Abstract
This research article aims to investigate the moderating role of financial development in Environmental Kuznets Curve (EKC) in the context of Malaysia for the period 1970–2016. As the time series variables are integrated of different order therefore, Auto-Regressive Distributed Lag (ARDL) model has been employed to estimate the long-run equilibrium relationship among the variables. The results indicate that EKC does exist for Malaysia and financial development has negative impact on carbon emission. Moreover, financial development is found to have significant moderating impact on income environment relation. More financial development brings early turning point of the EKC. The results recommend that financial development can be used as one of the policy measures to reduce the environmental cost of economic growth in Malaysia.
Similar content being viewed by others
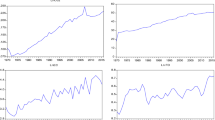
Explore related subjects
Discover the latest articles, news and stories from top researchers in related subjects.Avoid common mistakes on your manuscript.
Introduction
The Environment Kuznets Curve (EKC) has attracted the policies and priorities of the development economists since 1990s. It describes continues economic growth as a cause, as well as remedy, to the environmental problem of the day. The seminal work to establish the EKC theory came from the various research works (Beckerman 1992; Grossman and Krueger 1995; Panayotou 1993; Shafik and Bandyopadhyay 1992). According to the EKC hypothesis, economic growth generates environmental pollution at the early stages of economic development. However, as the economy reaches to a sufficient level of economic development, economic growth generates the conditions that are conducive for a better environment. Consequently, environmental pollution forms a nonlinear relationship with economic growth as at the first place it increases with the increase
EKC has gained increasing popularity among the academicians and policymakers during the last two decades. Since the debate started over the existence and shape of the EKC, policymakers all over the world have been carefully watching the pros and cons of the EKC. The critical question for them remained unresolved “whether economic growth should continue to be the main priority, with protection of the environment a secondary consideration, or whether explicit policies to control environmental degradation are urgently required today.”
Several empirical studies such as Arrow et al. (1995), Bradford et al. (2000), Dinda (2004), Dasgupta et al. (2006), Aslanidis (2009), Costantini and Martini (2010), Chowdhury and Moran (2012), Al Sayed and Sek (2013), and Chow and Li (2014) have studied the income-environment nexus to validate the EKC theory. However, these studies have diverse empirical outcome as they found different peak turning point of the EKC.
The peak turning point of the EKC has important policy implications for developing countries. The steeper EKC implies that high environmental cost is attached to economic growth, while the flatter EKC implicates low environmental cost of economic growth. The developing countries cannot compromise over economic growth as it is critical for them to eradicate unemployment and poverty. The debate, therefore, is on how developing countries can achieve the flatter EKC. The conclusive results about the slope of the EKC and its determining factor in the literature are still pending.
The income environment nexus took new twist with the introduction of additional variables that have impact on the slope and shifting of the EKC. The pollution in a country in fact does not depend necessarily on its income only, population, financial development, urbanization, and open trade are considered other important determinants. Similarly, the influence of financial development on the pollution has not been given due consideration.
Financial development affects the pollution in a country through different pathways. First, it can cause the pollution by increasing the access of industrial and commercial firms to the capital. Second, FD attracts foreign direct investment inflows that speed up the economic growth process, facilitates research and development (R&D), and, therefore, changes the dynamics of environmental improvement. Third, it provides developing countries with the opportunities to use novel and updated environment-friendly technologies to produce clean production that results in improved local and global environment. Fourth, well-functioning financial system also supports foreign trade activities that lead to more economic growth and better environment (Sadorsky 2011). Finally, contrary to the first and the second pathways as noted by Tamazian et al. (2009), increased economic growth spurred by financial development may increase environmental degradation and industrial pollution. Moreover, it is also argued that financial sector development relies more on the use of energy that leads to more environmental degradation
In this backdrop, current study examines the moderating role of FD to determine the slope of the EKC in the context of Malaysia that is a middle-income country with a per capita income of $ 11078.27 in 2018 and 8.53 metric ton CO2 emission per person in 2016 (The World Bank 2018). The bank estimates that CO2 emission from Malaysia has increased by 3.09% from 1997 to 2018. According to Zainuddin (2017), Malaysia has planned to slash CO2 emission by 25% as part of a national effort to contribute in global efforts to tackle the dangers of climate change and global warming.
To the best of the author’s knowledge, the studies that have examined the moderating role of FD to minimize the environmental cost of economic growth in the EKC framework especially in the context of Malaysia are scarce. Previous studies on Malaysia such as Saboori et al. (2012), Saboori and Sulaiman (2013), Lau et al. (2014), Begum et al. (2015), and Gill et al. (2017b) have examined the links between pollution, trade, renewable energy, energy consumption, economic growth, and FDI. Nevertheless, these studies have overlooked the impact of FD on environment quality, especially when the influence of FD on the slope of the EKC has not been determined yet. Moreover, these research studies also have diverse empirical output about the slope and height of the EKC for Malaysia. Mixed outcome of the research studies suggests that existence of the EKC in middle-income countries like Malaysia is a still ongoing debate. Current study, therefore, backs the existing literature in this regard.
Literature review
Despite the plethora of the empirical studies, the debate remained unresolved about the existence and shape of EKC. The empirical literature about the EKC started from pioneering work of Beckerman (1992), Grossman and Krueger (1995), and Panayotou (1995). They examined links between economic growth and several environmental measures and concluded that environment quality gradually improves with the process of economic development. Economic growth initially leads to environmental degradation; however, after a certain position of economic development, it leads to the improvement of environment.
Following these seminal work, a number of empirical studies such as Raymond (2004), Stern (2004), Vollebergh et al. (2005), Managi and Jena (2008), Fodha and Zaghdoud (2010), and Gill et al. (2017a, b) studied the growth-environment nexus. These studies employed different specifications, estimation techniques, and data set and found a range of conflicting results. Some studies reported a monotonically increasing relation between income and environment, other found nonlinear inverted U-shaped relation. Some studies also found N-shaped relationship. Moreover, studies that confirmed the EKC relationship between income and environment did not agree on any unique peak turning point of the EKC.
The literature on the linkages between financial development and environment is also in infancy state. Some studies found negative influence of financial development on environment while others found positive. For instance, Dasgupta et al. (2001) showed that well-established financial system provide enabling environment to the firms to lower CO2 emission. Cole and Elliott (2005) claimed that developed financial tools like treasury bonds, leasing, loans, and financial derivatives enable the small- and medium-scale companies to attain the economies of scale and to lessen the usage of the natural resources. Claessens and Feijen (2007) also claimed that financial development lead to better environmental performance.
Tamazian et al. (2009) examined the influence of financial development on environmental quality in South Africa, Brazil, India, China, and Russia (BRICS) countries for the period 1992–2004. They found that both economic growth and financial development lead to environmental improvement in the long run. Tamazian and Rao (2010) claimed that financial development played a critical role in transitional economies for environmental disclosure. However, they warned that financial development might prove harmful if it was not supported by institutional reforms.
These claims are also evident in the empirical investigation of Yuxiang and Chen (2011). They argued that financial development plays a key role to promote technological spillover that in return lead to environmental efficiencies and reduction in GHG emissions. Jalil and Feridun (2011) found a negative significant coefficient on financial development against CO2 emission in China. Similarly, Ozturk and Acaravci (2013) concluded a causality link running from financial development to per capita CO2 emission in Turkey implying that financial development reduces the pollution. According to recent study by Nasreen et al. (2017), financial stability helps to improve the environment and makes the EKC to exist. They investigated these nexuses in South Asian countries for the period 1980–2012.
On the contrary, various studies also claim a negative impact of financial development on the environmental performance. For instance, Sadorsky (2010) and Zhang (2011) claimed a pollution enhancing role of financial development. According to them, financial development reduces financing costs that leads to increases in industrial and commerce activities and, therefore, increases the CO2 emission. Moreover, financial development also spurs consumption of electronics by facilitating consumers with loans that also eventually lead to more emissions of greenhouse gasses (GHG). Shahbaz and Lean (2012) claimed that financial development facilitates FDI that leads to increase in CO2 emissions in developing countries. Similarly, Lee et al. (2015) did not support the EKC hypothesis and role of financial development in mitigating the GHG emission.
Abid (2017) studied the impact of financial development on the EKC in African, Middle Eastern and European countries for the period 1990–2011 using generalized method of moments (GMM). He also found a monotonically increasing relation between economic growth and CO2 emission in all sample countries and, therefore, rejected the EKC hypothesis. Hence, there are mixed and inconclusive findings regarding the existence of the EKC. Also, the role of financial development in moderating the income environment relation has been less explored.
Methodology
Model
To estimate the EKC relationship between income and environment, the following basic general model has been used by Shafik (1994) and Grossman and Krueger (1995).
In Eq. 1, CO2 is per capita emission, Y stands for per capita GDP, Z represents other determinants of CO2, and utis an error term of the model. Based on the EKC hypothesis, β1 is likely to be positively significant and β2 is negatively significant.
As the current study aims to estimate the impact of financial development (FD) on the EKC, therefore, FD is considered as an independent variable in the EKC model. Moreover, to avoid any misspecification of the model, two important determinants of CO2 emission, foreign direct investment (FDI) and energy consumption (EC), are also included in Eq. 2.
The turning point of the EKC where environment starts to improve with further economic growth is calculated by using Eq. 3.
Several studies that advocate the role of institutional factors in income environment relation use these factors as explanatory variables. In this specification, absent variables cannot affect the turning point of the EKC rather they can only change the amplitude of the EKC. Such studies are, therefore, unable to address the issue of the different turning point of the EKC due to different level of institutional development. In this backdrop, FD is entered in the EKC specification in Eq. 4 interactively with income, so that turning point income becomes context specific Aubourg et al. (2008).
This specification provides a way to empirically investigate the different turning points of the EKC corresponding to different level of financial development. According to Aubourg et al. (2008), this specification is unique in terms of tracing out the true impact of any variable on the turning point of the EKC. Equation 4 allows locating the turning point per capita GDP values inclusive of financial sector development. With this specification, the turning point income level of the EKC has become the function of the level of the FD in Eq. 5.
Besides, to determine the significance of interaction term in the EKC model, Wald test for zero restriction of the parameter for interaction term has been employed.
Estimation technique
The ordinary least square (OLS) method assumes that time series variables under investigation must be stationary. However, most of the time series variables are prone to the problem of nonstationary. In this case, cointegration procedure can be utilized to evaluate the possible equilibrium relationship among the variables. Mostly, three main cointegration tests are found in literature: Engle and Granger (2001) that is based on the residuals of the mode, Phillips and Hansen (1990) that is based on modified OLS, and Johansen (1995) that is based on multiple system of the equations.
According to these tests, for the presence of the cointegration relationship, the nonstationary time series variables must be integrated on the same order. However, Autoregressive Distributed Lag (ARDL) introduced by Pesaran and Shin (1998) can find the cointegration relation among the time series variables that are integrated of different order. Moreover, ARDL arrests both long-run and short-run dynamics of cointegration relation. Pesaran and Shin (1998) also claim that ARDL estimates are corrected for endogeneity and serial correlation problem.
Due to these conveniences, the current study utilizes ARDL model to estimate the relationship between time series variables. Including intercept and time trend, the general ARDL (p,q,r,s,v) for Eqs. 2 and 4 can be written in the form of Eqs. 6 and 7 respectively.
In Eqs. 6 and 7, (p, q, r, s, v) are lag order of the ARDL model. While βi and γi are the estimates of short-run and long-run cointegration equilibrium relationship. The lag structure of the models is determined by using the Akaike Information Criterion (AIC). After determining optimal lag structure, bounds test procedure is used to examine the cointegration relationship between the variables of the model. According to the bounds test
The H0 is rejected in case F-statics of bounds test exceed critical values of the upper bond. The H1 is accepted that long-run equilibrium cointegrated association does exist between the variables of the model.
In ARDL procedure, after settling the cointegration relation between time series variables, the coefficients of long-run cointegration relation are estimated. Finally, short-run ARDL model that is also known as an error-correction model (ECM) is estimated in the following way for Eq. 2 and for Eq, 4 in the form of Eqs. 8 and 9 respectively.
For the presence of the cointegration relation in the model, the coefficient on ECTt − 1 must be negatively significant. The value of λ indicates how much short-run disequilibrium is adjusted to long-run equilibrium. The diagnostic tests of normality, functional form, heteroscedasticity, and of serial correlation are also conducted to prove the robustness of the results. Moreover, to examine the stability of estimates during the study period, the stability tests like cumulative sum of squares (CUSUMQ) and cumulative sum (CUSUM) developed by Brown et al. (1975) and Chowdhury and Moran (2012) are also employed.
Data
Current study utilizes annual time series data for Malaysia spanning from 1970 to 2016. The data has been attained from the World Development Indicator 2017. CO2 emissions are measured in metric ton per capita: Yt is GDP per capita (constant 2000 US$), EC is energy consumption per capita measured in kilograms of oil equivalent, FDI is foreign direct net investment flows as a percentage of GDP, while FD is domestic credit to private sector as a percentage of the GDP.
Empirical results
The analysis starts with the descriptive statistics. Descriptive statistics explain the degree of the reliability and variations in the variables. Table 1 details the descriptive statistics of the variables that have been employed in the EKC model. It is one of the assumptions of the OLS model that dependant and independent variable must have significant variation. The high value of standard deviation and high difference between maximum and minimum values indicate that variables have substantial variation. Moreover, skewness and J.B statistics indicate that variables have normal distribution and do not pose the problem of skewness.
The correlation analysis unfolds how much variables are correlated to each other. This information is very useful in regression analysis to avoid the problem of multicollinearity. Table 2 reveals the correlation analysis where independent variables are not strongly correlated to cause the problem of multicollinearity.
The next step in the analysis is the examination of unit root properties of the time series variables. The Augmented Dickey-Fuller test (ADF) by Dickey and Fuller (1981) and Phillips-Perron (PP) test by Kwiatkowski et al. (1992) have been employed for the purpose. Moreover, Akaike Information Criterion (AIC) has been used to determine the lag length of the ADF and PP tests.
According to the ADF and PP test statistics in Tables 3 and 4, H0 that the time series variables CO2, Y, Y2, EC and FD have unit root (non-stationary) at levels cannot be rejected. However, Ho is rejected at first difference of the variables. Hence, based on ADF and PP unit root tests, it can be concluded that all the time series variables except FDI are integrated of order one I (1). While FDI is observed to be stationary at level and can be termed as integrated of order I(0). Because all the variables are not integrated in the same order, therefore, ARDL is the most suitable estimation technique for these settings.
With a view to be able to carry out ARDL analysis, it is necessary to select optimum lag structure of dependent and independent variables. For this purpose, AIC has been employed. The AIC results for Eqs. 2 and 4 are reported in Figs. 1 and 2 respectively. The optimal ARDEL model for Eq. 2 is found (1,0,0,4, 2, 0) and for Eq. 4 is (1,0,0,4, 2, 3) as these models have minimum AIC values.
The ARDL bounds test approach is aimed at establishing the presence of cointegration relationship among the variables. This approach is based on joint F-statistics. Table 5 presents the F-statistics for both ARDL (1,0,0,4, 2, 0) and ARDL (1,0,0,4, 2, 3). The F-statistics exceed the upper critical values by Pesaran et al. (2001). Therefore, H0 of no cointegration for both equations are rejected. The H1 that variables are cointegrated for both equations are accepted. Hence, it is concluded that long-run equilibrium relationship for both equations do exist.
After finding the robust proof of cointegration in both equations, the next step is the estimation of short-run and long-run relationship. The estimated results for Eq. (6) are reported in Table 6. According to the long-run results, the coefficient of Y is positive significant and of Y2 is negatively significant. It implies that per capita CO2 emission forms EKC relation with GDP per capita in Malaysia. These results are in line with Saboori et al. (2012), Saboori and Sulaiman (2013), and Apergis and Ozturk (2015). The coefficient on financial development is negatively significant. It implies that more financial development can reduce the emissions of CO2. These results are in line with Ozturk and Acaravci (2013) and Shahbaz et al. (2013) who claim that financial development curtails environmental degradation during the growth process.
The coefficient of Coint Eq. (− 1) is negatively significant that is an additional evidence of the existing of the long-run cointegrating relation. Its value − 0.57 implies that in the current year, nearly 57% disequilibrium in CO2 per capita is adjusted back to long-run equilibrium.
The models also pass through diagnostic and stability tests. The diagnostic test results for Eq. (6) are reported in lower part of Table 6. The test statistics do not reject the H0 of no heteroscedasticity, no autocorrelation, the models are correctly specified, and residuals are normally distributed. Therefore, it can be claimed that estimated coefficients are correctly specified.
It is expected that macroeconomic variables in Malaysia may have structural breaks during the sample period of the study. Therefore, the stability of the long-run coefficients along with short-run movement for both equations has been examined by CUSUM and CUSUMSQ graphs. Generally, Chow test is used to examine the stability of the estimators nevertheless, this test needs prior information of structural break in the data. While, CUSUM and CUSUMSQ tests do not need such information (Ozturk and Acaravci 2013). Figure 3 shows that the graph of the test statistics for Eq. (6) falls between the critical bonds of 5% critical level. It is, therefore, deduced that the coefficients are steady through the time period of the study.
To find the moderating role of the financial development, it is taken as interaction term (FD×Y) in Eq. 4. The results are reported in the Table 8. The coefficient on FD×Y is negatively significant. This result can be interpreted as, at any given level of income, improvement in financial development reduces CO2 emissions and at any given level of financial development increase in income lead to decline of CO2 emissions in Malaysia.
Moreover, two control variables of the model, i.e., FDI and energy consumption (EC) also have positive coefficient in both models. It implies that both control variables also contribute to the increase in CO2 emission (Table 7).
To trace out the true impact of the financial development on income environment relation, Eq. (5) can be utilized using the coefficients on Y, Y2, and (FD×Y). Taking the minimum value of the financial development, the GDP per capita turning point of the EKC where CO2 emission starts to decline with further economic growth is observed at $ 5327.1. However, taking average value of the financial development, the peak turning point per capita GDP arrives earlier at $ 4617.6. Moreover, at maximum value of financial development, the turning point GDP per capita reaches further earlier at $ 3957.6. The peak tuning point of the EKS corresponding to average, minimum, and maximum level of the financial development is given in Table 8.
These results indicate that financial development has the capacity to change the income environment relation during the process of economic development in Malaysia. By improving the financial institutions, the peak turning point of the EKC, where pollution starts to decline with further economic growth can be achieved earlier. Financial development therefore, can be considered as one of the important policy variables to minimize the environmental cost of economic growth in Malaysia.
The coefficient of Coint Eq (− 1) is negatively significant that is another proof of the existence of the long-run cointegrating relation. Its value, − 0.43, implies that in the current year, nearly 43% disequilibrium in CO2 per capita is adjusted back to long-run equilibrium.
The Wald test of coefficient restriction has been applied to examine whether the interaction term in Eq. (4) should be included or not. The results of the test are shown in Table 9. The t-statistics, F-statistics, and chi-square statistics reject the H0 at 5% significance that interaction term does not matter in Eq. (4). Therefore, H1 is accepted that interaction term does matter in the said equation. Hence, Wald test of coefficient restriction also supports the inclusion of the interaction term in the EKC model to trace the true impact of financial development on the turning point GDP per capita.
This model also passes through diagnostic and stability tests. The diagnostic test results for Eq. 7 are reported in the lower part of Table 7. The tests statistics do not reject the H0 of no heteroscedasticity, no autocorrelation, the models are correctly specified, and residuals are normally distributed. Hence, it can be claimed that estimated coefficients in this model are robust and model is correctly specified.
As a final point, the stability of the long-run coefficients along with short-run movement for Eq. 7 also has been examined by CUSUM and CUSUMSQ graphs. Figure 4 shows that graph of the test statistics for Eq. 7 fall between the critical bonds of 5% critical level. It is, therefore, concluded that the coefficients are steady through the study period.
Conclusion and policy implications
The empirical literature concludes that improved financial institutions are likely to invite more FDI and spur trade and commerce activities. It may result in more income and employment generation along with more expenditure on research and development. As this situation brings more economic growth, therefore, it affects the dynamics of the environmental performance of a country. It is well documented in the relevant literature that innovation and technological growth lead to energy efficiencies, hence, reduce environmental degradation.
The current study confirms that financial development has significant moderating role on the relationship between income and environment in Malaysia. Hence, the early turn of EKC for CO2 emission can be achieved by improving the financial institutions. Improved financial sector leads to efficient allocation of the resources and efficiency in trade and commerce activities that ultimately lead to overall economic efficiency. This efficiency reduces the environmental losses, therefore, reduces the CO2 emission.
The key findings send important signals to the policymakers that institutional development, specifically financial development, can play an important role to mitigate the GHG emissions. The global warming and climate changes have become global challenge for the world communities. The census has developed that man-made greenhouse gasses (GHG) are the primary cause of global warming and climate changes. The world economies including Malaysia have given voluntarily GHG reduction targets in the December 2015 Paris Submit. Current study recommends that Malaysia can reduce GHG by improving financial development without hurting the economic growth. The findings are parallel to the findings of Katircioğlu and Taşpinar (2017) who found positive moderating role of financial development to reduce the trade-off between pollution and economic growth.
References
Abid M (2017) Does economic, financial and institutional developments matter for environmental quality? A comparative analysis of EU and MEA countries. J Environ Manag 188:183–194
Al Sayed AR, Sek SK (2013) Environmental Kuznets curve: evidences from. Appl Math Sci 7(22):1081–1092
Apergis N, Ozturk I (2015) Testing environmental Kuznets curve hypothesis in Asian countries. Ecol Indic 52:16–22
Arrow K, Bolin B, Costanza R, Dasgupta P, Folke C, Holling CS et al (1995) Economic growth, carrying capacity, and the environment. Ecol Econ 15(2):91–95
Aslanidis N (2009) Environmental Kuznets Curves for Carbon Emissions: A Critical Survey. Working Paper No 51. Spain: University of Teramo
Aubourg RW, Good DH, Krutilla K (2008) Debt, democratization, and development in Latin America: how policy can affect global warming. J Pol Anal Manag 27(1):7–19
Beckerman W (1992) Economic growth and the environment: whose growth? Whose environment? World Dev 20(4):481–496
Begum RA, Sohag K, Abdullah SMS, Jaafar M (2015) CO2 emissions, energy consumption, economic and population growth in Malaysia. Renew Sust Energ Rev 41:594–601
Bradford DF, Schlieckert R, & Shore SH (2000) The environmental Kuznets curve: exploring a fresh specification (No. w8001). National Bureau of Economic Research
Brown RL, Durbin J, & Evans JM (1975) Techniques for testing the constancy of regression relationships over time. Journal of the Royal Statistical Society: Series B (Methodological) 37(2):149-163
Chow GC, Li J (2014) Environmental Kuznets curve: conclusive econometric evidence for CO2. Pac Econ Rev 19(1):1–7
Chowdhury RR, Moran EF (2012) Turning the curve: a critical review of Kuznets approaches. Appl Geogr 32(1):3–11
Claessens S, Feijen E (2007) Financial sector development and the millennium development goals. World Bank Working Paper No. 89
Cole MA, Elliott RJ (2005) FDI and the capital intensity of “dirty” sectors: a missing piece of the pollution haven puzzle. Rev Dev Econ 9(4):530–548
Costantini V, Martini C (2010) A modified environmental Kuznets curve for sustainable development assessment using panel data. Int J Glob Environ Issues 10(1):84–122
Dasgupta S, Laplante B, Mamingi N (2001) Pollution and capital markets in developing countries. J Environ Econ Manag 42(3):310–335
Dasgupta S, Hamilton K, Pandey KD, Wheeler D (2006) Environment during growth: accounting for governance and vulnerability. World Dev 34(9):1597–1611
Dickey DA, & Fuller WA (1981) Likelihood ratio statistics for autoregressive time series with a unit root. Econometrica: Journal of the Econometric Society :1057–1072
Dinda S (2004) Environmental Kuznets curve hypothesis: a survey. Ecol Econ 49(4):431–455
Engle R, Granger C (2001) Co-integration and error-correction: representation, estimation, and testing. Econ Soc Monogr 33:145–172
Fodha M, Zaghdoud O (2010) Economic growth and pollutant emissions in Tunisia: an empirical analysis of the environmental Kuznets curve. Energy Policy 38(2):1150–1156
Gill AR, Viswanathan KK, & Hassan S (2018) The Environmental Kuznets Curve (EKC) and the environmental problem of the day. Renewable and Sustainable Energy Reviews 81:1636–1642
Gill AR, Viswanathan KK, & Hassan S (2018) A test of environmental Kuznets curve (EKC) for carbon emission and potential of renewable energy to reduce green house gases (GHG) in Malaysia. Environment, development and sustainability 20(3):1103–1114
Grossman, Krueger (1995) Economic environment and the economic growth. Q J Econ 110(2):353–377
Jalil A, Feridun M (2011) The impact of growth, energy and financial development on the environment in China: a cointegration analysis. Energy Econ 33(2):284–291
Johansen S (1995) Likelihood-based inference in cointegrated vector autoregressive models. Oxford University Press, Oxford
Katircioğlu ST, Taşpinar N (2017) Testing the moderating role of financial development in an environmental Kuznets curve: empirical evidence from Turkey. Renew Sust Energ Rev 68:572–586
Kwiatkowski D, Phillips PC, Schmidt P, Shin Y (1992) Testing the null hypothesis of stationarity against the alternative of a unit root: how sure are we that economic time series have a unit root? J Econ 54(1-3):159–178
Lau L-S, Choong C-K, Eng Y-K (2014) Investigation of the environmental Kuznets curve for carbon emissions in Malaysia: do foreign direct investment and trade matter? Energy Policy 68:490–497
Lee J-M, Chen K-H, Cho C-H (2016) The relationship between CO2 emissions and financial development. The Singapore Economic Review 60(5):1550117
MacKinnon JG (1990) “Critical Values for Cointegration Tests”. Canada: Department of Economics, Queen’s University
Managi S, Jena PR (2008) Environmental productivity and Kuznets curve in India. Ecol Econ 65(2):432–440
Nasreen S, Anwar S, Ozturk I (2017) Financial stability, energy consumption and environmental quality: evidence from South Asian economies. Renew Sust Energ Rev 67:1105–1122
Ozturk I, Acaravci A (2013) The long-run and causal analysis of energy, growth, openness and financial development on carbon emissions in Turkey. Energy Econ 36:262–267
Panayotou T (1993) Empirical tests and policy analysis of environmental degradation at different stages of economic development (No. 992927783402676). International Labour Organization
Panayotou T (1995) Environmental degradation at different stages of economic development, Beyond Rio: the environmental crises and sustainable livelihoods in the Third World. Macmillan Press, London
Pesaran MH, Shin Y (1998) An autoregressive distributed-lag modelling approach to cointegration analysis. Econ Soc Monogr 31:371–413
Pesaran MH, Shin Y, Smith RJ (2001) Bounds testing approaches to the analysis of level relationships. J Appl Econ 16(3):289–326
Phillips PC, Hansen BE (1990) Statistical inference in instrumental variables regression with I (1) processes. Rev Econ Stud 57(1):99–125
Raymond L (2004) Economic growth as environmental policy? Reconsidering the environmental Kuznets curve. J Public Policy 24(03):327–348
Saboori B, Sulaiman J (2013) Environmental degradation, economic growth and energy consumption: evidence of the environmental Kuznets curve in Malaysia. Energy Policy 60:892–905
Saboori B, Sulaiman J, Mohd S (2012) Economic growth and CO 2 emissions in Malaysia: a cointegration analysis of the environmental Kuznets curve. Energy Policy 51:184–191
Sadorsky P (2010) The impact of financial development on energy consumption in emerging economies. Energy Policy 38(5):2528–2535
Sadorsky P (2011) Financial development and energy consumption in Central and Eastern European frontier economies. Energy Policy 39(2):999–1006
Shafik N (1994) Economic development and environmental quality: an econometric analysis. Oxford economic papers 46(4):757–774
Shafik N, Bandyopadhyay S (1992) Economic growth and environmental quality: time-series and cross-country evidence, vol 904. World Bank Publications, Washington, DC.
Shahbaz M, Lean HH (2012) Does financial development increase energy consumption? The role of industrialization and urbanization in Tunisia. Energy Policy 40:473–479
Shahbaz M, Hye QMA, Tiwari AK, Leitão NC (2013) Economic growth, energy consumption, financial development, international trade and CO2 emissions in Indonesia. Renew Sust Energ Rev 25:109–121
Stern DI (2004) The rise and fall of the environmental Kuznets curve. World Dev 32(8):1419–1439
Tamazian A, Rao BB (2010) Do economic, financial and institutional developments matter for environmental degradation? Evidence from transitional economies. Energy Econ 32(1):137–145
Tamazian A, Chousa JP, Vadlamannati KC (2009) Does higher economic and financial development lead to environmental degradation: evidence from BRIC countries. Energy Policy 37(1):246–253
Vollebergh HRJ, Dijkgraaf E, Melenberg E (2005) Environmental Kuznets curves for CO2: Heterogeneity versus homogeneity. Center Discussion Paper 2005-25, University of Tilburg
Yuxiang K, Chen Z (2011) Financial development and environmental performance: evidence from China. Environ Dev Econ 16(1):93–111
Zainuddin A (2017) Malaysia to slash another 25% of CO2 emission by 2030. The Malaysia Reserve Retrieved from https://themalaysianreserve.com/2017/06/21/malaysia-slash-another-25-co2-emission-2030/
Zhang Y-J (2011) The impact of financial development on carbon emissions: an empirical analysis in China. Energy Policy 39(4):2197–2203
Author information
Authors and Affiliations
Corresponding author
Additional information
Responsible editor: Philippe Garrigues
Publisher’s note
Springer Nature remains neutral with regard to jurisdictional claims in published maps and institutional affiliations.
Rights and permissions
About this article
Cite this article
Gill, A.R., Hassan, S. & Haseeb, M. Moderating role of financial development in environmental Kuznets: a case study of Malaysia. Environ Sci Pollut Res 26, 34468–34478 (2019). https://doi.org/10.1007/s11356-019-06565-1
Received:
Accepted:
Published:
Issue Date:
DOI: https://doi.org/10.1007/s11356-019-06565-1