Abstract
Background
Non-small cell lung cancer (NSCLC) is the leading cause of cancer-related death worldwide. Lung adenocarcinoma (LUAD) and squamous cell carcinoma (LUSC) are the most common subtypes of NSCLC. Despite genetic differences between LUAD and LUSC have been clarified in depth, the metabolic differences of these two subtypes are still unclear.
Methods
Totally, 128 plasma samples of NSCLC patients were collected before initial treatments, followed by determination of LC-ESI-Q TRAP-MS/MS. Differentially expressed metabolites were screened based on a strict standard.
Results
Based on the integrated platform of targeted metabolome and lipidome, a total of 1141 endogenous metabolites (including 809 lipids) were finally detected in the plasma of NSCLC patients, including 16 increased and 3 decreased endogenous compounds in LUAD group when compared with LUSC group. Thereafter, a logistic regression model integrating four differential metabolites [2-(Methylthio) ethanol, Cortisol, d-Glyceric Acid, and N-Acetylhistamine] was established and could accurately differentiate LUAD and LUSC with an area under the ROC curve of 0.946 (95% CI 0.886–1.000). The cut-off value showed a satisfactory efficacy with 92.0% sensitivity and 92.9% specificity. KEGG functional enrichment analysis showed these differentially expressed metabolites could be further enriched in riboflavin metabolism, steroid hormone biosynthesis, prostate cancer, etc. The endogenous metabolites identified in this study have the potential to be used as novel biomarkers to distinguish LUAD from LUSC.
Conclusions
Our research might provide more evidence for exploring the pathogenesis and differentiation of NSCLC. This research could promote a deeper understanding and precise treatment of lung cancer.
Similar content being viewed by others
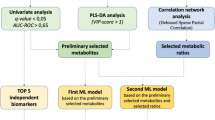
Avoid common mistakes on your manuscript.
1 Introduction
Lung cancer is a malignant tumor with the highest morbidity and mortality in the world, with five-year survival rate less than 20% (Bray et al., 2020; Herbst et al., 2018). Non-small cell lung cancer (NSCLC) accounts for approximately 85% of lung cancers. Among NSCLC, lung adenocarcinoma (LUAD) and squamous cell carcinoma (LUSC) are the most common subtypes (Ettinger et al., 2017). There are many differences in their molecular structure, genetic characteristics and treatment methods, so it is very important to accurately distinguish between these two subtypes.
LUAD is an alveolar epithelial cell carcinoma from adenoid differentiation or mucus-producing cancer cells while LUSC is an epithelial basal cell carcinoma that shows keratinization and intercellular bridges (Travis, 2020). Clinical treatment strategy is quite different between LUAD and LUSC (Relli et al., 2019). Chemotherapy drugs (such as pemetrexed) are very effective in treating LUAD, but they are not recommended for LUSC patients. Besides, antiangiogenic drugs are generally not available in treating LUSC due to frequent bleeding of this population. Driver gene targeted therapy is mainly adapted to LUAD whereas immunotherapy is more effective in treating LUSC (Minguet et al., 2016). Therefore, in order to obtain a satisfactory clinical outcome, a comprehensive examination and pathological classification is necessary before the treatment.
Tissue biopsy is the gold standard for clinical pathological diagnosis and molecular typing of NSCLC. Initially, hematoxylin-eosin (HE) staining observation of tumor tissues under an optical microscope was used to distinguish between LUAD and LUSC. However, it is difficult to make an accurate diagnosis when the tumor structure is unclear due to low differentiation, necrosis or serious extrusion. Nowadays, immunohistochemical method has been widely recommended in clinical practice to distinguish LUAD from LUSC by employing multiple sensitive markers [for example, napsin-A (NAPSA), thyroid transcription factor-1 (TTF-1) and tumor protein p63 (TP63)] (Ao et al., 2014; Schwartz & Rezaei, 2013; Zhan et al., 2015). Although genetic differences between LUAD and LUSC have been clarified in depth, the metabolic differences of these two subtypes are still unclear (Wang et al., 2020).
Metabolomics is an emerging discipline that characterizes a comprehensive profile of endogenous metabolites in biological systems. In particular, metabolomics has been widely used in diagnosing diseases, understanding disease mechanisms, identifying novel drug targets and customizing drug treatments (Wishart, 2016). Metabolome represents the downstream output of the genome and proteome. In contrary to genome and proteome which indicate what may occur, metabolome reveals what is currently happening. In the field of precision medicine, metabolome can promote accurate diagnosis and personalized treatment by characterizing metabolic phenotypes of a cell, organ or biological system. In the present study, we aimed to distinguish the metabolic phenotypes between LUAD and LUSC based on a comprehensive targeted metabolomic platform. The investigation and classification of metabolic phenotype of these two NSCLC subtypes will facilitate a deeper understanding and precise therapy of lung cancer.
2 Methods
2.1 Study participants
A total of 162 patients were initially enrolled from the cancer center of Wuhan Union Hospital, all of which were confirmed NSCLC patients. The heparin anticoagulated plasma samples were collected before treatments, centrifugated at 3500 rpm for 10 min at 4 °C, followed by pipetting supernatant plasma and immediately transferring to a − 80 °C freezer until metabolic analysis. The present study analyzed 128 plasma samples (28 LUSC and 100 LUAD patients) of them. The other patients were not included in this study because 21 of them were diagnosed as other subtypes such as poorly differentiated carcinoma and sarcomatoid carcinoma and the pathological diagnosis of rest patients remained unknown. The pathological subclassification of NSCLC patients were according to the acknowledged WHO guidelines (Osmani et al., 2018).
2.2 Targeted metabolomic analysis
The plasma samples were thawed on ice and mixed by a 3 volume of ice-cold methanol, the mixture was then whirled for 3 min and centrifuged with 12,000 rpm at 4 °C for 10 min. Then the supernatant was collected and centrifuged at 12,000 rpm at 4 °C for 5 min. Finally, the supernatant was collected again for LC-MS/MS analysis.
The sample extracts were analyzed using a liquid chromatography-electrospray ionization tandem mass spectrometry (LC-ESI-MS/MS) system (Shim-pack UFLC SHIMADZU CBM A system, https://www.shimadzu.com/; MS, QTRAP® System, https://sciex.com/). The analytical conditions were as follows: UPLC: column, Waters ACQUITY UPLC HSS T3 C18 (1.8 μm, 2.1 mm × 100 mm); column temperature, 40 °C; flow rate, 0.4 mL/min; injection volume, 5 µL; solvent system, water (0.1% formic acid): acetonitrile (0.1% formic acid); gradient program, 95:5 v/v at 0 min, 10:90 v/v at 11.0 min, 10:90 v/v at 12.0 min, 95:5 v/v at 12.1 min, 95:5 v/v at 14.0 min.
LIT and triple quadrupole (QQQ) scans were acquired on a triple quadrupole-linear ion trap mass spectrometer (QTRAP), QTRAP® LC-MS/MS System, equipped with an ESI Turbo Ion-Spray interface, operating in positive and negative ion mode and controlled by Analyst 1.6.3 software (Sciex). The ESI source operation parameters were as follows: source temperature 500 °C; ion spray voltage (IS) 5500 V (positive), − 4500 V (negative); ion source gas I (GSI), gas II (GSII) and curtain gas (CUR) were set at 55, 60, and 25.0 psi, respectively; the collision gas (CAD) was high. Instrument tuning and mass calibration were performed with 10 and 100 µmol/L polypropylene glycol solutions in QQQ and LIT modes, respectively. A specific set of MRM transitions were monitored for each period according to the metabolites eluted within this period.
2.3 Targeted lipidomic analysis
The plasma samples were melted on ice, vortexed for 10 s and then centrifuged with 3000 rpm at 4 °C for 5 min. 50 µL of each sample was taken and homogenized with 1 mL mixture (include methanol, MTBE and internal standard). The mixture was whirled for 2 min, followed by addition of 500 µL water, and whirled again for 1 min. After centrifugation with 12,000 rpm at 4 °C for 10 min, 500 µL supernatant of each sample was taken and concentrated. Next, dissolve the extract with 100 µL mobile phase B, then stored in − 80 °C. Finally, take the dissolving solution into the sample bottle for LC-MS/MS analysis.
The sample extracts were analyzed using an LC-ESI-MS/MS system (Shim-pack UFLC SHIMADZU CBM A system, https://www.shimadzu.com/; MS, QTRAP® System, https://sciex.com/). The analytical conditions were as follows, UPLC: column, Waters ACQUITY UPLC HSS T3 C18 (1.8 μm, 2.1 mm × 100 mm); column temperature, 40 °C; flow rate, 0.4 mL/min; injection volume, 5 µL; solvent system, water (0.04% acetic acid): acetonitrile (0.04% acetic acid); gradient program, 95:5 v/v at 0 min, 5:95 v/v at 11.0 min, 5:95 v/v at 12.0 min, 95:5 v/v at 12.1 min, 95:5 v/v at 14.0 min. The subsequent MS detection is consistent with the above-mentioned targeted metabolomics analysis.
2.4 Statistical analysis
The following results were obtained with R software. At first, the data of endogenous metabolites in terms of homogeneity and reproducibility was visualized by principal component analysis (PCA). Then, the orthogonal partial least squares discriminant analysis (OPLS-DA) was further applied to remove irrelevant variables. The variable importance in the projection (VIP) values of each metabolite were obtained to measure the contribution of the variable to the model. The validity of OPLS-DA model was judged by R2Y (the interpretability of the model for the categorical variable Y) and Q2 (predictability of the model).
The following data were analyzed using SPSS 23.0 software (SPSS, Chicago, IL, USA). The levels of differential metabolites were expressed using violin plots (Fig. 4). A non-parametrical Kolmogorov-Smirnov test was used for confirming a normal distribution of the data. Differences between the two groups were examined using two-tailed independent samples T-test. P values were presented when variances were equal or inequal, in which a Levene’s test was used to assess variance homogeneity. The logistic regression analysis was performed to evaluate the diagnostic value of the combined biomarkers model. Model performance was assessed by the receiver operating characteristic curve (ROC).
The analysis of metabolic pathways was conducted by the Kyoto Encyclopedia of Genes and Genomes (KEGG, https://www.kegg.jp/) (Kanehisa & Goto, 2000). The enrichment of differential expression metabolites was visualized as bubble chart. The function of metabolites was assigned according to the Human Metabolome Database (HMDB, https://hmdb.ca) (Wishart et al., 2009).
3 Results
3.1 Baseline of the study population
The clinical characteristics of the LUAD and LUSC patients were displayed in Supplementary Table 1. It was shown that LUSC patients were significantly older than LUAD patients (p < 0.001). Male accounted for a greater proportion in LUSC patients (86%) when compared with LUAD patients (64%). It was noteworthy that a large number of non-smokers (54%) were diagnosed with LUAD while only 25% of LUSC patients has no smoking history. The two types of lung cancer patients have no significant difference on BMI and disease stage.
3.2 Detection of endogenous metabolites in plasma of NSCLC patients
The present study was conducted based on an integrated platform of targeted metabolome and lipidome, which contained more than 3000 characterized compounds (Supplementary Table 2). A total of 128 plasma samples were tested in the present study, which were extracted by hydrophilic and hydrophobic methods, respectively, and each sample was then detected using UPLC-MS/MS in positive and negative modes (Fig. 1). Finally, 1141 compounds were detected, which were qualitatively and quantitatively categorized into 32 types (Table 1).
In this study, quality control (QC) samples (a mixture of sample extracts) were inserted into the queue to monitor the repeatability of the analytical method in every ten samples. The method stability was assessed by overlaying the total ion current diagrams (TIC diagrams) of different QC samples. The results showed that the curves of the TIC diagrams were highly overlapped, and the retention time and peak intensity were consistent, demonstrating that the signal was stable throughout the analysis process (Supplementary Fig. 1 A–D). In addition, PCA map was used to estimate the degree of variability and the overall metabolic difference. Our results showed that the QC samples were not separated from each other, indicating the stability of this analytic method (Supplementary Fig. 1E, F).
3.3 Identification of differential metabolities in LUAS and LUSC groups
The raw metabolomic and lipidomic data was displayed using an OPLS-DA score map, which showed clear distinction between LUAD and LUSC group (Fig. 2A). Subsequent permutation test of the generated model showed that R2X = 0.365, R2Y = 0.929, Q2 = 0.547 (Fig. 2B), confirming that this model was reliable with respect to prediction performance. Figure 2C showed the S-plot of OPLS-DA, in which the metabolites near the upper right and lower left corners indicated differential expression (red dots, VIP ≥ 1; green dots, VIP < 1)
Among the 1141 detected endogenous metabolites, we screened differential components based on both Fold Change (FC) variations and VIP values (FC>2/ < 0.5 and VIP > 1.0, Fig. 3A, B). The result of screening for differential metabolites was visualized in a volcano map (Fig. 3C). A total of 19 differential metabolites were identified, including 3 down-regulated and 16 up-regulated differential metabolites (Table 1). Moreover, the Pearson correlation analysis method is applied to perform correlation analysis on these 19 metabolites (Fig. 3D). Several pairs of them showed strong correlation including 2-Hydroxybutanoic Acid/d-Glyceric Acid, cis-1-Pentadecenoic Acid (C15: 1)/2-(Methylthio) ethanol, Inosine/Hypoxanthine-9-β-d-Arabinofuranoside, Inosine/6-Methylnicotinamide, 6-Methylnicotinamide/ Hypoxanthine-9-β-d-Arabinofuranoside, 5-Aminosalicylic Acid/N-Acetylhistamine, 5-Aminosalicylic Acid/1,4-Dihydro-1-Methyl-4-Oxo-3-Pyridinecarboxamide and Riboflavin/Lumichrome.
3.4 Identification of diagnostic efficacy of differential metabolites
As mentioned above, 19 differential metabolites were screened out between LUAD and LUSC groups (Fig. 4). We explored whether the demographic characteristics of patients had influences on the plasma metabolic profiles of them by plotting four heatmaps (Supplementary Fig. 2). It showed that the levels of 19 differential expressed metabolites were not affected by these factors remarkably, except that inosine, cis-1-pentadecenoic acid (C15: 1) and hypoxanthine-9-β-d-arabinofuranoside seemed to fluctuate in a certain extent between different groups.
In order to evaluate the diagnostic efficacy of these metabolites, ROC curves were plotted for each metabolite or different groups of metabolites combinations which were based on logistic regression models. The Akaike Information Criterion (AIC) was used to screen the optimal combination, with the lower the AIC value representing the better the model effect. Among all the diagnostic models, a logistic regression model including four differential metabolites [2-(Methylthio)ethanol, Cortisol, d-Glyceric Acid, and N-Acetylhistamine] was selected due to its lowest AIC value (data not shown). This model was demonstrated to be an ideal diagnostic tool with an area under the ROC curve of 0.946 (95% CI 0.886–1.000). The cut-off value was calculated based on Youden’s J statistic abovementioned and showed a satisfactory efficacy with 92.0% sensitivity and 92.9% specificity (Fig. 5).
3.5 KEGG functional enrichment analysis
In addition, we performed an enriched pathway analysis for the screened metabolites by using KEGG database. As shown in Supplementary Fig. 3, related pathways could be classified into metabolic pathways, vitamin digestion and absorption, riboflavin metabolism, glycerolipid metabolism, ABC transporters, etc. (Supplementary Fig. 3A). According to the rich factors of KEGG pathway classification, these differentially expressed metabolites could be further enriched in riboflavin metabolism, steroid hormone biosynthesis, prostate cancer, etc. (Supplementary Fig. 3B).
It is noteworthy that pathways with highest enrichment factors (steroid hormone biosynthesis and prostate cancer) were all due to the differential expression of cortisol. Therefore, we further studied cortisol related genes based on The Cancer Genome Atlas (TCGA) database via the website of Gene Expression Profiling Interactive Analysis (GEPIA, http://gepia.cancer-pku.cn) (Tang et al., 2017). The results showed that steroid hormone acute regulatory protein (StAR), a rate-limiting protein of cortisol production, was an important factor in LUAD and LUSC. Specifically, as shown in Supplementary Fig. 4A, StAR expression was downregulated in LUAD tumor tissue when compared with adjacent normal tissue. On the contrary, the expression of StAR in LUSC tumor tissue was upregulated compared with the paired adjacent normal tissue. Moreover, StAR significantly differentiated the overall survival of both LUAD and LUSC (Supplementary Fig. 4C, E), where high expression of StAR predicted a better prognosis.
4 Discussion
Lung cancer is one of the most prevalent malignant tumors worldwide, with NSCLC accounting for an estimated 80–85% of those (Torre et al., 2016). Despite the remarkable progress acquired in the treatment of lung cancer in recent decades, the overall survival rate of this disease is still not satisfactory (2019). Accurate diagnosis of histopathological subtypes of NSCLC is of great significance for the precise lung cancer treatment. Currently, the gold standard for the diagnosis of adenocarcinoma and squamous carcinoma of NSCLC is liver biopsy. The difference between LUAD and LUSC requires visual inspection by an experienced pathologist. However, it is difficult to make an accurate diagnosis when the tumor structure is unclear. Therefore, complementary diagnostic biomarkers are still needed to assist NSCLC pathological typing and subsequent precise treatment decisions.
Previous study had demonstrated that LUAD and LUSC were vastly distinct diseases at the molecular, pathological and clinical levels (Relli et al., 2019). The differences between them have been discussed based on analyzing DNA methylation, RNA, miRNA expression and protein (Sun et al., 2017; Zhang et al., 2020). Fan et al. discussed the mRNA-related biomarkers as potential treatment strategies for LUAD and LUSC by analyzing TCGA database (Liao et al., 2020; Liao et al., 2020). Dong et al. demonstrated that DSG3 and KRT14 were differentially expressed between LUAD and LUSC patients by analyzing Gene Expression Omnibus (GEO) microarray data and 40 pairs of tumor tissue samples (Dong et al., 2020). Zhang et al. had discovered that plasma circulating tumor DNA (ctDNA) was correlated to histology types in NSCLC patients, and then established a ctDNA-based histologic classification model with an accuracy of 90% (Zhang et al., 2019). Xiang et al. found that the plasma levels of homovanillic acid and serotonin hydrochloride were significantly different between patients with LUAD and LUSC, but further relationship remained unknown (Xiang et al., 2018). Despite the extensive studies aimed to discover differences between two kinds of pathological subtypes on genetic and transcriptional aspects, there are still limited achievements in differentiating them on metabolic level. Therefore, it is necessary to conduct a comprehensive study of the metabolic characteristics of these two main subtypes of lung cancer, which will facilitate a deeper understanding and precise therapy of NSCLC.
Metabolomics and lipidomics (a subsection of metabolomics) are emerging disciplines that characterize the comprehensive profile of endogenous metabolites in biological systems. As a high-throughput platform, metabolomics can capture metabolic variations that reflect the disease process. In a recent published work of Kowalczyk et al., the differences in metabolic characteristics between patients with both early and late stages of LUAD and LUSC patients were explored. Their results showed that the levels of PC 15:0/22:6 and PC 18:1/22:6 were significantly different between LUAD and LUSC patients of early stage (Kowalczyk et al., 2021). In contrast, more differential metabolites including deoxycholic acid, glycocholic aicd, linoleic acid, arachidonic acid, and kinds of phospholipids were discovered in the comparison of plasma of LUAD, LUSC and large cell cancer (Kowalczyk et al., 2021).
Interestingly, the results of lipidome in the current study show that the difference in lipid profiles between LUAD and LUSC is very limited, as demonstrated by merely four differentially expressed metabolites out of 809 detected lipid compounds. In contrast, as many as 15 differentially expressed metabolites, which belong to organic acid, hormones, nucleotides and others, were found even though only 332 kinds of endogenous metabolites were discovered in the plasma of NSCLC patients. These results seem to be inconsistent with the findings of Kowalczyk et al. In their work, most of the differentially expressed metabolites belong to the lipids. The potential reason remains obscure, and the conflicting metabolic profiles are usually seen in discovering the differential metabolites of NSCLC subtypes. To the best of our knowledge, several studies have attempted to identify differential metabolites in plasma of NSCLC patients, and discovered specific plasma metabolomics profiles including organic acids, amino acids, fatty acids, lipids or carbohydrates, but the results of them are not always coherent among these different studies (Chen et al., 2018; Kumar et al., 2017; Ni et al., 2019; Puchades-Carrasco et al., 2016; Xiang et al., 2018). We speculate that the population differences and different detection methods may lead to these inconsistent results. Nevertheless, in these studies, organic acids are usually screened out as the differential expressed metabolites. For example, linoleic acid is overexpressed in the plasma of LUAD patients when compared with LUSC counterparts both in our study and the work of Kowalczyk et al.
In the present study, 1141 metabolites were detected in the plasma of NSCLC patients. An OPLS-DA model based on all the metabolites levels revealed that LUAD patients is significantly different from that of LUSC, reflecting distinct metabolic profiles caused by two kinds of pathological conditions. Further, 19 of the 1141 metabolites was selected as differentially expressed metabolites based on strict screening standards (both FC > 2/ < 0.5 and VIP > 1.0). As mentioned in the method section, all the enrolled patients were newly diagnosed NSCLC patients and has not received any treatment yet. Therefore, differences of these metabolites in two groups may not be related to drug treatment. In order to classify NSCLC pathological subtype more accurately, we tested different combinations of the 19 differentially expressed metabolites and identified the optimal combination containing four metabolites based on the AIC index. Finally, 2-(Methylthio)ethanol, cortisol, d-Glyceric Acid and N-Acetylhistamine were integrated to establish a potent diagnostic model. This integrated diagnostic model showed an efficient diagnostic ability to distinguish between LUSC and LUAD, with an area under the ROC curve of 0.946 (95% CI 0.886–1.000), as well as high sensitivity and specificity.
Taken together, in our study, the concentrations of plasma endogenous metabolites (such as alcohol/amines, heterocyclic compounds, nucleotides, organic acid) differ much more significantly between LUAD and LUSC group when compared with lipids levels. According to the annotations of KEGG and HMDB databases, it is noteworthy that among the 19 differentially expressed metabolites, 4-Pyridoxic Acid, d-Glyceric Acid, Pantothenate, 2-Hydroxybutanoic Acid, Undecanedioic Acid, Inosine and Riboflavin (all of which are not belong to lipid compound) have been reported to related to colorectal cancer (Brown et al., 2016; Goedert et al., 2014; Ni et al., 2014; Sinha et al., 2016). However, their relevance to lung cancer remain unclear. Therefore, the physiological effects of these endogenous metabolites should attract the attention of NSCLC researchers.
Notably, KEGG functional enrichment analysis of the 19 differential metabolites showed that cortisol was highly correlated with the pathways of maximum enrichment factor. Therefore, we further studied cortisol related genes using TCGA data and found that StAR expression might be an important factor in LUAD and LUSC. Abnormal expression of transporter proteins in cell membrane has already been demonstrated to be associated tumor progression and development in lung cancer (Wang et al., 2017). Herein, StAR, as a membrane transporter protein, mediates the transport of cholesterol from outer to inner mitochondrial membrane, which is the initial and rate-limiting step of cortisol production (Manna & Stocco, 2005). The production of cortisol depends on StAR, and previous study demonstrated the increase of cortisol production in human adrenocortical cells was associated with elevated expression of StAR in human adrenocortical cells (Ping et al., 2012). Consistent with metabolomics data (the level of cortisol in the plasma of LUSC patients was significantly increased compared with LUAD patients), the expression of StAR in LUSC tumor tissues was also upregulated compared with LUAD ones. StAR was significantly correlated with the prognosis of LUSC and LUAD patients (Supplementary Fig. 4). However, whether it is upregulated in the adrenocortical cells of LUSC patients remains to be further studied.
In addition, D-glyceric acid, an organic acid which was selected as a one of the discriminators of LUAD and LUSC in our study, is derived from the biosynthesis of glycerol (Habe et al., 2009). Aquaporin 3 (AQP3) is also a transporter of membrane, which delivers glycerol for synthesizing the lipid second messenger in the regulation of keratinocyte proliferation and differentiation (Wang et al., 2017). The analysis of TCGA data showed that AQP3 is over-expressed in the tumor tissues of LUAD patients compared with LUSC ones (Supplementary Fig. 5). Interestingly, our findings reveal that the level of d-glyceric acid is significantly higher in the plasma of LUAD patients than that of LUSC counterparts, suggesting that the increased content of d-glyceric acid might be related with the over-expression of AQP3. However, the overall survival and disease-free survival of LUAD and LUSC subtype patients seemed not to be correlated with the expression of AQP3. The specific role of d-glyceric acid in the proliferation and differentiation of NSCLC need to be further explored.
Despite the interesting results, we have to admit that there exist some limitations in the present study. First, the sample size is too small and distributed unbalanced between LUAD and LUSC groups. Second, a validation set for the ROC curve is lack in our research. Third, this study did not include a group of healthy human plasma samples for comparisons with LUAD/LUSC patients. Last but not the least, tissue metabolomic analysis is lack here, which is crucial to identify potential biomarkers derived from the secretion of tumor tissues.
5 Conclusions
In summary, the present study has plotted comprehensive metabolic profiles of LUAD and LUSC landscapes by employing a platform of targeted metabolome and lipidome. This investigation identifies novel endogenous metabolites that may be served as key small molecules in the development and differentiation of NSCLC. However, more in-depth mechanism researches and larger cohort studies are needed to verify these small molecular differences and metabolic pathway abnormalities for clinical application to NSCLC precise diagnosis, treatment and prevention.
Data availability
All data generated or analyzed during this study are included in this published article and its supplementary information files.
References
Ao, M. H., Zhang, H., Sakowski, L., Sharma, R., Illei, P. B., Gabrielson, E., Askin, F., & Kay Li, Q. (2014). The utility of a novel triple marker (combination of TTF1, napsin A, and p40) in the subclassification of non-small cell lung cancer. Human Pathology, 45, 926–934. https://doi.org/10.1016/j.humpath.2014.01.005
Bray, F., Ferlay, J., Soerjomataram, I., Siegel, R. L., Torre, L. A., & Jemal, A. (2020). Global cancer statistics 2018: GLOBOCAN estimates of incidence and mortality worldwide for 36 cancers in 185 countries (vol 68, pg 394, 2018). Ca-a Cancer Journal for Clinicians, 70, 313–313. https://doi.org/10.3322/caac.21609
Brown, D. G., Rao, S., Weir, T. L., O’Malia, J., Bazan, M., Brown, R. J., & Ryan, E. P. (2016). Metabolomics and metabolic pathway networks from human colorectal cancers, adjacent mucosa, and stool. Cancer Metab, 4, 11. https://doi.org/10.1186/s40170-016-0151-y
Chen, Y., Ma, Z., Zhong, J., Li, L., Min, L., Xu, L., Li, H., Zhang, J., Wu, W., & Dai, L. (2018). Simultaneous quantification of serum monounsaturated and polyunsaturated phosphatidylcholines as potential biomarkers for diagnosing non-small cell lung cancer. Science and Reports, 8, 7137. https://doi.org/10.1038/s41598-018-25552-z
Dong, Y., Li, S., Sun, X., Wang, Y., Lu, T., Wo, Y., Leng, X., Kong, D., & Jiao, W. (2020). Desmoglein 3 and keratin 14 for distinguishing between lung adenocarcinoma and lung squamous cell carcinoma. Oncotargets and Therapy, 13, 11111–11124. https://doi.org/10.2147/OTT.S270398
Ettinger, D. S., Wood, D. E., Aisner, D. L., Akerley, W., Bauman, J., Chirieac, L. R., D’Amico, T. A., DeCamp, M. M., Dilling, T. J., Dobelbower, M., Doebele, R. C., Govindan, R., Gubens, M. A., Hennon, M., Horn, L., Komaki, R., Lackner, R. P., Lanuti, M., Leal, T. A., … Hughes, M. (2017). Non-small cell lung cancer, version 5.2017, NCCN clinical practice guidelines in oncology. Journal of the National Comprehensive Cancer Network, 15, 504–535. https://doi.org/10.6004/jnccn.2017.0050
Goedert, J. J., Sampson, J. N., Moore, S. C., Xiao, Q., Xiong, X., Hayes, R. B., Ahn, J., Shi, J., & Sinha, R. (2014). Fecal metabolomics: Assay performance and association with colorectal cancer. Carcinogenesis, 35, 2089–2096. https://doi.org/10.1093/carcin/bgu131
Habe, H., Fukuoka, T., Kitamoto, D., & Sakaki, K. (2009). Biotransformation of glycerol to d-glyceric acid by acetobacter tropicalis. Applied Microbiology and Biotechnology, 81, 1033–1039. https://doi.org/10.1007/s00253-008-1737-2
Herbst, R. S., Morgensztern, D., & Boshoff, C. (2018). The biology and management of non-small cell lung cancer. Nature, 553, 446–454. https://doi.org/10.1038/nature25183
Kanehisa, M., & Goto, S. (2000). KEGG: Kyoto encyclopedia of genes and genomes. Nucleic Acids Research, 28, 27–30. https://doi.org/10.1093/nar/28.1.27
Kowalczyk, T., Kisluk, J., Pietrowska, K., Godzien, J., Kozlowski, M., Reszeć, J., Sierko, E., Naumnik, W., Mróz, R., Moniuszko, M., Kretowski, A., Niklinski, J., & Ciborowski, M. (2021). The ability of metabolomics to discriminate non-small-cell lung cancer subtypes depends on the stage of the disease and the type of material studied. Cancers (basel). https://doi.org/10.3390/cancers13133314
Kumar, N., Shahjaman, M., Mollah, M. N. H., Islam, S. M. S., & Hoque, M. A. (2017). Serum and plasma metabolomic biomarkers for lung cancer. Bioinformation, 13, 202–208. https://doi.org/10.6026/97320630013202
Liao, Y., Wang, Y., Cheng, M., Huang, C., & Fan, X. (2020a). Weighted gene coexpression network analysis of features that control cancer stem cells reveals prognostic biomarkers in lung adenocarcinoma. Frontiers in Genetics, 11, 311. https://doi.org/10.3389/fgene.2020.00311
Liao, Y., Xiao, H., Cheng, M., & Fan, X. (2020b). Bioinformatics analysis reveals biomarkers with cancer stem cell characteristics in lung squamous cell carcinoma. Frontiers in Genetics, 11, 427. https://doi.org/10.3389/fgene.2020.00427
Manna, P. R., & Stocco, D. M. (2005). Regulation of the steroidogenic acute regulatory protein expression: Functional and physiological consequences. Current Drug Targets: Immune, Endocrine and Metabolic Disorders, 5, 93–108. https://doi.org/10.2174/1568008053174714
Minguet, J., Smith, K. H., & Bramlage, P. (2016). Targeted therapies for treatment of non-small cell lung cancer–Recent advances and future perspectives. International Journal of Cancer, 138, 2549–2561. https://doi.org/10.1002/ijc.29915
Ni, J., Xu, L., Li, W., Zheng, C., & Wu, L. (2019). Targeted metabolomics for serum amino acids and acylcarnitines in patients with lung cancer. Experimental and Therapeutic Medicine, 18, 188–198. https://doi.org/10.3892/etm.2019.7533
Ni, Y., Xie, G., & Jia, W. (2014). Metabonomics of human colorectal cancer: New approaches for early diagnosis and biomarker discovery. Journal of Proteome Research, 13, 3857–3870. https://doi.org/10.1021/pr500443c
Osmani, L., Askin, F., Gabrielson, E., & Li, Q. K. (2018). Current WHO guidelines and the critical role of immunohistochemical markers in the subclassification of non-small cell lung carcinoma (NSCLC): Moving from targeted therapy to immunotherapy. Seminars in Cancer Biology, 52, 103–109. https://doi.org/10.1016/j.semcancer.2017.11.019
Ping, J., Lei, Y. Y., Liu, L., Wang, T. T., Feng, Y. H., & Wang, H. (2012). Inheritable stimulatory effects of caffeine on steroidogenic acute regulatory protein expression and cortisol production in human adrenocortical cells. Chemico-Biological Interactions, 195, 68–75. https://doi.org/10.1016/j.cbi.2011.11.001
Puchades-Carrasco, L., Jantus-Lewintre, E., Pérez-Rambla, C., García-García, F., Lucas, R., Calabuig, S., Blasco, A., Dopazo, J., Camps, C., & Pineda-Lucena, A. (2016). Serum metabolomic profiling facilitates the non-invasive identification of metabolic biomarkers associated with the onset and progression of non-small cell lung cancer. Oncotarget, 7, 12904–12916. https://doi.org/10.18632/oncotarget.7354
Relli, V., Trerotola, M., Guerra, E., & Alberti, S. (2019). Abandoning the notion of non-small cell lung cancer. Trends in Molecular Medicine, 25, 585–594. https://doi.org/10.1016/j.molmed.2019.04.012
Schwartz, A. M., & Rezaei, M. K. (2013). Diagnostic surgical pathology in lung cancer: Diagnosis and management of lung cancer, 3rd ed: American college of chest physicians evidence-based clinical practice guidelines. Chest, 143, e251S-e262S. https://doi.org/10.1378/chest.12-2356
Sinha, R., Ahn, J., Sampson, J. N., Shi, J., Yu, G., Xiong, X., Hayes, R. B., & Goedert, J. J. (2016). Fecal microbiota, fecal metabolome, and colorectal cancer interrelations. PLoS ONE, 11, e0152126. https://doi.org/10.1371/journal.pone.0152126
Sun, F. H., Yang, X., Jin, Y., Chen, L., Wang, L., Shi, M., Zhan, C., Shi, Y., & Wang, Q. (2017). Bioinformatics analyses of the differences between lung adenocarcinoma and squamous cell carcinoma using the cancer genome atlas expression data. Molecular Medicine Reports, 16, 609–616. https://doi.org/10.3892/mmr.2017.6629
Tang, Z., Li, C., Kang, B., Gao, G., Li, C., & Zhang, Z. (2017). GEPIA: A web server for cancer and normal gene expression profiling and interactive analyses. Nucleic Acids Research, 45, W98–W102. https://doi.org/10.1093/nar/gkx247
Torre, L. A., Siegel, R. L., Ward, E. M., & Jemal, A. (2016). Global cancer incidence and mortality rates and trends—An update. Cancer Epidemiology, Biomarkers & Prevention, 25, 16–27. https://doi.org/10.1158/1055-9965.EPI-15-0578
Travis, W. D. (2020). Lung cancer pathology: Current concepts. Clinics in Chest Medicine, 41, 67–85. https://doi.org/10.1016/j.ccm.2019.11.001
Wang, B. Y., Huang, J. Y., Chen, H. C., Lin, C. H., Lin, S. H., Hung, W. H., & Cheng, Y. F. (2020). The comparison between adenocarcinoma and squamous cell carcinoma in lung cancer patients. Journal of Cancer Research and Clinical Oncology, 146, 43–52. https://doi.org/10.1007/s00432-019-03079-8
Wang, X., Tao, C., Yuan, C., Ren, J., Yang, M., & Ying, H. (2017). AQP3 small interfering RNA and PLD2 small interfering RNA inhibit the proliferation and promote the apoptosis of squamous cell carcinoma. Molecular Medicine Reports, 16, 1964–1972. https://doi.org/10.3892/mmr.2017.6847
Wishart, D. S. (2016). Emerging applications of metabolomics in drug discovery and precision medicine. Nature Reviews. Drug Discovery, 15, 473–484. https://doi.org/10.1038/nrd.2016.32
Wishart, D. S., Knox, C., Guo, A. C., Eisner, R., Young, N., Gautam, B., Hau, D. D., Psychogios, N., Dong, E., Bouatra, S., Mandal, R., Sinelnikov, I., Xia, J., Jia, L., Cruz, J. A., Lim, E., Sobsey, C. A., Shrivastava, S., Huang, P., … Forsythe, I. (2009). HMDB: A knowledgebase for the human metabolome. Nucleic Acids Research, 37, D603–D610. https://doi.org/10.1093/nar/gkn810
Xiang, C., Jin, S., Zhang, J., Chen, M., Xia, Y., Shu, Y., & Guo, R. (2018). Cortisol, cortisone, and 4-methoxyphenylacetic acid as potential plasma biomarkers for early detection of non-small cell lung cancer. International Journal of Biological Markers, 33, 314–320. https://doi.org/10.1177/1724600818778754
Zhan, C., Yan, L., Wang, L., Sun, Y., Wang, X., Lin, Z., Zhang, Y., Shi, Y., Jiang, W., & Wang, Q. (2015). Identification of immunohistochemical markers for distinguishing lung adenocarcinoma from squamous cell carcinoma. Journal of Thoracic Disease, 7, 1398–1405. https://doi.org/10.3978/j.issn.2072-1439.2015.07.25
Zhang, B., Niu, X., Zhang, Q., Wang, C., Liu, B., Yue, D., Li, C., Giaccone, G., Li, S., Gao, L., Zhang, H., Wang, J., Yang, H., Wu, R., Ni, P., Wang, C., Ye, M., & Liu, W. (2019). Circulating tumor DNA detection is correlated to histologic types in patients with early-stage non-small-cell lung cancer. Lung Cancer, 134, 108–116. https://doi.org/10.1016/j.lungcan.2019.05.034
Zhang, H., Jin, Z., Cheng, L., & Zhang, B. (2020). Integrative analysis of methylation and gene expression in lung adenocarcinoma and squamous cell lung carcinoma. Frontiers in Bioengineering and Biotechnology, 8, 3. https://doi.org/10.3389/fbioe.2020.00003
(2019). Lung cancer: Some progress, but still a lot more to do. The Lancet, 394, 1880–1880. https://doi.org/10.1016/S0140-6736(19)32795-3
Acknowledgements
This study was supported by the National Key R&D Program of China (Grant No. 2017YFC0909900) and the National Natural Science Foundation of China (Grant No. 81903901). We sincerely thank Dr. Hui Zheng from Wuhan Metware Biotechnology Co., Ltd. and his colleagues for their technical support.
Author information
Authors and Affiliations
Contributions
PC: Sample and data collection, writing-original draft preparation, conceptualization; SW: writing-original draft preparation; WG: manuscript revision and data analysis; QZ: sample collection; WG: data analysis; QL: data collection; RZ: data collection; XD: conceptualization; SX: conceptualization; YL: conceptualization; SS: conceptualization; YH: manuscript revision and conceptualization; YZ: supervision.
Corresponding authors
Ethics declarations
Conflict of interest
The authors declare no conflicts of interest.
Ethical approval
This study protocol had been approved by the Ethics Committee of the Union Hospital, Tongji Medical College, Huazhong University of Science and Technology and written informed consent was obtained from all patients.
Additional information
Publisher’s Note
Springer Nature remains neutral with regard to jurisdictional claims in published maps and institutional affiliations.
Supplementary Information
Below is the link to the electronic supplementary material.
Rights and permissions
About this article
Cite this article
Cao, P., Wu, S., Guo, W. et al. Precise pathological classification of non-small cell lung adenocarcinoma and squamous carcinoma based on an integrated platform of targeted metabolome and lipidome. Metabolomics 17, 98 (2021). https://doi.org/10.1007/s11306-021-01849-5
Received:
Accepted:
Published:
DOI: https://doi.org/10.1007/s11306-021-01849-5