronics, Eco- and Energy Saving Systems and Technologies,” No. BG05M2OP001-1.002-0023.
Author information
Authors and Affiliations
Corresponding author
Additional information
Technical Editor: Izabel Fernanda Machado.
Publisher's Note
Springer Nature remains neutral with regard to jurisdictional claims in published maps and institutional affiliations.
Rights and permissions
About this article
Cite this article
Maximov, J.T., Duncheva, G.V., Anchev, A.P. et al. A cost-effective optimization approach for improving the fatigue strength of diamond-burnished steel components. J Braz. Soc. Mech. Sci. Eng. 43, 33 (2021). https://doi.org/10.1007/s40430-020-02723-6
Received:
Accepted:
Published:
DOI: https://doi.org/10.1007/s40430-020-02723-6