Abstract
Improving the ecological efficiency of farmland use (EEFU) has become an important part of ensuring food security and solving environmental pollution problems. At present, the Chinese government is actively promoting large-scale farmland transfer to reduce the level of farmer-/plot-scale farmland fragmentation (FF), so it is crucial to clarify the effect of landscape-scale FF on EEFU. This study applies the non-dynamic panel and threshold models in an empirical study of the municipal administrative regions along the Yangtze River Economic Belt (2000, 2005, 2010, and 2015). The results reveal that there is a single threshold for the effects of area, shape, and distance fragmentation on EEFU with farmland area per capita (FAPC) as the threshold variable. The threshold values are 1.548, 1.373, and 1.542, respectively. The effects of area and distance fragmentation on EEFU are initially promoted and then suppressed; however, shape fragmentation always has an inhibitory effect on EEFU. These findings suggest that ignoring the condition of FAPC of different regions and promoting large-scale farmland transfer blindly will give rise to the decline of EFFU. These results are conducive to the sustainable utilization of farmland and the formulation of related policies.
Similar content being viewed by others
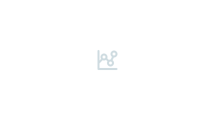
Explore related subjects
Discover the latest articles, news and stories from top researchers in related subjects.Avoid common mistakes on your manuscript.
Introduction
As the most populous nation, farmland is under great pressure for supporting social economic development and ensuring food security in China (Deng et al. 2015; Liu 2018). On the one hand, China underwent rapid urbanization in the past 40 years. The conversion of farmland to construction land has not only become an important guarantee for local government finances (Zhong et al. 2019), but also provides space for urban expansion, especially for high-quality farmland around the city (Wu et al. 2017). On the other hand, farmland is still the main means of production for about 5.516 million China’s farmers (Population Investigation Division, National Bureau of Statistics of China 2020). Efficient use of cultivated land resources is an important route for farmers to achieve income growth. Therefore, the Chinese center government always attaches great importance on the utilization and protection of farmland resources (Lu et al. 2018a; Zhang et al. 2019; Jin et al. 2019) and has launched many principal campaigns to maintain the quantity and quality of farmland, e.g., the dynamic balance system of farmland quantity and the basic farmland protection system (Wu et al. 2017). Also, in order to ensure food security and raise farmers’ income for rural poverty reduction, the Chinese center government has also implemented a number of agricultural subsidy policies to encourage farmers to actively engage in agricultural production (Huang et al. 2013).
In recent years, benefited from the large amount of chemical fertilizer input (Yu 2016), China’s grain production has shown a sustained growth trend, and the current outputs equaled about one fifth of world corresponding supplies (Liu et al. 2020). From the perspective of farmland use practices, focusing only on economic benefits while ignoring the associated ecological (environmental) problems of farmland use will inevitably cause land degradation and environmental pollution (Balota et al. 2014), such as heavy metal pollution (Zhang et al. 2020), soil compaction (Prokop et al. 2018), greenhouse gas emissions (Lu et al. 2018a), and nonpoint source pollution (Dalu et al. 2018; Ruan et al. 2020). Relevant researches have also pointed out that one-third of the world’s farmland is polluted and damaged due to unreasonable utilization (Verheijen et al. 2009; Cao et al. 2020). Thus, balancing the benefits between economic and ecological plays a key role in realizing the sustainable utilization of farmland resources (Hou et al. 2019a).
Ecological efficiency (eco-efficiency), the achievement of the highest possible economic benefits with the least possible input of resources and damage to the environment, has been widely studied by scholars and governments (Schaltegger and Sturm 1990). Eco-efficiency has been applied to urbanization (Bai et al. 2018), agricultural production (Bonfiglio et al. 2017), and energy consumption (Yang et al. 2018). Similarly, in order to quantify the sustainability of farmland use, many scholars have examined and discussed EEFU (Lu et al. 2018a; Ren et al. 2019; Hou et al. 2019a). For example, on the basis of the comprehensive consideration of economic output, nonpoint source pollution, and carbon emissions, Feng et al. (2015) point out that China’s EEFU showed a significant downward trend from 1993 to 2013. Sabiha et al. (2017) computed the eco-efficiency of high-yielding farmland in Bangladesh and found that undesirable output or on-farm environmental damage induces an efficiency loss of 20%. In addition, Hou et al. (2019a) focused on the impact of urbanization on EEFU.
At present, the Chinese government is actively promoting large-scale farmland transfer to reduce the level of FF (Xie and Lu 2017). Thus, the impact of FF on farmland use has been a topic of interest for researchers. One view is that FF is the main obstacle to improving agricultural productivity and realizing agricultural modernization (Ali and Deininger 2014; Latruffe and Piet 2014; Sklenicka 2016; Sklenicka et al. 2017; Lu et al. 2018b). In contrast, many studies suggest that FF can enrich the agricultural production structure (Bellon and Taylor 1993), spread the working time of agricultural production across different seasons (Bellon 1996), reduce land ownership-related conflicts (Ntihinyurwa et al. 2019), and increase biodiversity (Thenail and Baudry 2004; Thenail et al. 2009). However, the above research focuses more on farmer-/plot-scale FF and less on the effects of FF on the utilization of farmland at the landscape scale.
In fact, due to the large population and limited farmland resources in rural areas, the tense human-land relationship is the main factor for the farmer-/plot-scale FF in the rural areas of China, which has also been confirmed by many scholars (Liu et al. 2017; Xie and Lu 2017). In recent years, the rapid development of the social economy has significantly changed the relationship between humans and land in China’s rural areas, thus increasing FAPC and promoting the transfer of farmland (Long 2014). With the expansion of the farmland management size for farmers, farmer-/plot-scale FF have gradually evolved into landscape-scale FF. Consequently, the effect of landscape-scale FF on the EEFU is also worthy of attention, especially for relevant policy design and decision making in the future (Del Corral et al. 2011; Latruffe and Piet 2014). More importantly, farmland transfer policy implies a hypothesis that landscape-scale FF is beneficial to EEFU. Unfortunately, the effects of landscape-scale FF on EEFU remains unclear. Additionally, the variation of FAPC is a dominant factor for the different type transformation of FF from farmer-/plot-scale to landscape-scale, but few studies have explored the effects of landscape-scale FF on EEFU in combination with changes in FAPC.
In this study, we selected Fragstats 4.2 software and the slack-based measure (SBM) undesirable model to calculate the landscape-scale FF and the EEFU, respectively. Further, according to the threshold model proposed by Hansen (1999), we constructed an analysis model with FAPC as the threshold variable to analyze the impact of FF on EEFU. Prior to this analysis, we used the non-dynamic panel model to perform a comparative analysis to provide a useful reference for relevant policy formulation. Accordingly, the specific contents of this study are as follows: “Literature review” systematically summarizes the relationship of different scales of fragmentation and the effect of FF on farmland use. “Data sources” describes how landscape-scale FF and EEFU are calculated and explains the construction of threshold and non-dynamic panel models. “Methodology” introduces the study area situation and the related data acquisition methods and process. “Results and discussion” presents the findings of the empirical analysis and discussion. “Conclusions” details research conclusions.
Literature review
FF definitions and their interrelationship
Many scholars have defined the concept of FF from different perspectives. For example, Mcpherson (1982) pointed out that FF means that a household’s farmland resources are divided into several spatially separated plots. The same or similar concepts have been used in many relevant studies, such as the plot size of each household/farm (Carter and Yao 2002), the number of plots owned by the household/farm (Xie and Lu 2017), and the average plot size of the household/farm (Niroula and Thapa 2005). In contrast, to measure FF, most studies use the plot size of each household/farm rather than the number of plots operated by the household and the farm’s average plot size. Since farmland plot is a basic operational unit, Niroula and Thapa (2007) consider it meaningful to analyze the impact of FF on farmland utilization. Consequently, plot size, shape (single plot or multiple plots), and distance (between the plot and the farm, as well as among the plots), which usually influence the cost of farmland utilization and abnegation of modern technologies, are the most commonly used variables to represent the level of FF (Del Corral et al. 2011; Kawasaki 2010). It can be seen that the FF concepts mentioned above are defined from the perspective of whether the use of farmland is beneficial for farmers, so they are often referred to as farmer-/plot-scale FF. In contrast, some scholars have also proposed some FF indicators at the landscape scale, such as the number, size, and shape of plots; distance of the plots from the farmer’s residence; and distance between the plots, which was calculated at the municipality level (Jia and Petrick 2014; Latruffe and Piet 2014).
As we all know, only land suitable for agricultural production can be developed or converted into farmland. Hence, farmland patches have different sizes, shapes, and locations at the landscape scale, which determine the basic conditions of farmland use, as represented by labels (1), (2), and (3) in Fig. 1. However, since China has a large population but not enough farmland, farmers often own several widely dispersed plots of different quality in order to achieve a fair distribution of farmland, as denoted by labels A-1, A-2, and A-3 in Fig. 1 (Tan et al. 2006; Lu et al. 2018b). One patch is usually divided into many plots for different farmers (Sklenicka 2016). Currently, landscape-scale farmland patches could lead to the separation of farmland plots from the border, shape, and spatial distance perspective to some extent, but due to the decentralized farmland management of farmers, the FF caused by the division of farmland patches is more serious (Latruffe and Piet 2014; Guo et al. 2019; Carter and Yao 2002; Ntihinyurwa et al. 2019). With improvements at the social and economic levels, many members of rural populations have moved to cities. The increase in FAPC in rural areas brings the possibility of farmland transfer. Since farmers with high labor productivity have high marginal output, in general, they are always motivated to expand the area of their farmland by leasing or exchanging farmland management rightsFootnote 1 (Janus et al. 2018). At this time, farmers are more inclined to transfer adjacent farmland to reduce costs and increase income (Guo et al. 2019). For example, in order to expand the farmland area, farmer A exchanged the plot A-2 with the plot C-1 from farmer C at stage 2 and rented the plot B-1 from farmer B at stage 3 (Fig. 1) In this context, patches (1) will be managed independently by farmer A at stage 3, and thus the condition of farmland patches (1) which will have a direct impact on farmland use activities, i.e., at stages 3 and 4 in Fig. 1. With the increase rate of rural population loss, the area owned by each farmer expanded. However, if the income of some farmland patches is less than the opportunity cost or the operating costs, these farmlands will be marginalized or even abandoned by farmers, as shown in stage 4 in Fig. 1 (Strijker 2005). Li and Li (2019) have also confirmed that a large number of farmlands have been abandoned in mountainous areas where the farmland landscape is very fragmented.
The FF has attracted widespread attentions since it plays an important role in the utilization of farmland. It is important to note that landscape-scale FF can always affect farmland use activities, but the degree of effect varies with the change in the FAPC (Di Falco et al. 2010; Kawasaki 2010; Latruffe and Piet 2014; Xie and Lu 2017). In addition, to facilitate an understanding of fragmentation, this study uses the term “plot”, rather than “patch”, in the following sections.
Effect of FF on farmland utilization
Most studies have considered FF to be an obstacle to farmland utilization (Di Falco et al. 2010; Latruffe and Piet 2014). For example, Kawasaki (2010) investigated the costs and benefits of FF by using panel data obtained from Japanese rice farms and revealed that FF increases production costs and offsets economies of size. Further, FF also increases the time and fuel required to travel between plots and reduce the size of land through the loss or wastage of some areas at their boundaries (Carter and Yao 2002; Ntihinyurwa et al. 2019). Moreover, based on household survey data collected from the Jiangsu province in China, Lu et al. (2018b) confirmed that an increase in the Simpson index per unit resulted in an average increase in costs by 39%, whereas an increase in plot size resulted in an average decrease in costs by 8%. In addition, relevant studies have shown that farmers are not enthusiastic about adopting modern technology and investments in farmland construction when plots are very small or irregularly shaped (Di Falco et al. 2010; Tan et al. 2010). In addition, some scholars reveal that FF will increase the cost of water transportation (Kawasaki 2010; Tan et al. 2010). With the increase in agricultural production costs, FF has become the main cause for a reduction in agricultural labor productivity (Jia and Petrick 2014), agricultural land circulation (Xie and Lu 2017), and the abandonment of farmland (Lu et al. 2018b).
However, some scholars clarify that FF also has many positive effects. On the one hand, FF has undeniably played an important role in agricultural development (Lin 1992). For instance, the Household Responsibility System in China has significantly promoted the development of agriculture and guaranteed a high demand for agricultural products in the development of the national economy (Xu et al. 2019). Following the implementation of land reforms in 1991, the transformation of large farms to highly intensive small-scale farms promoted the rapid development of Bulgarian agriculture (Buckwell and Davidova 1993) and a similar phenomenon also occurred in Central and Eastern European countries (Dijk 2002). As clarified by Di Falco et al. (2010), FF plays an important role in ensuring food security; hence, policy makers should not despise small farmers and consider them burdensome. On the other hand, some scholars have found that FF can enrich the agricultural production structure (Bellon and Taylor 1993), offset labor insufficiency during busy seasons and surplus labor during slack seasons (Bellon 1996), and reduce land ownership-related conflicts (Ntihinyurwa et al. 2019). Moreover, FF reduces agricultural production risks arising from the occurrence of disease, hail, droughts, floods, and other natural disasters and, thereby, ensures food security (Del Corral et al. 2011).
Overall, FF affect many aspects of farmland use activities, especially the relationship between input and output of farmland. It is important to note that the above concept of FF highlights the influence of farmer-scale or plot-scale FF on the input-output of farmland utilization. As EEFU represents the combination relationship of input and output; however, few researches examine how FF affects the EEFU at landscape scale. As noted in “FF definitions and their interrelationship”, farmland use activities can also be influenced by landscape-scale FF, which will inevitably affect the EEFU. However, previous studies have not provided conclusive evidence on the effect of landscape-scale FF on EEFU. In addition, with the change in the FAPC, the impact of the landscape-scale FF on the EEFU is complex. Therefore, it is likely that there is not a linear relationship between FF and EEFU.
Data sources
Study area
The Yangtze River Economic Belt (YREB), which is the area where the main river and the tributaries of the Yangtze flow, encompasses eight provinces and two municipalities (Fig. 2). It has a total area of 2.05 million km2 and accounts for more than 40% of the country’s population and GDP (Hou et al. 2019a). As one of the country’s three major grain-producing areas, the YREB contributes to China’s food security. Therefore, the rational and complete utilization of all farmland is the basic condition for food security in the YREB. However, in recent years, due to the increase in the cost of land utilization brought about by fragmentation, the phenomenon of abandonment and idle farmland is more serious along the YREB. Moreover, fertilizer, pesticide use, and nonpoint source pollution have increased in the region (Wang et al. 2018; Jin et al. 2018). Therefore, the analysis of FF’s impact on the EEFU in the YREB has immense practical significance.
Data sources and processing
We obtained the data on the region’s farmland (with spatial resolution of 30 × 30 m2 taken by the US Landsat TM/ETM satellite) from the land-use database provided by the Resources and Environment Scientific Data Centre (http://www.resdc.cn) (Deng et al. 2015). Using the geographic information system technology, we calculated each municipal district’s farmland area, which is the basic unit of analysis in this study. This study covers 4 years: 2000, 2005, 2010, and 2015. Social and economic statistics (e.g., data on machinery, fertilizers, length of highways, GDP, and the primary industry labor force) were obtained from the China Regional Economic Statistical Yearbook (2000, 2005, and 2010). In addition, the number of people engaged in units of the primary industry (guidance department on agricultural technology) was provided by the China City Statistical Yearbook (2000, 2005, 2010, and 2015). Since the China Regional Economic Statistical Yearbook lacks the relevant data for 2015, this study consulted the statistical yearbooks of each province to obtain the required index data. Regarding the EEFU, we selected labor force, agricultural machinery, and use of fertilizers as the input vector; the loss of nitrogen and phosphorus from fertilizers as undesirable production; and the primary industry’s output value as desirable production. Further, to achieve interregional comparability, we used the input and output per unit area to calculate the EEFU. The formula for the calculation is as follows: Zi = Fi/Ai, where Zi is the average input or output of farmland use, Fiis the input of production factors or output of products, Ai is the farmland area in the municipal administrative jurisdiction, and the subscript i represents a municipal administrative district. Moreover, we used the loss coefficient and fertilizer input to calculate the undesirable outputs of farmland use. The concrete calculation formula is wastage = input number of fertilizers × loss coefficients (Zhao et al. 2016). To achieve comparable values in different periods, we adjusted the price-related variables to a unified price.
Methodology
Calculation of the farmland fragmentation
FF can be described from three perspectives: size, shape, and distance fragmentation of plots (Lu et al. 2018b). As a result, this study selects the following three indicators to reflect the landscape-scale FF, and Fragstats 4.2 software is used to calculate the corresponding index value.
Plot size coefficient of variance
The plot size coefficient of variance (PScov) reflects the heterogeneity of a region’s farmland area (Zhang et al. 2015). The larger the PScov value, the higher the dispersion degree of the region’s farmland plot area. Further, with an increase in FAPC, the PScov value of plot area can better reflect the influence of plot area on farmland use than the average plot area.
where n is the number of farmland plots in the region, ai is the area (m2) of the farmland plot i, MPS is the mean plot area (m2), and PSSD represents the plot size standard deviation.
Area-weighted mean shape index
Many studies have pointed out that the shape of farmland plots can affect mechanical input and marginal income, such as Latruffe and Piet (2014), because the irregular shape of farmland not only increases the difficulty of farming but also reduces the utilization efficiency of agricultural machinery. However, by increasing the plot area of farmland, the negative impact of irregular shape will be weakened. Therefore, we selected the area-weighted mean shape index (AWMSI) to reflect the farmland’s shape fragmentation level. The larger the AWMSI, the more complex and irregular the plot shape. The specific formula is as follows:
where n is the number of farmland plots in the region; Pi and ai are the perimeter (m) and area (m2) of farmland plot i, respectively; and A is the total area of farmland plots in a region.
Mean nearest neighbor distance
In general, the distance between farmland plots results in an increase in transportation costs and a reduction in the efficiency of fixed assets (Carter and Yao 2002; Zhou et al. 2019). The mean nearest neighbor (MNN) is the main index used to measure landscape-level plot proximity (Riitters and Costanza 2019). In general, the larger the MNN, the larger the distance between farmland plots. Conversely, the plots of farmland were close to each other and distributed in aggregation. The specific formula is as follows:
where dminij is the closest distance between plots i and j and n denotes the number of plots in the region.
Calculation of the eco-efficiency of farmland use
Over the years, the Data Envelopment Analysis (DEA) model has become a popular model to measure efficiency (Charles et al. 2019). However, traditional DEA models (e.g., CCR and BBC models) ignore the input-output slack. Hence, Tone (2002) constructed an SBM of the DEA model. Subsequently, by introducing undesirable outputs into the SBM model, the SBM-undesirable model for eco-efficiency evaluation was constructed (Cooper et al. 2007). The specific operating principles of SBM-undesirable are as follows: the input vector can be expressed as x ∈ Rm and the two output vectors as yg ∈ Ra and yk ∈ Rb. According to the actual process of farmland use, all kinds of input and output are greater than or equal to zero, that is, X ≥ 0, Yg ≥ 0, and Yk ≥ 0. Therefore, we can define the production possible set as p = {(x, yg, yk)| x ≥ Xλ, yg ≥ Yg, yk ≥ YKλ}, λ ∈ Rn, λ ≥ 0. Based on this, The SBM-undesirable model for calculating EEFU can be shown as follows:
where D−, Dg, and Dk are the relaxed variables; λ is the weight vector; when ρ is less than 1, it means that at least one of D−, Dg, and Dk is not zero, and there is room for improvement of EEFU.
Selection of other variables
Besides FF, many factors can affect the process of farmland use and thereby EEFU. Specifically, the proportions of the rural labor force and farmland area determine the allocation of farmland in rural regions, as well as determining the input-output relationship in the process of farmland use (Lin 1992). Many studies suggest that the presence of surplus rural labor force restricts the input of agricultural machinery and, thereby, limits economic benefits (Xie and Lu 2017). Moreover, a large labor force will also inhibit farmland transfer, and further aggravate the degree of farmland fragmentation (Latruffe and Piet 2014; Lu et al. 2018b). Contrarily, industrial development promotes the transfer of the agricultural labor force, and the contribution of the tertiary industry to the gross domestic product (GDP) often indicates the level of industrial development (Cai 2008). Additionally, the acquisition of sufficient market and technological information can promote acquisition of more advanced production technologies and optimization of the input-output relationship for farmland use, whereas the business volume of postal services can reflect the regional level of information communication (Chen et al. 2009). According to location theory, the provision of adequate highway transportation measures can reduce production costs and, thereby, improve the economic benefits of farmland use (Xiao et al. 2015). Furthermore, to improve the output of farmland use, the Chinese government has established a Special Guidance Department on Agricultural Technology to provide technical guidance on the use of farmland, and the number of people working in this department usually indicates the level of governmental investment (Wang and Li 2014). Since 2006, a series of policies that support and benefit agricultural development has been promulgated in China, including the abolishment of agricultural taxes and provision of an increase in agricultural subsidies. Such policies cannot only solve the issue of insufficient funds in agricultural production but also improve the economic revenues of farmland use (Wang et al. 2018). Hence, in this study, the aforementioned indicators are the control variables, as shown in Table 1.
Model establishment and estimation
Non-dynamic panel model
Model establishment
According to the “Literature review”, the EEFU is the result of comprehensive influence of external factors on farmland use. The Stochastic Impacts by Regression on Population, Affluence and Technology (STIRPAT) model is classically used to analyze the impact of external factors and human activities on the environment (Ehrlich and Holdren 1971). Therefore, the STIRPAT model can be used as a basic model to analyze the FF’s impact on the EEFU. The specific forms of the STIRPAT model are as follows:
where I denotes the environment pressure; P is the number of people; A denotes the per capita affluence; T represents the technology level; e is the error term; a denotes the model’s fit coefficient; and b, c, and d denote the elastic coefficients of P, A, and T, respectively.
To eliminate heteroscedasticity, this model usually uses natural logarithmic transformation:
Since panel data may have individual effects in large-scale studies, the removal of individual-specific means—a traditional method—is usually required to eliminate individual effects. Along with obtaining the index selection result, we expanded Equation (8) to incorporate the influence factors of the EEFU to examine FF’s impact on the EEFU. The non-dynamic panel model is defined as follows:
where X denotes the control variables, including lnFAPC, lnLID, lnLHR, lnBVPS, lnPEPI, and FAP; β is the regression coefficient; ε is the scalar value assumed to be identical and independent; and i and t denote the individual and time, respectively.
Estimation and test
According to the empirical analysis practice, unit root tests and cointegration test should be performed on panel data to avoid spurious issues (Hou et al. 2019b). However, short panel data usually cannot reflect the time trend and the stationary nature of the sequence; hence, Baltagi (2008) suggests that the non-stationarity of short panel data be ignored. In addition, fixed-effect, random-effect, and mixed-effect models are used to estimate panel data; therefore, F test (Baltagi et al. 2007; Wooldridge 2005), Hausman test (Hausman 1978), and LM test (Breusch and Pagan 1980) were used to determine which estimation model we should select. Further, the cross-section correlation, heteroscedasticity, and sequence correlation in the panel data would render ineffective the traditional estimation method based on standard deviation statistical inference (Driscoll and Kraay 1998). Based on this issue, Hoechle (2007) proposed a regression method called the “Driscoll-Kraay standard error” which effectively overcomes the shortcomings of cross-section correlation, heteroscedasticity, and sequence correlation in panel data.
Threshold model
According to the method by Hansen (1999), we constructed a threshold model to analyze FF’s impact on the EEFU. There may be multiple thresholds; however, multiple thresholds and a single threshold are set in the same manner. Therefore, we used a single-threshold model to explain the model establishment process. This single-threshold model is defined as follows:
where I(·) is the indicator function which usually takes a value of 0 or 1; tdv represents the threshold dependent variable and denotes lnPScovi, t, lnAWMSIi, t, and lnMNNi, t, respectively; lnFAPC is the threshold variable; θ is the threshold value that should be estimated; and εi, t is assumed to be independent and identical. The other variables have the same concepts as those defined in Equation (9). When one among lnPScovi, t, lnAWMSIi, t, and lnMNNi, t is selected as the threshold dependent variable, the remaining variables will be added to the control variables. Moreover, single, double, and triple thresholds are, in turn, identified by a self-sampling process. For threshold effect test and significance test of threshold value, refer to the study by Hansen (1999).
Results and discussion
Analysis of the regression results of non-dynamic panel data
In Table 2, (1), (2), and (3) represent the estimation results of the mixed-, fixed-, and random-effect models, respectively. Further, the F test result rejected the original hypothesis H0 : all ui = 0, indicating that the fixed-effect model is superior to the mixed-effect model. Simultaneously, according to the Hausman test results, p < 0.01. Accordingly, we rejected the original hypothesis that there is no difference between the random- and fixed-effect models. The fixed-effect model is more suitable than the random-effect model for estimating FF’s impact on the EEFU. However, the Pesaran test (Pesaran et al. 2004), Wooldridge test (Wooldridge 2005), and modified Wald tests (Greene 2000) revealed that the corresponding p values were less than 0.01, indicating that panel data could not exclude the possibility of cross-section correlation, heteroscedasticity, and sequence correlation. Therefore, we used the fixed-effect Driscoll-Kraay model’s standard error to estimate the regression coefficient, and Table 3 depicts the results of this estimate.
Among the control variables, lnFAPC, lnLHR, lnLID, and FAP have a significant effect on the lnEEFU. Conversely, lnBVPS and lnPEPI have a significant negative effect on lnEEFU. It can thus be concluded that continuing to reduce the rural population, improve rural traffic conditions, and increase favorable agriculture policy will play an important role in improving the EEFU. This is similar to the results of other studies (Xie and Lu 2017; Hou et al. 2019a). Specifically, rural population transfer and favorable agriculture policy promote the substitution of agricultural machinery for rural labor, thus reducing the cost of farmland use. The improvement of traffic conditions is conducive to the cultivation of cash crops, thus raising incomes. In contrast, the effect of the development of the tertiary industry on the EEFU is quite constrained, as industrial development has caused a large number of young laborers to leave the countryside, leaving behind the elderly. Contrary to expectations, lnBVPS has a negative effect on lnEEFU. This could be because farmers pay more attention to economic benefits and ignore the negative environmental benefits brought by farmland use, which is also the root cause of a large number of chemical fertilizers and pesticides lost in the process of farmland use in China. In addition, according to the negative effects of lnPEPI on lnEEFU, although it is only at 10% significance, we speculate that guidance department on agricultural technology still focuses on the economic rather than ecological benefits of farmland use. Further, three types of FF were observed to have no significant impact on the EEFU, for which there may be two reasons: first, landscape-level FF does not affect farmland use activities; hence, FF’s impact on the EEFU is not significant. Second, there is a nonlinear relationship between the FF and the EEFU. So, it is necessary to use threshold model to test the impact of landscape-scale FF on the EEFU.
Analysis of the threshold regression result
Table 3 reports that the p statistic of the F test is less than 0.01; accordingly, we rejected the original hypothesis that there is no threshold effect. According to the F statistics and 95% confidence interval, there is a single threshold for the impacts of the three types of FF (size, shape, and distance) on the EEFU. In this case, we used a single-threshold model for the regression analysis.
Table 4 reports the regression results with lnPScov, lnAWMSI, and lnMNN as the threshold-dependent variables, respectively. We found that lnPScov and lnMNN have similar effects on lnEEFU. When lnFAPC does not exceed the threshold value (1.548 and 1.542), size and distance fragmentation promote EEFU improvement. On the contrary, they will inhibit EEFU improvement. Specifically, when lnFAPC is lower than threshold value, spatially dispersed and different-scale farmland plot can satisfy the requirements of farmers’ agricultural production activities. In fact, landscape-scale FF does not have a direct impact on the EEFU at this time. On the contrary, landscape-scale FF creates conditions for the farmer-/plot-scale FF’s positive effect on EEFU. For example, a small plot reduces fertilizer input and its undesirable output and may also reduce the cost of farmland use because there is no need to manage different space of the plot (as stages 1 or 2 in Fig. 1) (Sklenicka 2016). However, in the context of social economy development, the increase of FAPC also increases the plot size managed by farmers, thus increasing the effect of landscape-FF on farmers’ farmland use activities (as stages 3 or 4 in Fig. 1).
Unlike the two aforementioned types of FF, lnAWMSI has a negative effect on lnEEFU, irrespective of whether the lnFAPC crosses the threshold value (1.373). The possible reason is shape fragmentation restricts the input of agricultural machinery, increases the cost, and reduces the economic benefits of farmland use. Comparing the regression coefficient, we find that when the lnFAPC does not exceed the threshold value, the regression coefficient of shape fragmentation is only −0.0677. In contrast, the regression coefficient becomes −0.4501. In addition, the significance level of regression coefficient also changed greatly, from 5 to 10%. It is also confirmed that with the increase of FAPC, the effect of landscape-scale FF on farmland use is increasingly prominent.
The regression coefficients of other control variables were only mildly altered compared with the regression outcomes of the non-dynamic panel and threshold models; however, the significance level did not change, which demonstrates that the threshold model’s assessment findings are robust (Hansen 1999). Thus, we have reason to believe that the results of panel threshold model are more credible. This confirms our initial hypothesis that there is a nonlinear relationship between landscape-scale FF and EEFU. The above research results have strong policy implications regarding the economic and ecological benefits of farmland use. When farmers only consider economic benefits, the promotion of large-scale farmland transfer is not conducive to solving the ecological and environmental problems of farmland use. In response, in regions that exceed the threshold value, the local government should not only actively promote large-scale farmland transfer, it should also give play to the positive effects of policies such as improved traffic conditions, labor transfer, and agricultural subsidies so as to offset the negative effects of landscape-scale FF on the EEFU. On the contrary, for regions in which FAPC is lower than the threshold value, the transfer of farmland should not be promoted on a large scale, but should properly guide farmers’ chemical fertilizer reduction actions so as to solve the ecological and environmental problems of cultivated land use in combination with the positive role of other policies.
Conclusions
With the rapid development of the social economy, ecological (environmental) problems caused by farmland use are becoming increasingly prominent. Therefore, promoting a balance between economic and ecological benefits is key to realizing the sustainable utilization of farmland. At present, in the context of the promotion of large-scale farmland transfer to reduce the level of farmer-/plot-scale FF, the impact of landscape-scale FF on the EEFU remains unclear. Therefore, in this study, non-dynamic panel and threshold effect models were applied to conduct an empirical analysis based on panel datasets of the YREB’s municipal administrative regions in the years 2000, 2005, 2010, and 2015.
The study concludes that there is a nonlinear relationship between landscape-scale FF and EEFU under the constraint of lnFAPC, in which the threshold values of area, shape, and distance fragmentation are 1.548, 1.373, and 1.542, respectively. Landscape-scale FF is not always conducive to the balance of economic and ecological benefits of farmland use. Promoting large-scale farmland transfer blindly will cause the intensification of eco-environmental problems in the utilization of farmland. In contrast, combining the FAPC of different regions, the implementation of farmland transfer policy in some areas alone becomes a feasible measure to alleviate the eco-environmental impacts of farmland use. At the same time, exerting the positive effect of the shrinking rural population, improving rural traffic conditions, and increasing favorable agriculture policy have remarkable effects on improving EEFU.
Undeniably, using artificial measures to change the landscape-scale FF is also a measure to reduce the negative effect of landscape-scale FF on EEFU. However, the reclamation of farmland usually leads to the reduction and fragmentation of other types of landscapes (e.g., forest and grass), while the withdrawal of farmland from agricultural production may not be conducive to food security. Therefore, we do not recommend that the impact of landscape-scale FF on EEFU be reduced by changing the landscape characteristics of farmland.
Data availability
All data generated or analyzed during this study are included in this published article.
Notes
In China, farmlands are under the ownership of village collectives, and this ownership cannot be sold to others.
References
Ali D, Deininger K (2014) Is there a farm-size productivity relationship in African agriculture? Evidence from Rwanda. Land Econ 91:317-343. https://doi.org/10.3368/le.91.2.317
Bai YP, Deng XZ, Jiang SJ, Zhang Q, Wang Z (2018) Exploring the relationship between urbanization and urban eco-efficiency: evidence from prefecture-level cities in China. J Clean Prod 195:1487-1496. https://doi.org/10.1016/j.jclepro.2017.11.115
Balota E, Yada I, Amaral H, Nakatani A, Dick R et al (2014) Long-term land use influences soil microbial biomass P and S, phosphatase and arylsulfatase activities, and S mineralization in a Brazilian oxisol. Land Degrad Dev 25:397-406. https://doi.org/10.1002/ldr.2242
Baltagi B (2008) Econometric Analysis of Panel Data. John Wiley & Sons, West Sussex, England
Baltagi B, Egger P, Pfaffermayr M (2007) Estimating models of complex FDI: are there third-country effects? J Econ 140(1):260-281. https://doi.org/10.1016/j.jeconom.2006.09.009
Bellon MR (1996) Landholding fragmentation: are folk soil taxonomy and equity important? A case study from Mexico. Hum Ecol 24:373-393. https://doi.org/10.1007/BF02169395
Bellon MR, Taylor JE (1993) "Folk" soil taxonomy and the partial adoption of new seed varieties. Economic Development and Cultural Change, University of Chicago Press 41(4):763-786. https://doi.org/10.1086/452047
Bonfiglio A, Arzeni A, Bodini A (2017) Assessing eco-efficiency of arable farms in rural areas. Agric Syst 151:114-125
Breusch TS, Pagan AR (1980) The Lagrange multiplier test and its applications to model specification in econometrics. Rev Econ Stud 47(1):239-253. https://doi.org/10.2307/2297111
Buckwell A, Davidova S (1993) Potential implications for productivity of land reform in Bulgaria. Food Policy 18:493-506. https://doi.org/10.1016/0306-9192(93)90006-W
Cai F (2008) The terminative era of unlimited supply of labor. Financ Econ 3:16-17
Cao H, Zhu XQ, Heijman W, Zhao K (2020) The impact of land transfer and farmers’ knowledge of farmland protection policy on pro-environmental agricultural practices: the case of straw return to fields in Ningxia, China. J Clean Prod 277:123701. https://doi.org/10.1016/j.jclepro.2020.123701
Carter MR, Yao Y (2002) Local versus global separability in agricultural household models: the factor price equalization effect of land transfer rights. Am J Agric Econ 84:702-715. https://doi.org/10.1111/1467-8276.00329
Charles V, Aparicio J, Zhu J (2019) The curse of dimensionality of decision-making units: a simple approach to increase the discriminatory power of data envelopment analysis. Eur J Oper Res 279(3):929-940. https://doi.org/10.1016/j.ejor.2019.06.025
Chen Z, Huffman WE, Rozelle S (2009) Farm technology and technical efficiency: evidence from four regions in China. China Econ Rev 20:153-161. https://doi.org/10.1016/j.chieco.2009.03.002
Cooper WW, Seiford LM, Tone K, Zhu J (2007) Some models and measures for evaluating performances with DEA: past accomplishments and future prospects. J Prod Anal 28:151-163. https://doi.org/10.1007/s11123-007-0056-4
Dalu T, Wasserman R, Wu Q, Froneman W, Weyl OLF (2018) River sediment metal and nutrient variations along an urban-agriculture gradient in an arid austral landscape: implications for environmental health. Environ Sci Pollut Res 25(3):2842-2852. https://doi.org/10.1007/s11356-017-0728-1
Del Corral J, Perez JA, Roibas D (2011) The impact of land fragmentation on milk production. J Dairy Sci 94:517-525. https://doi.org/10.3168/jds.2010-3377
Deng XZ, Huang JK, Rozelle S, Zhang JP, Li ZH (2015) Impact of urbanization on cultivated land changes in China. Land Use Policy 45:1-7. https://doi.org/10.1016/j.landusepol.2015.01.007
Di Falco S, Penov I, Aleksiev A, Rensburg TMV (2010) Agrobiodiversity, farm profits and land fragmentation: evidence from Bulgaria. Land Use Policy 27:763-771. https://doi.org/10.1016/j.landusepol.2009.10.007
Dijk TV (2002) Scenarios of Central European land fragmentation. Land Use Policy 20:149-158. https://doi.org/10.1016/S0264-8377(02)00082-0
Driscoll JC, Kraay AC (1998) Consistent covariance matrix estimation with spatially dependent data. Rev Econ Stat 80:549-560 https://www.jstor.org/stable/2646837
Ehrlich PR, Holdren JP (1971) Impact of population growth. Science 171:1212-1217. https://doi.org/10.1126/science.171.3977.1212
Feng YG, Peng J, Deng ZB, Wang J (2015) Spatial-temporal variation of cultivated land’s utilization efficiency in China based on the dual perspective of non-point source pollution and carbon emission. China Population, Resources and Environment 25(8):18-25. https://doi.org/10.3969/j.issn.1002-2104.2015.08.003
Greene WH (2000) Econometric Analysis, 4th edn. Prentice Hall, Upper Saddle River, New Jersey
Guo Y, Zhong FN, Ji YQ (2019) Economies of scale and farmland transfer preferences of large-scale households: an analysis based on land plots. Chin Rural Econ 4:7-21
Hansen BE (1999) Threshold effect in non-dynamic panels: estimation, testing, and inference. J Econ 93:345-368. https://doi.org/10.1016/S0304-4076(99)00025-1
Hausman JA (1978) A specification tests in econometrics. Econometrica 46:1251-1271. https://doi.org/10.2307/1913827
Hoechle D (2007) Robust Standard Errors for Panel Regressions with Cross-sectional Dependence. Stata Journal 7:281–312. https://doi.org/10.1177/1536867X0700700301
Hou XH, Liu JM, Zhang DJ, Zhao MJ, Xia CY (2019a) Impact of urbanization on the eco-efficiency of cultivated land utilization: a case study on the Yangtze River Economic Belt, China. J Clean Prod 238:117916. https://doi.org/10.1016/j.jclepro.2019.117916
Hou XH, Liu JM, Zhang DJ (2019b) Regional sustainable development: the relationship between natural capital utilization and economic development. Sustain Dev 27:183-195. https://doi.org/10.1002/sd.1915
Huang JK, Wang XB, Rozelle S (2013) The subsidization of farming households in China’s agriculture. Food Policy 41:124-132. https://doi.org/10.1016/j.foodpol.2013.04.011
Janus J, Mika M, Leń P, Siejka M, Taszakowski J (2018) A new approach to calculate the land fragmentation indicators taking into account the adjacent plots. Surv Rev 50:1-7. https://doi.org/10.1080/00396265.2016.1210362
Jia LL, Petrick M (2014) How does land fragmentation affect off-farm labor supply: panel data evidence from China. Agric Econ 45(3):369-380. https://doi.org/10.1111/agec.12071
Jin G, Deng XZ, Zhao XD, Guo BS, Yang J (2018) Spatiotemporal patterns in urbanization efficiency within the Yangtze River Economic Belt between 2005 and 2014. J Geogr Sci 28(8):1113-1126. https://doi.org/10.1007/s11442-018-1545-2
Jin G, Li ZH, Deng XZ, Yang J, Chen DD, Li WQ (2019) An analysis of spatiotemporal patterns in Chinese agricultural productivity between 2004 and 2014. Ecol Indic 105:591-600. https://doi.org/10.1016/j.ecolind.2018.05.073
Kawasaki K (2010) The costs and benefits of land fragmentation of rice farms in Japan. Aust J Agric Resour Econ 54:509-526. https://doi.org/10.1111/j.1467-8489.2010.00509.x
Latruffe L, Piet L (2014) Does land fragmentation affect farm performance? A case study from Brittany, France. Agric Syst 129:68-80. https://doi.org/10.1016/j.agsy.2014.05.005
Li SF, Li XB (2019) The mechanism of farmland marginalization in Chinese mountainous areas: evidence from cost and return changes. J Geogr Sci 29(4):531-548. https://doi.org/10.1007/s11442-019-1613-2
Lin J (1992) Rural reforms and agricultural growth in China. Am Econ Rev 82:34-51 https://www.jstor.org/stable/2117601
Liu YS (2018) Introduction to land use and rural sustainability in China. Land Use Policy 74:1-4. https://doi.org/10.1016/j.landusepol.2018.01.032
Liu YS, Yang YY, Li YR, Li JT (2017) Conversion from rural settlements and arable land under rapid urbanization in Beijing during 1985-2010. J Rural Stud 51:141-150. https://doi.org/10.1016/j.jrurstud.2017.02.008
Liu YS, Zou LL, Wang YS (2020) Spatial-temporal characteristics and influencing factors of agricultural eco-efficiency in China in recent 40 years. Land Use Policy 97:104794. https://doi.org/10.1016/j.landusepol.2020.104794
Long HL (2014) Land use policy in China: introduction. Land Use Policy 40:1-5. https://doi.org/10.1016/j.landusepol.2014.03.006
Lu XH, Kuang B, Li J (2018a) Regional differences and its influencing factors of cultivated land use efficiency under carbon emission constraint. J Nat Resour 33(4):657-668. https://doi.org/10.11849/zrzyxb.20170454
Lu H, Xie HL, He YF, Wu ZL, Zhang XM (2018b) Assessing the impacts of land fragmentation and plot size on yields and costs: a translog production model and cost function approach. Agric Syst 161:81-88. https://doi.org/10.1016/j.agsy.2018.01.001
Mcpherson M (1982) Land fragmentation: a selected literature review. In: Development Discussion Papers. 141. Harvard University, p 85
Niroula G, Thapa G (2005) Impacts and causes of land fragmentation, and lessons learned from land consolidation in South Asia. Land Use Policy 22(4):358-372. https://doi.org/10.1016/j.landusepol.2004.10.001
Niroula G, Thapa G (2007) Impacts of land fragmentation on input use, crop yield and production efficiency in the mountains of Nepal. Land Degrad Dev 18(3):237-248. https://doi.org/10.1002/ldr.771
Ntihinyurwa PD, de Vries WT, Chigbu UE, Dukwiyimpuhwe PA (2019) The positive impacts of farm land fragmentation in Rwanda. Land Use Policy 81:565-581. https://doi.org/10.1016/j.landusepol.2018.11.005
Pesaran MH, Schuermann T, Weiner SM (2004) Modeling regional interdependencies using a global error-correcting macroeconometric model. J Bus Econ Stat 22(2):129-162. https://doi.org/10.1198/073500104000000019
Population Investigation Division, National Bureau of Statistics of China (2020) China Statistical Yearbook. China Statistics Press, Beijing
Prokop P, Kruczkowska B, Syiemlieh HJ, Bucała-Hrabia A (2018) Impact of topography and sedentary swidden cultivation on soils in the hilly uplands of North-East India. Land Degrad Dev 29:2760-2770. https://doi.org/10.1002/ldr.30180
Ren YF, Fang CL, Lin XQ, Sun S, Li GD, Fan B (2019) Evaluation of the eco-efficiency of four major urban agglomerations in coastal eastern China. J Geogr Sci 29:1315-1330. https://doi.org/10.1007/s11442-019-1661-7
Riitters K, Costanza J (2019) The landscape context of family forests in the United States: anthropogenic interfaces and forest fragmentation from 2001 to 2011. Landsc Urban Plan 188:64-71. https://doi.org/10.1016/j.landurbplan.2018.04.001
Ruan S, Zhuang Y, Hong S, Zhang L, Wang Z, Tang X, Wen W (2020) Cooperative identification for critical periods and critical source areas of nonpoint source pollution in a typical watershed in China. Environ Sci Pollut Res 27(10):10472-10483. doi:https://doi.org/10.1007/s11356-020-07630-w
Sabiha N, Salim R, Rahman S (2017) Eco-efficiency of high-yielding variety rice cultivation after accounting for on-farm environmental damage as an undesirable output: an empirical analysis from Bangladesh. Australian of Journal of Agricultural and Resource Economics 61(2):247-264. https://doi.org/10.1111/1467-8489.12197
Schaltegger S, Sturm A (1990) Öologische Rationalitat German/in English: environmental rationality. Unternehmung 4:117-131
Sklenicka P (2016) Classification of farmland ownership fragmentation as a cause of land degradation: a review on typology, consequences, and remedies. Land Use Policy 57:694-701. https://doi.org/10.1016/j.landusepol.2016.06.032
Sklenicka P, Zouhar J, Trpáková I, Vlasák J (2017) Trends in land ownership fragmentation during the last 230 years in Czechia, and a projection of future developments. Land Use Policy 67:640-651. https://doi.org/10.1016/j.landusepol.2017.06.030
Strijker D (2005) Marginal lands in Europe: causes of decline. Basic Appl Ecol 6:99-106. https://doi.org/10.1016/j.baae.2005.01.001
Tan S, Heerink NBM, Kuyvenhove A (2010) Impact of land fragmentation on rice producers' technical efficiency in South-East China. NJAS-Wageningen Journal of Life Sciences 57(2):117-123. https://doi.org/10.1016/j.njas.2010.02.001
Thenail C, Baudry J (2004) Variation of farm spatial land use pattern according to the structure of the hedgerow network (bocage) landscape: a case study in northeast Brittany. Agric Ecosyst Environ 101:53-72. https://doi.org/10.1016/S0167-8809(03)00199-3
Thenail C, Joannon A, Capitaine M, Souchère V, Mignolet C, Schermann N, di Pietro F, Pons Y, Gaucherel C, Viaud V, Baudry J (2009) The contribution of crop-rotation organization in farms to crop-mosaic patterning at local landscape scales. Agric Ecosyst Environ 131:207-219. https://doi.org/10.1016/j.agee.2009.01.015
Tone K (2002) A slacks-based measure of efficiency in data envelopment analysis. Eur J Oper Res 143:32-41. https://doi.org/10.1016/S0377-2217(01)00324-1
Verheijen FGA, Jones RJA, Rickson RJ, Smith CJ (2009) Tolerable versus actual soil erosion rates in Europe. Earth Sci Rev 94:23-38. https://doi.org/10.1016/j.earscirev.2009.02.003
Wang LJ, Li H (2014) Cultivated land use efficiency and the regional characteristics of its influencing factors in China: by using a panel data of 281 prefectural cities and the stochastic frontier production function. Geogr Res 33:1995-2004. https://doi.org/10.11821/dlyj201411001
Wang JY, Zhang ZW, Liu YS (2018) Spatial shifts in grain production increases in China and implications for food security. Land Use Policy 74:204-213. https://doi.org/10.1016/j.landusepol.2017.11.037
Wooldridge JM (2005) Fixed-effects and related estimators for correlated random-coefficient and treatment-effect panel data models. Rev Econ Stat 87(2):385-390. https://doi.org/10.1162/0034653053970320
Wu YZ, Shan LP, Guo Z, Peng Y (2017) Cultivated land protection policies in China facing 2030: dynamic balance system versus basic farmland zoning. Habitat Int 69:126-138. https://doi.org/10.1016/j.habitatint.2017.09.002
Xiao R, Sl S, Mai GC, Zhang ZH, Yang CX (2015) Quantifying determinants of cash crop expansion and their relative effects using logistic regression modeling and variance partitioning. Int J Appl Earth Obs Geoinf 34:258-263. https://doi.org/10.1016/j.jag.2014.08.015
Xie HL, Lu H (2017) Impact of land fragmentation and non-agricultural labor supply on circulation of agricultural land management rights. Land Use Policy 68:355-364. https://doi.org/10.1016/j.landusepol.2017.07.053
Xu XB, Hu HZ, Tan Y, Yang GS, Zhu P, Jiang B (2019) Quantifying the impacts of climate variability and human interventions on crop production and food security in the Yangtze River Basin, China, 1990-2015. Sci Total Environ 665:379-389. https://doi.org/10.1016/j.scitotenv.2019.02.118
Yang L, Wang KL, Geng JC (2018) China's regional ecological energy efficiency and energy saving and pollution abatement potentials: an empirical analysis using epsilon-based measure model. J Clean Prod 194:300-308. https://doi.org/10.1016/j.jclepro.2018.05.129
Yu FW (2016) New ideas of green development of Xi Jinping and Green Transformation of Agriculture. China Rural Survey 5:1-9
Zhang F, Tiyip T, Feng ZD, Kung HT, Johnson VC, Ding JL, Tashpolat N, Sawut M, Gui DW (2015) Spatio-temporal patterns of land use/cover changes over the past 20 years in the middle reaches of the Tarim River, Xinjiang, China. Land Degrad Dev 26:284-299. https://doi.org/10.1002/dr.2206
Zhang DJ, Jia QQ, Xu X, Yao SB, Chen HB, Hou X, Zhang J, Jin G (2019) Assessing the coordination of ecological and agricultural goals during ecological restoration efforts: a case study of Wuqi County, Northwest China. Land Use Policy 82:550-562. https://doi.org/10.1016/j.landusepol.2019.01.001
Zhang M, Wang X, Liu C, Lu J, Qin YH, Mo Y, Xiao P, Liu Y (2020) Identification of the heavy metal pollution sources in the rhizosphere soil of farmland irrigated by the Yellow River using PMF analysis combined with multiple analysis methods-using Zhongwei city, Ningxia, as an example. Environ Sci Pollut Res 27(14):16203-16214. https://doi.org/10.1007/s11356-020-07986-z
Zhao LP, Hou DL, Wang YP, He K (2016) Study on the impact of urbanization on the environmental technology efficiency of grain production. China Population, Resources and Environment 26:153-162. https://doi.org/10.3969/j.issn.1002-2104.2016.03.019
Zhong TY, Zhang XL, Huang XJ, Liu F (2019) Blessing or curse? Impact of land finance on rural public infrastructure development. Land Use Policy 85:130-141. https://doi.org/10.1016/j.landusepol.2019.03.036
Zhou X, Chen W, Wang YN, Zhang DJ, Wang Q, Zhao M, Xia X (2019) Suitability evaluation of large-scale farmland transfer on the Loess Plateau of Northern Shaanxi, China. Land Degrad Dev 30:1258-1269. https://doi.org/10.1002/ldr.3313
Funding
This work was funded by MOE (Ministry of Education of China) Humanities and Social Sciences Fund (Grant No. 18YJCZH049), China Postdoctoral Science Foundation (Grant No. 2018M633596 and 2020M683595), Natural Science Basic Research Plan in Shaanxi Province of China (Grant No. 2018JQ7005 and 2020JM-170), and National Natural Science Foundation of China (Grant No. 71873098 and 42071416).
Author information
Authors and Affiliations
Contributions
Xianhui Hou and Jingming Liu organized and completed the original draft, and Xianhui Hou, Jingmin Liu, and Minjuan Zhao proposed research ideas and methods and finalized the paper, Daojun Zhang and Yuqing Yin collected and analyzed data.
Corresponding authors
Ethics declarations
Ethics approval and consent to participate
Not applicable.
Consent for publication
Not applicable.
Competing interest
The authors declare that they have no competing interest.
Additional information
Responsible Editor: Philippe Garrigues
Publisher’s note
Springer Nature remains neutral with regard to jurisdictional claims in published maps and institutional affiliations.
Rights and permissions
About this article
Cite this article
Hou, X., Liu, J., Zhang, D. et al. Effect of landscape-scale farmland fragmentation on the ecological efficiency of farmland use: a case study of the Yangtze River Economic Belt, China. Environ Sci Pollut Res 28, 26935–26947 (2021). https://doi.org/10.1007/s11356-021-12523-7
Received:
Accepted:
Published:
Issue Date:
DOI: https://doi.org/10.1007/s11356-021-12523-7