Abstract
Introduction
Data incompleteness in pharmacovigilance (PV) health records limits the use of current causality assessment methods for drug-induced liver injury (DILI). In addition to the inherent complexity of this adverse event, identifying cases of high causal probability is difficult.
Objective
The aim was to evaluate the performance of an improved, algorithmic and standardised method called the Pharmacovigilance-Roussel Uclaf Causality Assessment Method (PV-RUCAM), to support assessment of suspected DILI. Performance was compared in different settings with regard to applicability and differentiation capacity.
Methods
A PV-RUCAM score was developed based on the seven sections contained in the original RUCAM. The score provides cut-off values for or against DILI causality, and was applied on two datasets of bona fide individual case safety reports (ICSRs) extracted randomly from clinical trial reports and a third dataset of electronic health records from a global PV database. The performance of PV-RUCAM adjudication was compared against two standards: a validated causality assessment method (original RUCAM) and global introspection.
Results
The findings showed moderate agreement against standards. The overall error margin of no false negatives was satisfactory, with 100% sensitivity, 91% specificity, a 25% positive predictive value and a 100% negative predictive value. The Spearman’s rank correlation coefficient illustrated a statistically significant monotonic association between expert adjudication and PV-RUCAM outputs (R = 0.93). Finally, there was high inter-rater agreement (K w = 0.79) between two PV-RUCAM assessors.
Conclusion
Within the PV setting of a pharmaceutical company, the PV-RUCAM has the potential to facilitate and improve the assessment done by non-expert PV professionals compared with other methods when incomplete reports must be evaluated for suspected DILI. Prospective validation of the algorithmic tool is necessary prior to implementation for routine use.
Similar content being viewed by others
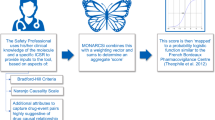
Avoid common mistakes on your manuscript.
The Pharmacovigilance-Roussel Uclaf Causality Assessment Method (PV-RUCAM) is a supportive tool to facilitate case evaluation. Quantitative scoring indicates a degree of relatedness to a suspect medicinal product, and a quality rating system puts the individual case safety report in context. |
A standardised method is essential for liver-injury reports to be properly assessed by all non-expert pharmacovigilance professionals. |
1 Introduction
Medication-induced liver injury constitutes a serious safety concern. Commonly referred to as drug-related hepatotoxicity and drug-induced liver injury (DILI), it can be fatal or lead to severe morbidity [1]. Available biomarkers reflect the extent of liver injury, yet they are not able to establish a causal relationship between liver injury and the incriminated medication [2,3,4,5]. Diagnosis of DILI is made by exclusion of alternative explanations and the result of a probabilistic causality assessment. Different causality instruments perform reasonably well in comparison to the ‘gold standard’ of hepatology expert opinion [6]. Nonetheless, a formal and mechanistic explanation is often lacking [7]. During the development of medicinal products, exposure is only possible through clinical trials. Relatively low numbers of patients are exposed, and patients with pre-existing hepatic impairment are often excluded. When combined with DILI’s idiosyncratic nature and the low event frequency, this makes pre-approval recognition difficult [5, 6, 8, 9]. Following market authorisation, patient exposure often grows rapidly. Frequencies may change, and there is a need for early action to assess whether there is a causal relationship in case of suspected DILI. Applying appropriate methods to identify early cases suggestive of drug causation is of high importance, since the Food and Drug Administration (FDA) considers two non-confounded, serious, DILI (‘Hy’s law’) cases as cause for serious concern [10, 11]. Recent methods aim to assess causality by making use of graphical workflows and statistical analyses of all available clinical, hepatic, safety-related data, in addition to standard tables and narratives [12]. Case reports providing thoroughly documented chronological sequences of transaminases, precise details of exposure(s) to medicinal products and other relevant information support firm conclusions being drawn. However, spontaneous pharmacovigilance (PV) reports are often incomplete, and data quality varies [13].
Hepatology centres, clinicians and health authorities often select the scale by the Council for International Organizations of Medical Sciences (CIOMS), also widely known as the Roussel Uclaf Causality Assessment Method (RUCAM), as their preferred causality assessment method [5, 14, 15]. In fact, over 20 international registries and regulatory registries frequently apply it [9]. Structured and standardised, it considers all core elements to evaluate hepatotoxicity [16,17,18]. These include relevant laboratory values of transaminases ALT,Footnote 1 ASTFootnote 2 and ALP,Footnote 3 vitamin-K-dependent clotting factors and TBIL;Footnote 4 patient medical history in general and liver history in particular; risk factors such as regular alcohol use; confounding factors such as non-drug toxic products and any concomitant medication; and, finally, liver chemistry response after suspected medication discontinuation as well as response to re-challenge with the medication. Time dependence and covariation of the biological parameters are usually necessary to confirm causality [15,16,17, 19, 20].
This article describes a proof of concept of a new method of initial standardised, medication-induced liver injury, causality assessment based on data available in electronic health records. Our objective was to develop an algorithmic approach with consistent applicability, irrespective of data quality or completeness, and with accurate differentiation capacity. The focus was on maximising detection of all possible DILI cases for further consideration, rather than to rule out such cases.
2 Materials and Methods
2.1 The PV-RUCAM
The RUCAM remains the only validated method for the assessment of DILI since its introduction in 1993 [9, 15]. Nonetheless, to be applicable, it requires very complete case reports with input by experts. It is not advised to apply the RUCAM in post-marketing reports, and it has been criticised for its unsatisfactory inter-rater variability [9]. We believe this is due to certain variables lacking exhaustive definitions, notably the assessment of current or past comorbidities, leading to subjective evaluations. The lack of well-defined criteria has previously been underlined [6].
This is why we developed a new method, the Pharmacovigilance-Roussel Uclaf Causality Assessment Method (PV-RUCAM), which is based on the original RUCAM, but is applicable to PV individual case study reports (ICSRs) with incomplete datasets (Table 1). The quantitative scoring includes all seven scoring domains from the original: time to onset of events either from the beginning or cessation of culprit medicinal product intake; dechallenge if treatment was interrupted; risk factors including pregnancy and alcohol use; use of concomitant medicinal or toxic products; exclusion of other causes other than liver injury; the suspect treatment’s safety profile for hepatotoxicity; and course of readministration if carried out. It is acknowledged that rechallenge is often not considered, because of the potential risks, and rechallenge without very careful consideration is usually not recommended. Outputting a score ranging from −10 to +14, the method represents the probability of the suspect medication’s responsibility reflected on an ordinal distribution scale of relatedness. Scores higher than 8 have a "highly probable" relationship; scores of 6–8 are “probable”; scores of 3–5 are “possible”; scores of 1–2 are “unlikely”; and those less than 0 indicate "relationship excluded". Cut-off values are the same as those in the original RUCAM [17, 18]. The operational use of the PV-RUCAM is described in Sect. 2.5.
The RUCAM scoring sheet is available on the National Institute of Diabetes and Digestive and Kidney Diseases (NIDDK) LiverTox website [21]. Adapting the seven assessment sections of the original RUCAM to the context of incomplete PV data required five adjustments.
-
1.
Differentiating liver injury type (hepatocellular, cholestatic or mixed) would require a ratio calculation based on enzyme levels. This is not possible in the majority of PV case reports since complete results of liver tests are rarely stated.
-
2.
The PV-RUCAM set days of onset latency equal to hepatocellular injury timeframes of the RUCAM, as this type of injury is most common [17, 18]. A score of 0 was added in case of no reported time to onset. Subsequent treatments usually have shorter timelines, but a conservative approach was taken to avoid underscoring [22].
-
3.
Unreported age or other absent risk factors are given a score of 0.
-
4.
The course of reaction when the medicinal product is stopped or re-introduced, also referred to as de- and re-challenge, was simplified. The RUCAM algorithm uses enzyme chronologies to evaluate course of reaction, which was adapted for missing lab values. For example, if events disappear after medication cessation, a suggestive dechallenge is recorded.
-
5.
Temporal relations to concomitant medicinal products were removed. These are not formally required to be recorded and are only occasionally described in case narratives. On the other hand, the concomitant suspectedness classification in the RUCAM was retained.
Additional Electronic Supplementary Material (ESM) supports assessment of domains in the PV-RUCAM.
-
Concomitant medicinal products including over 1200 drugs are listed in ESM 2. Containing substances that have equivocal evidence supporting the risk of liver-associated damage, a conservative approach was taken, since many mechanisms of injury and accurate risk classification remain unknown. The list was derived from the agents available on the LiverTox website [21]. In addition, internal Novartis ‘Hepatotoxicity Clinical Safety Standard Guideline’ medications were added [10].
-
Comorbidities are listed in ESM 3i and 3ii, with over 480 preferred terms (PTs). Hepatology experts identified these with searches in the Medical Dictionary for Regulatory Activities (MedDRA®, version 18.1). Relevance may vary depending on the condition’s context, such as an incidental finding versus a historical condition. PTs to individually define ‘alcohol use’ and ‘alcoholism’ are listed in ESM 4.
2.2 Setting
Gauging performance of the PV-RUCAM method required two evaluations. First against complete-data ICSRs to valuate against the validated RUCAM method and assess the differentiation capacity. Secondly against PV expert adjudication to determine performance in the intended PV setting. This was particularly important since data quality varies significantly in PV databases.
2.3 Data Collection
Liver-specific adverse events rich in data, adjudicated for and against causality, were needed for the first evaluation. ICSRs were obtained from the Innovative Medicines Initiative’s (IMI) Safer and Faster Evidence-based Translation (SAFE-T) consortium. Various potential hepatotoxins were under investigation in patients with broad demographics (age, sex and nationality). All ICSRs were scored with the RUCAM against the adjusted PV-RUCAM. Cases had an inherent nature to be of higher probability because the consortium focused on qualification of new biomarkers for DILI and thus a priori included patients with a high likelihood to have experienced DILI. ICSRs with lower likelihoods were obtained from a liver panel that had reviewed clinical trial reports with potential hepatotoxicity in 2014. The panel used global introspection and the Drug-Induced Liver Injury Network (DILIN) causality scale rather than the RUCAM. The non-numerical causality categories of the DILIN scale limited a direct comparison. Furthermore, although both methods have five degrees of distribution, there are discrepancies in the way terminologies are applied. Attributions of ‘unlikely’, ‘possible’ and ‘probable’ may be shifted or weighted differently albeit present in both. This required collapsing the five-degree scales of the PV-RUCAM and DILIN into ‘yes’ or ‘no’ suspected DILI causality. We took the same approach as Rockey et al. [22], defining ‘yes’ as probable or higher, and ‘no’ as possible or lower.
The second evaluation included spontaneous PV drug–event reports extracted from the global safety database of Novartis, Oracle Argus. All reports were liver-related injuries reported in temporal association with the heart failure treatment sacubitril/valsartan (LCZ696). Globally available to patients since June 2015, this angiotensin receptor neprilysin inhibitor has potential risk for hepatotoxicity [23]. It was selected in order to have one suspect medicinal product with recent reports of varying origin, quality and severity. PV experts also adjudicated using the DILIN. In contrast to the first evaluation, keeping the medicinal product controlled in all ICSRs was necessary to accurately assess how fluctuations in data quality may affect overall adjudication.
2.4 Drug Causality Adjudication Processes
To compare the PV-RUCAM against methods chosen as standards, several datasets were required. Table 2 summarises the ICSR sources and settings as well as methods used to adjudicate.
2.4.1 Evaluation of Drug Causation by SAFE-T
Cases provided by the IMI SAFE-T consortium, a public–private partnership focusing on clinical qualification of new biomarkers for drug-induced liver, kidney and vascular injury, were adjudicated by three liver experts. Data were prepared by the principal investigator of the respective study. Each case was presented graphically on three to four slides to the adjudication committee at a dedicated teleconference, with details such as baseline characteristics, medication history, time course of liver enzyme elevations and a preliminary calculation of the RUCAM score. Following the group discussion, the adjudication committee then assessed final causality for each case. Reports of potential DILI were obtained between 2014 and 2015.
2.4.2 Evaluation of Drug Causation by Liver Panel
A panel of three hepatologists evaluated clinical trial patients reporting hepatotoxicity-related adverse events during sacubitril/valsartan’s development. The panel systematically reviewed all hepatotoxicity cases with standard summary tables and narratives, and any discrepancies between experts were resolved by consensus. Running the RUCAM in addition to expert assessment using the DILIN scale was beyond the resources of this work and therefore not repeated.
2.4.3 Evaluation of Drug Causation by PV-Expert Adjudication
Consensus opinion amongst PV experts was considered as the standard for the evaluation of real-world PV electronic medical records, often lacking key data elements. Assessment on a case-by-case basis was conducted by experienced medical specialists responsible for the safety of LCZ696. This group comprised two senior physicians, a senior scientist and a PV scientist. The process was systematically repeated, first each expert individually assigned a probability of the culprit drug’s responsibility, using a causality likelihood scale from the DILIN [24, 25] (Table 3). No further actions were required if full agreement was initially reached. If assigned likelihoods differed between experts, discrepancies were jointly discussed. Full joint agreement was required with justified conclusions. Evaluations were also summed for the aggregate analysis of the topic. As a mandatory section in LCZ696’s periodic safety update reports, this complies with current causality assessment practices. Note that these are also reviewed globally by health authorities.
2.4.4 Evaluation of Drug Causation by the PV-RUCAM Algorithm
The PV-RUCAM algorithm consists of assessing seven core components (Table 1). Prior to assigning points with this scoring sheet, operational rules were applied (Table 4). This included identifying any of the 56 specific hepatotoxicity-related PTs (see ESM 1). These were selected from 273 available terms in the standard MedDRA® query ‘drug-related hepatic disorders-comprehensive search’. In the event that no matching terms were identified, or other operational rules applied, cases were excluded and assigned a score of 0. Case data relevant to each domain of the PV-RUCAM scoring sheet (Table 1) were searched for and evaluated with the aid of the ESM files. The forth domain, ‘concomitant medicinal products’, was supported with a list of concomitant products (ESM 2). In the event of identified matching terms, −2 or −3 points were assigned depending on the link to injury. The fifth domain, ‘exclusion of other causes of liver injury’, was supported with lists of comorbid conditions and alcohol-related PTs (ESM 3i, 3ii and 4). The relevance of a matching reported term to the domain is mentioned in the ESM.
The same observer was assigned to evaluate all cases. The PV dataset was also reviewed by a second observer to determine inter-rater agreement in its intended setting.
2.5 Data Quality Classification
The PV-RUCAM can establish a degree of relatedness to any PV electronic medical record containing the required information about medications and the nature of the hepatic dysfunction. Nonetheless, it does not indicate or reflect the degree of quality of the information content. The inability to treat data neutrally in the event of gaps (null values) may lead to overestimation of scores and hence to false positives. To circumvent this limitation, three quality categories were recognised in an effort to separate high-scoring reports of true relatedness from ones that were poorly documented. We recommend classifying the case before or after applying the PV-RUCAM using the definitions below:
-
Category A or ‘satisfactory quality’: reports with sufficient documentation to undertake a causality assessment. Three domains must be included for a case to meet the minimum requirements: reports of the onset latency; concomitant products; and medical history.
-
Category B or ‘unsatisfactory quality’: reports with inadequate documentation to assume and support a causal relationship; applicable when the minimum requirements of ‘A’ are unmet. We recommend the adoption of targeted follow-up questions as the most effective and efficient method of improving the quality.
-
Category O or ‘unclassified’: reports were causality was excluded for a non-quality-related reason. This applies to cases where one or more of the four operational rules hindered the use of the PV-RUCAM.
2.6 Statistical Analyses
Patients from the three cohorts were described with standard descriptive statistics. Contingency tables were used to compare assigned degrees of drug–event relatedness between methods. Cohen’s weighted Kappa coefficient (K w) was applied to measure the agreement between methods as well as the inter-rater variability. Agreement by percentage is not sufficient, as it does not consider the influence of chance, and a weighted K can account for the degree of disagreement [26, 27]. It is recommended for ordered categories and attaches greater emphasis on larger differences [28, 29]. Weighted Kappa values usually range from 0 to 1, where 1 indicates maximal agreement accuracy. One-sided upper 95% confidence limit (U95CL) was derived to put K w values in scope of their minimum threshold of acceptability [30]. The monotonic association between the PV-RUCAM and PV-expert adjudication was assessed with Spearman’s correlation coefficient. Sensitivity, specificity, and positive and negative predictive values were determined using PV experts as the reference mark [31].
3 Results
3.1 Patient Characteristics in Study Cohorts
Clinical characteristics of each dataset are shown in ESM 5. The 49 reports reviewed by SAFE-T have various suspect drugs. The most recurrent included eight reports of acetaminophen, five reports of amoxicillin, six reports of flucloxacillin, and a range of chemotherapies and some miscellaneous products. Age range varied considering the standard deviation of the mean age, 55.2 ± 16.4. No deaths were reported, and the injuries were mostly seen in women (59%). Confounding co-medications were prevalent, in just under 50% of all cases.
The clinical trial liver panel and PV experts adjudicated 21 and 36 cases, respectively, all associated with LCZ696. Elevated patient age in LCZ696 reports was expected, the two datasets averaging 71.5 ± 9.0 and 61.2 ± 11.0 years. The majority of cases were men, 69 and 81%, respectively. The PV dataset had four deaths (11%), and there were three in the LCZ696 clinical trial cases (14%). Potential confounding concomitants were common across both datasets, 72.2 and 95.2%, respectively. Considering the severity of heart failure with comorbidities, a larger number of co-administered products per patient is not surprising; thus the probability of confounders increases. The risk associated with current or past use of alcohol was lower, but still statistically significant, averaging 37% across all reports. There were no reported pregnancy risks.
3.2 Comparison of ICSRs Adjudicated by the SAFE-T Consortium Against the PV-RUCAM
Assessment of the 49 drug–event pairs adjudicated by experts of the SAFE-T consortium was carried out with the RUCAM scoring sheet [18]. Scores ranged from +3 to +10 (±standard error), and were consequently distributed across three of the five possible causality categories. A mean of 7.65 ± 1.79 was found, and Table 5 shows how cases were spread across the degrees of relatedness, including ‘highly probable’ (37%, n = 18), ‘probable’ (51%, n = 25) and ‘possible’ (12%, n = 6). Since the PV-RUCAM uses the same distribution scale, a direct comparison was possible. With an identical scoring average of 7.65 ± 2.01 and scores in a range of +3 to +13, the agreement in relative ranking was 69% (n = 34). Scores fell within one category of each other in 98% of cases (n = 48). The linear weighted Kappa showed moderate agreement, K w = 0.53 (U95CL = 0.74).
3.3 Comparison of ICSRs by a Clinical Trial Liver Panel Against the PV-RUCAM
Twenty-one reports evaluated by a liver panel during sacubitril/valsartan’s clinical development were compared against the PV-RUCAM to allow comparison across the entire causality distribution scale. The panel applied the DILIN adjudication scale. The ‘relationship excluded’ causality was predominant (62%, n = 13); two other categorisations were recorded, with 24% (n = 5) as ‘unlikely’ and 14% (n = 3) as ‘possible’.
Since terminological assumptions cannot be made, direct comparisons were undertaken in relative rankings of contingency tables. The liver panel adjudicated 15 cases as ‘unlikely’ (71%), five as ‘possible’ (24%) and one as ‘probable’ (5%). Table 6 shows an 86% (n = 18) agreement in relative ranking, with 95% (n = 20) of causalities within one category of each other. This reflects substantial statistical agreement, with a quadratic weighted K w = 0.68 (U95CL = 1). Across the two complete ICSR datasets of Sects. 3.2 and 3.3, scores ranged from −4 to +13 (18 of 25 possible scores were met).
3.4 Comparison of PV Sacubitril/Valsartan ICSRs by PV Experts Against the PV-RUCAM
Comprising 36 drug–event pairs, the third dataset was used to evaluate PV-RUCAM’s performance in its intended setting of PV case reports. Recent database cases were assessed separately by two observers. The comparison against PV expert adjudication showed 50% (n = 18) and 47% (n = 17) matching in relative rankings with observer A and B, respectively (Table 7). Significant discrepancies, more than one category apart, were observed in 19% (n = 7) and 14% (n = 5). Although scores ranged from −4 to 9, relationships were excluded prior to assessment in 11 of the 17 cases in this group due to operational rules. A quadratic weighted Kappa showed fair agreement between PV experts and PV-RUCAM observer A (K w = 0.39, U95CL = 0.50). There was low inter-rater variability between the two PV-RUCAM observers; the high relative ranking agreement of 81% (n = 29) was confirmed by a linear weighted K w = 0.79 (U95CL = 0.94).
In an attempt to account for discrepancies in scales, the dichotomous definitions separated drug causation into ‘yes’ or ‘no’ suspected DILI categories (Table 8). There were no false negatives. An agreement of 89% (n = 32) was recorded with observer A, resulting in 100% sensitivity, 91% specificity, a 25% positive predictive value and a 100% negative predictive value. Since few discrepancies were seen between observers, only observer A was used to obtain these values. A statistically significant monotonic association between PV experts and the PV-RUCAM was shown by a strong Spearman’s rank correlation of R = 0.93 (P = 0.008).
Regarding the data quality classification, a satisfactory category A was obtained for 25% of cases. The majority (44%) of records failed to meet minimum requirements and were classified as ‘B’, and 31% remained unclassified, i.e. ‘O’.
4 Discussion
This research compared a novel supportive causality assessment method for medication-induced liver injury (PV-RUCAM) against the validated hepatotoxicity assessment RUCAM method [9] and against consensual PV expert judgements.
Guidance from the International Conference on Harmonisation (ICH) for post-approval safety (E2D) states that inferences and imputations are to be avoided during report submission [32]. The same is true during causality adjudication. The data quality classification does address this to a certain extent. Nonetheless, significant incomplete data remain a major confounder during case assessment, limiting the ability to assess causality of liver-related injuries [4]. Post-marketing surveillance is often incapable of presenting compelling results to confirm harms of approved medications [33]. Over half of electronic records in a broader PV systems search did not report initial transaminase levels [13]. Global introspection by hepatology experts remains the gold standard [6]. The requirements for significant expertise and intrinsic subjectivity limit the possibility to standardise this approach. The RUCAM [17, 18] and the Maria and Victorino (M&V) method [34] are recognised assessment methods, but are difficult to apply when there are gaps in the data. The most recognised non-specific adverse drug reaction (ADR) evaluation methods include the Naranjo and Liverpool algorithms [35, 36] as well as a Bayesian logistical approach [37, 38]. The ADR evaluation methods are theoretically applicable, but they lack significant transferability in this context. From five likelihood categories, the highest and lowest ranked are rarely obtained with this assessment [35, 39]. This may represent a systematic bias, and it appears to be due to incomplete data.
Random datasets from over 4 million electronic medical records from the global Novartis PV database were used in this research. As compared with the original RUCAM, our new algorithm showed good overlap, with 69% direct agreement in causality classification. The aim was to have the PV-RUCAM version as close as possible to the original RCAUM whilst being able to apply it to any electronic medical record as available in a large, existing PV database. The most notable adjustment regarded removing enzyme requirements. As a result, the type of injury could not be specified and timeframe correlations of the onset and the course of reaction had to be simplified. The moderate agreement observed with Cohen’s Kappa coefficient, K w = 0.53, hints to some discrepancies that may have been caused by these changes. This may have been counterbalanced with the supportive lists of hepatotoxins and weighted comorbidities in the PV-RUCAM versions. Also, the automated reading of the PV-RUCAM datasets makes this first analytical step on a given case less open to subjectivity. The standardisation limits subjectivity and reduced inter-observer variability, allowing its use by qualified patient safety professionals and not limiting adoption only to hepatology experts [9].
Various causality assessment methods were compared, including liver-specific methods as well as general approaches without specificity for a particular body system or therapeutic area (Table 9).
When compared with the two datasets adjudicated by consensual expert judgement, the PV-RUCAM performed reasonably well since agreement in relative rankings was seen in half of all ICSRs. Moreover, the key objective of having no false negatives was observed when dichotomously collapsed as ‘yes’ or ‘no’ suspected DILI. Across the three datasets, reports categorised by the PV-RUCAM were expressed continuously across the five degrees of relatedness, in a similar fashion to the original RUCAM and consensual expert judgement. This was an improvement compared with methods, such as the Naranjo and Liverpool algorithms, which had previously shown poor differentiation capacity [35, 39]. The disposition of the randomly selected PV electronic medical records had a strong tendency towards an ‘unlikely’ relationship, comprising 70% (n = 25) of all cases adjudicated by PV experts. No reports of ‘definite’ or ‘highly likely’ causality were identified. Only 17% (n = 6) of all PV cases reported transaminases, and the majority did not meet requirements to be of satisfactory quality. The corresponding equivalents in the PV-RUCAM, defined as ‘unlikely’ and ‘relationship excluded’ likelihoods, amounted to 75% (n = 27) of cases.
Discrepancies in adjudication, observed in both sacubitril/valsartan datasets, were predominantly due to two recurrent scale items which were major confounders: concomitant medicinal products and searches for alternative aetiologies. The two domains range from 0 to −3 and +2 to −2, respectively. The large interval is more likely to change case classification. Teschke and Danan [4] recently emphasised the importance of distinguishing other likely explanations, including pre-existing conditions. Coding comorbidities in electronic medical records must comply with health authority prerequisites; as such, the PV-RUCAM complementary PT lists (ESM 3i and 3ii) facilitate the identification of appropriate terms amongst the thousands of possibilities. Previously, such inconsistencies were reported to lead to low agreement [35]. Four of seven cases had no relative agreement, because of a 3-point difference in the ‘exclusion of other causes of injury’. An alternative explanation may be the arbitrary non-standardised characteristics of the DILIN. It requires extensive practice and experience to unequivocally adjudicate a case with resembling terminology [24, 25].
Overall, the two main strengths of the PV-RUCAM are its automated applicability to any electronic medical record data set and its usability by non-specialist PV professionals. Besides the positive predictive value, the PV-RUCAM’s sensitivity, specificity, and high negative predictive value showed improvement over the RUCAM, Naranjo and Bayesian approaches [18, 37, 39]. The use of the PV-RUCAM is especially interesting to post-marketing PV, with frequently incomplete datasets. To place resulting causality scores in context, we recommend adding a quality rating based on a valid quality classification system. This should encourage follow-ups if minimum requirements are unmet.
4.1 Limitations
PV-RUCAM is limited by the data quality of the available ICSRs (source data). As a consequence, incentivising physicians to improve quality is urgent. Facilitating the process through digital information management may be the way forward. Our study was a proof of concept with a small number of cases. Prospective validation is necessary to confirm our initial findings. Inherent bias towards a suspect drug may occur, especially for physicians who are repeatedly informed about certain hepatotoxins. Previous liver-related awareness was not considered. The weighted Kappa does not detect bias between two raters [28]. For example, rivaroxaban was disproportionally signalled because of our historical understanding of a known hepatotoxic anticoagulant, ximelagatran [1, 16].
Consideration of liver enzymes and their temporal associations was reduced for greater applicability of the PV-RUCAM in its intended setting. The four required methodological changes had repercussions compared to the original RUCAM. The inability to differentiate between types of injuries can affect implications drawn by observers when the injury is put in context. It also quantitatively impacts other domains of the assessment, notably time to onset and the course of reaction. Many ICSRs were susceptible to being affected since an increasingly high portion of the bona fide SAFE-T cases, 85.7%, had a suggestive dechallenge. The PV-RUCAM identified 10% more, perhaps also since temporal associations of dechallenge were not adapted to liver tests based on injury type. The impact may be visible if scores are directly compared, less so in the subsequent causality classifications. Similarly, inconsistencies occurred because timeframe correlations of concomitant medicinal products with injury onset were removed in the PV-RUCAM. A 25% increase in suspected concomitants was recorded in the SAFE-T ICSRs adjudicated with the PV-RUCAM. This may partly explain the 20% of cases that were underscored as compared to the SAFE-T adjudication. Thus, this modification may cause significant changes to the causality adjudication.
PV-RUCAM has most value when used in its entirety. Considering the operational information (Table 4), the scoring sheet (Table 1) should systematically be supported with an a priori previously established list of hepatotoxins (ESM 2) and differential diagnoses (ESM 3i and 3ii). However, this makes the assessment more labour intensive, and similarly, the Bayesian logistical method was not recommended to be adopted in routine practice because of its complex and time-consuming characteristics [36]. We recommend that PV professionals receive appropriate training prior to use of the PV-RUCAM. Moreover, manual human extraction of case data is necessary to assess domains of the PV-RUCAM. An automated PV-RUCAM querying a database would resolve this problem. A software solution is currently under development, and the flow diagram can be made available. This may reduce the required resources and discrepancies with regard to tedious and error-prone searches for alternative causes. Furthermore, iterative updates to the lists will be required for the method to stay current and applicable.
Finally, the time dependence of liver-specific laboratory test observations remains the key criterion for a thorough assessment [12]. The PV-RUCAM does not assess this because the differentiation of liver injury type (hepatocellular, cholestatic or mixed) requires ratio calculations based on test levels over time [17, 18]. This is not possible in the majority of PV case reports, since complete results of liver tests are rarely stated. In addition, gold standard clinical methods based on expert opinion rely on time series of liver tests and possibly rechallenge [6].
5 Conclusions
Preliminary appraisal of this novel method supporting the causality assessment of suspected DILI in electronic health records has shown it performs well in a bona fide setting as well as its intended PV setting, often with non-source-verifiable data. No false negatives or missed suspected cases were identified. As a well-structured and standardised tool, it may be used by all non-expert PV professionals. Prospective validation across a broad range of products in different therapeutic areas is necessary to further refine the algorithmic differentiation capacity.
Notes
ALT: alanine aminotransferase.
AST: aspartate aminotransferase.
ALP: alkaline phosphatase.
TBIL: total bilirubin.
References
Goldkind L, Laine L. A systematic review of NSAIDs withdrawn from the market due to hepatotoxicity: lessons learned from the bromfenac experience. Pharmacoepidemiol Drug Saf. 2006;15(4):213–20. doi:10.1002/pds.1207.
Sarges P, Steinberg JM, Lewis JH. Drug-induced liver injury: highlights from a review of the 2015 literature. Drug Saf. 2016;38:801. doi:10.1007/s40264-016-0427-8.
Watkins PB, Merz M, Avigan MI, Kaplowitz N, Regev A, Senior JR. The Clinical Liver Safety Assessment Best Practices Workshop: rationale, goals, accomplishments and the future. Drug Saf. 2014;37(1):1–7. doi:10.1007/s40264-014-0181-8.
Teschke R, Danan G. Diagnosis and management of drug-induced liver injury (DILI) in patients with pre-existing liver disease. Drug Saf. 2016;39(8):729–44. doi:10.1007/s40264-016-0423-z.
Teschke R, Wolff A, Frenzel C, Schwarzenboeck A, Schulze J, Eickhoff A. Drug and herb induced liver injury: Council for International Organizations of Medical Sciences scale for causality assessment. World J Hepatol. 2014;6(1):17–32. doi:10.4254/wjh.v6.i1.17.
Regev A, Seeff LB, Merz M, Ormarsdottir S, Aithal GP, Gallivan J, et al. Causality assessment for suspected DILI during clinical phases of drug development. Drug Saf. 2014;37(1):47–56. doi:10.1007/s40264-014-0185-4.
Senior JR. Evolution of the Food and Drug Administration approach to liver safety assessment for new drugs: current status and challenges. Drug Saf. 2014;37(1):9–17. doi:10.1007/s40264-014-0182-7.
Kaplowitz N. Idiosyncratic drug hepatotoxicity. Nat Rev Drug Discov. 2005;4(6):489–99. doi:10.1038/nrd1750.
Danan G, Teschke R. RUCAM in drug and herb induced liver injury: the update. Int J Mol Sci. 2015;17(1):1–33. doi:10.3390/ijms17010014.
Close P, Collins W, Pellet P, Warner BA. Hepatotoxicity clinical safety standard guideline. Novartis Drug Saf Epidemiol. 2013;1:22–37.
FDA. Guidance for Industry: Drug-induced liver injury: premarketing clinical evaluation. 2009; http://www.fda.gov/downloads/Drugs/…/Guidances/UCM174090.pdf. Accessed 06 Jul 2016.
Avigan MI, Bjornsson ES, Pasanen M, Cooper C, Andrade RJ, Watkins PB, et al. Liver safety assessment: required data elements and best practices for data collection and standardization in clinical trials. Drug Saf. 2014;37(1):19–31. doi:10.1007/s40264-014-0183-6.
Agarwal VK, McHutchison JG, Hoofnagle JH. Important elements for the diagnosis of drug-induced liver injury. Clin Gastroenterol Hepatol. 2010;8(5):463–70. doi:10.1016/j.cgh.2010.02.008.
Miljkovic M, Dobric S, Dragojevic-Simic V. Accuracy and reproducibility of two scales in causality assessment of unexpected hepatotoxicity. J Clin Pharm Ther. 2012;37(2):196–203. doi:10.1111/j.1365-2710.2011.01282.x.
Garcia-Cortés M, Stephens C, Lucena MI, Fernandez-Castañer A, Andrade RJ. Causality assessment methods in drug induced liver injury: strengths and weaknesses. J Hepatol. 2011;55(3):683–91. doi:10.1016/j.jhep.2011.02.007.
Raschi E, de Ponti F. Drug- and herb-induced liver injury: progress, current challenges and emerging signals of post-marketing risk. World J Hepatol. 2015;7(13):1761–71. doi:10.4254/wjh.v7.i13.1761.
Danan G, Benichou C. Causality assessment of adverse reactions to drugs-I. A novel method based on the conclusions of international consensus meetings: application to drug-induced liver injuries. J Clin Epidemiol. 1993;46(11):1323–30. doi:10.1016/0895-4356(93)90101-6.
Benichou C, Danan G, Flahault A. Causality assessment of adverse reactions to drugs-II. An original model for validation of drug causality assessment methods: case reports with positive rechallenge. J Clin Epidemiol. 1993;46(II):1331–6. doi:10.1016/0895-4356(93)90102-7.
Björnsson E, Olsson R. Outcome and prognostic markers in severe drug-induced liver disease. Hepatology. 2005;42(2):481–9. doi:10.1002/hep.20800.
Marrero J, Ahn J, Rajender Reddy K. ACG clinical guideline: the diagnosis and management of focal liver lesions. Am J Gastroenterol. 2014;109:1–20. doi:10.1038/ajg.2014.213.
United States National Institute of Diabetes and Digestive and Kidney Diseases (NIDDK) -. LiverTox, clinical and research information on drug-induced liver injury. https://livertox.nih.gov/drugliverinjury.html. Accessed 26 Jun 2016.
Rockey DC, Seeff LB, Rochon J, Freston J, Chalasani N, Bonacini M, et al. Causality assessment in drug-induced liver injury using a structured expert opinion process: comparison to the Roussel-Uclaf causality assessment method. Hepatology. 2010;51(6):2117–26. doi:10.1002/hep.23577.
Abraham M, Berhkin M, Weitbruch M, Romano M, Zhang A. Sacubitril/valsartan 50,100, 200 mg film-coated tablets Core Data Sheet. In: Novartis Pharma AG, Drug Regulatory Affairs. 2015. p. 107–23.
Hoofnagle JH. Drug-Induced Liver Injury Network (DILIN). Hepatology. 2004;40:773.
Fontana RJ, Watkins PB, Bonkovsky HL, Chalasani N, Davern T, Serrano J, et al. Drug-induced liver injury network (DILIN) prospective study: rationale, design and conduct. Drug Saf. 2009;32(1):55–68. doi:10.2165/00002018-200932010-00005.
Sim J, Wright CC. The kappa statistic in reliability studies: use, interpretation, and sample size requirements. Phys Ther. 2005;85(3):257–68.
Altman D. Practical statistics for medical research. Chapman and Hall; 1991. doi:ISBN:9780412276309.
Ludbrook J. Statistical techniques for comparing measurers and methods of measurement: a critical review. Clin Exp Pharmacol Physiol. 2002;29(7):527–36. doi:10.1046/j.1440-1681.2002.03686.x.
Cohen J. A coefficient of agreement for nominal scales. In: Educational and Psychological Measurement. 1960. p. 37–46. doi:10.1177/001316446002000104.
Walsh JF, Reznikoff M. Bootstrapping: a tool for clinical research. J Clin Psychol. 1990;46:928– 930.
Attia J. Moving beyond sensitivity and specificity: using likelihood ratios to help interpret diagnostic tests. Aust Prescr. 2003;26(5):111–3. doi:10.18773/austprescr.2003.082.
International Conference on Harmonisation. ICH harmonised tripartite guideline post-approval safety data management: definitions and standards for expedited reporting E2D. 2003; https://www.ich.org/fileadmin/Public_Web_Site/ICH_Products/Guidelines/Efficacy/E2D/Step4/E2D_Guideline.pdf. Accessed 18 Jan 2016.
Moore TJ, Furberg CD. Electronic health data for postmarket surveillance: a vision not realized. Drug Saf. 2015;38(7):601–10. doi:10.1007/s40264-015-0305-9.
Maria VA, Victorino RM. Development and validation of a clinical scale for the diagnosis of drug-induced hepatitis. Hepatology. 1997;26(3):664–9. doi:10.1002/hep.510260319.
Théophile H, André M, Miremont-Salamé G, Arimone Y, Bégaud B. Comparison of three methods (an updated logistic probabilistic method, the Naranjo and Liverpool algorithms) for the evaluation of routine pharmacovigilance case reports using consensual expert judgement as reference. Drug Saf. 2013;36(10):1033–44. doi:10.1007/s40264-013-0083-1.
Agbabiaka TB, Savović J, Ernst E. Methods for causality assessment of adverse drug reactions: a systematic review. Drug Saf. 2008;31(1):21–37. doi:10.2165/00002018-200831010-00003.
Arimone Y, Bégaud B, Miremont-Salamé G, Fourrier-Réglat A, Molimard M, Moore N, et al. A new method for assessing drug causation provided agreement with experts’ judgment. J Clin Epidemiol. 2006;59(3):308–14. doi:10.1016/j.jclinepi.2005.08.012.
Théophile H, André M, Arimone Y, Haramburu F, Miremont-Salamé G, Bégaud B. An updated method improved the assessment of adverse drug reaction in routine pharmacovigilance. J Clin Epidemiol. 2012;65(10):1069–77. doi:10.1016/j.jclinepi.2012.04.015.
Garcia-Cortes M, Lucena MI, Pachkoria K, Borraz Y, Hidalgo R, Andrade RJ. Evaluation of Naranjo Adverse Drug Reactions Probability Scale in causality assessment of drug-induced liver injury. Aliment Pharmacol Ther. 2008;27(9):780–9. doi:10.1111/j.1365-2036.2008.03655.x.
Naranjo CA, Busto U, Sellers EM, Sandor P, Ruiz I, Roberts E a, et al. A method for estimating the probability of adverse drug reactions. Clin Pharmacol Ther. 1981;30:239–45. doi:10.1038/clpt.1981.154.
Kaplowitz N. Causality assessment versus guilt-by-association in drug hepatotoxicity. Hepatology. 2001;33(1):308–10. doi:10.1053/jhep.2001.21083.
Acknowledgements
The research leading to these results has received support from the Innovative Medicines Initiative Joint Undertaking under grant agreement n° 115003, the resources of which are composed of financial contributions from the European Union’s Seventh Framework Programme (FP7/2007-2013) and EFPIA companies, in kind contribution.
Author information
Authors and Affiliations
Corresponding author
Ethics declarations
Funding
The first author was funded as a Novartis associate during the execution of this work. No other sources of funding were used to assist in the preparation of this study.
Conflicts of interest
David Lewis has no conflicts of interest that are directly relevant to the content of this study. Dr Lewis is a full-time employee of Novartis Pharmaceuticals AG, Basel Switzerland. Dr Lewis holds shares in Novartis and GlaxoSmithKline, and has options on Novartis stock. Statement regarding conflicts of interest: ‘This publication has not been influenced or amended as a result of my employment or my shareholdings or stock options. I affirm that the content of the draft is scientific, evidenced-based, current and is not subject to bias.’ Christoph Meier has no conflicts of interest that are directly relevant to the content of this study. Henk Johan Streefkerk has no conflicts of interest that are directly relevant to the content of this study. Dr Streefkerk is a full-time employee of Novartis Pharmaceuticals AG, Basel Switzerland. Dr Streefkerk has options on Novartis stock. Michael Merz has no conflicts of interest that are directly relevant to the content of this study. Dr Merz is a full-time employee of Novartis Institutes for BioMedical Research, Basel, Switzerland. Erik Scalfaro has no conflicts of interest that are directly relevant to the content of this study. Mr Scalfaro was an associate of Novartis Pharmaceuticals AG, Basel Switzerland.
Electronic supplementary material
Below is the link to the electronic supplementary material.
40264_2017_541_MOESM1_ESM.pdf
Supportive information may be found via online access of this article. ESM: (1) specific hepatotoxicity-related PTs; (2) list of concomitant medicinal products; (3i) and (3ii) list of comorbidities and their relevance to the domain ‘exclusion of other causes of injury’; (4) list of PTs to define ‘alcohol use’ and ‘alcoholism’; and (5) dataset of clinical characteristics. (PDF 188 kb)
Rights and permissions
About this article
Cite this article
Scalfaro, E., Streefkerk, H.J., Merz, M. et al. Preliminary Results of a Novel Algorithmic Method Aiming to Support Initial Causality Assessment of Routine Pharmacovigilance Case Reports for Medication-Induced Liver Injury: The PV-RUCAM. Drug Saf 40, 715–727 (2017). https://doi.org/10.1007/s40264-017-0541-2
Published:
Issue Date:
DOI: https://doi.org/10.1007/s40264-017-0541-2