Abstract
One of the key elements of the hydrogeological cycle and a variable used by many water resource operating models is the variation in groundwater level (GWL). One of the biggest obstacles to the drawdown analysis and GWL forecasts is the absence of accurate and complete data. The application of diverse numerical models has been regarded as a reliable approach in recent years. Such models are able to determine the GWL for any given region by utilising a wide variety of statistics, data, and field measurements such as pumping experiments, geophysics, soil and land use maps, topography and slope data, a plethora of boundary conditions, and the application of complex equations. Artificial intelligence-based models need significantly less information. The purpose of this research is to predict the changes of GWL of Shazand plain by using the PSO-ANN, ACA-ANN hybrid methods and deep learning methods LSTM, LS-SVM, and ORELM and comparing with GMS numerical model. The model’s accuracy is evaluated using a two-stage validation and verification process. Then Taylor’s diagram was used to select the best model. Results show that ORELM with R, Nash, RMSE and NRMSE values equal to 0.977, 0.955, 0.512 and 0.058 respectively was the best performance in the test stage. After that is the PSO-ANN model. Using the Taylor diagram is another certain way to guarantee that you’ve picked the best possible model. The research results show that there is a link between the ORELM and the place that is most central to the reference point. Since the GMS model is complex and requires a large amount of data and a time-consuming calibration and validation process, the ORELM model can be utilised with certainty to predict the GWL across the entire plain. This research suggests that instead of using numerical models with a complex and time-consuming structure, deep learning methods with the least required data and with high accuracy should be used to forecast the groundwater level.
Similar content being viewed by others
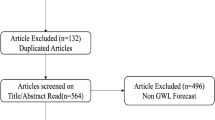
Explore related subjects
Discover the latest articles, news and stories from top researchers in related subjects.Avoid common mistakes on your manuscript.
Introduction
Recent population expansion in Iran that is unsustainable, limits on accessible surface water, and increased usage of the country’s aquifers have led to irreversible harm to the country’s natural resources, which have been depleted to an alarming degree as a consequence. The substantial decrease in GWL is further exacerbated by the fact that aquifers are being contaminated with a wide variety of contaminants as a result of their proximity to urban, industrial, and agricultural regions. As a result, groundwater management and conservation have to be integrated as a fundamental concept and foundation in the planning of the nation in order to put a stop to the continual decline in quantity and quality of the resource. The use of groundwater resources as a dependable backup has been the subject of research recently due to the rise in population in regions where surface water is either in short supply or unreliable. Therefore, in order to make the most of the groundwater resource in terms of its efficiency and effectiveness in planning and development, it is essential to adopt approaches for properly projecting GWL changes, and this is particularly critical during dry years and years with low water levels. When looking at the quantitative and qualitative effects that development has on groundwater resources, mathematical and computer modeling of these resources is seen as a powerful tool in order to make the most effective use of these resources. This is the case whether or not one considers the effects of development on groundwater resources. Several different mathematical and computational models have been considered over the last few decades in an effort to understand the hydraulic behaviour of groundwater resources and predict shifts in GWL. It has been demonstrated that GWL variations can be accurately simulated in numerical models by incorporating a wide range of factors, including topography, geology, boundary and ecological conditions, aquifer physical and hydraulic properties, wetted surface and river sections, aquifer hydraulic parameters, water distribution and extraction practises in the plain, and others. Topography, geology, border, and biological conditions, aquifer physical and hydraulic characteristics, wetted surface and river sections, and aquifer physical and hydraulic properties are only a few examples (Fleckenstein et al. 2010; Luo and Sophocleous 2011; Zampieri et al. 2012; Samani et al. 2018). Most of these models, such MODFLOW and GMS, need extensive production and processing of input data and maps in a standard format since they were developed using finite difference numerical methods. This is essential for producing reliable outcomes (Todd and Kenneth 2001; Yanxun et al. 2011; Irawan et al. 2011; Lachaal et al. 2012). Given the clear relationship between the two, researchers have a strong incentive to examine the effects of climate factors like temperature and precipitation across the entire system and to predict GWL variations in the coming years under the influence of these parameters using mathematical modelling (Klove et al. 2014; Shrestha et al. 2016; Lemieux et al. 2015; Panda et al. 2012; Erturk et al. 2014). Reason being, there can be no denying the two are linked. To do so, we need to include in information and factors that are often missing when modelling surface and groundwater (Fleckenstein et al. 2010; Graham et al. 2015; Ramírez-Hernández et al. 2013; Xie et al. 2016; Mohammed et al. 2023; Moghadam et al. 2019; Alizadeh et al. 2021; Goorani and Shabanlou 2021).
In this regard, there are several studies integrated surface and groundwater models in an attempt to mimic GWL oscillations throughout the whole of the plain. The primary objective of these models is to replicate the saturated and unsaturated zones, respectively. The saturated and unsaturated soil zones are simulated simultaneously in a linked model of surface and groundwater. This has the advantage of allowing for the calculation of the surface and groundwater exchange at varying time and spatial intervals based on the full hydroclimatology water budget in each basin. Another advantage of this type of model is that it can be used to determine the amount of water that can be extracted from the ground. The adoption of this strategy in many aquifers, however, is not possible since it requires a diverse range of data and intricate maps (Zeinali et al. 2020a; b). With the use of simulation tools, the complexities of the connections and formulae involved in managing uniform systems of surface and groundwater resources may be reduced. In order to boost the researcher’s confidence in the modeling process, it is necessary to make use of one or more highly effective simulation tools. These tools should be able to display complex systems in the context of the current reality, and they should also give the researcher the opportunity to participate in the expansion of the model. These models are notorious for the high price tags that they carry (Hu et al. 2016; Ivkovic 2009; Pahar and Dhar 2014; Bayesteh and Azari 2021).
The real system runs the risk of being oversimplified by the model, which also runs the risk of misrepresenting how the system behaves. If the system being investigated is oversimplified, the data coming from the model might not be adequate. In contrast to numerical procedures and mathematical models, approaches that are straightforward, reliable, and call for a minimal amount of data while still producing accurate results quickly and at a low cost are of the utmost significance. They are quicker and more accurate than the methods that were used in the past to predict GWL shifts and the volume of groundwater storage (Soltani and Azari 2022; Guzman et al. 2019; Nadiri et al. 2019; Malekzadeh et al. 2019a, b; Poursaeid et al. 2020, 2021, 2022; Azizpour et al. 2021, 2022; Yosefvand and Shabanlou 2020).
Artificial intelligence (AI)-based approaches, such as GMDH, ELM, LS-SVM, ORELM, and hybrid algorithms, have been extensively used in recent decades to forecast hydroclimatology elements including temperature, rainfall, river flow, changes in the water level of surface reservoirs, and groundwater (Samani et al. 2022; Bilali et al. 2022). Climate, precipitation, river flow, variations in surface reservoir levels, and groundwater are all examples of such variables (Ebtehaj et al. 2020; Zeynoddin et al. 2020; Azari et al. 2021; Poursaeid et al. 2022; Bilali et al. 2021). To wit: (Wang and Hu 2005; Ebtehaj et al. 2016; Zeynoddin et al. 2018; Soltani et al. 2021; Esmaeili et al. 2021; Jalilian et al. 2022; Nourmohammadi Dehbalaei et al. 2023; Zarei et al. 2022).
The findings make it quite clear that each aquifer need its own unique combination of new boundary conditions, data, bedrock information and maps, meteorological parameters, geology, soil map, and data collected from wells related to that area in order to make use of the mathematical models that are often utilised in this context. To predict GWL fluctuations with little data and knowledge, an alternative approach that can be employed with the same degree of accuracy and in less time than mathematical models is essential. Reasons for this include the extensive data and statistical analysis needed, as well as the time and effort involved in calibrating and verifying such models. Yet, many plains lack the expertise required for hydraulic analysis and system modelling of groundwater resources, making it impossible to create a valid estimate of the GWL. As a result, predicting the GWL is challenging.
Therefore, the use of methods based on deep learning and hybrid models, considering the low required data and high accuracy in forecasting, can be a breakthrough in this field and is one of the achievements of this research. The major goal of this research is to compare the results of the numerical model with the predictions made by AI for the oscillations in the GWL. To do this, we’ll first use AI to forecast GWL variations instead of traditional methods. Comparing the results of the GMS numerical model to those of several deep learning and hybrid methods is another goal of this research. Hybrid and deep learning methods such as PSO-ANN, ICA-ANN, LSTM, LS-SVM, and ORELM are examples.
Materials and methods
Study area
The basin of the Qara Chai River contains the 273.19 km2 Shazand Plain. The maximum transmissivity of 2500 square metres per day has been calculated in the middle parts of the Shazand plain based on pumping tests, and this hydrodynamic coefficient decreases toward the edge of the plain to less than 500 m2 per day. The plain’s typical specific yield is thought to be between 5 and 6%. The research region has 757 agricultural wells, 19 industrial wells (Shazand refinery and power plant), and 36 springs and aqueducts, according to the most recent figures collected in 2009. The primary river that cuts across the plain is a tributary of the Shara river, one of the Qara Chai river’s principal tributaries. This river enters the Komijan plain from the north of the region in the Khandab area and flows the entire length of the range from south to north. The research of the role of extraction wells in the reduction of river discharge, notably in the centre and northern regions of the plain, has always been suggested as one of the difficulties. River leakage into the aquifer increases in volume when the hydraulic gradient between the river level and the groundwater level (GWL) is large, further complicating river-aquifer interactions in the area. In order to replace mathematical models, it is crucial to propose a straightforward yet highly accurate model based on the architecture of artificial intelligence that does not require studying how the river interacts with the aquifer or the use of complicated equations. In this study, the performance of these models is compared to other reliable mathematical models, such GMS, to confirm their suitability (Fig. 1).
Construction of groundwater model
Based on observations of groundwater flow in the Shazand plain, the presumed grid orientation is northward at 250 by 250 m. The resulting model grid consists of 13,189 cells (121 rows and 109 columns) spaced at 250 m apart, with 4382 of those cells being actively used. In this study, the inflow and outflow borders of the Shazand plain are reconstructed using the general head boundary programme. This package’s inflow and outflow are affected by the boundary’s hydraulic gradient and the boundary cell’s conductance. For the bedrock map of the plain, geologists use information from geophysical sections and well logs. Groundwater model upper layer boundaries are also determined by the DEM map of the plain. In order to recreate the functioning wells found in the Shazand Plain, the GMS model makes use of the WELL package7 and is able to identify the well cells. The recharge of the plain is an important part of the groundwater model. Due to factors like soil type, geology, vegetation, precipitation, and topography, groundwater recharge can vary widely. With the RCH package, the GMS model may account for the recharge. The zoning method was used to estimate the hydrodynamic parameters of the aquifer. The zoning of the studied area was done to calculate the hydraulic conductivity and specific yield, based on the logs of observational, exploratory and piezometric wells, as well as geophysical sections prepared from the area. According to the type of soil and sediments of each zone, the initial values of hydraulic conductivity and specific yield were estimated. Finally, in the calibration and validation stages, the optimized value of hydraulic conductivity and specific drainage was taken into account for each zone.
In the groundwater simulation portion, the final zoning of the important model inputs, namely hydraulic conductivity and specific yield, is prepared so that the model can replicate the GWL variations for 6 years in a row. This is necessary in order for the model to be able to do its job. After that, the correctness of the model is examined using calibration and validation procedures that include both steady-state and transient modes of operation. Owing to the fact that we now have data dating back 6 years (October 2015 to September 2021).
Artificial intelligence models
To foresee GWL variations in the Shazand plain, this investigation makes use of both the GMS numerical model and models based on artificial intelligence. We have established this fact at the beginning of this subsection. To take into consideration the complexity of mathematical models, save time, and prevent the need to deal with an excessive amount of data, this is done. For starters, we use information from 21 piezometers over a statistical period of 18.5 years to construct the groundwater unit hydrograph of the Shazand plain. This aids in depicting GWL oscillations throughout the whole plain (April 2003 to September 2021). First, Thiessen Polygons are drawn in a geographic information system, and then the weight of each piezometer is calculated to produce the groundwater unit hydrograph. The resulting groundwater graph is shown in this example. The groundwater unit hydrograph and the water level fluctuations measured by these piezometers are shown in Fig. 2.
When all relevant data has been collected, many models are used to provide GWL predictions over the whole plain. These models include the PSO-ANN, ICA-ANN hybrid, LSTM, LS-SVM, and ORELM. To achieve this, we utilise the GWL values from the most recent month as the model outputs while also considering the properties of the groundwater unit (UH) and precipitation (P) from prior months and with various delays. For a variable number of inputs, the optimal model structure may be attained by splitting the data into a train dataset of 70% and a test dataset of 30%. This produces the highest degree of agreement with the real data and the lowest potential error rate. We choose the model with the best predictions based on the root-mean-square-error, root-mean-square-absolute-error, and correlation coefficient (R) (4). When all other options have been exhausted, the Taylor diagram is used to confirm that the optimal model has been selected. The best-performing model according to three metrics (standard deviation, correlation coefficient, and root mean square error) for evaluating simulation accuracy is shown in this graph (RMSE).
Where\({X}_i^{obs}\) is the ith observation data, \({X}_i^{sim}\) is the ith simulated data, \({X}_{Mean}^{obs}\)and \({X}_{Mean}^{sim}\)are the mean of observed and simulated data, and n is the total number of observations.
Deep learning
Methods of machine learning based on the use of deep neural networks that use existing data to calculate future behaviors and outputs. If we look at this definition, we understand that deep learning is actually one of the methods of machine learning. Deep learning models use several algorithms. Although no network is perfect, some algorithms are better suited for certain tasks. Long short-term memory networks (LSTM), multilayer perceptrons (MLP) and radial basis function networks (RBF) are some examples of deep learning algorithms. One of the limitations of artificial intelligence-based methods is that the length of the time series of data used for the training and test periods must be sufficient so that the results can be provided to the new data. Another limitation is that the input data to the artificial intelligence algorithm must be normal, that is, all the data must be transformed into the interval between zero and one, and after fitting the model, the prediction results are converted from the interval of zero and one into real data (Fig. 3).
Long-short-term memory (LSTM) deep learning model
Deep learning models, which are subsets of artificial intelligence (AI) models, are used to address difficult nonlinear time series issues. For long-term data, the LSTM is a popular deep learning model that can forecast changes in time series based on long-term dependencies within the data. The LSTM’s basic structure is depicted in Fig. 5. The RNN network’s problem with long-term memory is fixed by a particular variety of RNN network called the LSTM network. The “Gate” internal mechanics of the LSTM network. These gates regulate the information flow. Additionally, they outline which sequenced data should be maintained and which should be removed based on importance. In order to create the intended output, it maintains the network of crucial information across the sequence chain.
The LSTM transforms the time series, or input sequence x, into the output sequence y using eqs. 5 and 6. From t = 1 through t = t, this mapping is carried out iteratively. In this mapping, the initial values of C0 and h0 are taken to be zero (Langridge et al. 2020).
Where,
- AL, t:
-
the input vector at time t.
- ht − 1:
-
the hidden layer at time t − 1.
- U:
-
the matrice of weights
- W:
-
connections for input-to-hidden and hidden-to-hidden
- ft:
-
Output vector with range (0, 1)
- σ(·):
-
the kernel function (logistic sigmoid)
- Wf, Uf and bf:
-
the trainable variables set for the forget gate
- \({C}_t^{\%}\):
-
update vector with range (−1, 1)
- tanh (*):
-
the hyperbolic tangent
- \({W}_{C_t^{\%}}\), \({U}_{C_t^{\%}}\),\({b}_{C_t^{\%}}\):
-
other sets of trainable variables
i t denotes the forget gate with the range (0,1). Wi, Ui and biare a set of trainable variables, specified forthe input gate. Eqs. 5 to 7 update the cell state:
where O represents the element-wise multiplication. The output gate is thelast gate that controls the cell state ct.
o tis in the range (0, 1) and Wo, Uo and bo are defined for the output gate (learnable parameters). ht is calculated as follows:
Least squares support vector machines (LSSVM)
In typical SVMs, quadratic programming is used to solve the issue. This approach falls far short of design constrained optimization. The quadratic programming problem is solved using LS-SVM. Instead, it addresses this flaw by employing a set of linear equations. To solve the optimization problem, LS-SVMs use the following basic equation:
Where,
- ei:
-
the regression error for training data(Fig. 4)
- C:
-
the regularization constant (≥0)
- N:
-
training data number
This optimization problem is similar to a regular convex optimization problem. To solve this problem, the method of Lagrange multipliers is used. Eq. (12) has been developed for the Lagrangian with the Lagrange multipliers, ai ∈ R.
The optimal conditions are obtained by differentiating the lagrangian:
In the subsequent linear Karush–Kuhn–Tucker (KKT) system, w and e are replaced in the Lagrangian results:
In this equation, I stands the size N identity matrix, and y = [y1, …, yN]T, 1v = [1, …, 1]T, a = [a1, …, aN]T are N by 1 vector. Moreover, the elementin row k and column i of Ω is computed in terms of thesubsequent equation (Wang and Hu 2005).
According to Mercer’s theorem (Cristianini and Shawe-Taylor 2000), the inner product 〈φ(x), φ(xi)〉 can be specified through a kernel K(x, xi), so Ωki can be expressed as Eq. (18).
Estimating the LSSVM-based function as well as the Gaussian radial basis (RBF) kernel isexpressed in Eq. (16).σ2denotes the kernel parameter, and ai and b are the solutions to Eq. (12).
Outlier robust extreme learning machine (ORELM)
In their 2004 and 2006 presentations, Haung and colleagues introduced a feedforward single-layer neural network called the Extreme Learning Machine (ELM) (Huang and Siew 2004; Huang et al. 2006). The ELM uses an analytical formula and a random number generator to determine the output weights. The basic structure of this approach is shown in Fig. 2a. The main difference between an ELM and a Single Layer Feedforward Neural Network is that the ELM’s output neurons are not biassed (SLFFNN). The following is a mathematical description of a single-layer feed-forward neural network with n hidden nodes.
To be more specific, G(ai, bi, x) is the output of the ith node for the input x, βi is the weight between the ith hidden node and the output node, and ai (\(a_i\;\in R^n\) ) and bi are training factors of hidden nodes. For the additive hidden node G(ai, bi, x), the multi-type activation function g(x) may be expressed as:
Activation functions are used to determine a neuron’s final output response. Activation functions are used to calculate a response after a collection of weighted input signals has been implemented. Figure 5 illustrates the nonlinear activation functions of the ELM. These functions include the step function (hardlim), the sigmoid function (sig), the sinusoidal function (sin), the triangle bias function (tribas), and the radial bias function (radbas).
Outlier data is unavoidable when working with AI-based models; nonetheless, their elimination is not possible since their existence is frequently intrinsic to the issue itself. That’s why it includes some proportion of the overall training error (e). It is the presence of outliers in such data that causes the data to be sparse. Rather than utilising the l2-norm, Zhang and Luo (2015) generated the output weigh matrix (β) by taking the sparsity of the training error (e) into account. This is because they were aware that the l0-norm more accurately captures sparsity.
(β), is the output weights matrix (woor is the same aswoutput).
(or in some references it is presented in this form))wo(is output weights matrix):
As shown above, this is not a convex programming issue. Writing the problem in a manageable convex relaxation form without sacrificing the sparsity feature is a common starting point for analysis of this issue. L1-norm is used to obtain the sparse term. Substituting l0 − norm by l1 − norm ensures the existence of the sparsity characteristic, or the existence of limit events, and leads to the minimising of convexity (a smaller error function) (rare data).
By nature of being a constrained convex optimization problem, the aforementioned equation perfectly suits the domain of the AL multiplier method.
The penalty parameter here μ = = 2N/‖y‖1 implies on the Lagrangian multiplier vector λ∈Rn. By iteratively minimising the following function, we may get the optimal response, represented by (e,), as well as the Lagrangian multiplier vector, denoted by (λ).
PSO-ANN and ICA-ANN hybrid models
To build a fully interconnected network, scientists turn to the multi-layer perceptron (MLP). Unlike single-layer perceptron neural networks, multilayer networks may be taught to handle nonlinear situations and those needing a large number of evaluations. A neural network will have m neurons in its input layer if there are m features in the training data, and the m inputs will be multiplied by n weights W. This product is the neural network’s output. The characteristics of a dataset are the many components that make up the variable that may be changed to affect the outcome. For n hidden layer neurons, you’ll need n sets of weights (W1, W2,..., Wn) to multiply the weights from the X inputs. In order to make an accurate prediction, it is necessary to fine-tune the network at each of its layers by determining the optimal values for its weights. This may be accomplished by following the steps outlined in the previous sentence. The network may be trained in a number of different methods, and the weights can be modified as necessary depending on the situation until a significant mistake is found. Constructing a hybrid model by combining the MLP model with the optimization approach is one of the helpful tactics that can be implemented in this domain. In this study, we make use of two distinct hybrid modeling approaches: PSO-ANN and ICA-ANN. To find the optimal weights, the structures of these models employ the colonial competition and particle swarm optimization algorithms. In order to get optimal performance with these models, the RMSE must be minimised. Weights in the model structure are generated and rectified, and the algorithm’s iteration count is adjusted accordingly, until the minimum error is achieved.
To clarify the work steps for the numerical model and methods based on deep learning, the flowchart of the research steps is shown in Fig. 6.
Results and discussion
Findings from numerical simulations
The groundwater model’s hydraulic conductivity and specific yield are verified to guarantee an accurate representation of the water table before the GWL is modelled. At this point, a statistical comparison between the calculated GWL levels and the actual levels at the site of the observational wells on the Shazand plain is performed using the root mean square error (RMSE). In Fig. 7a, we can see that the value of the index predicted by the steady-state model is very close to 0.7, corroborated by the data collected for this study. The groundwater model was recalibrated and validated over a six-year period, from October 2015 to September 2021 (Fig. 7b), and the results show that the model can accurately reproduce GWL variations due to stresses placed on it, with an RMSE of less than 0.5 when all study months are taken into account.
Artificial intelligence based GWL forecast
Instead of using complex numerical models with huge data volumes, like GMS, this study 18ormaliz artificial neural network methodologies to predict the time series of GWL. The following are a few examples of such models: This is crucial in settings where either there are not enough data points or conditions are already favourable for the development of complex numerical models. Such that changes in the underground water level may be predicted with high precision using a very limited number of input variables. The study objectives cannot be achieved without feeding precipitation and GWL data from the preceding and subsequent months (t-1, t-2, t-3, t-6, t-12, and t-24) into every AI method and hybrid model. Observed data from the piezometers is used to construct the groundwater level (GWL) hydrograph for the current month (t). The model has produced these results. The accuracy with which these models may predict future GWL variations in the Shazand plain is measured using the root-mean-squared error (RMSE), 18ormalized root-mean-squared error (NRMSE), 18ormalized absolute standard error (NASH), and the correlation coefficient. The most salient results that may be gleaned from using these models are shown in Table 1. Table 1 demonstrates that, when all metrics are included, the ORELM model achieves better outcomes than its rivals throughout both the training and testing phases. The LS-SVM model then provides the subsequent degree of accuracy in predictions. Figure 8 shows the point distribution around the Y = X line for the PSO-ANN, ICA-ANN, LSTM, LS-SVM, and ORELM models, along with the squared value of the correlation coefficient for making the optimal AI model selection during the modelling test phase. Using this data, we can choose the most effective AI system. The ORELM model seems to be more reliable since its points tend to congregate along the Y = X line and have a more uniform distribution. In Fig. 9, we can see how the better model’s (ORELM) predicted values for the GWL have evolved over time in contrast to the observed values throughout the train and test phases.
In this research, according to the values of different statistical indicators, the ORELM model had a better performance. But in many researches, choosing the best model based on each statistical index may have different results. For example, one model may be better based on the correlation coefficient index (R), but another model may be selected based on other indices such as RMSE, NASH or NRMSE. This issue has also been seen in many researchs (Zeynoddin et al. 2018; Zeynoddin et al. 2020; Azari et al. 2021; Soltani et al. 2021; Bilali et al. 2021). Therefore, it is difficult and confusing to make a decision based on statistical indicators, which may confuse us in choosing the best model.
In such cases, the researcher selects the superior model based on one or two indicators that have a significant difference compared to other indicators. Another way is to use the Taylor diagram, which simultaneously shows the best model graphically using three indicators of correlation coefficient, standard deviation and RMSE. In this research, Taylor’s diagram was used as a supplementary solution to select the best model.
Choosing the superior model based on Taylor diagram
We are able to increase our chances of selecting the optimal model if we make use of a Taylor diagram. Figure 10 is an illustration of the Taylor diagram, which may be used to assist in making a decision on which of the PSO-ANN, ICA-ANN, LSTM, LS-SVM, and ORELM approaches to use for forecasting the GWL on the plain.
The Centered RMSE calculates the amount of deviation that exists between the point that is observed and the point that is generated by each technique. Compatible models have sets of simulated values that have a standard deviation that is comparable to that of the observed quantities and a coefficient of determination that is equal to one. (Zeynoddin et al. 2018).
The data that were observed for the GWL are denoted by point A, whereas the assessment findings for the models discussed before are indicated by points B, C, D, E, and F. The correlation coefficient and standard deviation are presented below in order to facilitate an analysis of the degree of accuracy achieved by various approaches. This diagram contrasts the suggested method with the PSO-ANN, ICA-ANN, LSTM, and LS-SVM methodologies, as well as the ORELN approach, in order to evaluate how accurately the predictions are made. The distance between the points generated by the different models (B, C, D, E, and F) and the point that was seen (A) is measured. These models are referred to as “point generators.” A model that is consistent with the quantities that have been measured is a collection of points that have a correlation coefficient that is close to 1 and a standard deviation that is comparable to the values that have been measured (Zeynoddin et al. 2018).
The Taylor diagram analysis shows that the PSO-ANN, ICA-ANN, and LSTM, LS-SVM techniques (points B, C, D, and E) are less accurate in forecasting the GWL based on the unit hydrograph of the plain, whereas the ORELM approach (point F) has very little difference with the observed quantities. These results stem from the fact that the ORELM technique shows a negligible dissimilarity to the actual numbers. As a result, the ORELM is connected to the node that is located in the area that is geographically closest to the RP (point E). As a consequence of this, the ORELM technique performs much better than other methods when it comes to predicting the GWL.
Taylor’s diagram, which shows the superior model with more certainty based on 3 different criteria (correlation coefficient, standard deviation and RMSE), has been used in various researches and is suggested as a reliable solution (Nourmohammadi Dehbalaei et al. 2023; Paul et al. 2023; Moradi et al. 2023).
Results from using the ORELM AI model show that this method has the least amount of error in predicting the GWL throughout the statistical period of 228 months. Therefore, the RMSE value for this method is close to 0.6 for the train and test phases combined. When the transient period of 6 years is included in the numerical GMS model, the root-mean-squared error (RMSE) is around 0.4, proving that the ORELM model accurately predicts the GWLvariations without requiring a large amount of data, without employing the challenging modelling procedure based on governing equations, and in a significantly shorter amount of time. It’s important to note that the GMS model’s simulation time is close to 72 months because it needs a lot of data, relevant maps, and not enough data. However, as AI models only use precipitation and groundwater level (GWL) data from piezometers, it is assumed that the length of the prediction period is 228 months. But the main advantage of conceptual models compared to models based on artificial intelligence is that they are able to simulate the distribution groundwater level in the plain.
Conclusion
This paper’s most important conclusion is the possibility of long-term GWL prediction using only piezometric data and precipitation information, a fairly sparse dataset compared to numerical methods. No meteorological factors, soil, geology, stratigraphy, geophysical data, well operating logs, data on the extraction of water from wells, springs, aqueducts, or the interaction of surface and groundwater are used in this prediction of the GWL using artificial intelligence techniques. In addition, the GWL may be predicted without expensive and time-consuming calibration and validation of mathematical models, as well as without the usage of complex maps and software. Water resource professionals will find this to be extremely useful in areas where there are either no available statistics (basins), no available statistics (aquifers), or a lack of essential data and reliable maps (plains). Because using AI models to predict fluctuations in GWL during dry and rainy years requires minimal time or money but can yield tremendously useful management information. Artificial intelligence models (PSO-ANN, ICA-ANN, LSTM, LS-SVM, and ORELN) were shown to be superior than the GMS numerical model in forecasting GWL fluctuations, according to an examination of their performance on Shazand Plain. Results show that ORELM with R, Nash, RMSE and NRMSE values equal to 0.977, 0.955, 0.512 and 0.058 respectively was the best performance in the test stage. After that is the PSO-ANN model. The ORELM model is the most accurate artificial intelligence tool for forecasting the GWL of the Shazand plain, and this was further confirmed by the extended error criteria of the Taylor diagram. The GWL is a key component of the water budget, therefore understanding its dynamics through time is crucial. The deep learning models utilised in this work may be suggested, particularly in areas where fundamental statistics are lacking or mathematical models cannot be used. Base on the results the models developed for this study may be used to future investigations of the interplay between rivers and aquifers in other regions, such as the Shazand Plain, where similar findings have been obtained. How the surface water and groundwater will interact may be predicted with great precision under these conditions. As a result, you won’t have to worry about any intricate equations or connections. One of the most important achievements of using deep learning models to predict the groundwater level in this research is the significant reduction in the time to achieve results compared to numerical simulation methods such as GMS. In these models, there is no need for complex information and various maps and time-consuming simulations.
Data availability
The data will be available upon reasonable request
References
Alizadeh A, Rajabi A, Shabanlou S et al (2021) Modeling long-term rainfall-runoff time series through wavelet-weighted regularization extreme learning machine. Earth Sci Inform 14:1047–1063. https://doi.org/10.1007/s12145-021-00603-8
Azari A, Zeynoddin M, Ebtehaj I, Sattar AMA, Gharabaghi B, Bonakdari H (2021) Integrated preprocessing techniques with linear stochastic approaches in GWL forecasting. Acta Geophysica 69:1395–1411
Azizpour A, Izadbakhsh MA, Shabanlou S, Yosefvand F, Rajabi A (2021) Estimation of water level fluctuations in groundwater through a hybrid learning machine. Groundw Sustain Dev 15:100687
Azizpour A, Izadbakhsh MA, Shabanlou SY, Rajabi F (2022) A simulation of time-series groundwater parameters using a hybrid metaheuristic neuro-fuzzy model. Environ Sci Pollut Res 29:28414–28430
Bayesteh M, Azari A (2021) Stochastic optimization of reservoir operation by applying hedging rules. J Water Resour Plan Manag 147(2):04020099
Bilali AE, Lamane H, Taleb A, Nafii A (2022) A framework based on multivariate distribution-based virtual sample generation and DNN for predicting water quality with small data. J Clean Prod 368:133227
Bilali AE, Taleb A, Brouziyne B (2021) Comparing four machine learning model performances in forecasting the alluvial aquifer level in a semi-arid region. J Afr Earth Sci 181:104244
Cristianini N, Shawe-Taylor J (2000) An introduction to support vector machines and other kernel-based learning methods. Cambridge University Press, Cambridge. https://doi.org/10.1017/CBO9780511801389
Ebtehaj I, Bonakdari H, Shamshiband S (2016) Extreme learning machine assessment for estimating sediment transport in open channels. Eng Comput 32:691–704. https://doi.org/10.1007/s00366-016-0446-1
Ebtehaj, I., Bonakdari, H., Zeynoddin, M., Gharabaghi, B. and Azari, A. 2020. Evaluation of preprocessing techniques for improving the accuracy of stochastic rainfall forecast models. Int J Environ Sci Technol 17, 505–524. https://doi.org/10.1007/s13762-019-02361-z
Erturk A, Ekdal A, Gurel M, Karakaya N, Guzel C, Gonenc E (2014) Evaluating the impact of climate change on groundwater resources in a small Mediterranean watershed. Sci Total Environ 499:437–447
Esmaeili F, Shabanlou S, Saadat MA (2021) Wavelet-outlier robust extreme learning machine for rainfall forecasting in Ardabil City, Iran. Earth Sci. Inform. https://doi.org/10.1007/s12145-021-00681-8
Fleckenstein JH, Krause S, Hannah DM, Boano F (2010) Groundwater-surface water interactions-new methods and models to improunderstanding of processes and dynamics. J Adv Water Resource 33:1291–1295
Goorani Z, Shabanlou S (2021) Multi-objective optimization of quantitative-qualitative operation of water resources systems with approach of supplying environmental demands of Shadegan Wetland. J Environ Manag 292:112769
Graham PW, Andersen MS, McCabe MF, Ajami H, Baker A, Acworth I (2015) To what xtent do long-duration high-volume dam releases influence river–aquifer interactions? A case study in New South Wales, Australia. Hydrogeol J 23:319–334
Guzman SM, Paz JO, Tagert MLM, Mercer AE (2019) Evaluation of seasonally classified inputs for the prediction of daily GWLs: NARX networks vs support vector machines. Environ Model Assess 24(2):223–234
Hu L, Xu Z, Huang W (2016) Development of a river-groundwater interaction model and its application to a catchment in northwestern China. J Hydrol 543:483–500
Huang G-B, Siew C-K (2004) Extreme learning machine: RBF network case. In: ICARCV 2004 8th Control, Automation, Robotics and Vision Conference, Kunming, China, vol 2, pp 1029-1036. https://doi.org/10.1109/ICARCV.2004.1468985
Huang GB, Zhu QY, Siew CK (2006) Extreme learning machine: theory and applications. Neurocomputing 70(1–3):489–501
Irawan D, Puradimaja D, Silaen H (2011) Hydrodynamic Relationshipbetween ManMade Lake and Surrounding Aquifer, Cimahi, Banduge,Indonesia. J World Acad Sci Eng Technol 58:100–103
Ivkovic KM (2009) A top–down approach to characterise aquifer–river interaction processes. J Hydrol 365:145–155
Jalilian A, Heydari M, Azari A, Shabanlou S (2022) Extracting optimal rule curve of dam reservoir base on stochastic inflow. Water Resour Manag 36(6):1763–1782. https://doi.org/10.1007/s11269-022-03087-3
Klove B, Ala-Aho P, Bertrand G, Gurdak JJ, Kupfersberger H, Kværner J, PulidoVelazquez M (2014) Climate change impacts on groundwater and dependent ecosystems. J Hydrol 518:250–266
Lachaal F, Mlayah A, Bedir M, Tarhouni J, Leduc C (2012) Implementation of a 3-D and GIS tools: the Zeramdine-Beni Hassen Mioceneaquifer system (east-central Tunisisa). J Comput Geosci 48:187–198
Langridge M, Gharabaghi B, McBean E, Bonakdari H, Walton R (2020) Understanding the dynamic nature of time-to-peak in UK streams. J Hydrol 583:124630. https://doi.org/10.1016/j.jhydrol.2020.124630
Lemieux J, Hassaoui J, Molson J, Therrien R, Therrien P, Chouteau M, Ouellet M (2015) Simulating the impact of climate change onthe groundwater resources of the Magdalen Islands. J Hydrol 3:400–423
Luo, Y, Sophocleous, M (2011) Tow-way coupling of unsaturated-saturated flow by integrating the SWAT and MODFLOW models with application in an irrigation district in arid region of West China. J Arid Land, 3(3), https://doi.org/10.3724/SP.J.1227.2011.00164
Malekzadeh M, Kardar S, Saeb K, Shabanlou S, Taghavi L (2019a) A novel approach for prediction of monthly ground water level using a hybrid wavelet and non-tuned self-adaptive machine learning model. Water Resour Manag 33:1609–1628. https://doi.org/10.1007/s11269-019-2193-8
Malekzadeh M, Kardar S, Shabanlou S (2019b) Simulation of groundwater level using MODFLOW, extreme learning machine and wavelet-extreme learning machine models. Groundwater for sustainable development. Groundwater for. Sustain Dev 9:100279
Moghadam RG, Izadbakhsh MA, Yosefvand F et al (2019) Optimization of ANFIS network using firefly algorithm for simulating discharge coefficient of side orifices. Appl Water Sci 9:84. https://doi.org/10.1007/s13201-019-0950-8
Mohammed KS, Shabanlou S, Rajabi A, Yosefvand F, Izadbakhsh MA (2023) Prediction of groundwater level fluctuations using artificial intelligence-based models and GMS. Appl Water Sci 13(2):54
Moradi A, Akhtari A, Azari A (2023) Prediction of groundwater level fluctuation using methods based on machine learning and numerical model. J Appl Res Water Wastewater 10(1):20–28
Nadiri AA, Naderi K, Khatibi R, Gharekhani M (2019) Modelling GWL variations by learning from multiple models using fuzzy logic. Hydrol Sci J 64(2):210–226
Nourmohammadi Dehbalaei F, Azari A, Akhtari AA (2023) Development of a linear–nonlinear hybrid special model to predict monthly runof in a catchment area and evaluate its performance with novel machine learning methods. Appl Water Sci 13(5):1–23. https://doi.org/10.1007/s13201-023-01917-2
Pahar G, Dhar A (2014) A dry zone-wet zone based modeling of surface water and groundwater interaction for generalized ground profile. J Hydrol 519(27):2215–2223
Panda DK, Mishra A, Kumar A (2012) Quantification of trends in GWLs of Gujarat in western India. Hydrol Sci J 57(7):1325–1336
Paul A, Afroosa M, Baduru B, Paul B (2023) Showcasing model performance across space and time using single diagrams. Ocean Model 181:102150
Poursaeid M, Mastouri R, Shabanlou S, Najarchi M (2021) Modelling qualitative and quantitative parameters of groundwater using a new wavelet conjunction heuristic method: wavelet extreme learning machine versus wavelet neural networks. Water Environ J 35:67–83
Poursaeid M, Mastouri R, Shabanlou S et al (2020) Estimation of total dissolved solids, electrical conductivity, salinity and groundwater levels using novel learning machines. Environ Earth Sci 79:453
Poursaeid M, Poursaeid AH, Shabanlou S (2022) A comparative study of artificial intelligence models and A statistical method for groundwater level prediction. Water Resour Manag 36:1499–1519
Ramírez-Hernández J, Hinojosa-Huerta Q, Peregrina-Llanes M, Calvo-Fonseca A, Carrera-Villa E (2013) Groundwater responses to controlled water releases in the limitrophe region of the Colorado River: Implications for management and restoration. J Ecol Eng 59:93–103
Samani S, Vadiati M, Delkash M, Bonakdari H (2022) A hybrid wavelet–machine learning model for qanat water flow prediction. Acta Geophysica:1–19
Samani S, Ye M, Zhang F, Pei YZ, Tang GP, Elshall A, Moghaddam AA (2018) Impacts of prior parameter distributions on Bayesian evaluation of groundwater model complexity. Water Sci Eng 11(2):89–100
Shrestha S, Bach TV, Pandey VP (2016) Climate change impacts on groundwater resources in Mekong Delta under representative concentration pathways (RCPs) scenarios. Environ Sci Pol 61:1–13
Soltani K, Azari A (2022) Forecasting groundwater anomaly in the future using satellite information and machine learning. J Hydrol 612(2):128052
Soltani K, Ebtehaj I, Amiri A, Azari A, Gharabaghi B, Bonakdari H (2021) Mapping the spatial and temporal variability of flood susceptibility using remotely sensed normalized difference vegetation index and the forecasted changes in the future. Sci Total Environ 770:145288. https://doi.org/10.1016/j.scitotenv.2021.145288
Todd WR, Kenneth RB (2001) “Report: delineation of capture zones for municipal wells in fractured dolomite”. Sturgeon Bay, Wisconsin, USA. Hydrogeol J 9:432–450
Wang H, Hu D (2005) Comparison of SVM and LS-SVM for regression. In: 2005 International Conference on Neural Networks and Brain, Beijing, pp 279–283. https://doi.org/10.1109/ICNNB.2005.1614615
Xie YC, Shanafield PG, Simmons M, Zheng CT, C. (2016) Uncertainty of natural tracer methods for quantifying river–aquifer interaction in a large river. J Hydrol 535:135–147
Yanxun S, Yuan F, Hui Q, Xuedi Z (2011) Research and Application ofGroundwater Numerical Simulation-A Case Study in Balasu Water Source. Proced Environ Sci 8:146–152
Yosefvand F, Shabanlou S (2020) Forecasting of groundwater level using ensemble hybrid wavelet–self-adaptive extreme learning machine-based models. Nat Resour Res 29:3215–3232
Zampieri M, Serpetzoglou E, Anagnostou EN, Nikolopoulos EI, Papadopoulos A (2012) Improving the representation of river–groundwater interactions in land surface modeling at the regional scale: observational evidence and parameterization applied in the community land model. J Hydrol 420(421):72–86
Zarei N, Azari A, Heidari MM (2022) Improvement of the performance of NSGA-II and MOPSO algorithms in multi-objective optimization of urban water distribution networks based on modification of decision space. Appl Water Sci 12(6):133. https://doi.org/10.1007/s13201-022-01610-w
Zeinali M, Azari A, Heidari M (2020a) Simulating unsaturated zone of soil for estimating the recharge rate and flow exchange between a river and an aquifer. Water Resour Manag 34:425–443
Zeinali M, Azari A, Heidari M (2020b) Multiobjective Optimization for Water Resource Management in Low-Flow Areas Based on a Coupled Surface Water–Groundwater Model. J Water Resource Plann Manag (ASCE) 146(5):04020020
Zeynoddin M, Bonakdari H, Azari A, Ebtehaj I, Gharabaghi B, Madavar HR (2018) Novel hybrid linear stochastic with non-linear extreme learning machine methods for forecasting monthly rainfall a tropical climate. J Environ Manag 222:190–206
Zeynoddin M, Bonakdari H, Ebtehaj I, Azari A, Gharabaghi B (2020) A generalized linear stochastic model for lake level prediction. Sci Total Environ 723:138015
Zhang K, Luo M (2015) Outlier-robust extreme learning machine for regression problems. Neurocomputing 151:1519–1527
Funding
“The authors declare that no funds, grants, or other support were received during the preparation of this manuscript.”
Author information
Authors and Affiliations
Contributions
All authors contributed to the study conception and design. Material preparation, data collection and analysis were performed by All authors. The first draft of the manuscript was written by Saeid Shabanlou and all authors commented on previous versions of the manuscript. All authors read and approved the final manuscript.
Corresponding author
Ethics declarations
Consent for publication
Not applicable
Competing interests
The authors declare no competing interests.
Additional information
Communicated by: H. Babaie
Publisher’s note
Springer Nature remains neutral with regard to jurisdictional claims in published maps and institutional affiliations.
Rights and permissions
Springer Nature or its licensor (e.g. a society or other partner) holds exclusive rights to this article under a publishing agreement with the author(s) or other rightsholder(s); author self-archiving of the accepted manuscript version of this article is solely governed by the terms of such publishing agreement and applicable law.
About this article
Cite this article
Amiri, S., Rajabi, A., Shabanlou, S. et al. Prediction of groundwater level variations using deep learning methods and GMS numerical model. Earth Sci Inform 16, 3227–3241 (2023). https://doi.org/10.1007/s12145-023-01052-1
Received:
Accepted:
Published:
Issue Date:
DOI: https://doi.org/10.1007/s12145-023-01052-1