Abstract
Agricultural carbon mitigation is critical for China to encourage the sustainable development of agriculture and achieve the carbon peak by 2030 and carbon neutrality by 2060. By exploring the impact mechanism of the carbon emission intensity (CEI) of grain production, we can effectively promote the low-carbon transformation of agricultural production and ensure the sustainable development of the food supply. This article analyzes the temporal and spatial evolution of the total carbon emission (TCE) and CEI of staple crops and adopts a dynamic spatial model to explore the influence mechanism and spatial spillover effects of the CEI of grain production based on evidence from China’s major grain-producing provinces from 2002 to 2018. The results indicate that the TCEs of rice, wheat, and maize fluctuate upward and that the CEI in most producing areas decreases with low-low agglomeration (or high-high agglomeration). Among the influencing factors, technology is the main factor reducing CEI. Technical efficiency, urbanization, industrial structure, agricultural agglomeration, and agricultural trade openness can be transmitted to neighboring areas through spatial spillover mechanisms. The spatial spillover mechanisms are resource flow, technology spillover, and policy learning, producing the demonstration effect and siphon effect. Based on our findings, agricultural technology innovation and popularization, urbanization, optimization of the agricultural structure, financial payments, and factor flow among regions should be improved to encourage the low carbon transformation of grain production.
Similar content being viewed by others
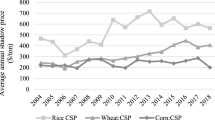
Explore related subjects
Discover the latest articles, news and stories from top researchers in related subjects.Avoid common mistakes on your manuscript.
Introduction
The outbreak of the global food crisis in the 1970s, the reappearance of the international food crisis in 2008, and the food trade protection measures triggered by COVID-19 in 2020 have deepened the reflection on the national food security system. How to use resources efficiently, reduce agricultural carbon emissions (ACE), and increase the sustainability of food production is particularly important (Fan and Brzeska 2014). The agricultural sector interacts with the natural environment, and this industry’s carbon emissions have become an important source of global carbon emissions (Gan et al. 2014; Davis et al. 2015; Dogan et al. 2016). Agriculture accounts for approximately 9–11% of China’s total greenhouse gas emissions (Nayak et al. 2015). China’s traditional food security concept has maximum output as the main goal. The extensive production method simply pursues high grain yields and has seriously damaged the ecological environment, leading to poor sustainability of food security. According to China’s National Bureau of Statistics, China’s grain output increased from 462,175 to 669,490 kt in 2000–2020, which is related to China’s traditional food security concept with the main goal of maximizing production. This extensive mode of grain production leads to increasing energy consumption and carbon emissions. As the most populous country, China needs sustainable food production. The food security concept of sustainable development emphasizes the shift to the enhancement of development capabilities. This shift not only accentuates the improvement in comprehensive food production capacity but also focuses on long-term development, sustainable use of natural resources, and protection of the ecological environment. Therefore, China should analyze the carbon emissions of grain and study its influence mechanism, which would encourage sustainable agricultural development and help achieve the carbon peak by 2030 and carbon neutrality by 2060.
Scholars focus on the production, supply, and consumption of sustainable grain development. Resource utilization (Allouche 2011), modern agrotechnologies (Spiertz 2010), and farming systems (Thomasa et al., 2010; Marra and kaval 2000) are considered to be important issues in planting. In the food supply sector, scholars focus on the sustainability of storage facilities (Essien et al. 2018), the certification of markets (Arthur and Peter 2015), and short food supply chains (Vitters et al. 2019). Some studies also explore the impact of knowledge gaps regarding food safety (Mohammad et al. 2019), risk sharing, and the drivers to participate (Marianne et al. 2017) on shaping sustainable food supply systems. Price (Kaczorowska et al. 2019), visual imperfections (Gracia and Gómez 2020), residents’ sustainable behavior (Bar et al. 2011), food waste-based biogas, and biofertilizers (Hervé Corvellec 2016) are research focuses regarding food consumption. Creating a learning environment, limiting the consumption of ultra-processed foods and guiding the consumption of seasonal, and organic and local production are considered to promote sustainable food consumption (Emma et al. 2013; Fardet and rock 2020).
Scholars have focused on the temporal characteristics of ACE at the national level and the spatial differences in ACE at the regional level. Regarding temporal evolution, scholars have demonstrated that China’s ACE continues to increase (Huang et al. 2019). China’s total ACE in 2016 was 272.022 million tons (Wang et al. 2020). Some scholars suggest that the trend of China’s ACE has an inverted U shape (Liu et al. 2020). Other scholars propose that China’s ACE has experienced three stages: fluctuating growth, slow decline, and new growth (Xu and Bai 2013). Regarding spatial differences, the coupling degree between ACE and agricultural economic growth in central China was higher than that in the western regions (Han et al. 2018). The areas with higher total carbon emissions were concentrated in the central provinces and large agricultural provinces, and the eastern coastal provinces had higher carbon intensity (Bo et al. 2011). A specific analysis of different grain types is required because of their unique growth cycles and farming methods. The carbon emission efficiency of rice showed a rising trend, and the efficiency of single cropping was significantly higher than that of double cropping (Yong et al. 2018). The carbon emissions per unit area of rice and wheat were found to be \(8.80 \pm 5.71\) and \(4.18 \pm 1.13\) t CO2eq/ha, respectively (Kashyap and Agarwal 2021). It was also found that maize not only had higher grain yields but also possessed much smaller carbon emissions than wheat (Hou et al. 2021). Low-carbon agricultural technologies should be adapted to local grain farming methods and form a regionally differentiated system (Xiong et al. 2021).
In addition to measuring ACE, scholars have used empirical methods to investigate the influencing factors of ACE, mainly the resource environment, population, economy, mode of production, and policy systems. Different soil and water resources, land use patterns, weather, and climate conditions have different effects on regional agricultural carbon emissions. The provinces with high soil–water compatibility were more efficient at curbing ACE than those with low soil–water compatibility (Zhao et al. 2018). The replacement of forests by farmland or pastures resulted in significant carbon emissions, which can account for up to 68% of total carbon emissions (Baumann et al. 2016). Additionally, weather and seasonal climate can significantly affect ACE (Bai et al. 2018). Economic and demographic factors are positive determinants of regional ACE (Xiong et al. 2016; Chen et al. 2020). In addition, industrial structure, energy efficiency, and labor transfer have a significant impact on ACE (Li and Zhao 2013). However, scholars have different views on the degree of influence of the population and economy on ACE (Xiong et al. 2020; Liu et al. 2019). Agricultural technology investment can result in widespread influences on ACE (Zhang et al. 2013; Xu et al. 2020), but the results of studies considering the influence direction do not offer a unanimous conclusion (Cui et al. 2018; Zhang and Fang 2013). Fertilizer has been considered the main carbon source of ACE (Yu 2016), and the variation of 92.51% has been explained by the two main factors of average nitrogen application per ha and urbanization rate (Tian et al. 2016). Therefore, effective nitrogen fertilizer management practices should be improved (Wang et al. 2014). Policy and institutional factors are also drivers affecting ACE (Girija et al. 2015). Policy measures to implement land-saving strategies have the technical potential to significantly reduce net ACE (Lamb et al. 2016). The establishment of temporary certified emission reductions could partially internalize the carbon sequestration function of forests, thereby reducing regional ACE (Galinato and Uchida 2010).
Although many studies have achieved positive results in the fields of ACE measurement and influencing factors, the research remains insufficient. Research achievements in estimating the TCE of grain have been observed, but research on the measurement of CEI by considering different grain types remains scant. Many studies have analyzed the influence mechanism and spatial effect of TCE on grain production. However, there is a gap in analyses on the influence mechanism and spatial effect of the CEI. To promote the low carbon transformation of grain production and ensure the sustainability of grain production, it is important to explore the influence mechanism of the CEI of different types of grain. This paper differs from existing studies in two respects. First, this paper uses China’s rice, wheat, and maize as examples, improves upon the calculation method of grain carbon emissions used in the literature, more accurately measures the TCE and CEI of grain production, and analyzes the evolution of temporal and spatial patterns. Rice, wheat, and maize are the three major grain varieties in China and are widely distributed in most provinces of China. According to China’s National Bureau of Statistics in 2020, the output of rice, wheat, and maize were 211,860 kt, 134,250 kt, and 260,670 kt, respectively, accounting for 31.64%, 20.05%, and 38.94% of China’s total grain output,. Second, this paper uses spatial econometrics methods to analyze the influence mechanism and spatial spillover effect of the CEI of grain production. In the context of China’s food security policy, there is a Kuznets curve effect between grain production and carbon emissions (environmental Kuznets curve, EKC); that is, the increase in grain production leads to an increase in TCE in the short term. As it studies the influence mechanism of the CEI of grain production rather than the TCE, the study is more in line with the development concept of promoting low carbon transformation and ensuring the sustainability of grain production. Therefore, this paper divides grain crops into rice, wheat, and maize; calculates the carbon emissions of grain types in their production area; depicts their spatial and temporal evolution characteristics; and focuses on the influence mechanism and spatial spillover effect. This study will facilitate the creation of a set of more informed adaptation policies of agricultural emission reduction for different categories and regions.
The rest of this paper is arranged as follows. The next section presents the methodology, data, and econometric model. The “Empirical results” section empirically analyzes the temporal and spatial evolution of the total carbon emission (TCE) and CEI of staple crops and adopts the dynamic spatial model to explore the influence mechanism and spatial spillover effects of the CEI of grain production based on the evidence from China’s major grain-producing provinces in 2002–2018. The last section is the discussion and policy implications.
Methodology and data
Total carbon emissions and carbon emissions intensity
The main sources of TCE include carbon sources from grain production and CH4 emissions from rice planting (Liu et al. 2020; Min and Hu 2012). The carbon sources from grain production include the consumption of chemical fertilizers, pesticides, plastic films, and diesel fuels, as well as agricultural irrigation and plowing activities (Wu et al. 2020; Liu et al. 2018; Zhang et al. 2020). Therefore, the TCE of grain production can be expressed as:
where \(E_{i}^{tol}\) is the TCE of grain \(i\) (include wheat, maize, and rice); \(E_{i,\gamma }\) is the carbon emission of carbon source \(\gamma\); \(T_{i,\gamma }\) is the amount of carbon source \(\gamma\); \(\delta_{i,\gamma }\) is the carbon emission coefficient of carbon source \(\gamma\); \(\gamma\) consists of four elements: fertilizer, agricultural plastic film, diesel fuel, and plowing; \(E_{i}^{ACH}\) represents the pesticide consumption carbon emissions; \(E_{i}^{ICR}\) represents the carbon emissions of agricultural irrigation; \(E_{{CH_{4} }}\) represents the carbon emissions of \(CH_{4}\) from rice planting; \(\lambda_{i}^{{CH_{4} }}\) is selectivity coefficient, \(\lambda_{i}^{{CH_{4} }} = 1\) (\(i\) represents rice), or \(\lambda_{i}^{{CH_{4} }} = 0\) (\(i\) represents wheat or maize). The carbon emission coefficient of chemical fertilizer consumption is 0.8956 kg/kg (Oak Ridge National Laboratory, ORNL); the carbon emission coefficient of plastic film consumption is 5.1800 kg/kg (Institute of Resource, Ecosystem and Environment of Agriculture, IREEA); the carbon emission coefficient of diesel fuel consumption is 0.5927 kg/kg (IPCC); and the carbon emission coefficient of plowing is 3.1260 kg/hm2 (College of Agronomy and Biotechnology, China Agricultural University, CAB). Based on the classification of grain, this paper calculates the carbon emissions of rice, wheat, and maize in each main production province, respectively.
In particular, this paper focuses on the carbon emission efficiency of the consumption of resources (such as fertilizers, pesticides, diesel, plastic film, plowing, and electricity) and the impact mechanism in grain production, rather than life cycle carbon footprint measurement (excluding the biological carbon sequestration of different food varieties). When calculating the carbon emissions of different grain varieties (wheat, rice, and maize), this paper draws on the research approaches of Zhang et al. (2019) and Liu and Yang (2021) and mainly examines the carbon emissions caused by the consumption of resources, such as fertilizers, pesticides, diesel, plastic film, plowing, and electricity, in grain production. It is assumed that these resources have a fixed carbon emission coefficient when they are provided. Therefore, this paper adopts the same emission coefficient when examining the temporal and spatial differences in carbon emission efficiency and the mechanism of grain production.
This paper uses Francesco et al. (2012) and Dumortier and Elobeid (2021) to calculate the carbon emissions intensity of agricultural production and uses the carbon emissions per unit grain production to express the carbon emissions intensity:
where \(CEI_{i}\) represents the carbon emissions intensity of grain \(i\); \(Y_{i}\) represents the production of grain \(i\).
The carbon emissions of pesticide consumption can be expressed as
where \(E_{ij}^{ACH}\) represents the pesticide consumption carbon emissions of grain \(i\) in \(j\) province; \(COS_{ij}^{CH}\) is the pesticide cost per ha of grain \(i\) in \(j\) province; \(\overline{P}_{ij}^{CH}\) represents the pesticides average price of grain \(i\) in \(j\) province; \(ARE_{ij}\) represents the planting area of grain \(i\) in \(j\) province; and \(\delta^{ACH}\) represents the carbon emission coefficient of pesticide consumption, which is 4.9341 kg/kg (ORNL).
The carbon emissions of agricultural irrigation can be expressed as
where \(E_{ij}^{IRC}\) represents the carbon emissions of agricultural irrigation; \(EIR_{ij}\) represents the electricity consumption for agricultural irrigation of grain \(i\) in \(j\) province; \(COS_{ij}\) is the agricultural irrigation cost per ha; \(WAR_{ij}\) is the agricultural water cost per ha; \(PEL_{j}\) represents the average cost of electricity for agricultural irrigation; \(IRC_{ij}\) represents the carbon emissions for agricultural irrigation of grain \(i\) in \(j\) province; \(PV_{j}\) represents the proportion of thermal power generation; \(\delta_{ce}\) represents the carbon emissions coefficient of coal equivalent, with the value of 0.69 kg/kg (EIA); \(\partial^{IRC}\) is the conversion coefficient between electric power and coal equivalent, with the value of 0.1229 kgce/kWh, which comes from China Electric Power statistical yearbook; \(ARE_{ij}\) represents the planting area of grain \(i\) in \(j\) province.
The carbon emissions of \(CH_{4}\) from rice planting can be expressed as:
where \(E_{{CH_{4} }}\) represents the carbon emissions of \(CH_{4}\) from rice planting; \(\kappa\) is the mass specific gravity of \(C\) atom in \(CH_{4}\), which is 0.75; \(\delta_{j}^{{CH_{4} }}\) represents the carbon emissions coefficient of \(CH_{4}\), and the data derived from the study of Min and Hu (2012), showed in Table 1.
Spatial panel model
Before building a spatial model for empirical analysis, we should measure the spatial autocorrelation of variables at first. When measuring spatial autocorrelation, the Global Moran Index (GMI) is used to analyze the overall spatial agglomeration, while the Local Moran Index (LMI) focuses on the spatial agglomeration around a region. The GMI can be expressed as:
\(S_{0} = \sum\nolimits_{i = 1}^{n} {\sum\nolimits_{j = 1}^{n} {w_{i,j} } }\); \(Z_{I} = \frac{I - E\left[ I \right]}{{\sqrt {V[I]} }}\).
\(E\left[ I \right] = {{ - 1} \mathord{\left/ {\vphantom {{ - 1} {\left( {n - 1} \right)}}} \right. \kern-\nulldelimiterspace} {\left( {n - 1} \right)}}\); \(V\left[ I \right] = E\left[ {I^{2} } \right] - E\left( I \right)^{2}\).where \(w_{i,j}\) represents the spatial weight. The value range of GMI is \(\left[ {{ - }1,1} \right]\), greater than zero means positive correlation, less than zero means negative correlation, equal to zero means no correlation, close to 1 means the same attribute aggregation, and close to − 1 means different attribute aggregation. The LMI can be expressed as
where \(w_{i,j}\) represents the spatial weight. The LMI greater than zero indicates that the high value (low value) of the area is surrounded by the surrounding high value (low value), and it also means that it is surrounded by the same attribute. The LMI less than zero means that the high value (low value) of the area is surrounded by the surrounding low value (high value), and it also means that it is surrounded by different attributes.
When constructing the spatial panel model, the ordinary static spatial model ignores the lag effect of the dependent variable in the previous period. In fact, the current results of the CEI of grain production are often affected by the carbon emissions level of the previous period, and this effect always has Matthew effect. Therefore, based on the research of Elhorst et al. (2012), this paper constructs a spatial panel model including dynamic effects:
where \(X_{i,j,t}\) is the factor \(j\) in a module of area \(i\); \(w_{i}\) is the i-th row of the spatial weight matrix \(W\), and \(W\) includes distance weight, economic weight, and carbon emissions weight; \(\eta_{t}\) represents the time effect; \((\mu_{i} + \varepsilon_{it} )\) represents the compound interference terms; and \(m_{i}\) represents the i-th row of spatial weighting matrix \(M\) of compound interference terms.
When \(\tau = {0}\) and \(\phi = {0}\), Eq. 9 is a static spatial panel model; if \(\lambda = {0}\), Eq. 9 becomes a spatial Durbin model (SDM); if \(\lambda = {0}\) and \(\delta = {0}\), Eq. 9 becomes a spatial autoregressive model (SAR); if \(\tau = {0}\) and \(\delta = {0}\), Eq. 9 becomes a spatial autocorrelation model (SAC); and if \(\tau = \rho = {0}\) and \(\delta = {0}\), Eq. 9 becomes a spatial error model (SEM).
Geographical distance weight matrix can be expressed as
where \(d_{ij}\) represents the geographical distance between \(i\) and \(j\), which measured by Euclidean distance between the provincial capital cities (Zhang et al., 2020), and coefficient \(\alpha\) (the reciprocal of the minimum distance between provincial capital cities) is used to quantify the variables without dimension, and the measurement unit has no influence on the empirical results.
Economic weight matrix can be expressed as:
where \(\overline{Y}_{i}\) represents the average GDP of geographical unit \(i\). During 2002–2018; \(\overline{Y}\) represents the average GDP of all regions.
Carbon emissions weight matrix can be expressed as
where \(\overline{R}_{i}\) represents the average carbon emission of grain production of geographical unit \(i\) during 2002–2018; \(\overline{R}\) represents the average carbon emission of grain production of all regions.
Variable selection and data sources
Analysis of influencing factors
Production technology is a key factor affecting ACE (Zhang et al. 2013; Xu et al. 2020; Cui et al. 2018; Zhang and Fang 2013); therefore, the technical efficiency of grain production (PE) is used. Using grain planting area, direct cost, indirect cost, and labor quantity as four input indices and grain output as the output index, the production technical efficiency of rice, wheat, and maize is calculated. This paper uses the EBM model to measure technical efficiency. Tone and Tsutsui (2010) proposed an epsilon-based measure (EBM) model containing both radial and nonradial distance functions. Under the assumption of constant returns to scale, the input-oriented EBM model is as follows:
where \(\gamma^{*}\) represents the PE under the EBM model; \(x_{ik}\) and \(y_{rk}\) represent the \(i\) input and the \(r\) output of the \(k\) decision-making unit, respectively; \(m\) and \(s\) represent the number of inputs and outputs, respectively; \(\lambda\) represents the linear combination coefficient of the decision-making unit; \(\theta\) represents the planning parameter of the radial part; \(S_{i}^{ - }\) represents the relaxation value of input elements; \(w_{i}^{ - }\) represents the relative importance of each input index and meets \(\sum\nolimits_{i = 1}^{m} {w_{i}^{ - } } = 1\), \((w_{i}^{ - } \ge 0)\); \(\varepsilon_{x}\) represents the importance of the nonradial part in the efficiency value calculation; and \(0 \le \varepsilon_{x} \le 1\). The advantage of this model is that it relaxes the assumption of “factor input changes in the same proportion” in the traditional data envelopment analysis (DEA) method, making the measurement results more realistic.
Some studies have shown that economic factors are significant influencing factors of ACE (Xiong et al. 2016). Therefore, per capita agricultural output value (PAV) and agricultural trade openness (ATO) are used and calculated by dividing the total agricultural output value by the number of employees in the primary industry and the proportion of the actual total agricultural import and export in the agricultural added value, respectively.
Per capita agricultural output value (PAV):
where \(AV_{i}\) represents total agricultural output value of region \(i\); \(NFIE_{i}\) represents the number of employees in the primary industry of region \(i\).
Agricultural trade openness (ATO):
where \(TAT_{i}\) represents the gross value of grain import and export and \(ADA_{i}\) represents the added value of grain industry.
Because urbanization and industrial structure have significant impacts on ACE (Liu et al. 2019), the urbanization level (UL), grain industrial structure (INS), and grain production agglomeration degree (ADG) are used and are calculated by the proportion of the urban population in the total population, the proportion of planting output value in the total output value of agriculture, and the location quotient method, respectively.
Urbanization level (UL):
where \(UP_{i}\) represents the urban population and \(TP_{i}\) represents the total population.
Grain industrial structure (INS):
where \(PIV_{i}\) represents the grain output value and \(AV_{i}\) represents total agricultural output value.
Agglomeration degree of grain production (ADG):
where \(PIV_{i}\) represents the grain output value of region \(i\); \(GDP_{i}\) represents the GDP of region \(i\);\(PIV_{T}\) represents the grain output value of all regions; and \(GDP_{T}\) represents the GDP of all regions.
Studies have shown that weather and seasonal climate change can significantly affect ACE. Therefore, the proportion of agricultural disaster area (DSA) is used and calculated by the proportion of disaster area in the sown area of grain.
Proportion of agricultural disaster area (DSA):
where \(SA_{i}\) represents the agricultural disaster area and \(PA_{i}\) represents the agricultural planting area.
In addition, policy and institutional factors affect ACE (Girija et al. 2015); thus, this paper uses the proportion of agricultural financial expenditure (AFP) and the proportion of financial expenditure on environmental protection (EFP) as variables, which are calculated by the proportion of agricultural financial expenditure in the total regional financial expenditure and the proportion of financial expenditure on environmental protection in the total regional financial expenditure, respectively.
Proportion of agricultural financial expenditure (AFP):
where \(AF_{i}\) represents the agricultural financial expenditure of region \(i\) and \(TF_{i}\) represents the total financial expenditure of region \(i\).
Proportion of financial expenditure on environmental protection (EFP):
where \(EF_{i}\) represents the financial expenditure on environmental protection of region \(i\) and \(TF_{i}\) represents the total financial expenditure of region \(i\).
Impact mechanism
This study selects the following factors to explain the impact mechanism of CEI (Fig. 1). These factors can influence the CEI directly or through spatial spillover effects. First, we analyze the direct impact of various factors on the CEI. The improvement in technical efficiency improves the efficiency of input factors, reduces their input amount, and reduces carbon emission intensity. A decrease in agricultural disaster area means an increase in effective production and leads to a reduction in the CEI of these regions. The improvement in agricultural trade openness means increasing the import of grain from countries with high agricultural production efficiency. On the basis of comparative advantage theory, importing grain from countries with comparative advantages in production is conducive to reducing China’s grain carbon emission intensity. The improvement in agglomeration means that the agricultural structure tends toward grain planting. In these areas, specialized production forms the accumulation and innovation of grain production technology, reducing the carbon emission intensity. The improvement in the grain industrial structure leads to an increasing proportion of the planting industry in the total agricultural output value, and the intensive tendency of agricultural production produces economies of scale to improve the efficiency of resource utilization. Therefore, the innovation and application of grain production technology can focus on grain planting and improve the efficiency of resource utilization, reducing carbon emissions intensity. The increase in agricultural output per capita means that the demand for low carbon development is increasing, which promotes the application of agricultural low carbon technology and thus reduces the carbon emission intensity of grain. With the advancement of urbanization and the reduction in rural labor, agricultural scale management has become a realistic choice. The efficient utilization of agricultural inputs has promoted the reduction of grain carbon emission intensity in this period. The increase in agricultural financial expenditure can increase the funds for agricultural production and significantly improve the agricultural production infrastructure and equipment to reduce the carbon emission intensity of grain. The increase in financial expenditure on environmental protection can reduce CEI by increasing investment in the research and development (R&D) of low-carbon technology and increasing the institutional cost of carbon emissions.
These factors also have significant spatial spillover effects. They can be transmitted to neighboring areas through spatial spillover mechanisms, for example, factor flow, technology spillover, policy learning, and the demonstration effect (positive spatial spillover effect) and siphon effect (Zhang et al. 2020). Specifically, higher technical efficiency, through the mechanisms of technology spillover and policy emulation, can pass the advanced technology of breeding, planting, purchasing, storage, and processing to areas with small economic gaps, and this approach promotes the optimization and upgrading of production equipment, technology, and management in these areas to reduce their CEI. When the industrial agglomeration degree of grain is relatively high, it reflects the high level of specialization of grain production in the region, and it can attract the related resources in the surrounding areas and eventually increase the surrounding areas’ CEI through resource flow. A higher level of economic development means a better working environment and salary conditions, stronger consumer demand, employment opportunities, and investment opportunities; it can further attract capital, talent, technology, data, and other production factors in the surrounding areas, thereby increasing the surrounding areas’ CEI. Policy is one of the important tools for the government to intervene in the development of industries. The improved agricultural emission reduction policies implemented in some provinces can play a role in inducing agricultural production entities to adopt green production technologies, thereby promoting green agricultural development; this policy experience is then recognized by other regions and incorporated into the regional policy of agricultural emission reduction. It creates a spillover effect of the supporting policy.
After selecting the influencing factors of CEI, the dynamic spatial panel model of the CEI of grain production can be expressed as
Data sources
In this paper, rice, wheat and maize were selected as the research objects, and the data were taken from 2002 to 2018. The rice, wheat, and maize TCEs and CEIs in 31 Chinese provinces (excluding Hong Kong, Macao, and Taiwan) were measured. Then, three main grain-producing areas (Table 2) were selected to build a dynamic spatial model to explore the influence mechanism and spatial spillover effect of the CEI of grain production. Among the variables involved in this paper, the data of grain planting area, grain output, total population, urban population, agricultural disaster area, regional financial expenditure, regional agricultural financial expenditure, and regional financial expenditure on environmental protection are from the National Bureau of Statistics of China. The data of direct cost, indirect cost, labor quantity, agricultural plastic film consumption, fuel cost, fertilizer consumption, pesticide cost, total irrigation cost, and irrigation water cost are from the Compilation of Cost–Benefit Data of Agricultural Products in China. The data of total agricultural output value and the number of employees in the primary industry come from the statistical yearbooks of various provinces. The grain output value and food output value data are from the China Rural Statistical Yearbook. The data on the import and export of grain and agricultural products are from the database of the General Administration of Customs of China. The guidance for the use of pesticides in rice, wheat, and maize is from the Ministry of Agriculture and Rural Affairs of China, and the price data of various pesticides are from Shijinongyao (website: www.nongyao001.com). The electricity price data for agricultural irrigation are from the State Grid Corporation of China (website: www.sgcc.com.cn). The diesel price data are from the Wind database.
The price of pesticides is adjusted by the price index of pesticides and their means of production to obtain the current price of each year. The electricity price for agricultural irrigation is adjusted according to the electricity consumption price index of rural residents, and the current price of each year is obtained. The direct cost and indirect cost of grain production were reduced by the price index of agricultural production based on 2002. Other data are original data.
Empirical results
Analysis of the temporal and spatial pattern evolution of grain carbon emissions
From 2002 to 2018, the TCE of rice, wheat, and maize increased from 32,365.920 to 45,558.268 kt. Specifically, the TCE increased rapidly in 2008–2015 with an annual growth rate of 4.594%, and the TCE fell back to 45,000 kt in 2015–2018. The evolution of carbon emissions is analyzed in the following sections.
Evolution of rice carbon emissions
From 2002 to 2018, China’s TCE of rice increased from 16,158.459 to 17,938.854 kt, and the agglomeration area of TCE shifted from Central and South China to Central and Northeast China (Fig. 2 a and b). In 2018, the top five provinces in terms of the TCE from rice production were Jiangxi, Hunan, Jiangsu, Heilongjiang, and Anhui; their combined carbon emissions accounted for 53.859% of China’s rice TCE. From 2002 to 2018, the CEI of rice in more than 70% of the provinces showed a decreasing trend. In 2018, the spatial pattern of CEI showed a trend of decreasing from coastal areas to inland areas. The CEI of the main producing areas in Central China and South China was significantly higher than that of the main producing areas in Northeast China. From 2002 to 2018, the CEI of Heilongjiang decreased the most, from 80.943 to 60.010 kg/t; the CEI of Jiangxi had the smallest change, with a reduction of 5.310 kg/t; the CEIs of Jiangsu and Anhui were relatively high and increased by 9.748 kg/t and 9.287 kg/t, respectively. The calculation results show that the TCE and CEI of the main producing areas in Central China, East China, and South China are relatively high, while the main production areas in Northeast China have relatively high TCE and low CEI.
Evolution of wheat carbon emissions
From 2002 to 2018, China’s TCE of wheat increased from 8384.411 to 10,396.460 kt, and the agglomeration area of TCE was in the Huang-Huai-Hai Plain and Northwest China (Fig. 3 a and b). In 2018, the top five provinces in terms of the TCE from wheat production were Henan, Shandong, Hebei, Anhui, and Jiangsu, and their combined carbon emissions accounted for 65.901% of China’s wheat TCE. From 2002 to 2018, the CEI of wheat decreased in more than 60% of the provinces. In 2018, the spatial pattern of CEI was high in the north and south and low in the middle. The CEI of the main producing areas in the Huang-Huai-Hai Plain (3H Plain), Sichuan, Shaanxi, and Hubeiwas lower than that of South China and the main producing area in Northeast China. The CEI of Henan was the lowest, 62.191 kg/t in 2018, which was at a low level from 2002 to 2018. The CEI of Hebei decreased the most, from 112.920 to 88.099 kg/t. The calculation results demonstrate that the TCEs of the main producing areas in Northeast Chinaare low and the CEIs are relatively high, while the main producing areas in the Huang-Huai-Hai Plain have relatively high TCE and low CEI.
Evolution of maize carbon emissions
From 2002 to 2018, China’s TCE of maize increased from 7823.050 to 17,222.954 kt, and the agglomeration area of TCE was in Northeast and North China (Fig. 4 a and b). In 2018, the top five provinces in terms of the TCE from maize production were Heilongjiang, Inner Mongolia, Jilin, Shandong, and Henan, and their combined carbon emissions accounted for 51.106% of China’s maize TCE. In 2018, the spatial pattern of CEI showed the characteristic of I-shaped prominence, and the CEI of Northeast, Southwest, and North China was lower than that of Southeast, Central, and Northwest China. From 2002 to 2018, the ECI of Heilongjiang was at a low level (54.011 kg/t in 2018), decreasing by 2.791 kg/t. From 2002 to 2018, the CEI of Shandong decreased by 8.977 kg/t, but the CEI of Inner Mongolia was relatively high, with an increase of 0.964 kg/t. The calculation results demonstrate that the TCE and CEI of the main producing areas in Northwest China are relatively high and that the main producing areas in Northeast and North China have relatively high TCE and low CEI.
Analysis of impact factors on carbon emission intensity
Before using the spatial panel model for empirical analysis, the spatial correlation of the CEI of rice, wheat, and maize in China is tested. In this paper, the spatial correlation is tested by using the GMI, and the results are shown in Table 3. Under the distance matrix, economic matrix, and carbon emission matrix, the spatial correlation of the rice, wheat, and maize CEIs is significant. Grain planting depends on regional agricultural resources, showing a significant spatial agglomeration effect. Grain carbon emissions are the unexpected output of grain planting, so they are also likely to show a strong spatial correlation. Therefore, this paper builds the spatial panel model of the distance matrix, economic matrix, and carbon emission matrix for analysis.
When the spatial panel econometric analysis is conducted, the spatial panel model should be selected. This paper draws on the testing method of Elhorst (2014) to assess the form of the spatial panel model (SAR, SAC, SEM, SDM) and determines whether it has fixed effects or random effects according to the Hausman test (Table 4). Therefore, the dynamic SDM is used for the rice, wheat, and maize CEIs. With the help of Stata software, this paper analyses the factors and spillover effects of the CEIs of rice, wheat, and maize through a distance matrix, economic matrix, and carbon emission matrix (Table 5).
The estimated results of the rice model demonstrate that the spatial autoregressive coefficients are significant (1% level), indicating that the rice model has a significant spatial autoregressive effect in the distance matrix, economic matrix, or carbon emission matrix (Table 5). Considering \(R^{2}\) and log-likelihood, the dynamic SDM under the economic matrix is used to analyze the factors and spillover effect on rice’s CEI. With the same judgment basis, the dynamic SDM under the economic matrix is also used to analyze wheat and maize (Table 5).
Technical efficiency has a meaningful negative impact on the CEI of grain production, and this negative impact is the largest, reflecting that improving technical efficiency is the most important factor in reducing the CEI (Table 5). The improvement in technical efficiency is manifested in the application of advanced production technology and the management mode, which improves the efficiency of input factors, reduces their input amount, and reduces the CEI. Regarding the difference in technical efficiency, the improvement in technical efficiency had the largest negative impact on the CEI of maize (− 4.3485), followed by rice (− 2.1682), and the smallest negative impact on wheat (− 2.1489). The policies implemented by the Chinese government, such as the temporary storage system of maize (2008–2015), improved seed subsidies, and agricultural machinery purchase subsidies, encouraged farmers to adopt new technologies, equipment, and processes. For instance, the mechanization levels of Heilongjiang and Jilin are significantly higher than those of other parts of the country. Because the improvement in mechanization level increases technical efficiency, the CEI of maize in these areas is low. Additionally, the Chinese government has implemented the reform of “market pricing + subsidy” for maize since 2016, which has gradually increased the impact of the market on maize production. Under market regulation, the development of green maize planting and silage technology shortens the planting cycle of maize and improves the utilization rate of maize plants, thus reducing the CEI of maize.
Agricultural output per capita has a negative impact on the CEI of grain production. The increase in agricultural output per capita means that the demand for low carbon development is increasing, which promotes the application of agricultural low carbon technology and thus reduces the CEI of grain production. The increase in agricultural output per capita had the largest negative impact on maize (− 1.4945), followed by rice (− 0.8714), and the smallest negative impact on wheat (− 0.7930). Inner Mongolia, Gansu, Xinjiang, Yunnan, and other western provinces that produce maize have a worse ecological environment; thus, they must pay additional attention to the protection of the agricultural ecology. This causes a more extensive application of low carbon technology in agriculture, which leads to the greatest negative impact on the CEI of maize.
The urbanization level has a negative impact on the CEI of grain production. With the advancement of urbanization and the reduction in rural labor, agricultural scale management has become a realistic choice. The efficient utilization of agricultural inputs has promoted the reduction of grain CEI in this period. The increase in urbanization had a meaningful negative impact on the CEI of rice (− 2.6343), but the impact on wheat was positive (4.4104), and the impact on maize was not significant. Jiangxi, Hunan, Jiangsu, Heilongjiang, and other main rice-producing provinces in China’s eastern region have a high urbanization rate, while Henan, Hebei, Anhui, and other main wheat-producing provinces in China’s central region have a low urbanization rate. Therefore, urbanization has different effects on regions in different stages.
The proportion of agricultural disaster area has a positive impact on the CEI of grain production. An increase in agricultural disaster area means a decrease in effective production area; thus, the regions implement necessary measures to increase the expected yield per unit. The excessive use of agricultural resources then occurs, which leads to an increase in the CEI of these regions. The increase in agricultural disaster area had the greatest positive impact on rice (0.2785), followed by wheat (0.1329) and maize (0.0571). Because the Yangtze River Basin is often affected by floods, the southeast coast is often affected by typhoons, and the northeast is often affected by freezing, and the rice cultivation in these areas is more affected by disasters. Therefore, the agricultural disaster area has the largest impact on rice.
The grain industrial structure has a negative impact on the CEI of grain production. The improvement in the grain industrial structure means an increasing proportion of the planting industry in the total agricultural output, and the grain tendency of agricultural production is obvious. Therefore, the innovation and application of grain production technology can focus on grain planting and improve the efficiency of resource utilization, reducing carbon emissions intensity. The improvement in grain industrial structure had the greatest negative impact on rice (− 1.9786), but the impact on wheat and maize was not significant. Because the agricultural production in rice-producing areas is mainly oriented to grain, the CEI of grain can be significantly reduced through the accumulation of agricultural production technology.
Agricultural trade openness has a negative impact on the CEI of grain production. China mainly imports grain from the USA, Ukraine, Canada, and other countries with high agricultural production efficiency. On the basis of comparative advantage theory, importing grain from these countries is conducive to reducing China’s grain CEI. The increase in agricultural trade openness had the greatest negative impact on maize (− 3.1013), followed by rice (− 1.9919), but the impact on wheat was not significant. The reason for this finding is that, on the one hand, the proportion of wheat imports relative to the total output is low, and the increase in imports cannot significantly reduce the CEI of wheat. The main wheat-producing areas belong to Central China, and the provinces that import wheat are, for example, Beijing, Guangdong, Fujian, and Zhejiang. The mismatch between grain production and trade leads to the insignificant impact of agricultural trade openness on wheat.
The agglomeration of grain production has a negative impact on the CEI of grain production. The improvement in agglomeration means that the agricultural structure tends toward grain planting. In these areas, specialized production forms the accumulation and innovation of grain production technology, reducing the CEI. The increasing agglomeration of grain production had the greatest negative impact on rice (− 1.8831), followed by the negative impact on maize (− 1.1678), and the least negative impact on wheat (− 0.7946).
Agricultural financial expenditure has a negative impact on the CEI of grain production. The increase in agricultural financial expenditure can increase the funds for agricultural production and significantly improve the agricultural production infrastructure and equipment, reducing the CEI of grain. The increase in agricultural financial expenditure had a significant negative impact on the CEI of rice (− 0.0610), but the impact on wheat was positive (0.2968), and the impact on maize was not significant. Since 2005, the minimum purchase price policy of wheat has been implemented, which is beneficial to increasing grain yield and farmers’ income and cannot facilitate an increase in productivity (Tong et al. 2019; Jia et al. 2019). The improved seed subsidies and agricultural machinery purchase subsidies for rice improve production efficiency. Therefore, agricultural financial expenditure has different effects on rice and wheat. The proportion of financial expenditure directly related to maize production is relatively low; thus, the impact of agricultural financial expenditure on maize is not significant.
Financial expenditure on environmental protection has a negative impact on the CEI of grain production. The increase in financial expenditure on environmental protection can reduce the CEI of grain by increasing the investment in the research and development (R&D) of low carbon technology and increasing the institutional cost of carbon emissions. The increase in financial expenditure on environmental protection had the greatest negative impact on maize (− 1.1392), followed by rice (− 1.1041) and wheat (− 0.2953). The main maize-producing areas belong to Central and Western China. In these areas, the environment and resources are worse, and low carbon agricultural technology is more widely used than in other areas, leading to the greatest negative impact on maize. The regions with high CEIs of wheat include Shanxi, Jilin, and Fujian. The financial expenditure on environmental protection of these provinces is low; thus, the impact on wheat is not significant.
In addition, the lagged CEI of grain production CEI (− 1) had the greatest positive impact on wheat (0.8786), followed by maize (0.2041) and rice (0.1185). The difference in the natural endowment of resources and the growth attributes of grain, shown by CEI (− 1), helps explain why the CEI of wheat is higher than that of rice and maize.
Spatial spillover effect analysis
Although the influencing factors directly affect the intensity of grain carbon emissions, they can also be transmitted to neighboring areas through spatial spillover mechanisms, for example, factor flow, technology spillover, and policy learning and the demonstration effect (positive spatial spillover effect) and siphon effect (Zhang et al. 2020). In this paper, the partial differential effect decomposition method is used to calculate the direct effects and spillover effect of influencing factors on the CEI of rice, wheat, and maize (Table 6).
From the perspective of the spatial effect decomposition of the factors affecting the CEI of rice, technical efficiency has a demonstration effect, and urbanization, grain industrial structure, and agglomeration have a siphon effect. Regions with higher technical efficiency, through the mechanisms of technology spillover and policy emulation, can pass the advanced technology of breeding, planting, purchasing, storage, and processing to areas with small economic gaps (hereinafter referred to as the surrounding area).Footnote 1 This promotes the optimization and upgrading of production equipment, technology, and management in these areas and then reduces their CEI. Urbanization enables regions to have a better working environment and salary conditions and stronger consumer demand, employment opportunities, and investment opportunities. It further attracts capital, talent, technology, data, and other production factors in the surrounding areas, thereby increasing the surrounding areas’ CEI. When the industrial structure and agglomeration of rice are relatively high, it reflects the high level of specialization of rice production in the region. Higher specialization attracts the related resources in the surrounding areas and eventually increases the surrounding areas’ CEI through resource flow. Combined with the local spatial agglomeration of rice represented by the local Moran’s I, the main rice-producing areas in Northeast China (Heilongjiang, Liaoning and Jilin) show LL (low-low) agglomeration, indicating a significant demonstration effect on the CEI of surrounding areas (Fig. 5a). In addition, the CEI of the main rice-producing areas (e.g., Hubei, Hunan, Jiangxi, and Fujian) presents HH (high-high) agglomeration, and the level is higher than that of the northeastern region. This is an important reason for the continuous northward shift of China’s rice production center (Xu et al. 2013).
From the decomposition of the spatial effect of factors relevant to wheat, technical efficiency has a demonstration effect, and urbanization has a siphon effect. There is a demonstration effect of technology in the main wheat-producing areas due to the mechanism of technology spillover and policy emulation, and there is a siphon effect of urbanization due to the advantages of urbanization in capital, labor, and technology. Combined with the local spatial agglomeration of wheat, the main wheat-producing areas (e.g., Shandong, Hebei, Henan, Jiangsu, and Anhui) show LL (low-low) agglomeration, and they have significant demonstration effects on surrounding areas (Fig. 5b). This also reflects that the increase in wheat production efficiency is the main reason for the lower CEI of the Huang-Huai-Hai Plain.
The decomposition of the spatial effect of the factors relevant to maize demonstrates that technical efficiency has a demonstration effect, and urbanization and agricultural trade openness have a siphon effect. The technology of the main maize-producing areas has a demonstration effect due to the high mechanization and high plant utilization rate, and the urbanization of the main maize-producing areas has a siphon effect due to the advanced production technology and complete service conditions. As nonmain maize-producing areas, the maize import provinces (e.g., Guangdong, Yunnan, Jiangsu, and Anhui) have strong agricultural trade competitiveness. This attracts capital and information in the surrounding areas, which creates a siphon effect. Combined with the local spatial agglomeration of maize, the main producing areas (e.g., Hebei, Inner Mongolia, Jilin, Liaoning, Heilongjiang, Shandong, and Henan) show LL (low-low) agglomeration, and they have significant demonstration effects on the surrounding areas (Fig. 5c). It also reflects that the increase in maize production efficiency is the main reason for the lower CEI of Northeast and North China.
Discussion and policy implications
Discussion
This paper used rice, wheat, and maize as examples, adjusted the calculation method of grain carbon emissions, measured the TCE and CEI of grains in China, and analyzed the evolution of the spatial and temporal pattern. Next, the paper explored the influence mechanism and spillover effect of CEI by using the dynamic spatial model. This article has two main contributions. (1) This paper uses China’s rice, wheat, and maize as examples, improves the calculation method of grain carbon emissions in the literature, more accurately measures the TCE and CEI of grain production, and analyses the evolution of temporal and spatial patterns. (2) This paper uses spatial econometrics methods to analyze the influence mechanism and spatial spillover effect of the CEI of grain production.
From the temporal and spatial pattern evolution, the TCE of the grains showed a fluctuating upward trend, and the CEI in the main producing areas decreased. This is consistent with Wang et al. (2020) and Xu and Bai (2013). The main reason for the increase in TCE was the increase in sowing area and total yield. Both the TCE and CEI of rice in Central, East, and South China were high, while the TCE of Northeast China was high, and the CEI was low. The TCE of wheat in Northeast China was low and the CEI was relatively high, while the Huang-Huai-Hai Plain had relatively high TCEs and low CEI. The TCE and CEI of maize in Northwest China were high, while the TCE of Northeast and North China was high, and the CEI was low.
From the influence mechanism, different factors have significant heterogeneous effects, which are caused by production technology and the regional economic and resource endowments of the main producing areas. This result is consistent with Zhao et al. (2018), Xiong et al. (2016), and Zhang et al. (2013). The results show that technical efficiency has the greatest negative impact on the CEI of grain production, reflecting that the improvement in technical efficiency is the main measure of grain carbon emission reduction. The agricultural output per capita has a negative impact on the CEI of grain production. Urbanization has a significant negative impact on the CEI of rice and a positive impact on the CEI of wheat, while the impact on maize is not significant. The proportion of disaster area has a positive impact on the CEI of grain. The industrial structure and agglomeration of grain production have a negative impact on the CEI of grain production, and they are realized by gathering production technology, knowledge, and capital. Agricultural trade openness has a negative impact on the CEI of maize and rice, but the impact on wheat is not significant. Agricultural financial expenditure has a significant negative impact on rice and a significant positive impact on the CEI of wheat, while the impact on maize is not significant. The financial expenditure of environmental protection has a negative impact on the CEI of grain production. The difference in natural endowment and growth attributes helps explain why the CEI of wheat is higher than that of rice and maize.
The factors (e.g., technical efficiency, urbanization, grain industrial structure and agglomeration, and agricultural trade openness) can be transmitted to neighboring areas. This result is consistent with Wu et al. (2020). The spatial spillover mechanisms are resource flow, technology spillover, and policy learning, producing the demonstration effect and siphon effect. Spatial effect decomposition of the rice model demonstrates that technical efficiency has a demonstration effect and that urbanization, grain industrial structure, and grain production agglomeration have a siphon effect. Spatial effect decomposition of the wheat model demonstrates that technical efficiency has a demonstration effect and that urbanization has a siphon effect. Spatial effect decomposition of the maize model demonstrates that technical efficiency has a demonstration effect and that urbanization and agricultural trade openness have a siphon effect. These spatial spillover effects are also important reasons for the northward transfer of the grain production center and the agglomeration of low carbon emission areas.
Policy implications
The results show that improvements in grain technical efficiency, urbanization, agricultural structure, agricultural trade openness, and agricultural policies have significant implications for the CEI of grain production and that these influencing factors have spatial spillover effects. Therefore, the following recommendations are proposed. First, we should strengthen the innovation and popularity of agricultural technology by constructing a grain technology service system, popularizing high-efficiency and energy-saving agricultural machinery, cultivating grain seeds of high quality, and improving the utilization efficiency of agricultural inputs. We should accelerate the upgrading of agricultural machinery and technology, eliminate backward agricultural machinery, promote advanced agricultural technologies, and reduce carbon emissions from the use of agricultural machinery. Taking saving fertilizer and pesticides as the starting point, energy-saving agricultural technology, and biological control technology should be popularized and applied, the use of pesticides should be reduced and the utilization rate of pesticides should be improved. We should increase the coverage of soil testing and formula fertilization, improve the efficiency of chemical fertilizer, and reduce agricultural carbon emissions from the source. Second, we should promote orderly urbanization by implementing a reasonable urbanization policy. We should prevent the excessive expansion of urban space caused by recklessly pursuing the urbanization rate, coordinate the process of urbanization and the optimization of agricultural industry distribution, and give full play to the positive role of urbanization in agricultural carbon emission reduction. Third, the agricultural structure should be optimized by reorganizing and improving the efficiency of agricultural resources in major grain-producing areas. On the premise of ensuring food security, the agricultural industrial structure should be further adjusted, the regional distribution and planting structure of grains should be optimized, the planting of crops with high resource consumption should be reduced, and the planting of high-yield and stress-resistant crops should be increased. Fourth, the transfer payments for energy conservation and emission reduction technology should be improved through the assessment responsibility system of emission reduction and the ecological compensation technology system of low carbon agriculture. We should increase agricultural investment in energy conservation, emission reduction and environmental protection; formulate medium- and long-term plans for carbon emission reduction of different types of grain; and establish agricultural emission reduction policies, laws, and regulations. Fifth, we should strengthen the flow and sharing of production factors among regions and establish the coordination and governance mechanism of agricultural emission reduction across regions. We should focus on the changes in agricultural carbon emission reduction policies in adjacent areas; strengthen regional cooperation and information sharing; use the external spillover effect of technology, agricultural policies, and other influencing factors; and apply agricultural low-carbon technologies in a wider range through regional linkage.
Data Availability
The datasets used and analyzed during the current study are available from the corresponding author on reasonable request.
Notes
Note: The abscissa represents the deviation between the sample value and the mean value, and the ordinate represents the spatial lag value; if the abscissa value is greater than 0 and the ordinate value is greater than 0, it means the high/high (H-H) positive spatial association (SA); if the abscissa value is less than 0 and the ordinate value is greater than 0, it means the low/high (L-H) negative SA; if the abscissa value is less than 0 and the ordinate value is less than 0, it means the low/low (L-L) positive SA; if the abscissa value is greater than 0 and the ordinate value is less than 0, it means the high/low (H-L) negative SA; 1, Beijing; 2, Tianjing; 3, Hebei; 4, Shanxi; 5, Inner Mongolia; 6, Liaoning; 7, Jilin; 8, Heilongjiang; 9, Shanghai; 10, Jiangsu; 11, Zhejiang; 12, Anhui; 13, Fujian; 14, Jiangxi; 15, Shandong; 16, Henan; 17, Hubei; 18, Hunan; 19, Guangdong; 20, Guangxi; 21, Hainan; 22, Chongqing; 23, Sichuan; 24, Guizhou; 25, Yunnan; 26, Tibet; 27, Shaanxi; 28, Gansu; 29, Qinghai; 30, Ningxia; 31, Xinjiang
References
Allouche J (2011) The sustainability and resilience of global water and food systems: political analysis of the interplay between security, resource scarcity, political systems and global trade. Food Policy 36(S1):3–8. https://doi.org/10.1016/j.foodpol.2010.11.013
Arthur M, Peter O (2015) Certification of markets, markets of certificates: tracing sustainability in global agro-food value chains. Sustainability 7(9):1–21. https://doi.org/10.3390/su70912258
Bai Y, Deng X, Jiang S, Zhao Z, Miao Y (2018) Relationship between climate change and low-carbon agricultural production: a case study in Hebei Province, China. Ecol Indic 105:438–447. https://doi.org/10.1016/j.ecolind.2018.04.003
Bar M, Krystallis A, Saab M, Kügler GG (2011) Investigating the gap between citizens’ sustainability attitudes and food purchasing behaviour: empirical evidence from Brazilian pork consumers. Int J Consum Stud 35(4):391–402
Baumann M, Gasparri I, Piquer-Rodríguez M, Pizarro GG, Griffiths P, Hostert P, Kuemmerle T (2016) Carbon emissions from agricultural expansion and intensification in the Chaco. Glob Change Biol 23(5):13521. https://doi.org/10.1111/gcb.13521
Bo L, Suying FU, Zhang J, Yu H (2011) Carbon functions of agricultural land use and economy across China: a correlation analysis. Energy Procedia 5(22):1949–1956. https://doi.org/10.1016/j.egypro.2011.03.336
Chen W, Peng Y, Yu G (2020) The influencing factors and spillover effects of interprovincial agricultural carbon emissions in China. PLoS ONE 15(11):e0240800. https://doi.org/10.1371/journal.pone.0240800
Cui H, Zhao T, Shi H (2018) STIRPAT-based driving factor decomposition analysis of agricultural carbon emissions in Hebei, China. Pol J Environ Stud 27(4):1449–1461
Davis KF, Yu K, Rulli MC, Pichdara L, D’Odorico P (2015) Accelerated deforestation driven by large-scale land acquisitions in Cambodia. Nat Geosci 8:772–775. https://doi.org/10.1038/ngeo2540
Dogan E, Sebri M, Turkekul B (2016) Exploring the relationship between agricultural electricity consumption and output: new evidence from Turkish regional data. Energy Policy 95:370–377. https://doi.org/10.1016/j.enpol.2016.05.018
Dumortier J, Elobeid A (2021) Effects of a carbon tax in the United States on agricultural markets and carbon emissions from land-use change. Land Use Policy 103(8):105320. https://doi.org/10.1016/j.landusepol.2021.105320
Elhorst JP (2014) Matlab software for spatial panels. Int Reg Sci Rev 37(3):389–405. https://doi.org/10.1177/0160017612452429
Elhorst JP, Lacombe DJ, Piras G (2012) On model specification and parameter space definitions in higher order spatial econometric models. Reg Sci Urban Econ 42(1–2):211–220. https://doi.org/10.1016/j.regsciurbeco.2011.09.003
Emma W, Mat J, Debra S, Richard K, Judy O (2013) Creating a learning environment to promote food sustainability issues in primary schools? Staff perceptions of implementing the food for life partnership programme. Sustainability 5(3):1128–1140. https://doi.org/10.3390/su5031128
Essien E, Dzisi KA, Addo A (2018) Decision support system for designing sustainable multi-stakeholder networks of grain storage facilities in developing countries. Comput Electron Agric 147:126–130. https://doi.org/10.1016/j.compag.2018.02.019
Fan S, Brzeska J (2014) Feeding more people on an increasingly fragile planet: China’s food and nutrition security in a national and global context. J Integr Agric 3(6):1193–1205. https://doi.org/10.1016/S2095-3119(14)60753-X
Fardet A, Rock E (2020) Ultra-processed foods and food system sustainability: what are the links? Sustainability 12(15):1–26. https://doi.org/10.3390/su12156280
Francesco N, Tubiello, Mirella S, Simone R, Alessandro F (2012) Alessandro Ferrara. Analysis of global emissions, carbon intensity and efficiency of food production. Energia, Ambiente e Innovazione (4):87–93. https://www.researchgate.net/publication/266395060.
Galinato GI, Uchida S (2010) Evaluating temporary certified emission reductions in reforestation and afforestation programs. Environ Resource Econ 46(1):111–133. https://doi.org/10.1007/s10640-009-9338-9
Gan Y, Liang C, Chai Q, Lemke RL, Campbell CA, Zentner RP (2014) Improving farming practices reduces the carbon footprint of spring wheat production. Nat Commun 5:5012. https://doi.org/10.1038/ncomms6012
Girija P, Bradley R, David C, Bill B (2015) A framework for assessing local PES proposals. Land Use Policy 43:37–41. https://doi.org/10.1016/j.landusepol.2014.10.023
Gracia A, Gómez M (2020) Food sustainability and waste reduction in Spain: consumer preferences for local, suboptimal, and/or unwashed fresh food products. Sustainability 12(10):1–15. https://doi.org/10.3390/su12104148
Han H, Zhong Z, Yu G, Feng X, Liu S (2018) Coupling and decoupling effects of agricultural carbon emissions in China and their driving factors. Environ Sci Pollut Res 25(9):1–14. https://doi.org/10.1007/s11356-018-2589-7
Hervé Corvellec (2016) Sustainability objects as performative definitions of sustainability: the case of food-waste-based biogas and biofertilizers. J Mater Cult 21(3):383–401. https://www.researchgate.net/publication/275973437
Huang X, Xu X, Wang Q, Zhang L, Chen L (2019) Assessment of agricultural carbon emissions and their spatiotemporal changes in China, 1997–2016. Int J Environ Res Public Health 16(17):3105. https://doi.org/10.3390/ijerph16173105。
Hou L, Yang Y, Zhang X, Jiang C (2021) Carbon footprint for wheat and maize production modulated by farm size: a study in the north China plain. International Journal of Climate Change Strategies and Management, ahead-of-print (ahead-of-print). https://doi.org/10.1108/IJCCSM-10-2020-0110.
Jia JQ, Sun ZL, Li XD (2019) Does grain price support policy improve China’s total factor productivity? Take the minimum purchase price policy for wheat as an example. Rural Economy 1:67–72 ((in Chinese))
Kaczorowska J, Rejman K, Halicka E, Szczebyo A, Górska-Warsewicz H (2019) Impact of food sustainability labels on the perceived product value and price expectations of urban consumers. Sustainability 11(24):1–17. https://doi.org/10.3390/su11247240
Kashyap D, Agarwal T (2021) Carbon footprint and water footprint of rice and wheat production in punjab, India. Agricultural Systems (186):102959.
Lamb A, Green R, Bateman I, Broadmeadow M, Bruce T, Burney J, Carey P, Chadwick D, Crane E, Field R (2016) The potential for land sparing to offset greenhouse gas emissions from agriculture. Nat Clim Chang 6:488–492. https://doi.org/10.1038/NCLIMATE2910
Li P, Zhao J (2013) An empirical study on China’s regional carbon emissions of agriculture. Int J Asian Bus Inf Manag 4(4):67–77. https://doi.org/10.4018/ijabim.2013100105
Liu D, Zhu X, Wang Y (2020) China’s agricultural green total factor productivity based on carbon emission: an analysis of evolution trend and influencing factors. J Clean Prod 278(1):123692. https://doi.org/10.1016/j.jclepro.2020.123692
Liu M, Yang L (2021) Spatial pattern of China’s agricultural carbon emission performance. Ecol Ind 133:108345. https://doi.org/10.1016/j.ecolind.2021.108345
Liu X, Yu Y, Luan S (2019) Empirical study on the decomposition of carbon emission factors in agricultural energy consumption. IOP Conf Ser Earth Environ Sci (252):042045. 110.1088/1755–1315/252/4/042045
Liu Y, Zhang JB, Zhang L (2018) Analysis of carbon emission efficiency of rice in China under different rice planting patterns based on the DEA-SBM model. J China Agric Univ 23(06):177–186. https://doi.org/10.11841/j.issn.1007-4333.2018.06.20 ((in Chinese))
Marianne H, Fleur M, Guido VH (2017) Sustainability experiments in the agri-food system: uncovering the factors of new governance and collaboration success. Sustainability 9(6):1027–1050. https://doi.org/10.3390/su9061027
Marra MC, Kaval P (2000) The relative profitability of sustainable grain cropping systems: a meta-analytic comparison. J Sustain Agric 16(4):19–32. https://doi.org/10.1300/J064v16n04_04
Min J, Hu H (2012) Calculation of greenhouse gases emission from agricultural production in China. China Popul Resour Environ 22(7):21–27 ((in Chinese))
Mohammad ZH, Yu H, Neal JA, Gibson KE, Sirsat SA (2019) Food safety challenges and barriers in southern United States farmers markets. Foods 9:12. https://doi.org/10.3390/foods9010012
Nayak D, Saetnan E, Cheng K, Wang W, Koslowski F, Cheng Y, Zhu W, Wang J, Liu J, Moran D (2015) Management opportunities to mitigate greenhouse gas emissions from Chinese agriculture. Agr Ecosyst Environ 209:108–124. https://doi.org/10.1016/j.agee.2015.04.035
Spiertz H (2010) Food production, crops and sustainability: restoring confidence in science and technology. Curr Opin Environ Sustain 2:5–6. https://doi.org/10.1016/j.cosust.2010.10.006
Thomasa GA, Dalalb RC, Westonc EJ, Kinga AJ, Holmesa CJ, Orangea DN, Lehanec KJ (2010) Crop rotations for sustainable grain production on a vertisol in the semi-arid subtropics. J Sustain Agric 35(1):2–26. https://doi.org/10.1080/10440046.2011.530195
Tian J, Yang H, Xiang P, Liu D, Li L (2016) Drivers of agricultural carbon emissions in Hunan Province, China. Environ Earth Sci 75(2):121. https://doi.org/10.1007/s12665-015-4777-9
Tone K, Tsutsui M (2010) An epsilon-based measure of efficiency in DEA-A third pole of technical efficiency. Eur J Oper Res 207(3):1554–1563. https://doi.org/10.1016/j.ejor.2010.07.014
Tong XL, Di H, Yang XY (2019) Policy effects evaluation of grain minimum purchase prices: evidence from the wheat. Issues in Agricultural Economy (9):85–95. https://doi.org/10.13246/j.cnki.iae.2019.09.010. (in Chinese)
Vitters G, Torjusen H, Laitala K, Tocco B, Biasini B, Csillag P (2019) Short food supply chains and their contributions to sustainability: participants’ views and perceptions from 12 European cases. Sustainability 11(17):1–33. https://doi.org/10.3390/su11174800
Wang G, Liao M, Jiang J (2020) Research on agricultural carbon emissions and regional carbon emissions reduction strategies in China. Sustainability 12(7):1–20. https://doi.org/10.3390/su12072627
Wang W, Koslowski F, Nayak DR, Smith P, Saetnan E, Ju X, Guo L, Han G, Perthuis DC, Lin E (2014) Greenhouse gas mitigation in Chinese agriculture: distinguishing technical and economic potentials. Glob Environ Chang 26:53–62. https://doi.org/10.1016/j.gloenvcha.2014.03.008
Wu H, He Y, Chen W, Huang H (2020) Spatial effect and influencing factors of China’s agricultural carbon compensation rate based on spatial Durbin model. J Agrotechnical Econ (03):110–123. https://doi.org/10.13246/j.cnki.jae.2020.03.009. (in Chinese)
Xiong C, Chen S, Xu L (2020) Driving factors analysis of agricultural carbon emissions based on extended STIRPAT model of Jiangsu Province, China. Growth Change 51(3):1401–1416. https://doi.org/10.1111/grow.12384
Xiong C, Yang D, Xia F, Huo J (2016) Changes in agricultural carbon emissions and factors that influence agricultural carbon emissions based on different stages in Xinjiang, China. Sci Rep 6(1):36912. https://doi.org/10.1038/srep3691
Xiong C, Wang G, Su W, Gao Q (2021) Selecting low-carbon technologies and measures for high agricultural carbon productivity in Taihu Lake Basin, China. Environ Sci Pollut Res 4:1–8. https://doi.org/10.1007/s11356-021-14272-z
Xu X, Zhang N, Zhao D, Liu C (2020) The effect of trade openness on the relationship between agricultural technology inputs and carbon emissions: evidence from a panel threshold model. Environ Sci Pollut Res 28(8):9991–10004. https://doi.org/10.1007/s11356-020-11255-4
Xu C, Zhou X, Li F (2013) The research of rice production northward movement in China. Issues Agric Econ 34(7):35–40. https://doi.org/10.13246/j.cnki.iae.2013.07.007 ((in Chinese))
Xu L, Bai J (2013) Study on characteristics and reduction countermeasures of the agricultural carbon emission in China. Meteorol Environ Res (11):62–66. CNKI:SUN:MEVR.0.2013–11–016.
Zhang L, Pang J, Chen X, Lu Z (2019) Carbon emissions, energy consumption and economic growth: Evidence from the agricultural sector of China’s main grain-producing areas. Sci Total Environ 665:1017–1025. https://doi.org/10.1016/j.scitotenv.2019.02.162
Zhang WF, Dou ZX, He P, Ju XT, Powlson D, Chadwick D, Norse D, LuYL ZY, Wu L (2013) New technologies reduce greenhouse gas emissions from nitrogenous fertilizer in China. Proc Natl Acad Sci USA 110(21):8375–8380. https://doi.org/10.1073/pnas.1210447110
Zhang X, Zheng S, Yu L (2020) Green efficiency measurement and spatial spillover effect of China’s marine carbon sequestration fishery. Chinese Rural Economy 10:91–110 ((in Chinese))
Zhang Y, Fang G (2013) Research on spatial-temporal characteristics and affecting factors decomposition of agricultural carbon emission in Suzhou City, Anhui Province, China. Appl Mech Mater 291–294:1385–1388. https://doi.org/10.4028/www.scientific.net/AMM.291-294.1385
Zhao R, Liu Y, Tian M, Ding M, Cao L, Zhang Z, Chuai X, Xiao L, Yao L (2018) Impacts of water and land resources exploitation on agricultural carbon emissions: The water-land-energy-carbon nexus. Land Use Policy 72:480–492. https://doi.org/10.1016/j.landusepol.2017.12.029
Yong L, Zhang J, Lu Z (2018) Analysis of carbon emission efficiency of rice in China under different rice planting patterns based on the dea-sbm model. Journal of China Agricultural University. https://doi.org/10.11841/j.issn.1007-4333.2018.06.20 (in Chinese)
Yu L (2016) Analysis on the factor decomposition of carbon emissions caused by Chinese agricultural land use and the emission reduction measures. Nat Environ Pollut Technol 15(2):401–408
Funding
The research was financially supported by the National Social Science Foundation of China (No. 20CJY054), the Research Project of Philosophy and Social Sciences Think Tank in Henan’s Universities, China (No.2021-ZKYJ12), and the Priority Research Program of Chinese Academy of Sciences (No.XDA20010102).
Author information
Authors and Affiliations
Contributions
Zhi Li: Conceptualization; data curation; writing, original draft; investigation; funding acquisition. Jingdong Li: Supervision, methodology, software, formal analysis, validation, visualization, writing—reviewing and editing.
Corresponding author
Ethics declarations
Ethics approval and consent to participate
Not applicable.
Consent for publication
Not applicable.
Conflict of interest
The authors declare no competing interests.
Additional information
Responsible editor: Ilhan Ozturk
Publisher's Note
Springer Nature remains neutral with regard to jurisdictional claims in published maps and institutional affiliations.
Rights and permissions
About this article
Cite this article
Li, Z., Li, J. The influence mechanism and spatial effect of carbon emission intensity in the agricultural sustainable supply: evidence from china's grain production. Environ Sci Pollut Res 29, 44442–44460 (2022). https://doi.org/10.1007/s11356-022-18980-y
Received:
Accepted:
Published:
Issue Date:
DOI: https://doi.org/10.1007/s11356-022-18980-y