Abstract
Technological knowledge can be created via R&D investments, but it can also be eroded through depreciation. Knowing how fast knowledge depreciates is important for various reasons for practitioners and decision makers alike; especially if it comes to questions regarding how to “recharge” knowledge production processes within an ever changing global system. In this study, we use patent citation data to identify technological knowledge depreciation rates by adjusting for exogenous citation inflation and by disentangling the preferential-attachment dynamics of citation growth. Solar photovoltaic (PV) technology is employed as a case study. The rates calculated with our method are comparable to the few available estimates on technology depreciation rates in the PV industry. One of the advantages of the proposed method is that its underlying data are more readily available, and thus more replicable for the study of the knowledge depreciation rates in other relevant technology fields.
Similar content being viewed by others
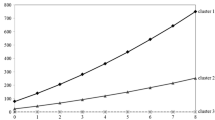
Avoid common mistakes on your manuscript.
Introduction
Clean technologies have been generally regarded as the key solution to sustainable human development (Johnstone et al. 2012; Perruchas et al. 2020). Emerging technologies such as solar photovoltaics, wind turbines, and electric cars are at the “frontier” of economic development (Binz et al. 2017; Huenteler et al. 2016a; Rosenberg 1963). As technological change in such industries often necessitates long-term trial and error processes, understanding the evolutionary patterns of clean technologies’ development trajectories is thus critical to accelerating technological evolution overall, as well as to facilitate the transitions to sustainable systems in the context of climate change.
Technological evolution has long been regarded as the upgrading of designs, that is, evolution in the artifact space (Huenteler et al. 2016a), while few research efforts have documented the vicissitude of technological knowledge aspects that actually underlie artifactual designs. Huenteler et al. (2016a) employ patent citation data in order to demonstrate the trajectory of technological knowledge (TK) generation processes, which in turn sheds light on the evolution of knowledge spaces shaped by the artifact space. However, Huenteler et al. (2016a) focus only on the creation of knowledge, but don’t provide further insights on knowledge depreciation caused by obsolescence and forgetting.
The depreciation of TK, just like the depreciation of tangible capital, eroding technological knowledge stocks (TKS) accumulated through research and development (R&D), is an inevitable phenomenon (Grubler and Nemet 2012). The rate of TK depreciation is a critical economic parameter that contains information about technological evolution and the economic environment, but how to measure it remains an open question (de Rassenfosse and Jaffe 2018). In the few available studies associated with TK depreciation, researchers mainly used R&D expenditure data or patent data to identify the rate, but these methods have various drawbacks such as containing too many assumptions and data collection difficulties.
To solve this problem, based on the recombinant innovation theory, this study uses a new method to identify the TK depreciation rate from patent citation data. Borrowing ideas from recent research on scientific and technological document citation behaviors (Higham et al. 2017a, b; Yin and Wang 2017), the proposed method offers two relevant improvements: rescaling citation data to account for citation inflation and disentangling the preferential-attachment dynamic. As a result, purely time-dependent depreciation patterns can be obtained via this approach.
To execute our method, we focus on the case of solar PV, one of the most important clean technologies. Solar PV systems, which produce energy through converting the electromagnetic spectrum of sunlight into electrical power (Binz et al. 2017), have the following characteristics. First, the market for solar PV systems has grown exponentially in the last two decades. The global total average installed cost of new utility-scale PV systems has decreased by over 50% in the last decade, which is primarily driven by PV module cost reduction (IRENA 2017). The rapidly changing market and hardware costs reflect the shock in solar PV technology development, which needs to be closely monitored for future R&D decisions. Second, the solar PV industry has undergone several strong shifts regarding the geographic locations of its key manufacturing areas and marketplaces. Its knowledge base has also globalized since the very early industry formation phase (Binz et al. 2017). The solar PV industry thus can be used to illustrate different knowledge production processes around the world. The results presented here demonstrate that the rates based on the proposed method are comparable to the few available estimates on technology depreciation rates in the PV industry.
This paper is structured as follows: “Technological knowledge depreciation and present approaches for estimating the depreciation rate” section presents the concept of TK depreciation and provides an overview of prior research on estimating the depreciation rate. “Patent citation data and methodological approach ” section presents our method and its logic in detail. “Technological knowledge depreciation for solar PV” section demonstrates the application of knowledge deprivation rates, while the final section provides discussion and concluding remarks.
Technological knowledge depreciation and present approaches for estimating the depreciation rate
Defining technological knowledge depreciation
TK has both commercial and technological value. The commercial value is represented as providing an entity with the commercial competitive advantage over industry peers, while the technological value is its potential for facilitating future inventions (de Rassenfosse and Jaffe 2018). The commercial value is always related to private innovation capital, but the technological value can also refer to the social and public-good effect (de Rassenfosse and Jaffe 2018). Because this study aims to deepen the understanding of the evolution of clean technologies, which is associated with social welfare, we focus on the depreciation of knowledge’s technological value.
There are two sources of TK depreciation: obsolescence and loss. Regarding obsolescence, and dating back to Schumpeter (1934), although technology invention processes have long been recognized as the recombination of existing knowledge, new invention always builds on recent technologies rather than old technologies because new technologies can better meet human needs (Arthur and Polak 2006; Grubler and Nemet 2012). Historical cases such as the invention of the locomotive destroying the carriage and blacksmithing industries can also speak the decay of old technologies as well as the obsolescence of underlying knowledge.
In addition to obsolescence, TK can also be lost. For example, as knowledge is embodied in peoples’ minds, staff turnover or forgetting will lead to the loss of knowledge. Both knowledge loss and knowledge obsolescence result in the depreciation of TK capital (Grubler and Nemet 2012).
Reviewing the available estimation methods
The TK depreciation rate, which reflects the trend of the economy and determines the decay of the TKS, is an important economic parameter (de Rassenfosse and Jaffe 2018). Scholars are keen to assess it, but among the available estimation methods, there is a significant divergence on identifying the actual knowledge input. Some researchers measure it with R&D investment data while others use patent data, which in turn leads to two main streams of employed methods.
In the first stream, scholars regard R&D investment as knowledge input and its discounted cumulant as the TKS. Some researchers use the accounting approach, in which the TKS works as a factor determining private firms’ forward-looking revenues and consequently influences the market value (Griliches 1981; Hall 2005) or firm earnings (Ballester et al. 2003). Scholars have also associated R&D investment with firms’ realized profits. In this line of inquiry, scholars derive the depreciation rate of knowledge capital by estimating production functions (Bernstein and Mamuneas 2006; Hall 2005; Huang and Diewert 2011). Both the forward- and backward-looking approaches rely on in-depth traditional economic theory but frequently at the cost of unreasonable assumptions, such as the constant R&D investment growth rate and the complete market. In addition, R&D data are not always available in certain technology sectors, which significantly decreases the repeatability and reproducibility of these methods and results.
In the stream based on patent data, the patent renewal model is the main method that dates back to the work of Pakes and Schankerman (1984). The basic premise of this model is that patentees will renew a patent only if its expected stream of revenue is higher than the renewal fees. Although some studies have refined this model (even containing patents’ option value) (Baudry and Dumont 2006; Bessen 2008; Pakes 1986), the dependence on the assumed renewal fees and value distribution of the patent still need to be considered with caution. Another misgiving of the renewal model is subtler. As renewal decisions are made by staff, the bounded rationality will inevitably influence the objectiveness of these choices.
Park et al. (2006) proposed that the knowledge depreciation rate could be simply calculated as the inverse mean age of the patents cited, which is based on technology cycle theory (TCT), and fully assessed using patent citation data. As a consequence, this method can be replicated across multiple technology sectors. However, the TCT method doesn’t control for the many possible exogenous growth factors in the patent system. The backward-citation setting may also be confusing as we cannot identify which part of the knowledge is depreciated at the calculated rate.
The method proposed in the present study is purely built on patent citation data. We disentangle exogenous patent growth and focus on forward citation distribution. The forward-looking perspective makes the calculation logic clearer and thus is expected to provide a novel and improved methodological approach to the relevant literature.
Patent citation data and methodological approach
Patent citation and knowledge depreciation
During TK production processes, recent and active knowledge serves as an input in the generation of knowledge, while other perhaps older and outdated knowledge is disregarded (Arthur 2009; Arthur and Polak 2006). The depreciation of knowledge, through obsolescence or forgetting, is a durable process and could be reflected in the decreasing frequency of use (Jaffe and Trajtenberg 1996). We believe that patent citation data are superior for exploring knowledge depreciation patterns for the following reasons.
First, patent citations are a valid proxy for the technological components that are included in inventions (Keijl et al. 2016; Trajtenberg et al. 1997). Tracing patent citation records, we can monitor which patent uses the component contained in a focal patent (the forward citation records), and the origin of components contained in a focal patent (the backward citation records) (Trajtenberg et al. 1997). Patent citation data contain detailed information on timing, e.g., the time a particular invention was developed, which in turn enables an exploration into the changes of a patent’s activeness over time, and thus reveal the depreciation pattern.
The second reason is that patent citations’ temporal distribution contains both the knowledge obsolescence and knowledge loss factors, which reflect the two sides of knowledge depreciation. For the obsolescence factor, knowledge created to solve old problems tends to raise new problems and exogenous shocks. New problems stimulate the invention of new knowledge (Arthur 2009). As a result of this evolutionary process, firms and their inventors need to use new knowledge, because it represents the solution to the latest problems that may not be solved using old knowledge (Kogler 2016). Regarding knowledge loss, inventors also have the "temporal local search" nature, because recently created knowledge occupies the freshest memory of firms and is embodied in their routines and using old knowledge requires extra effort to review it as it has been out of the company and its inventors’ memory (Nerkar 2003). Besides, the knowledge flow embedded in labor networks can be traced via patent data (Almeida and Kogut 1999; Lee et al. 2019), which provides the potential to explore knowledge loss caused by inventor turnover.
Patent citation data is easily available, well codified, and have been generally tested in technology production and innovation processes related research (Corredoira and Banerjee 2015; Jaffe and Trajtenberg 1996; Trajtenberg et al. 1997). Its temporal distribution problems have been thoroughly explored for a long time in both physics and knowledge diffusion fields (Higham et al. 2017a, b; Jaffe et al. 1993), from which we can borrow ideas to solve our problem.
Patent citation temporal distribution: combining growth and depreciation
Scholars have found that the probabilities of a patent being cited increase first and then decrease. Usually, patents tend to receive most of their citations within the first 5 years after grant (Jaffe and Trajtenberg 1996). To explain this phenomenon, especially while the mathematic function remains the subject of intense study, investigators focusing on knowledge diffusion have reached a consensus that there are at least two primary mechanisms dominating the patent citation temporal distribution. The first is the growth of the citation network caused by knowledge diffusion processes. Patent citation networks follow a preferential-attachment dynamic, which means if one patent has already received more citations than others, it tends to receive also more later on (Higham et al. 2017a, b).
The second mechanism underlying the patent citation distribution is aging, which captures the fact that the novelty of each patent fades over time (Wang et al. 2013). As the value of the later works is recognized, old patents gradually become obsolete until it is pointless to use or improve the old works through citation. It is apparent that knowledge ages after its birth (Higham et al. 2017a, b; Wang et al. 2013).
With the combination of the two aforementioned mechanisms and other potentials, predicting patent citation has long been elusive. Higham et al. (2017a) separated patent citation rates into two distinct parts: a potentially time-dependent aging function and a completely time-independent preferential-attachment-type growth kernel. After controlling for the exogenous causes of increasing citations, the citation rate for patents at time t can be expressed as:
where \(\lambda (t)\) is the citation rate of one patent at time t, \(A(t)\) is an exponent aging function, and \(f\left( t \right) \sim k^{\alpha }\) corresponds to the preferential-attachment dynamics. To identify the depreciation part, we need to separate the preferential-attachment dynamic from the citation temporal distribution.
Identifying the depreciation rate in solar PV industry: uncoupling two dynamics
Patent data in solar PV industry
To identify the TK depreciation rate in the solar PV industry, we collected granted patents relevant to solar PV applied from 1963 to 2015. The data were derived from the Thomson Innovation (TI) global patent database. Patents relevant to solar PV were identified using a search string developed byBinz et al. (2017), which resulted in a sample of 199,786 granted patents.Footnote 1 In order to prevent double counting of single inventions patented in multiple countries, all patent applications in the same Derwent World Patent Index (DWPI) patent family were recorded as one observation.
There are two available patent families in the TI database (INPADOCFootnote 2 and DWPI families). In this study, granted patents are gathered in the DWPI families which are more restricted. DWPI families are ‘expert-validated families based on novel technical content’ and are ‘constructed based on the ‘‘novelty principle”’ (Martínez, 2011, p. 47). In DWPI families, ‘a new family member to be added to a given family, it needs to have matching technical content with existing members’ (Martínez, 2011, p. 47), which makes sure that patents in one family protect the same and new technical content. Thus, it is consistent with our objective to trace the depreciation process of new knowledge. After combining patents in one patent family, we obtained 53,459 patent families. The analyses that follow in the present investigation are all based on this very patent family level.
For each patent family, the earliest priority date is used (referred to as the application date of a patent family in following analyses), which essentially is the earliest application date of the invention in any patent office of the world (Binz et al. 2017). The aim here is to determine the date that is closest to when the invention was actually created (Binz et al. 2017). We also collect the earliest publication date (referred to as the publication date of a patent family in following analyses) in each family, which is the date after which a particular piece of novel knowledge could be cited by the public, and, thus, this is also the actual date from which knowledge depreciation could be observed through citation dynamics.
In order to conduct patent family level citation analysis, we assign patent citations received by each granted patent to the corresponding patent family. After omitting the citations from patents that are not in our solar PV granted-patent sample, the family self-citations, and the repeated citations between two patent families, our final citation dataset contains 228,322 observations.
To explore the depreciation of knowledge belonging to different countries, we attempt to assign patent families to certain countries. In this context, we mainly use the ‘inventor criterion’, under which patent families are assigned to inventors’ residence countries, due to its good match to research and development (R&D) activity location (de Rassenfosse et al. 2013).
To be more specific, for each patent family, we assign it to the available geographic information of its patent member with the earliest application date because this patent within the family should be closest to the actual knowledge creation date. During the process of assigning patent families to their inventor residence countries, we also include inventor information derived from PATSTAT, a worldwide patent statistical database provided by EPO as well as the information in the TI database in order to maximize the ratio of this kind of geo-assignment exercise. For patent families that contain no inventor residence information, we assign these to patentee countries. If neither inventor nor patentee information is available, the patent families would be assigned to the earliest priority country in each family. Footnote 3Eventually, around 72% of the patent families are assigned to the inventor residence countries, around 6% are to patentee countries, and 22% are to the earliest priority countries (see Table 1 for a detailed overview).
Rescaling citation records: accounting for citation inflation
We observe that the additional citation curve doesn’t always follow an expected pattern showed in Jaffe and Trajtenberg (1996) that patents receive most of their citations in the first few years after grant. For example, patentsFootnote 4 in the solar PV industry published in 1981, 1990, and 1995 all received most of their citations from solar PV patents in around 2008 (Fig. 1), which is not consistent with many other studies (Jaffe and Trajtenberg 1996; Nemet 2009). This is due to a complex interplay of extrinsic and intrinsic factors that need to be considered and that raises other important questions regarding the growth patterns and dynamics of citation patterns in this particular technology field.
In the solar PV industry, the number of annual patent applications soared to over 6000 in 2011 from less than 1000 in 2000 (see Fig. 2). As new patents may need to cite the current knowledge stock, a sharp increase in the knowledge production output will most likely result in an increase of citations to existing, and even older, patents. However, an increased knowledge generation speed per se does not necessarily lead to a surge in citations to older patents. One also needs to consider how many citations new patents make in general, which is associated with the general average number of citations made by each patent in the respective field. Figure 3 shows that the average number of backward citations (from solar PV patents) received by each solar PV patent applied in different years. We find that the number increased significantly from less than 4 in 1981 to over 8 in around 2008.
The combination of growing patent application numbers along with the additional increase in average backward citation numbers results in citation inflation that can obscure the real trend in knowledge depreciation (Higham et al. 2017a). To correct for this problem, one efficient approach is to remove the source of systematic variation in the citation behavior over time (Hall et al. 2001; Higham et al. 2017a, b). In the present investigation, and following Higham et al. (2017a, b), we treat patenting increase as a comprehensive exogenous factor, which represents the combined effect of various factors like resource availability, policy bias, market trends, etc. These exogenous factors are not associated with the utility of prior technology and thus should be removed (Higham et al. 2017b). As for the average citation numbers among patents, these changes reflect that the linkage between new and old knowledge became either weaker or stronger (Chen 2017), which should be treated as an intrinsic factor in the knowledge production system (Higham et al. 2017b).
In specific, to deflate the citation inflation, we employed a similar strategy as pursued by Higham et al. (2017a, b). All of the citations to each focal patent are binned in 6-month periods after the publication year of the focal patent. The citation incoming dates are the application dates of citing patents. We “deflate” the additional citations in each 6-month period to each focal patent with the inflation-adjusting factor:
where \(\Delta T\) is 6 months and \(N(T,\Delta T)\) is the number of solar PV patent applications in the time interval \([T,T + \Delta T]\). Y indicates January 1st of the publication year of a focal patent.
For instance, if the focal patent is published in the year of 1983, Y denotes January 1st, 1983. \(N(Y,\Delta T)\) denotes the number of solar PV patent applications in the first half of 1983. If there are twice as many patent applications in the second half of 2010 as the applications in the first half of 1983, citations to the focal patent incoming in the former period would be given a weight of 0.5 to deflate the exogenous growth and to make the citations be “equivalent focal time citations”. Figure 4 provides the number of additional inflation-adjusted patent citations (from solar PV patents) in each 6-month period, which shows that this approach can deal with the extreme growth in the PV market in around 2008.
Separating knowledge depreciation from preferential-attachment dynamics
To make the best use of our data, the cohort of patents published between 1981 and 2000 was chosen as the focal patents of the investigation. Intra-industry patent citations to a focal patent were used to identify the depreciation rate. All citations to each patent are binned in 6-month periods according to their application dates after the focal patent’s publication year. That is, for a patent published in 1981, its citations received after 31 December 1981 would be binned in 6-month periods, while a citation before that date would be treated as an initially accrued citation. All citations to our focal patents are assigned a time t which denotes the time lag between the publication of the cited patent and the application of the citing patent.
To separate knowledge depreciation from preferential-attachment dynamics, we followed the method provided by Higham et al. (2017a, b) after we binned each citation to the respective 6-month period:
Step1: we established two time series for each focal patent: one is the number of additional citations received within each 6-month period. The other is the number of accumulated citations at the beginning of each time period. We denote the accumulated citation numbers as k and the additional citations within a 6-month period as \(\Delta k\). All these citations, including the accumulated citations, indicate inflation-adjusted citations.
Step2: we bin the citation count data on each focal patent into groups with similar k and t. This process could be explained with an L–t matrix (see Fig. 5), in which L represents the smallest integer that is not less than the logarithmic value of \(k + 1\) (in this study, the base of the logarithmic value is set to 1.5, with which we can get enough useful bins. We calculate the logarithmic value of \(k + 1\) in case k = 0) and t is the corresponding time lag. For example, if a patent received 3.1 (inflation-adjusted) citations in the third 6-month period after its publication year, and it had 0.3 (inflation-adjusted) accumulated citations at the beginning of the period. Because \({\text{0 } < \text{ log}}_{1.5} (1 + 0.3) \le 1\), and there is 1-year lag from the publication to receiving these citations, the number 3.1 will be put into the unit (t = 1 year, L = 1) in the L–t matrix. Citations to other focal patents with the same t and similar k would also be put into this unit. Citations to patents that have no accumulated citation would be set in the bins with L = 0 as \({\text{ - 1 < log}}_{1.5} (1 + 0) \le {0}\). The purpose of implementing logarithmic binning in the accumulated citation number dimension is to balance the observation counts in each bin.
With each fixed L, we could calculate the mean number of additional citations in each 6-month time period,\(\Delta k(t,t + \Delta t)\), and then obtain the respect time series. Each time series would reflect the changing of utility (citation rates) across the time of a certain group of patents (with a particular range of accumulated citations). We estimate the linear function between \(\ln (\Delta k(t,t + \Delta t))\) and time lag t, using weighted linear least-squares regression and the weights are the proportion of patents number in each data point. The coefficient of time t (\(e(t)\)) would reflect the extent of exponential depreciation for patents in certain bins.
To calculate the depreciation rate of the whole solar PV industry, we run the linear regression for different L-bins. The regression results are shown in Table 2 and Fig. 6. Figure 6a explicitly demonstrates that the slopes are similar among L-bins with L = 0, 2,3,4,5. Moreover, we also find a similar pattern as Higham et al. (2017a) that the higher the L is, the higher the intercept is, which is in line with the preferential-attachment dynamics of citation growth.
Regression results for the whole solar PV industry a the fitting lines, b the coefficients of t. Notes: In Fig. 6a, \(\ln (\Delta k(t,t + \Delta t))\) as a linear function of time lag t for fixed citation bins with the log-maximum at L = 0, 2, 3, 4, and 5. A logarithmic transformation has been conducted on \(\Delta k(t,t + \Delta t)\) in this panel, through which we could find the significant declining fitting lines showed in solid lines. Hollow circles are corresponding citation data. In Fig. 6b, hollow circles indicate coefficients of t (e(t)) estimated with weighted linear least-squares regression. Black dash-dot lines show the 95% confidence interval of e(t)s, and the red-solid line shows the weighted average value of e(t)s for bins with L = 0, 2, 3, 4, 5
Figure 6b gives the 95% confidence interval of each \(e(t)\)(black dash-dot lines) and the weighted arithmetic average (red solid line, −0.215) of the coefficients for L-bins with L = 0,2,3,4,5. The weights are the inverses of the variance for each \(e(t)\). We find that the average is located in the scope of the 95% confidence interval of the five \(e(t)s\), which shows the robustness of coefficients for varied L-bins.
For bins with L = 6,7,8, their \(e(t)s\) are higher than other bins, which indicates their relatively lower depreciation rates. This phenomenon makes sense because patents with pretty high-level citations may imply breakthrough inventions that would profoundly influence the evolution of technology (Corredoira and Banerjee 2015), and thus are expected to depreciate slowly.
We use the weighted average of \(e(t)s\) for bins with L = 0,2,3,4,5, which contain over 85% citation observations in the L–t matrix when t is between 0 and 12 years,Footnote 5 to denote the deprecation rate of the whole industry. In general, the TK depreciate rate in the solar PV industry estimated through the proposed method is around 21.5% per year.
We also employed this method to calculate the depreciation rates of knowledge from three different countries: the USA, Japan, and Germany. The country-level analyses are based on the citation data to patents assigned to certain countries in our patent cohort. Essentially, when calculating the rate for the USA, patents assigned to the USA and published between 1981 and 2000 become our focal patents. Citations on these focal patents are then processed in the same way as described above in order to identify the TK depreciation rate for the very country.
The rate in the USA is around 20.1%/year, in Japan is about 22.9%/year, and in Germany is about 21.2%/year. Although not the same, the rate in Japan is comparable to the estimate of 20.3%/year in Watanabe, Wakabayashi, and Miyazawa (2000) on technology depreciation rates in the PV industry, which identified the rates through a detailed industrial survey. All of the results are shown in Fig. 7.
The regression results for a the USA, b Japan, and c Germany. Notes: Top panels show the fitting lines between t and \(\ln (\Delta k(t,t + \Delta t))\). A logarithmic transformation has been conducted on \(\Delta k(t,t + \Delta t)\) in these panels, Bottom panels show the coefficients of t (e(t)) for varying L-bins. Figure 7 (a) the USA and (b) Japan show the regression results for bins with L = 0,2,3,4, and 5 (the base of logarithmic L-bins is 1.5), which is consistent with the setting of analysis for the whole industry. For (c) Germany, we set the logarithmic L-bin base as 2 due to the fewer observations, and then we have bins L = 0,1,2, and 3 that could have enough observations in each unit
Technological knowledge depreciation for solar PV
This section documents the remaining TKS building process in different countries with the previously calculated depreciation rates and illustrates the significance of knowledge depreciation.
First, following a simple assumption that there is a linear relationship between research, development, and demonstration (RD&D) investment and knowledge creation, which has been employed in the literature (e.g., Grubler and Nemet 2012; Watanabe et al. 2000), we use solar PV RD&D expenditures derived from the International Energy Agency(IEA)Footnote 6 as an indicator of knowledge input (see Table 4 in the Appendix). The work of Kim and Wilson (2019) provides subtler evidence for the rationality of this assumption. According to Kim and Wilson (2019), the share of renewable energy RD&D expenditure in the six technology fields of the EU's Strategic Energy Technology (SET) PlanFootnote 7 is around 27%. The shares of renewable energy codified knowledge creation indexes associated with patenting (i.e. patent applications and citation weighted patent applications) are similar to the share of RD&D expenditure, which implies the linear relationship between RD&D investment and technological knowledge creation in the renewable energy industry. Solar PV as one of the most important renewable technologies may share a similar pattern with the whole of renewable energy technologies.
Figure 8 shows the knowledge input statistics of Germany, Japan, and the USA from 1974 to 2015. The USA employed a different investment strategy from Japan and Germany. Compared with other countries, the USA invested much more in the early stage of the industry (before the 1980s), but then sharply reduced RD&D budgets. Around 2009, the USA underwent a second round of investment growth in the PV industry. In contrast to the high but erratic investment pattern in the USA, Germany and Japan 's patterns are low but stable.
To calculate the remaining TKS over time with the corresponding knowledge depreciation rates obtained in our study, the annual expenditures are discounted and then summed up. The mathematical expression of the remaining TKS is:
where \(TKS_{T,c}\) denotes the remaining TKS of country c in year T, \(RD\& D_{T,c}\) denotes the RD&D expenditure of country c in the solar PV industry in year T, \(e_{c}\) is the knowledge depreciation rate in country c.
Figure 9 shows the calculated results. We can find that without sufficient investment to recharge TKS, the USA' technological advance built through the early boom investment period eroded quickly. At the end of 2015, only 12.19% of the original RD&D remained in the USA PV industry (642.22 million US$2018 out of the total 5,266.96 million US$2018), the ratios in Japan and Germany are 14.79% and 13.25% respectively (see Table 3). The remaining ratio gap does not purely result from the varying depreciation rates. An important finding is that although the USA’s knowledge depreciation rate is lower than the rate in Japan and Germany, the remaining ratio in the USA is still lower than Japan’s and Germany’s, which indicates that the expenditure pattern is an important factor for knowledge stock creation. Consistent RD&D investment is important in a changing world with knowledge depreciation because TKS needs continuous recharging.
Existing R&D policy research has dealt with the relationship between policy consistency and technological innovation. Researchers reached a consensus on giving consistent signals to economic agents in the early stage of emerging industries to maintain innovation and reduce uncertainty (Yoon et al. 2017). Considering TK depreciation, we provided another reason for the importance of R&D policy consistency: sufficient continuous R&D input is essential for recharging TKS. Erratic investment patterns make TKS vulnerable to erosion, resulting in a substantial waste of R&D expenditures in the long run.
However, the importance of early-stage investment demonstrates some important factors that have not been previously considered in association with knowledge depreciation: the timing of R&D investment and technological learning. Accumulated R&D investment, especially in the early stage, induces technological learning to decrease the cost of new technology and then stimulate its diffusion (Ma 2010). We thus wonder whether knowledge created in different time periods experiences varying decay processes.
While exploring the time-dependent knowledge depreciation rate, an intuitive hypothesis could be that knowledge created in the early stage is important to stimulate following inventions and thus would be more durable (with lower knowledge depreciation rates).Indeed, grouping our whole solar PV patent dataset into five-year periods, beginning in 1981–1985 and ending in 1996–2000, we find that the depreciation rate for knowledge published in the period 1981–1985 is around 21.4%/year, in 1986–1990 and 1991–1995 are around 20.3%/year and 19%/year, while in 1996–2000 is around 24.8%/year.Footnote 8 This result cannot justify the importance of early-stage knowledge, because the rate for the period 1981–1985 is higher than that for the following two periods.
However, it is still too arbitrary to deny the influence of knowledge production period and its impact on the knowledge depreciation rate, because we have not considered knowledge content change across time. In the case of the solar PV industry, according to Huenteler, Schmidt, Ossenbrink, and Hoffmann (2016b, p. 110), “In solar PV the focus shifts over time from product innovations, which represent an average of 64% of the weight between 1972 and 1985, to process innovations with an average 73% of the weight in 1990–2009.” This raises the question if a potential shift in inventive focus impacts observed knowledge depreciation rate trends over time; something that should be explored in future research efforts.
Discussion and concluding remarks
The rate of TK depreciation is a critical economic parameter, but its measurement is still an open question in the innovation research area. The main purpose of this study was to identify the TK depreciation rate with a readily available data source, i.e. patent citation data, and which can also be applied in other technology domain studies. Borrowing ideas from recent investigations into citation behavior, we equipped the patent citation data with an exponential aging function after adjusting for citation inflation and after separating the preferential-attachment factor that indicates a certain annual depreciation ratio.
In the case of the solar PV industry, the aggregate industry TK depreciation rate is about 21.5% per year, but rates vary across different countries. The rate in the USA is 20.1% per year, while in Japan and Germany it is around 22.9% and 21.2% per year, respectively. Using the estimated TK depreciation rates and the knowledge build-up rate via RD&D, we calculate the PV industry’s remaining TKS over time. The erratic RD&D expenditures that took place in the USA led to a sharp decline in the TKS. In contrast, Japan and Germany have lower but more stable RD&D expenditures than the USA, which resulted in a more efficient knowledge recharging process. When considering TK depreciation, it is important to emphasize the need for a continuous recharge mechanism, which could be stable R&D efforts and consistent stimulation policies.
We also explore time-varying changes in the TK depreciation rate but have not found a significant trend. In our analysis of four five-year periods across 1981–2000, knowledge in the first and last five-year periods experiences a relatively higher depreciation rate than the periods in-between. Future research efforts should also consider other time-dependent factors, e.g., in the solar PV case, the shift from product to process invention in around 1985 should be considered to explain changing trends.
In addition, how a shift in invention focus might reflect the nature of technologies themselves could be relevant. Compared to the solar PV case, it could be very well that more complex technology, like wind turbine inventions, could experience shifts through different product components that comprise the overall technology (Huenteler et al. 2016b), which further reflects the difference on technology granularity. Indeed, there is some evidence showing that more granular technologies may be less susceptible to changes in the overall innovation environment (Grubler and Nemet 2012; Wilson et al. 2012). Future research efforts should aim to understand the link between TK depreciation rate and the technological nature of specific technology domains.
Besides the use of patent data to identify the TK depreciation rate, the present investigation has some further limitations that should be noted. First, we find that most citations in our dataset come from US patent families (see Table 5 in the Appendix). This bias may be relevant to the difference in technological systems across the world (Bacchiocchi and Montobbio 2010), which may in turn make our results based on the patent family level analyses biased towards the citations to/from US-developed patents and toward the citations from the USPTO.Footnote 9 Future research efforts could circumvent this bias by comparing the depreciation of TK developed abroad with that of TK developed domestically as well as including the effect of patent offices’ practices.
Second, since patents represent codified knowledge, we cannot use it to capture the depreciation of tacit knowledge embodied in the staff’s mind, organization, and even in institutional memory. In addition, the present method requires a sufficient amount of observations over a long period and thus might not be suitable for technologies that have emerged only very recently.
Notes
The Thomson Innovation (TI) global patent database collects all patents in one family. During the patent collection process, we first used the specific search string to identify solar PV patents. After that, and in order to make the search more comprehensive, we selected all the granted patents that could also be assigned to the same INPADOC families that matched our solar PV patent samples.
INPADOC families are for applications directly or indirectly linked through priorities, which protect the same or related inventions (Martínez 2011).
For patent families with both inventor and priority country information, we calculate the ratio of patent families with identical inventor residence and priority country information and find that the match-ratio is over 90%. In the same way, the ratio of patent families that contain a match between the inventor residence and patentee country information is over 96%.
In the following analyses, ‘patent’ would indicate ‘patent family’ mentioned in “Patent data in solar PV industry.” section
Patent applications are declining sharply after 2012 most likely due to the time lag between application and publication. Our focal patents are published between 1981 and 2000. Placing t between 0 and 12 avoids using data on patents applied after 2012, which in turn circumvents that application-publication lag.
In IEA data services website https://wds.iea.org/WDS/Common/Login/login.aspx, the fold Energy Technology RD&D Budgets(2019 edition) contains the Detailed Country RD&D Budgets report, in which people could find the solar PV RD&D expenditures of the three countries.
The six technology fields denote: Renewable Energy, Smart Grid, Energy Efficiency, Sustainable Transport, Carbon Capture and Storage, and Nuclear Power.
These time-variant depreciation rates are calculated based on the whole dataset, which is regardless of country of origin.
Bacchiocchi and Montobbio (2010, p. 461) show that for equivalent patents (patent members in one patent family), these receive many more citations from USPTO than from EPO.
References
Almeida, P., & Kogut, B. (1999). Localization of knowledge and the mobility of engineers in regional networks. Management Science, 45(7), 905–917.
Arthur, W. B. (2009). The Nature of Technology: What it is and how it Evolves. New York: Free Press.
Arthur, W. B., & Polak, W. (2006). The evolution of technology within a simple computer model. Complexity, 11(5), 23–31.
Bacchiocchi, E., & Montobbio, F. (2010). International Knowledge Diffusion and Home-bias Effect: Do USPTO and EPO Patent Citations Tell the Same Story? The Scandinavian Journal of Economics, 112(3), 441–470.
Ballester, M., Garcia-Ayuso, M., & Livnat, J. (2003). The economic value of the R&D intangible asset. European Accounting Review, 12(4), 605–633.
Baudry, M., & Dumont, B. (2006). Patent Renewals as Options: Improving the Mechanism for Weeding Out Lousy Patents. Review of Industrial Organization, 28(1), 41–62.
Bernstein, J. I., & Mamuneas, T. P. (2006). R&D depreciation, stocks, user costs and productivity growth for US R&D intensive industries. Structural Change & Economic Dynamics, 17(1), 70–98.
Bessen, J. (2008). The value of U.S. patents by owner and patent characteristics. Research Policy, 37(5): 932–945.
Binz, C., Tang, T., & Huenteler, J. (2017). Spatial lifecycles of cleantech industries – The global development history of solar photovoltaics. Energy Policy, 101, 386–402.
Chen, L. (2017). Do patent citations indicate knowledge linkage? The evidence from text similarities between patents and their citations. Journal of Informetrics, 11(1), 63–79.
Corredoira, R. A., & Banerjee, P. M. (2015). Measuring patent's influence on technological evolution: A study of knowledge spanning and subsequent inventive activity. Research Policy, 44(2), 508–521.
de Rassenfosse, G., Dernis, H., Guellec, D., Picci, L., & de la Potterie, B. V. P. (2013). The worldwide count of priority patents: A new indicator of inventive activity. Research Policy, 42(3), 720–737.
de Rassenfosse, G., & Jaffe, A. B. (2018). Econometric evidence on the depreciation of innovations. European Economic Review, 101, 625–642.
Griliches, Z. (1981). Market value, R&D, and patents. Economics Letters, 7(2), 183–187.
Grubler, A., & Nemet, G. (2012). Sources and Consequences of Knowledge Depreciation. Historical Case Studies of Energy Technology Innovation in: Chapter 24. In A. Grubler A., F., Gallagher,, & H. K.S., M., Jiang, K., Mytelka, L., Neij, L., Nemet, G. & C. Wilson. (Eds.), The Global Energy Assessment. Cambridge, UK: Cambridge University Press.
Hall, B. H. (2005). Measuring the Returns to R&D: The Depreciation Problem. Annales Déconomie Et De Statistique, 2005(79/80), 341–381.
Hall, B. H., Jaffe, A. B., & Trajtenberg, M. (2001). The NBER patent citation data file: Lessons, insights and methodological tools: National Bureau of Economic Research.
Higham, K. W., Governale, M., Jaffe, A. B., & Zulicke, U. (2017a). Fame and obsolescence: Disentangling growth and aging dynamics of patent citations. Physical Review E, 95(4).
Higham, K. W., Governale, M., Jaffe, A. B., & Zulicke, U. (2017b). Unraveling the dynamics of growth, aging and inflation for citations to scientific articles from specific research fields. Journal of Informetrics, 11(4), 1190–1200.
Huang, N., & Diewert, E. (2011). Estimation of R&D depreciation rates: a suggested methodology and preliminary application. Canadian Journal of Economics/revue Canadienne Déconomique, 44(2), 387–412.
Huenteler, J., Ossenbrink, J., Schmidt, T. S., & Hoffmann, V. H. (2016a). How a product's design hierarchy shapes the evolution of technological knowledge-Evidence from patent-citation networks in wind power. Research Policy, 45(6), 1195–1217.
Huenteler, J., Schmidt, T. S., Ossenbrink, J., & Hoffmann, V. H. (2016b). Technology life-cycles in the energy sector — Technological characteristics and the role of deployment for innovation. Technological Forecasting and Social Change, 104, 102–121.
IRENA. (2017). Boosting solar PV markets: The role of quality infrastructure. Abu Dhabi: International Renewable Energy Agency.
Jaffe, A. B., & Trajtenberg, M. (1996). Flows of knowledge from universities and federal laboratories: Modeling the flow of patent citations over time and across institutional and geographic boundaries. Proc Natl Acad Sci U S A, 93(23), 12671–12677.
Jaffe, A. B., Trajtenberg, M., & Henderson, R. (1993). Geographic Knowledge Spillovers as Evidenced by Patent Citations. Quarterly Journal of Economics, 108(3), 577–598.
Johnstone, N., Haščič, I., Poirier, J., Hemar, M., & Michel, C. (2012). Environmental policy stringency and technological innovation: evidence from survey data and patent counts. Applied Economics, 44(17), 2157–2170.
Keijl, S., Gilsing, V. A., Knoben, J., & Duysters, G. (2016). The two faces of inventions: The relationship between recombination and impact in pharmaceutical biotechnology. Research Policy, 45(5), 1061–1074.
Kim, Y. J., & Wilson, C. (2019). Analysing energy innovation portfolios from a systemic perspective. Energy Policy, 134, 110942.
Kogler, D. F. (Ed.). (2016). Evolutionary Economic Geograph - Theoretical and Empirical Progress. New York: Routledge.
Lee, C., Kogler, D. F., & Lee, D. (2019). Capturing information on technology convergence, international collaboration, and knowledge flow from patent documents: A case of information and communication technology. Information Processing & Management, 56(4), 1576–1591.
Ma, T. J. (2010). Coping with Uncertainties in Technological Learning. Management Science, 56(1), 192–201.
Martínez, C. (2011). Patent families: When do different definitions really matter? Scientometrics, 86(1), 39–63.
Nemet, G. F. (2009). Demand-pull, technology-push, and government-led incentives for non-incremental technical change. Research Policy, 38(5), 700–709.
Nerkar, A. (2003). Old is gold? The value of temporal exploration in the creation of new knowledge. Management Science, 49(2), 211–229.
Pakes, A. (1986). Patents as Options: Some Estimates of the Value of Holding European Patent Stocks. Econometrica, 54(4), 755–784.
Pakes, A., & Schankerman, M. 1984. The rate of obsolescence of patents, research gestation lags, and the private rate of return to research resources. Nber Chapters.
Park, G., Shin, J., & Park, Y. (2006). Measurement of depreciation rate of technological knowledge: Technology cycle time approach. Journal of Scientific & Industrial Research, 65(2), 121–127.
Perruchas, F., Consoli, D., & Barbieri, N. (2020). Specialisation, diversification and the ladder of green technology development. Research Policy, 49(3), 103922.
Rosenberg, N. (1963). Technological Change in the Machine Tool Industry, 1840–1910. Journal of Economic History, 23(4), 414–443.
Schumpeter, J. A. (1934). The theory of economic development. Cambridge, MA: Harvard University Press.
Trajtenberg, M., Henderson, R., & Jaffe, A. (1997). University Versus Corporate Patents: A Window On The Basicness Of Invention. Economics of Innovation and New Technology, 5(1), 19–50.
Wang, D., Song, C., & Barabási, A. L. (2013). Quantifying long-term scientific impact. Science, 342(6154), 127–132.
Watanabe, C., Wakabayashi, K., & Miyazawa, T. (2000). Industrial dynamism and the creation of a “virtuous cycle” between R&D, market growth and price reduction: The case of photovoltaic power generation (PV) development in Japan. Technovation, 20(6), 299–312.
Wilson, C., Grubler, A., Gallagher, K. S., & Nemet, G. F. (2012). Marginalization of end-use technologies in energy innovation for climate protection. Nature Climate Change, 2(11), 780–788.
Yin, Y., & Wang, D. (2017). The time dimension of science: Connecting the past to the future. Journal of Informetrics, 11(2), 608–621.
Yoon, J., Oh, Y., & Lee, J. D. (2017). The impact of policy consistency on technological competitiveness: A study on OECD countries. Energy Policy, 108, 425–434.
Acknowledgements
We are grateful to the two anonymous referees for comments and suggestions. This research was sponsored by the National Nature Science Foundation of China (Nos. 71571069 and 71811540350). The paper was developed based on the work in the Young Scientists Summer Program at the International Institute for Applied Systems Analysis, Laxenburg (Austria). We would like to acknowledge the technical assistance of Dieter F. Kogler (dieter.kogler@ucd.ie) via his TechEvo patent database that has been funded by the European Research Council under the European Union’s Horizon 2020 research and innovation programme (grant agreement No 715631, TechEvo) and by the Science Foundation Ireland (SFI) under the SFI Science Policy Research Programme (grant agreement No. 17/SPR/5324, SciTechSpace).
Author information
Authors and Affiliations
Contributions
All authors contributed to the study conception and design. Material preparation, data collection and analysis were performed by Jie Liu and Arnulf Grubler. The first draft of the manuscript was written by Jie Liu and Tieju Ma. The revised version was written by Jie Liu, Tieju Ma, and Dieter F. Kogler. All authors commented on previous versions of the manuscript. All authors read and approved the final manuscript.
Corresponding author
Appendix
Appendix
According to IEA: ‘There is a large increase in RD&D spending observed in 2009 due to the increased expenditures associated with the American Recovery and Reinvestment Act of 2009 (stimulus) spending. This is a one year appropriation (although actual expenditures may go into future years) and so 2010 saw a significant decrease.’ We have not specially processed the US 2009 data in the analysis because our main purpose is identifying the depreciation rate, rather than the knowledge stock. Using present US data can also show the importance of TKS continuous recharging.
Rights and permissions
About this article
Cite this article
Liu, J., Grubler, A., Ma, T. et al. Identifying the technological knowledge depreciation rate using patent citation data: a case study of the solar photovoltaic industry. Scientometrics 126, 93–115 (2021). https://doi.org/10.1007/s11192-020-03740-x
Received:
Published:
Issue Date:
DOI: https://doi.org/10.1007/s11192-020-03740-x