Abstract
Background
Anxiety disorders (AD) are common mental disorders, for which several cost-of-illness (COI) studies have been conducted in the past.
Objective
The aim of this review was to provide a systematic overview of these studies and an aggregation of their results.
Methods
A systematic literature search limited to studies published after 1999 was conducted in PubMed/MEDLINE in November 2018. We included top-down COI studies reporting costs for AD, and bottom-up COI studies reporting costs for AD and a non-diseased control group, and extracted data manually. Results of the top-down COI studies were aggregated by calculating the mean percentage of costs on gross domestic product (GDP) and health expenditure, while the results of the bottom-up studies were analyzed meta-analytically using the ‘ratio of means’ method and inverse-variance pooling. In this review, the logarithm of the relative difference in a continuous outcome between two groups is calculated and aggregated over the studies. The results can be interpreted as the relative change in costs imposed by a specific disease compared with baseline costs.
Results
We identified 13 top-down and 11 bottom-up COI studies. All top-down COI studies and four bottom-up COI studies reported costs for AD as a diagnostic group, four for generalized anxiety disorder (GAD), four for social anxiety disorder (SAD), and one for panic disorder. In top-down COI studies, direct costs of AD, on average, corresponded to 2.08% of health care costs and 0.22% of GDP, whereas indirect costs, on average, corresponded to 0.23% of GDP. In bottom-up COI studies, direct costs of patients with AD were increased by factor 2.17 (1.29–3.67; p = 0.004) and indirect costs were increased by factor 1.92 (1.05–3.53; p = 0.04), whereas total costs increased by factor 2.52 (1.73–3.68; p < 0.001). Subgroup analysis revealed an increase in direct costs by 1.60 (1.16–2.22; p = 0.005) for SAD and 2.60 (2.01–3.36; p < 0.001) for GAD. Measures of heterogeneity indicated high heterogeneity when pooling studies for direct costs, indirect costs, and total costs, but low to moderate heterogeneity when pooling studies for SAD or GAD.
Conclusions
Using methods that focused on relative rather than absolute costs, we were able to aggregate costs reported in different COI studies for ADs. We found that ADs were associated with a low proportion of health care costs on a population level, but significantly increased health care costs on an individual level compared with healthy controls. Our disorder-specific subgroup analysis showed that study findings are most homogeneous within specific ADs. Therefore, to get a more detailed picture of the costs of ADs, more studies for currently under researched ADs, such as panic disorder, are needed.
Similar content being viewed by others
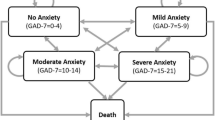
Explore related subjects
Discover the latest articles, news and stories from top researchers in related subjects.Avoid common mistakes on your manuscript.
Anxiety disorders (ADs) are associated with a small share of health expenditures and gross domestic product. |
Health care costs in patients with acute ADs are twice as much as in individuals without ADs. |
Future studies should provide more bottom-up research comparing costs for patients with specific ADs with non-diseased comparator groups. |
1 Introduction
Anxiety disorders (AD) are a group of mental disorders characterized by anxiety and fear. The most common specific ADs are generalized anxiety disorder (GAD), panic disorder (PD), social anxiety disorder (SAD), agoraphobia (AP) and specific phobias (SPs). In short, GAD is characterized by long-lasting anxiety not associated with specific objects or situations; PD is characterized by attacks of intense panic lasting minutes to hours that are associated with strong physical symptoms such as trembling, confusion, difficulty breathing, and others; SAD is characterized by fear of public embarrassment or social interaction; AP constitutes a specific fear of being in places from which one cannot easily escape; and SPs are characterized by fear that is triggered by specific stimuli or situations such as, for example, flying, heights, or spiders.
ADs are one of the leading causes of disease burden worldwide. According to the global burden of disease study, approximately 275 million individuals are affected by AD, and approximately 42 million incident cases occur per year worldwide [1]. These numbers correspond to approximately 26 million years lived with disability, the ninth highest value among all diseases worldwide and the seventh highest value for high-income countries. In a large review on mental disorders in Europe, Wittchen et al. [2] estimated a prevalence rate of 14.0% for ADs, which was by far the highest prevalence rate of all mental disorders. ADs affect women approximately twofold more often than men and tend to become chronic [3]. The age of onset starts in childhood, with incidence rates then increasing over adolescence and early adulthood, reaching a peak in middle age, and then decreasing again [3].
A large number of studies have investigated the economic impact of ADs, and contain two major categories of costs—direct costs and indirect costs. Direct costs encompass all monetarily valued medical and non-medical resources consumed in consequence of a disease, whereas indirect costs encompass the monetarily valued loss of productivity due to a disease. Two different types of studies estimating the economic impact of a disease must be distinguished according to the methodological approach used. Top-down cost-of-illness (COI) studies mostly analyze the costs of ADs as a group of diseases, for a specific country. In particular, the groups investigating the cost of mental disorders of the brain have conducted several such studies for different European countries [4,5,6,7,8,9,10,11]. On the other hand, bottom-up COI studies mostly focus on one single AD, such as GAD or PD.
From the viewpoint of a systematic literature review, the biggest challenge lies in the aggregation of COI data due to the heterogeneity between COI studies. Results of top-down COI studies strongly depend on the size of the underlying populations, differences in health care systems regarding access to health care, the treatments applied (e.g. psychotherapy vs. pharmaceuticals; inpatient treatment vs. outpatient treatment), and of course prices of the treatments applied. For example, the prices of identical pharmaceuticals often strongly differ between countries. In bottom-up COI studies, additional substantial heterogeneity is introduced by an inconsistent consideration of cost categories, in particular regarding indirect costs. There are several reasons for this problem. First, bottom-up COI studies often address research questions that refer to specific cost categories, for example the relationship between adherence to drug use and drug costs. Second, other than top-down studies, which are almost always conducted from a societal perspective, bottom-up COI studies are often conducted from a more limited perspective, for example a payers perspective, which limits the consideration of cost categories to costs relevant to the regarded payer. Third, because bottom-up COI studies are often conducted alongside clinical studies with very different research questions, study populations of bottom-up COI studies can differ substantially, which can also affect the consideration of cost categories. For example, a clinical study focusing on severely ill patients will have to consider inpatient costs, whereas a clinical study focusing on preclinical disease stages might be restricted to the analysis of outpatient costs. Although all of these reasons are legitimate consequences of differences in research questions, they pose a considerable problem for the aggregation of evidence.
A further important issue with bottom-up COI studies is the skewed distribution of cost data, which leads to a high relevance of individuals with very high costs. In particular, if bottom-up COI studies have low sample sizes, the resulting mean costs can be considerably influenced by only one or two persons, with very high costs. This issue is of particular interest since bottom-up COI studies are often conducted alongside clinical trials with smaller samples, compared with population surveys. Sample sizes of clinical trials are often based on power calculations, which use assumptions on distributions with smaller a priori probabilities of extreme values than the skewed distributions found in cost data. In consequence, bottom-up COI studies are often underpowered regarding the samples sizes actually needed to analyze cost data. Therefore, aggregation of the results over several bottom-up COI studies is desirable in order to increase the reliability of the evidence provided. Such studies have rarely been conducted until now. One specific limitation of most bottom-up COI studies preventing meta-analytic approaches is the absence of a comparator. Meta-analytic approaches target the aggregation of an ‘effect’, i.e. a difference between groups. In terms of COI studies, such an effect might be the difference in costs between a study population with and a study population without an AD. COI studies presenting not only the costs of patients with AD but also a healthy comparator population are relatively rare but are increasingly available.
To deal with the problems connected to the aggregation of absolute cost estimates from different COI studies, our analytic strategy will focus on generating relative cost estimates based on the assumption that these are more comparable between COI studies than absolute estimates. Therefore, this review does not focus on absolute top-down costs, but rather on the relative proportion of these costs on country-specific gross domestic product (GDP) and total health expenditures (HE). Furthermore, this review focuses on bottom-up COI studies that present cost data for patients, together with cost data of a healthy comparator and use of the ratio of means (ROM) as a measure of effect to be compared and aggregated over studies.
2 Methods
2.1 Search Strategy
We conducted a literature search of the Pubmed database (last updated 9 November 2018), limited to studies that were published from the year 2000 on. In addition, we searched the reference lists of eligible studies, as well as systematic or narrative reviews relating to ADs in an economic context. To identify relevant studies, the search string ((‘anxiety disorder’ or ‘anxiety disorders’ or ‘separation anxiety disorder’ or ‘selective mutism’ or phobia* or phobias or ‘social anxiety disorder’ or ‘panic disorder’ or agoraphobia or ‘generalized anxiety disorder’ or ‘induced anxiety’) and (cost or costs or economic* or expenditure* or financial* or spending*)) was used.
Studies were included if they reported costs either for a specific AD or for AD as a diagnostic group. For bottom-up studies, we defined ADs according to the current classification of ADs provided in the Diagnostic and Statistical Manual of Mental Disorders, 4th Edition (DSM-IV). Top-down studies reporting costs for ADs as a diagnostic group were also included if their definition of ADs included obsessive compulsive disorder or post-traumatic stress disorder. The reason for this procedure is that these two disorders have been defined as ADs in former versions of the DSM, but not in the current version. Bottom-up COI studies were included if they reported mean costs for an adult patient group with ADs and a control group not affected by ADs (only reporting excess costs, i.e. the difference between both groups was not sufficient), as well as the sample sizes of both groups.
Data for extraction were defined based on our former experience in conducting systematic reviews on COI studies, and covered the following clusters of information: ‘general study characteristics’ (type of AD considered and country; for bottom-up COI studies: diagnostic criteria, sample sizes, type of data and study population); ‘cost-related information’ (cost categories considered, year of costing, currency); ‘costs’ (top-down COI studies: direct, indirect, and total costs; bottom-up COI studies: mean and reported measure of variance of direct, indirect, and total costs).
2.2 Analysis
2.2.1 Top-Down Studies
We defined a ‘top-down’ study as every study reporting total costs on a population level, for a specific country or group of countries, irrespective of the specific method used to derive these costs. For every top-down study, country-specific data on GDP and HE corresponding to the years for which AD costs were reported, were gained from the OECD health statistics [12]. The OECD data on HE cover a broad range of costs across different financing schemes (e.g. governmental, insurance, private households), different functions (e.g. preventive, curative, long-term care, administration), as well as providers (e.g. inpatient, outpatient, non-medical). Therefore, all direct costs covered by top-down studies (including non-medical costs) should be covered by the HE data provided by the OECD. Using these data, the share of direct AD costs on HE (as a percentage), and the share of direct, indirect, and total costs on GDP (as a percentage) were calculated. This procedure avoided the necessity of adjusting costs for inflation or purchasing power differences. Finally, the cross-study means of these percentages were calculated as aggregated outcomes for top-down COI studies. We present unweighted means, as well as means weighted by population, to account for the differences in population size. Data on population size were taken from the OECD health statistics [12].
2.2.2 Bottom-Up Studies
We defined a ‘bottom-up’ study as every study that was based on a sample of persons, and reported average costs per person based on ‘measured’ data (i.e. we excluded, for example, studies that reported average costs per person, but were based on modeling approaches). Bottom-up COI studies were analyzed meta-analytically using the ROM method [13, 14]. The ROM is a meta-analytical effect measure for continuous outcome variables for which the log of the ROM between an intervention and a control group is calculated and aggregated over studies. The ROM can be easily interpreted as the percentage difference in outcome (i.e. costs) between the intervention and control groups (or, in our case, between an AD and a comparator group). The ROM provides one major advantage against other meta-analytical approaches for the aggregation of health care cost data. By relying on the relative difference between two samples instead of absolute differences, this method allows pooling studies with different outcome units, which is of particular interest when pooling cost data. Although there is a more or less clear definition, for example for direct costs, usually the only aspect direct costs reported in two different COI studies have in common is being called ‘direct costs’. As long as two different COI studies do not refer to, at least, the same health care system, and use the same measurement instruments, cost categories, and unit prices, the absolute costs of these two COI studies are not comparable to the extent necessary for meaningful pooling. In that sense, the frequent observation that absolute cost values reported in different disease-specific COI studies show cross-study variation up to several orders of magnitude, can, at least partly, be ascribed to heterogeneity in the outcome units reported. Using the ROM allows aggregating cost data from different COI studies by circumventing this heterogeneity.
First, the ROM of costs between individuals with and without AD was calculated and meta-analytically aggregated by generic inverse variance weighting. For this purpose, the natural logarithm of the ROM and its standard error are calculated based on the sample sizes, mean costs, and standard deviations reported in the studies analyzed. If no measure of variance was available for a study, we imputed a standard error from another study belonging to the same subgroup of pooled studies. To be conservative, we always used the highest value that was available. Since heterogeneity between studies had to be expected, a random-effects model was chosen. Heterogeneity was assessed using the I2 statistic (cut-offs values: I2 = 25%, I2 = 50%, and I2 = 75%, indicating low, moderate, high, and very high heterogeneity).
Meta-analyses were conducted for different subgroups of studies. Primary subgroup analyses were ‘direct costs’, ‘indirect costs’, and ‘total costs’, irrespective of the underlying disease. In two secondary subgroup analyses, direct costs for SAD and GAD were analyzed.
To analyze the influence of specific study characteristics on the ROM, we computed a random-effects meta-regression. The following potential effect modifiers were analyzed: ADs (GAD, PD, SAD [ref: ADs]), comparator group (cohort study or clinical trial, primary disease [ref: general population]), mean age in the anxiety group, data source (primary data or medical records [ref: claims data]), and inclusion of cost categories (inpatient costs, drug costs, nonmedical costs). Outpatient costs were not assessed due to collinearity (all studies investigated included outpatient costs). First, we conducted univariate analyses with each predefined potential effect modifier. In a second step, we included all variables for which we found a significant association in step one, in a multivariate analysis.
3 Results
Details of the search results are shown in Fig. 1. A literature search identified 2677 records, of which 184 were assessed for eligibility in full text. A total of 161 studies were excluded for different reasons, and one study was added from a reference list search, resulting in a final sample of 24 studies included in the review. Of the 24 studies included, 13 were top-down [4,5,6,7,8,9,10,11, 15,16,17,18,19] and 11 were bottom-up [20,21,22,23,24,25,26,27,28,29,30] COI studies, published between 1996 and 2017 (Table 1). A majority of 14 studies was conducted in Europe, 7 in North-America, 2 in Asia, and 1 in Australia. All top-down studies reported costs for ADs as a diagnostic group, except for Maercker et al. [6], who also reported costs for single ADs. Four bottom-up studies analyzed the costs of SAD [21, 22, 25, 26], four studies analyzed the costs of GAD [24, 27, 29, 30], and one study analyzed the costs of PD [26], whereas four studies analyzed the costs for ADs as a diagnostic group [20, 23, 28, 30].
The diagnostic criteria in eight bottom-up studies [20,21,22,23,24,25, 27, 30] belonged to either the DSM or International Classification of Diseases (ICD) classification systems (Table 2). In bottom-up studies, the sample sizes of persons affected by ADs ranged from 15 to 3669. Eight bottom-up studies [21, 22, 25,26,27,28,29,30] used primary data, compared with three bottom-up studies [20, 23, 24] that used either claims data or hospital records data. Three studies [21, 25, 29] recruited patients as well as controls in the general population, and one study [30] recruited patients in the general population aged 65+ years, whereas the remaining seven studies [20, 22,23,24, 26,27,28] used different types of populations (e.g. patients with specific primary diseases, cohorts recruited in hospitals).
3.1 Top-Down Studies
Top-down studies estimated direct costs associated with ADs to be, on average, 2.08% of health expenditures, with a between-study range of 0.12–5.50% (Table 3). This share corresponded to, on average, 0.22% of GDP (range 0.01–0.62%). Estimated indirect costs corresponded to, on average, 0.23% of GDP, ranging from 0.09 to 0.59% of GDP. Weighted means were slightly higher than unweighted means for all analyzed cost categories. If the percentage of direct costs on health expenditures is applied to the 2016 OECD health expenditures of all OECD countries (US$6504 billion purchasing power parities [PPP] of total health expenditures), health expenditures attributable to ADs of approximately US$135 billion PPP result. Applied to the GDP of all OECD countries in 2016 (US$54,061 billion PPP), the estimated shares corresponded to approximately US$118 billion PPP of direct costs and US$122 billion PPP of indirect costs. Here, we see a difference between the loss in GDP and the loss in health expenditures. This difference might, at least in part, be due to non-medical costs, which are assessed in the studies and summed up under direct costs. On the other hand, the estimates of ‘health expenditures’ in the OECD health statistics are without non-medical costs, which leads to an overestimation of the share of direct costs on health expenditures, compared with the share of direct costs on GDP.
Only four studies [5, 6, 15, 17] reported non-medical direct costs at a share of up to < 1% of direct costs. Further sub-cost categories were only reported by DuPont et al. [15] and Greenberg et al. [16]. In the study by DuPont et al., approximately 50% of direct costs were for nursing homes, followed by 19% for mental health organizations, 11% for drugs, 8% for outpatient services, and 4% for short hospital stays, while in the study by Greenberg et al., 62% of direct costs were for non-psychiatric treatment, 36% for psychiatric treatment, and 2% for drugs.
3.2 Bottom-Up Studies
The results of meta-analysis of bottom-up studies are shown in Figs. 2, 3, 4, 5 and 6. As can be seen, the presence of ADs was associated with a statistically significant ROM for total costs of 2.52 (CI 1.73–3.68). The ROM for direct costs was 2.17 (CI 1.29–3.67) and 1.92 (CI 1.05–3.53) for indirect costs. We also conducted subgroup analyses for SAD and GAD, which showed clear differences. Whereas SAD was associated with a ROM of 1.60 (CI 1.16–2.22), GAD was associated with a much higher ROM of 2.60 (CI 2.01–3.36).
Analysis of heterogeneity reflected by I2 revealed that studies that refer to the same AD (Figs. 5 and 6) were relatively homogeneous, indicated by I2 values of below 50%. In contrast, pooling studies over different ADs (Figs. 2, 3, 4) showed moderate heterogeneity for indirect and total costs, and high heterogeneity for direct costs. It was striking that only 6 of 11 studies reporting results for direct costs showed ROMs with confidence intervals not including 1. Furthermore, in the study by Rees et al. [26], the pooled ROM and heterogeneity for direct costs were strongly affected by the results for panic disorder (ROM 11.30). If this one estimate is excluded, the pooled ROM for direct costs drops from 2.17 to 1.79 (CI 1.33–2.42) and I2 drops from 94% to 74%, which is considerably lower but still indicates high heterogeneity. Since only Rees et al. [26] published excess costs for panic disorder, it remains unclear if the ROM resulting from their findings represents a realistic estimate of the increase in costs associated with panic disorder or a statistical outlier.
Five studies [22, 23, 25, 26, 29] reported details on single cost categories with significance testing for six excess cost calculations (Rees et al. reported excess costs for two different ADs). All six excess cost calculations included the outpatient sector, of which five were statistically significant. Only four studies reported excess costs for the inpatient sector, of which only one was statistically significant. Two studies reported excess costs for drugs, both of which were statistically significant. There was no clear cross-study pattern on the order of these three cost sectors in terms of share on total excess costs.
In univariate analyses (see Table 4), the influence of the following effect modifiers on the ROM was statistically significant: inpatient costs, GAD, PD, and cohort study/clinical trial. Including inpatient costs in the total costs was associated with a lower relative ROM. GAD and PD were associated with an increase in the ROM compared with the group with no distinction for specific ADs. The cohort study/clinical trial data source was associated with a larger ROM compared with the general population/employees data source. In the multivariate analysis, only the estimate for panic disorder remained statistically significant and might explain the heterogeneity between the study results.
4 Discussion
The aim of this systematic review was to provide an overview of the international literature on the costs of ADs and to aggregate the evidence as much as possible. We found enough evidence for both top-down and bottom-up studies to achieve this goal. With ROM pooling, we identified an approach that can be used for the meta-analytic aggregation of bottom-up COI studies, which is a clear research gap in the literature of COI reviews to date. Given the importance of meta-analysis for the generation of the highest levels of evidence, closing this gap seems to be an important step forward, which we hoped to foster with this work.
Overall, ADs seem to cause relevant health care costs, accompanied by indirect costs of similar magnitude. On a per-person level, ADs cost less than one-tenth of the costs of psychotic disorders and dementia, and only approximately one-quarter of the costs of mood disorders or addiction [5]. On a population level, AD costs are much more relevant because of their high prevalence, yet mood disorders, dementia, and psychotic disorders remain slightly more costly [5]. In the reported data, direct AD-attributable costs resulted almost only from medical costs, which occur for drugs as well as in the outpatient and inpatient sectors, although only the excess costs of drugs and outpatient visits clearly achieve statistical significance. However, due to the distributional pattern of inpatient cost data (a very large proportion of zero utilization), insignificance might be a consequence of a lack of statistical power. The very low magnitude of non-medical direct costs, might, at least in part, be a result of a measurement error, since non-medical costs are often underreported in COI studies, even in those that claim a societal perspective [31]. There are also further costs, such as, for example, costs for family members, that might be relevant for a full societal perspective but have not been considered in COI studies for ADs to date.
Beyond health care costs, indirect costs due to lost productivity play an important role, particularly from a societal perspective. The results of top-down studies show that overall indirect costs are of a similar magnitude than direct costs. Other than top-down COI studies, which all included indirect costs, only 5 of 11 bottom-up studies reported indirect costs that could be pooled. The resulting pooled estimate was of a similar magnitude than the estimate for direct costs, which is in line with the findings from the top-down studies. Similar to direct costs, heterogeneity was also considerable for indirect costs, for different reasons. For example, access to income compensation and/or effective treatment can affect the amount of work absence and, subsequently, indirect costs.
4.1 Methodology
To avoid problems connected to the comparison of absolute costs reported in top-down COI studies, we expressed the economic burden reported in top-down COI studies as the relative impact of ADs on the health care system and on the total economy at the time the studies were conducted. In doing so, transformations of reported cost values to adjust for differences in time as well as currencies and purchasing power were no longer necessary and respective uncertainties were avoided. In addition, this approach automatically adjusts for the population size of the relative countries.
Several aspects regarding ROM pooling need to be mentioned. First, the ROM only works for COI studies reporting mean costs for a group of individuals affected by a disease, together with a non-diseased comparator group. On the one hand, this precondition reduces the number of eligible studies. Most bottom-up COI studies are conducted without a non-diseased comparison group. In this review, for example, 29 studies, or three-quarters of all identified bottom-up COI studies, had to be excluded for not having a non-diseased comparator. On the other hand, focusing a literature review on the relative cost difference between a disease group and a comparator group, rather than absolute costs, considerably reduces problems connected to the analyses of absolute costs in diseased-only populations. First, problems related to between-study differences in prices, e.g. regarding different national price levels, unit cost prices, and intertemporal price differences due to inflation, are strongly reduced, if not eliminated. Second, between-study differences in the consideration of cost categories, a major source for between-study variation of absolute costs, should also lose relevance. This argument depends on the degree of homogeneity in the utilization of health care between different cost categories. If the extent to which health care utilization is increased by a disease is very similar across different cost categories, then heterogeneity in the consideration of cost categories should be of minor relevance if relative cost differences are analyzed. If, on the other hand, different cost categories are very unequally affected by a disease, then heterogeneity in the consideration of cost categories will still be very relevant, even if relative cost differences are analyzed. Third, focusing on relative differences between two study populations opens the possibility of meta-analytic aggregation of study results in a systematic review, which constitutes a more valuable review outcome than the presentation of single-study results.
There are also limitations for the application of this meta-analytic approach to COI studies. First, the heterogeneity indicators indicated higher heterogeneity for the pooling of direct costs, indirect costs, and total costs (costs were pooled across different ADs), as for the disease-specific pooling of social phobia and GAD. This indicates that there are relevant differences between AD subtypes that result in different costs of ADs for which the ROM cannot compensate, a finding one would expect. Our findings indicate that SAD seems to be a rather low-cost AD, whereas GAD tends to be rather expensive.
Second, the ROM cannot compensate for the variation in mean costs resulting from the skewed distribution of costs within studies, as well as differences in health care systems (e.g. number of psychotherapeutic sessions usually applied to treat ADs).
Third, all included bottom-up studies referred to adults. There have been studies analyzing economic aspects or costs of AD in children or adolescents, but these have not met the inclusion criteria for our review, in particular the need for a healthy comparator group. This presents a limitation since our estimates are most likely not transferable to children or adolescents, for two reasons. First, baseline costs will not be comparable because morbidity in children and adolescents differs from morbidity in adults in regard to quantity and disease spectrum. Second, the treatment of ADs (and the respective treatment costs) in children or adolescents might also differ from adults.
4.2 Study Comparability
From a methodological perspective, top-down COI studies were relatively homogeneous. Nine of 11 top-down COI studies were part of the so-called ‘costs of disorders of the brain in Europe’ study. Methodological approaches in general, and costing methods in particular, as well as data used from them, come with a homogeneity rarely seen among top-down COI studies conducted for a specific group of diseases.
Given the methodological homogeneity of top-down COI studies, it should not surprise that, with one exception (Sado et al. [17]), the results reported in top-down COI studies were also relatively homogenous. Sado et al. [17], who reported AD costs for Japan, found a considerably lower percentage of direct costs on health care expenditures and GDP than any other study, and, as might be expected as a consequence of this undertreatment expressed in very low direct costs, relatively high indirect costs. This divergence from the other studies might point to cultural differences regarding the treatment of ADs and respective cultural sensitivity of the cost estimates.
All top-down COI studies analyzed ADs as a disease group, although the diseases covered under this umbrella differed slightly. One must note that most of these top-down COI studies, which explicitly reported the ADs included, included post-traumatic stress disorder and obsessive compulsive disorders in their analysis following the AD definition provided in the DSM-III or DSM-IV. This changed as the DSM-V became the new standard of disease classification after 2013, since the DSM-V does not classify post-traumatic stress disorder and obsessive compulsive disorders as ADs. For at least the study by Sado et al. [17], which used the ICD-10 classification, these two disorders were definitely not included in the analysis. For a substantial proportion of European studies, this issue remains unclear, since the term ‘anxiety disorder’ was not specified in more detail. All top-down COI studies assessed all major cost categories (i.e. inpatient, outpatient, drug, non-medical and indirect costs), although there might be large differences of service inclusion within each major category, as well as monetary valuation of services.
Bottom-up COI studies were much more heterogeneous than top-down COI studies. First, bottom-up COI studies mostly referred to one specific AD. Second, the inclusion of cost categories showed much variation. Whereas outpatient costs were considered by all bottom-up COI studies, all other cost categories were, in part, missed, in particular non-medical and indirect costs. Further heterogeneity was based on differences in the underlying populations. Only three bottom-up COI studies used comparable populations in the sense that both groups (diseased and non-diseased) were taken from general population samples. The remaining study populations were rather unique, either because of the recruitment setting (e.g. specific medical institution, specific employer, clinical trial, etc.) or because of specific inclusion criteria (e.g. being at least 65 years of age or having a specific somatic disease). These differences can have a substantial impact on the ROM. For example, one might expect that analyzing older persons or persons with an underlying primary disease will result in a lower ROM than analyzing a general population cohort because of higher baseline costs. On the contrary, if an AD is analyzed as a comorbidity of a somatic disease, it is also possible that the AD interacts with the somatic disease, which might result in additional excess costs due to the worsened prognosis of the somatic disease. Another aspect is that if patients are recruited alongside a clinical trial, the diseased group might be more severely diseased than the average patient with the specific disease, resulting in a higher ROM. To get more insight into these aspects, we conducted a meta-regression including different variables that represent study characteristics for which we expected might influence the ROM. In univariate analyses, several variables were statistically significantly associated with the ROM; however, when analyzed in a multivariate regression, only one of these variables remained statistically significantly associated with the ROM. Here, one has to note that our sample size of 11 studies was quite low for meta-regression. Ignoring significance, the regression estimates show that PD in particular strongly increases the ROM, whereas SAD decreases the ROM compared with the average AD. Regarding sample populations, the estimates strengthen the hypothesis that using cohort or clinical trial data results in a higher ROM than in the general population, whereas the use of a sample with an underlying somatic disease reduces the ROM.
4.3 Study Generalizability
The generalizability of clinical studies, often underlying bottom-up COI studies, should be questioned because patients recruited into clinical trials are seldom representative of the total population of individuals affected by a disease, particularly if the underlying disease tends to be chronic. Since the results of top-down COI studies represent the costs for the entire diseased population, a comparison of the bottom-up findings with top-down findings can help to judge the generalizability of bottom-up costs. Based on a 12-month prevalence of ADs of 10%, a 2.17-fold increase in direct costs due to ADs, would correspond to a share of 10.5% of total health expenditures attributable to ADs [10%*(2.17−1) / (10%*2.17 + 90%) = 10.5%]. This portion is 5.6 times the average portion of direct costs for ADs on total health expenditures estimated in top-down COI studies, indicating that the cost estimates derived in bottom-up studies overestimated the true population mean of direct costs due to ADs. This phenomenon, resulting from including over-proportionally ill individuals into clinical studies, is well known and is based on the fact that, for practical reasons, study participants are often recruited when they are in contact with the health care system and are therefore, on average, in a more severe (or acute) disease stage than the average diseased person who does not seek contact with the health care system. Consequently, our pooled ROM estimate should be regarded as ‘for acute disease only’. On the other hand, top-down COI studies might underestimate the true costs of AD due to limitations in the assessment of costs that are not directly related to AD treatment, but rather indirectly ‘induced’ by ADs (e.g. somatic health care utilization due to physical conditions resulting from ADs). Whereas such costs can be well measured by bottom-up COI studies comparing patients with healthy controls, it might be challenging to fully assess such costs in a top-down design. In conclusion, our estimates should be regarded as lower and upper boundaries of a range within which the true estimate will most likely be.
5 Conclusions
We found that ADs are associated with a low proportion of health care costs on a population level, but a significant increase in health care costs when measured on an individual level. Our disorder-specific subgroup analysis showed that study findings are most homogeneous within specific ADs. Therefore, to get a more detailed picture of the costs of ADs, more studies for currently under researched ADs such as panic disorder are needed. With the ROM, we found a way to aggregate the results of bottom-up COI studies, which, to date, seems to be a rare but promising way to generate aggregated cross-study evidence in COI research. Although a relatively large number of bottom-up and top-down COI studies on ADs exist, the quantification of the true costs of AD remains a challenging effort since a combination of methodological differences between studies with general differences in health care utilization and pricing between countries generates large between-study heterogeneity. It remains a task for future research to implement more standardized methods for conducting COI studies to reduce this heterogeneity and increase cross-country, and even within country, comparability of COI studies.
Data Availability Statement
Data underlying the analysis of this study were either extracted from the included studies or taken from the OECD Health Data database. The authors do not have proprietary rights on any of these data.
References
GBD 2016 Disease and Injury Incidence and Prevalence Collaborators. Global, regional, and national incidence, prevalence, and years lived with disability for 328 diseases and injuries for 195 countries, 1990–2016: a systematic analysis for the Global Burden of Disease Study 2016. Lancet. 2017;390(10100):1211–1259.
Wittchen HU, et al. The size and burden of mental disorders and other disorders of the brain in Europe 2010. Eur Neuropsychopharmacol. 2011;21(9):655–79.
Bandelow B, Michaelis S. Epidemiology of anxiety disorders in the 21st century. Dial Clin Neurosci. 2015;17(3):327–35.
Fineberg NA, et al. The size, burden and cost of disorders of the brain in the UK. J Psychopharmacol. 2013;27(9):761–70.
Gustavsson A, et al. Cost of disorders of the brain in Europe 2010. Eur Neuropsychopharmacol. 2011;21(10):718–79.
Maercker A, et al. The costs of disorders of the brain in Switzerland: an update from the European Brain Council Study for 2010. Swiss Med Wkly. 2013;143:w13751.
Olesen J, et al. Cost of disorders of the brain in Denmark. Nord J Psychiatry. 2008;62(2):114–20.
Pugliatti M, et al. Cost of disorders of the brain in Italy. Neurol Sci. 2008;29(2):99–107.
Schoenen J, et al. Cost estimates of brain disorders in Belgium. Acta Neurol Belg. 2006;106(4):208–14.
Stovner LJ, et al. Cost of disorders of the brain in Norway. Acta Neurol Scand Suppl. 2010;190:1–5.
Wancata J, Sobocki P, Katschnig H. Cost of disorders of the brain in Austria in the year 2004 [in German]. Wien Klin Wochenschr. 2007;119(3–4):91–8.
OECD. OECD Health Statistics 2018. Available at: http://www.oecd.org/els/health-systems/health-data.htm. Accessed 29 Jun 2018.
Friedrich JO, Adhikari NK, Beyene J. The ratio of means method as an alternative to mean differences for analyzing continuous outcome variables in meta-analysis: a simulation study. BMC Med Res Methodol. 2008;8:32.
Friedrich JO, Adhikari NK, Beyene J. Ratio of geometric means to analyze continuous outcomes in meta-analysis: comparison to mean differences and ratio of arithmetic means using empiric data and simulation. Stat Med. 2012;31(17):1857–86.
DuPont RL, et al. Economic costs of anxiety disorders. Anxiety. 1996;2(4):167–72.
Greenberg PE, et al. The economic burden of anxiety disorders in the 1990s. J Clin Psychiatry. 1999;60(7):427–35.
Sado M, et al. Cost of anxiety disorders in Japan in 2008: a prevalence-based approach. BMC Psychiatry. 2013;13:338.
Shirneshan E, et al. Incremental direct medical expenditures associated with anxiety disorders for the U.S. adult population: evidence from the Medical Expenditure Panel Survey. J Anxiety Disord. 2013;27(7):720–7.
Sillanpää M, Andlin-Sobocki P, Lonnqvist J. Costs of brain disorders in Finland. Acta Neurol Scand. 2008;117(3):167–72.
Huang CJ, et al. Health care utilization and expenditures of persons with diabetes comorbid with anxiety disorder: a national population-based cohort study. Gen Hosp Psychiatry. 2015;37(4):299–304.
Acarturk C, et al. Economic costs of social phobia: a population-based study. J Affect Disord. 2009;115(3):421–9.
Dams J, et al. Excess costs of social anxiety disorder in Germany. J Affect Disord. 2017;213:23–9.
Marciniak M, et al. Medical and productivity costs of anxiety disorders: case control study. Depress Anxiety. 2004;19(2):112–20.
Olfson M, Gameroff MJ. Generalized anxiety disorder, somatic pain and health care costs. Gen Hosp Psychiatry. 2007;29(4):310–6.
Patel A, et al. The economic consequences of social phobia. J Affect Disord. 2002;68(2–3):221–33.
Rees CS, Richards JC, Smith LM. Medical utilisation and costs in panic disorder: a comparison with social phobia. J Anxiety Disord. 1998;12(5):421–35.
Rovira J, et al. The cost of generalized anxiety disorder in primary care settings: results of the ANCORA study. Community Ment Health J. 2012;48(3):372–83.
Rutledge T, et al. Anxiety associations with cardiac symptoms, angiographic disease severity, and healthcare utilization: the NHLBI-sponsored Women’s Ischemia Syndrome Evaluation. Int J Cardiol. 2013;168(3):2335–40.
Toghanian S, et al. Economic and humanistic burden of illness in generalized anxiety disorder: an analysis of patient survey data in Europe. Clinicoecon Outcomes Res. 2014;6:151–63.
Vasiliadis HM, et al. The excess healthcare costs associated with depression and anxiety in elderly living in the community. Am J Geriatr Psychiatry. 2013;21(6):536–48.
Drost R, et al. Conceptualizations of the societal perspective within economic evaluations: a systematic review. Int J Technol Assess Health Care. 2017;33(2):251–60.
Author information
Authors and Affiliations
Contributions
AK planned the review, conducted the literature search, extracted information, conducted analyses of top-down COI studies, and prepared the manuscript. HK conducted meta-analysis of bottom-up COI studies, prepared respective parts of the manuscript, and critically revised the manuscript prior to submission. Both AK and HK revised the manuscript after peer review. The authors thank Marc Martin for proofreading this manuscript.
Corresponding author
Ethics declarations
Funding
No funding was received for this study.
Conflicts of interest
Alexander Konnopka and Hannah König have no conflicts interest to declare.
Ethical Approval
This article does not contain any studies with human participants or animals performed by any of the authors.
Additional information
Alexander Konnopka and Hannah König share first authorship of this work.
Rights and permissions
About this article
Cite this article
Konnopka, A., König, H. Economic Burden of Anxiety Disorders: A Systematic Review and Meta-Analysis. PharmacoEconomics 38, 25–37 (2020). https://doi.org/10.1007/s40273-019-00849-7
Published:
Issue Date:
DOI: https://doi.org/10.1007/s40273-019-00849-7