Abstract
It is well established that poor inhibitory control confers both a vulnerability to, and maintenance of, addictive behaviors across the substance and behavioral spectrums. By comparison, the role of compulsivity in addictive behaviors has received less research focus. The neurocognitive literature to date is vast, and it is unclear whether there are any convincing lines of systematic evidence delineating whether and how aspects of impulsivity and compulsivity are shared and unique across different substance and behavioral addictive disorders. Such information has significant implications for our understanding of underlying mechanisms and clinical implications for assessing and treating neurocognitive deficits across addictions. Here, we conducted a systematic meta-review of the quantitative meta-analyses to date, specifically examining the neurocognitive functions central to impulsive-compulsive behaviors transdiagnostically across addictive behaviors. Out of 1186 empirical studies initially identified, six meta-analyses met inclusion criteria examining alcohol, cannabis, cocaine, MDMA, methamphetamine, opioid and tobacco use, as well as gambling and internet addiction. The pooled findings across the systematic meta-analyses suggest that impulsivity is a core process underpinning both substance and behavioral addictive disorders, although it is not equally implicated across all substances. Compulsivity-related neurocognition, by comparison, is important across alcohol and gambling disorders, but has yet to be examined systematically. The gestalt of findings to date suggests that both impulsivity and compulsivity are core constructs linked to addictive behaviors and may not be solely the secondary sequelae associated with the effects of prolonged substance exposure.
Similar content being viewed by others
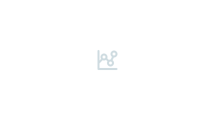
Avoid common mistakes on your manuscript.
Introduction
Currently, the neuropsychological assessment of individuals with addictive behaviors borrows heavily from established measures developed for use in acquired brain injury and other organic disorders (Lezak, Howieson, Bigler, & Tranel, 2012). A standard battery of neuropsychological tests typically characterises a broad spectrum of cognitive abilities, including intellectual functioning, processing speed, attention and working memory span, episodic memory and other, higher-order (executive) abilities (e.g. reasoning and conceptual flexibility; Lee et al., 2015). Despite impairments in these abilities having been linked to the neurobiological changes associated with chronic substance misuse (e.g., Stavro, Pelletier, & Potvin, 2013), the majority these dysfunctions do not appear to be linked to the motivation towards and lack of self-control to limit substance use or engagement with problem behaviors (exceptions include working memory; see Verdejo-Garcia, 2016). By contrast, many individuals with addictive behaviors exhibit neurocognitive deficits across a range of functions subserved by the motivational and self-regulatory circuitries, which heighten the risk of developing or maintaining approach behaviors across substance or behavioral addictions (Dalley, Everitt, & Robbins, 2011; de Wit, 2009; Hester, Lubman, & Yücel, 2010; Verdejo-Garcia, Lawrence, & Clark, 2008). Specifically, the tendency to act ‘impulsively’ and ‘compulsively’ are now considered two core impairments underpinning addictive behaviors, which contribute to poor recovery and relapse (Assadi, Yucel, & Pantelis, 2009; Bechara, 2005; Carter et al., 2016; Hester et al., 2010; Koob & Le Moal, 2001; Lubman, Cheetham, & Yücel, 2015; Verdejo-Garcia et al., 2012; Whelan et al., 2014; Yucel et al., 2012; Zalesky et al., 2012). Impulsivity is defined as “actions which are poorly conceived, prematurely expressed, unduly risky or inappropriate to the situation and that often result in undesirable consequences” (Daruna & Barnes, 1993), whereas compulsivity has been referred to as “actions which persist inappropriate to the situation, have no obvious relationship to the overall goal and which often result in undesirable consequences” (Dalley et al., 2011). Accordingly, the pathological expressions of these constructs are often maladaptive and related to impairments in top-down, cognitive control, but are distinct in other aspects of neurocognition (Robbins, Gillan, Smith, de Wit, & Ersche, 2012). Whereas impulsivity involves automatized responses with little forethought and is usually associated with a lack of insight, compulsivity tends to involve conscious consideration and deliberation (Dalley et al., 2011). Nevertheless, emerging evidence suggests that impulsivity and compulsivity are overlapping (i.e. both with elements of disinhibition) but distinct constructs (Yucel et al., in press) that cut across traditional diagnostic boundaries (Chamberlain, Stochl, Redden, & Grant, 2018; Prochazkova et al., 2018; Robbins et al., 2012; Yücel & Fontenelle, 2012) and may form a shared vulnerability to substance misuse and behavioral addictions. The robustness of this proposition remains an empirical question and necessitates a systematic evaluation of the extant literature. Clarifying whether and how the underlying structure of addictive disorders are similar and different transdiagnostically will contribute to the understanding of disease mechanisms and the classification of the relatively newer nosology of behavioral addictions (e.g., food addiction, Carter et al., 2016).
One key challenge in interrogating the constructs of impulsivity and compulsivity across addictive behaviors involves the multifactorial nature of these constructs, which are thought to encompass a range of biologically-validated neurocognitive functions (Robbins et al., 2012). Animal and human models have consistently shown that impulsivity comprises a minimum of three neurocognitive components (Broos et al., 2012; Dalley & Robbins, 2017; Fineberg et al., 2014; Torregrossa, Xie, & Taylor, 2012; Verdejo-Garcia et al., 2008):
-
i.
Response inhibition, that is, the inability to inhibit a pre-potent motor or cognitive response,
-
ii.
Reward discounting, including the tendency to choose a sooner, smaller reward over a later, larger reward (i.e., delay discounting), and
-
iii.
Disadvantageous decision-making, that is, difficulty weighing options, taking appropriate risks, and having a bias towards not using all the available information to reflect on the consequences of actions (reflection impulsivity).
By comparison, compulsivity is a relatively newer construct with other neurocognitive components (Yücel & Fontenelle, 2012). In addition to being underpinned by poor response inhibition, compulsivity has been argued to encompass at least four other neurocognitive domains (Fineberg et al., 2014; van Timmeren, Daams, van Holst, & Goudriaan, 2018):
-
i.
Contingency-related cognitive inflexibility, that is, difficulty shifting a learned behavior in response to changing stimulus-response contingencies,
-
ii.
Task or attentional set-shifting, that is, ability to switch attention between two competing tasks,
-
iii.
Attentional bias or disengagement, that is, difficulty disengaging from salient stimuli, and
-
iv.
Habit learning, that is, the learning of a conditioned stimulus-response that is insensitive to the goals or consequences of action.
Arguably, these neurocognitive components suggest that compulsivity may be a broader and more multifaceted construct than impulsivity. Borrowing from an obsessive-compulsive disorder framework, compulsivity additionally encompasses an affective component, which contributes to the irresistible urge to perform a behavior that may function to avoid or decrease a negative internal state (Chamberlain, Fineberg, Blackwell, Robbins, & Sahakian, 2006; Denys, 2011; Fineberg et al., 2014; Lubman, Yücel, & Pantelis, 2004) akin to the affective role of cravings in motivating approach behaviors. However, the affective processes underpinning impulsivity or compulsivity are beyond the scope of the current review and, as such, will not be further explored herein. Understanding how different neurocognitive functions underlying impulsivity and compulsivity are shared or unique to addictive behaviors transdiagnostically will allow us to better understand the cognitive mechanisms driving addictions. Clinically, it would assist with the quantification of addiction risk and relapse, the identification of those requiring more targeted and aggressive treatments, and the monitoring of treatment progress for interventions targeting these brain-based processes.
The second major challenge in consolidating and synthesizing the existing literature on impulsivity and compulsivity across the spectrum of addictive behaviors is the sheer scope of published studies to date, spanning the neuroscience, general medicine and psychology literatures. As such, instead of focussing on individual research studies, this review sought to employ a larger scope approach and systematically synthesize the published meta-analytic evidence examining impulsive and compulsive neurocognitive functions across addictive behaviors. We chose to be broad and not limit our review to a predetermined set of addictive disorders to ensure that we did not overlook certain domains of problem behaviors. A conscious decision was also made to limit our inclusion criteria to quantitative reviews, since it was not possible to reconcile qualitative observations with quantitative data. Further, we argue that quantitatively obtained summaries of extant findings would be more objective since it will be less open to subjective weighting of certain studies over others. Furthermore, the major advantage of synthesizing quantitative, meta-analytic findings rather than qualitative interpretations is that the former can numerically take into account biases associated with small samples, as well as being able to directly test the influence of clinical and demographic factors on heterogeneity across empirical studies (Lee et al., 2014). For the purposes of the current meta-review, we chose not to constrain our inclusion of studies based on a strict definition of what constitutes an ‘addiction’. It is clear from the literature that addiction is a broad concept, which has been difficult to define precisely (O'Brien, 2011; Sussman & Sussman, 2011). Further, as with many mental disorders there is a continuum of substance use behaviors ranging from no use to a threshold substance use disorder, with many categories such as heavy use, problematic use, and so on, falling intermediately along the spectrum. In order to incorporate all problematic use, we will use the term ‘addictive behaviors’ to refer to the full range of use. This is also consistent with the emerging dimensional psychiatry or Research Domain Criteria (RDoC) approaches, which espouses the use of transdiagnostic dimensions rather than arbitrarily defined categories to interrogate underlying disease phenotypes (Cuthbert, 2014; Cuthbert & Insel, 2013; Yucel et al., 2018).
Meta-Review Procedure
Consistent with previous meta-reviews (Chesney, Goodwin, & Fazel, 2014; Francke, Smit, de Veer, & Mistiaen, 2008), systematic searches of peer-reviewed meta-analytic studies up until February 2018 were conducted in PubMed and PsycInfo databases. Various combinations of search terms were used, including: review, meta-analy*, alcohol*, cannabis, marijuana, methamphetamine, opioid or opiate, heroin, nicotine, cocaine, gambl*, binge, addict*, risk-taking or risky decision, impuls*, compuls*, habit*, discounting, reward, expectancy, prediction error, cognitive control, and inhibition or disinhibition. These search terms were informed by a recent Delphi consensus study of international experts in addiction (Yucel et al., in press). Reference lists of relevant papers obtained through database searches were also checked for additional meta-analyses. Eligibility criteria were independently applied to all studies and coded for methodological quality by two raters (RSCL, SH).
Inclusion criteria were: i) a quantitative, meta-analytic review using a systematic search strategy reported in English according to the PRISMA and AMSTAR guidelines, ii) examining a neurocognitive task pertinent to impulsivity or compulsivity, and iii) a clearly defined addictive behavior with a clinical sample compared to a comparison control group. Meta-analyses were excluded if they: i) did not report the pooled means of task performance (e.g. only reporting correlations between tasks), or missing the inferential statistics associated with the standardized mean difference pooled scores (e.g., Z and P values), ii) collapsed different neurocognitive tasks into domain scores and did report task performance pooled individually (given the varying psychometric properties between measures), iii) used a systematic search strategy only to exhaustively search neuroimaging or questionnaire data (i.e., neurocognition was a secondary outcome and not exhaustively reviewed), and iv) there was a more recent, updated version of the meta-analysis published by the same researchers. Results for specific neurocognitive tasks were also excluded if: i) the pooled effect size used different scores from the same instrument (e.g., reaction time and total errors), or ii) if various versions of the same neurocognitive task were collapsed into the same analysis (e.g., substantially different probabilistic reversal learning tasks based broadly on the same paradigm).
The pooled data were extracted and organised by construct (e.g., impulsivity), measure (e.g., stop signal task), and addiction population (e.g., gambling disorder). The constructs were then summarised and interpreted according to the relevant neurocognitive constructs or subdomains (e.g., response inhibition).
Overview of Included Studies
Out of 1186 studies identified from database searches and reference lists, 228 articles were included for further screening of abstracts (see Fig. 1). Of these, 80 met eligibility criteria and underwent further, full-text assessment. In total, 74 papers were excluded, with the most common reason being a lack of systematic search strategy (n = 45). A total of six meta-analyses were included in the final quantitative synthesis (Chowdhury, Livesey, Blaszczynski, & Harris, 2017; Kovacs, Richman, Janka, Maraz, & Ando, 2017; Lipszyc & Schachar, 2010; Smith, Mattick, Jamadar, & Iredale, 2014; Stephan et al., 2017; van Timmeren et al., 2018). All included meta-analyses were published between 2010 and 2018.
There was substantial variation in how each meta-analysis defined their addictive behavior samples (see Table 1). Some opted for a constrained approach, and only included studies using narrowly-defined diagnostic thresholds (e.g., van Timmeren et al., 2018), whereas other studies opted for greater inclusion, selecting studies including both diagnostic as well as subthreshold addictive disorders (e.g., Smith et al., 2014). For the purposes of this meta-review, the verbatim diagnostic terminology used within a particular meta-analysis will be used henceforth (e.g., alcohol dependence or heavy drinking), given the differing thresholds applied across studies and difficulties with aggregating these approaches. No meta-analyses indicated a priori the specific setting or context required for study inclusion (e.g., inpatients who had undergone medically-managed detoxification). No meta-analyses explicitly included studies of individuals who were under the acute effects of intoxication although some did include current users. All reviews focussed on adults.
The six included meta-analyses represented a broad range of neurocognitive tasks across a breadth of addictive behaviors (see Table 2). In total, nine tasks were quantitatively reviewed across alcohol, cannabis, cocaine, MDMA, methamphetamine, opioid, and tobacco use, as well as non-substance-related addictive behaviors of gambling and excessive internet use. The neurocognitive tasks used were the stop signal task (SST) and go/no-go task (GNG), the Stroop interference test, Hayling test, Wisconsin card sorting test – perseverative errors (WCST), intra- or extra-dimensional set-shifting task – total errors (IED), card playing task – cards played (CPT), trail making test-part b (TMT-B), and the Iowa gambling task (IGT). In terms of the potential effects of diagnostic specificity and recent substance use affecting the standardized mean difference, the inclusion criteria are detailed in Table 1.
The methodological quality of included meta-analyses was also somewhat variable (Table 3). Most meta-analyses adhered to PRISMA guidelines in terms of methodology. However, on the whole, most reviews did not take into account potential risk of biases of included studies (e.g., poor matching to control groups, publication bias) or the quality of included studies.
Impulsivity Deficits
Based on the included studies, impulsivity was most commonly assessed using measures of response inhibition (SST, GNG task, Stroop, or Hayling tests) and disadvantageous decision-making (IGT). For a more detailed justification of how these measures tap into these functions, see Verdejo-Garcia et al. (2008).
The SST is commonly regarded as the gold standard paradigm for measuring response inhibition (Eagle & Robbins, 2003). In this task, participants are asked to press one button (e.g., left button) in response to a stimulus (e.g., left arrow), and another button (e.g., right button) in response to another stimulus (e.g., right arrow). These stimuli are known as ‘go’ trials. On a portion of trials, however, a ‘stop signal’ stimulus (e.g., a coloured dot or a beep sound) will appear after a delay (known as the ‘stop signal delay’; SSD), which will require the participant to inhibit the already initiated go response. The SSD is stair-cased (i.e. difficulty-level decreases or increases according to performance) to ensure performance is kept close to 50% correct. A ‘stop signal reaction time’ (SSRT) is computed from the distribution of responses indexing the average time required to inhibit a motor response.
The SSRT is one of the most commonly used measures of response inhibition, and was quantitatively reviewed across alcohol dependence (number of included primary studies, k = 6), heavy drinking (k = 7), cannabis dependence or chronic heavy use (k = 6), cocaine dependence or chronic heavy use (k = 9), methamphetamine dependence or chronic heavy use (k = 3), tobacco use (k = 5), internet addiction (k = 2), and gambling (k = 10). With the exception of tobacco- or cannabis-use and internet addiction, all other substance or addictive behaviors were associated with significant decrements in response inhibition performance as indexed by the SSRT. Specifically, methamphetamine use and gambling disorder were associated with medium to large effect size deficits in inhibitory control, followed by medium effect size decrements in the cocaine using pooled cohort. By comparison, alcohol dependence and heavy drinking were associated with the least pronounced response inhibition deficits at a small to medium effect size level, with the latter being the least impaired among the addictive behaviors with significant SST decrements. Cannabis use and gambling disorder were both associated with significant heterogeneity of effect sizes across studies. All other addictive behaviors were reported to exhibit no significant heterogeneity.
The GNG task is another commonly used measure indexing response inhibition (Gomez, Ratcliff, & Perea, 2007). In this task, participants are asked to make a response (e.g., press a button) when presented with a ‘Go’ stimulus (e.g. green shape), but not respond when presented with a No-Go stimulus (e.g., red shape). The number of No-Go commission errors is used to index poor response inhibition, although some have noted that this is not the purest measure of response inhibition since it also involves other, commonly impaired functions (e.g., poor sustained attention). Broadly, there are two configurations of the GNG task, one that includes frequent Go trials in order to predispose pre-potent Go responding, relative to much rarer No-Go trials. The second configuration of the GNG task includes equal proportions of the Go and No-Go trials and is considered by some as being less sensitive to response inhibition deficits (Smith et al., 2014).
The GNG task was reviewed across alcohol dependence (k = 10), heavy drinking (k = 9), cannabis dependence or chronic heavy use (k = 5), cocaine dependence or chronic heavy use (k = 7), chronic MDMA heavy use (k = 5), opioid dependence or chronic heavy use (k = 2), tobacco use (k = 6), internet addiction (k = 4) and gambling (k = 9). In descending magnitude of effect size from medium to small, response inhibition on the GNG task was deficient in those with alcohol dependence, cocaine use, MDMA use, and tobacco use. Effects were detected using the frequent-Go/rare-No-Go variation of the GNG task, with the exception of alcohol dependence where the equiprobable version was also able to detect a response inhibition deficit, albeit less strongly.
In terms of behavioral addictions, the most recent meta-analysis included more than three times the number of included studies than the earlier review, and identified a small to medium effect for response inhibition deficits in pathological gambling or gambling disorder. By contrast, studies were mixed for internet addiction, where the frequent-Go/rare-No-Go variation of the GNG task yielded results contradicting the equiprobable GNG task, whereby performance on the frequent-rare variant was paradoxically superior in those with excessive internet use compared to controls. The internet addiction findings need to be interpreted with caution given this was only based on two studies, and internet addiction being still a disorder under theoretical debate and requiring further empirical validation. Furthermore, inspection of the individual studies showed that this superior GNG finding was based on an outlier study with a lower threshold of inclusion for internet usage.
The Stroop colour-word interference test is a commonly used clinical and research instrument measuring disinhibition (Comalli, Wapner, & Werner, 1962). There are various versions of the task. Generally, it involves three parts. The first involves the participant reading a list of colour words (e.g. ‘green’, ‘red’, etc.) as quickly as possible. In the second part, the participant needs to name the coloured ink as quickly as possible. In the third and final part – the colour-word interference condition – participants are asked to name the ink colour whilst ignoring the printed word (where the printed word is the more automatic, pre-potent response that needs to be inhibited). Depending on the version of the Stroop task, the time taken in the colour-word interference condition or the number of correct responses in a set amount of time are commonly used to index cognitive inhibition.
The Stroop task has been quantitatively reviewed in alcohol use disorder (k = 17) and pathological gambling or gambling disorder (k = 9). Stroop task performance was deficient in both the alcohol use disorder and pathological gambling or gambling disorder samples at a medium effect size level.
The Hayling sentence completion test is a subscale from the Hayling and Brixton tests, indexing disinhibition (Burgess & Shallice, 1996). This task comprises two parts, administered by an experimenter or clinician. In the first part, participants are required to vocalise responses to fifteen sentence stems as quickly as possible with a word that fits the end of the sentence, both semantically and grammatically (i.e., paced cloze passage task). In the second condition, participants are asked to complete 15 sentences again but with a word that completely does not match or make sense when paired with the sentence stem. The number and types of errors, and response time for correct responses, are used to index cognitive disinhibition.
The Hayling task has only been meta-analysed in alcohol use disorder (k = 3). There was a very large pooled effect size deficit.
One ubiquitous task assessing disadvantageous decision making is the computerised IGT (Bechara, Damasio, Damasio, & Anderson, 1994). Participants are shown a deck of cards labelled A, B, C and D, and are instructed to select 100 cards from these decks. Each response will result in either a monetary win or loss. The A and B decks are disadvantageous decks with bigger wins but will result in a net loss over time due to larger penalties, whereas the C and D decks are advantageous in the longer term because they result in a net win despite yielding smaller gains during win trials. The net score is calculated by subtracting the number of selections from the disadvantageous decks from the advantageous decks.
Meta-analyses of the IGT has been conducted on alcohol use disorder (k = 22) and gambling disorder (k = 7). Gambling disorder was associated with a very large decrement in decision making on the IGT. Results for alcohol use disorder have been mixed, with a meta-analysis including fewer studies finding a large decision-making deficit and the other finding a medium sized deficit. This pattern or results was likely due to the stricter inclusion criteria of the former review, which only included studies that matched case to controls on age and educational attainment. Accordingly, the larger effect size decrement is likely to be more accurate and free from the confound of a poorly matched control group.
Compulsivity Deficits
Compulsivity-related neurocognition was most typically measured by tasks of attentional set-shifting (WCST, IED, TMT-B) and contingency-related cognitive inflexibility (CPT). For a more detailed description of how these measures index these functions, see van Timmeren et al. (2018).
The WCST is a traditional, clinical neuropsychological instrument that measures the ability to generate strategies to problem solve, and the ability to switch between concepts based on verbal feedback (Anderson, Damasio, Jones, & Tranel, 1991). This ‘flexibility’ or ‘reversal learning’ aspect of the WCST is considered to be closely related to the aberrant, strong habitual responding observed in compulsivity. In this task, participants are instructed to match 128 cards, one-by-one, to four key cards. They are not told how to match those cards, but they are told whether they are right or wrong. The cards show three types of stimuli – that can be categorised into colour, form and shape – and the participant is required to work out the rule to match each card and to change their strategy once the rule has changed. The number of mistakes they make in persevering with a previous, matching strategy, which is no longer correct, is known as ‘perseverative errors’ and is a common metric to index poor cognitive flexibility or reversal learning ability.
Meta-analyses have been conducted on the WCST in alcohol use disorder (k = 15) and pathological gambling or gambling disorder (k = 9). A medium effect size deficit was found in pathological gambling or gambling disorder, and a slightly larger medium to large decrement was found in alcohol use disorder.
IED is an abstract and computerised analogue of the WCST where the participant has to switch between responding to pink shapes or white lines (Sahakian & Owen, 1992). The index of set-shifting ability is typically the extradimensional shift errors or total errors. IED performance has only been quantitatively reviewed in pathological gambling or gambling disorder (k = 3), with a small to medium effect being found.
TMT-B is the second part of a commonly used paper and pencil test measuring attentional switching ability (Franzen, Paul, & Price, 1990). In this task, participants are required to join numbers and letters in ascending and alphabetical orders respectively, in alternating sequences. The index of poor attentional switching ability is the time taken to complete TMT-B (or the time relative to the first part). TMT-B performance has been quantitatively pooled in alcohol use disorder (k = 34) and pathological gambling or gambling disorder (k = 4). A small effect size was detected in gambling, whereas a medium to large decrement was found in alcohol use disorder.
The CPT is a computerised task of cognitive flexibility, predicated on changes in response and outcome contingencies (Newman, Patterson, & Kosson, 1987). Participants are presented with a deck of cards comprised of a predetermined set of “face” and “number” cards. Each trial begins with the presentation of the back of a card and the participant is required to either place a bet on that card being a face card, or discontinue the game and collect earnings to date. The betting amount is stair-cased after each trial depending on whether the participant was correct and the card was indeed a face card (i.e., increased next bet) or incorrect (i.e., decreased next bet). As the task progresses, the mean probability of losing increases steadily from block to block (i.e., from 10% to 100%). The number of cards played before deciding to quit is thought to be a measure of contingency-related cognitive flexibility. CPT performance has only been meta-analysed in pathological gambling or gambling disorder (k = 3). A medium to large effect size was found.
Summary of Findings
The current meta-review was the first to systematically synthesize the neurocognitive literature examining impulsive-compulsive constructs across the addictive behavior spectrum. To summarise, alcohol dependence and gambling disorder were consistently associated with impulsivity and compulsivity deficits across all the reviewed neurocognitive tasks to date. Drug dependence and other domains of behavioral addictions, however, have been less frequently examined and meta-analyses have only been conducted on the impulsivity paradigms, with neurocognitive measures addressing compulsivity not having been quantitatively reviewed thus far in these disorders. Specifically, psychostimulant dependence (i.e., methamphetamine, cocaine, MDMA) appeared to be most commonly associated with inhibitory control deficits, whereas evidence linking impulsivity to cannabis dependence was consistently lacking. Tobacco use, by comparison, appeared to be associated with mild impulsivity. In terms of the role of impulsivity in other addictive behaviors, such as opiate or excessive internet use, it remains premature to interpret given the dearth of studies, as well as the inconsistent application of diagnostic criteria in the latter, likely owing to the relative infancy of the behavioral addiction research and practice. These findings depart somewhat from established thinking that impulsivity operates both as a vulnerability marker and the toxic effect of prolonged substance exposure (Verdejo-Garcia et al., 2008) across the full alcohol and drug spectrum, such that impulsivity appeared to be more relevant for some substances (e.g., alcohol) compared to others (e.g., cannabis). This highlights the advantages of a meta-review approach that is better equipped to compare the strength of findings across the sheer quantity of reviews to date.
Implications
The gestalt of the neurocognitive evidence at present suggests that the role of impulsivity and compulsivity in addictive behaviors is not homogeneous across conditions. Specifically, seemingly different addictive behaviors (e.g., alcohol and gambling) appear to have more in common with each other, than alcohol appears to have with cannabis use. Accordingly, the mechanisms underlying risk and maintenance of substance misuse need to be qualified by the specific substance in question. In particular, inhibitory control deficits may play only a minor or negligible role in chronic cannabis use, whereas it is an important vulnerability marker as well as a chronicity marker for alcohol use disorders, given that such deficits were found in both alcohol dependence and heavy drinkers, but more so in the former (with implications for clinical staging). Clinically, cognitive control interventions may be more indicated for some substance use disorders but not others.
The significant impact of impulsivity and compulsivity in behavioral addictions, specifically in problem gambling, is notable. This finding suggests that the deleterious, neurocognitive sequelae of prolonged substance exposure may not be sufficient to reinforce the maladaptive approach behaviors that characterise addictions. That is, problem gamblers demonstrate both inhibitory control and cognitive flexibility deficits, comparable in magnitude to that of individuals dependent on alcohol, in keeping with current thinking (Goudriaan, Oosterlaan, De Beurs, & Van Den Brink, 2006). As such, it is reasonable to propose that impulsivity and compulsivity contribute to driving addictive behaviors transdiagnostically, even though in some addictive behaviors (e.g. alcohol use), these functions may additionally be a consequence of substance toxicity. Accordingly, clinical interventions designed to specifically target the neurocognitive mechanisms of addictive behaviors are needed, independent of treatments targeting the behaviors themselves.
More broadly, it is difficult to provide specific directions regarding how to implement the current findings in clinical practice, since implementation studies are currently lacking. We believe the present study could advance the neuroscience-informed approach of diagnosis and treatment of addictive behaviors by promoting the imperative of measuring the core neuropsychological constructs of impulsivity and compulsivity (Franken & van de Wetering, 2015, Yucel et al., in press). The use of neuropsychological measures of impulsivity and compulsivity in clinical practise could be helpful in several ways. First, we could use these neuropsychological functions as a surrogate outcome for treatment progress, which are typically difficult to measure in substance use disorders (Perlis, 2011). For example, improvement of impulsivity can function as a proxy for treatment progress. Second, neuropsychological markers can contribute to the confirmation of a diagnosis (Perlis, 2011), in keeping with the RDoC approach (Cuthbert & Insel, 2013; Yucel et al., 2018). The third benefit would be that these markers could function as predictors of treatment outcome (Marhe & Franken, 2014; Marhe, Luijten, van de Wetering, Smits, & Franken, 2013; Perlis, 2011). Fourth, the profiling of neurocognition will facilitate more efficient and effective treatments for addictive behaviors by providing a starting point for more personalized forms of treatment (Perlis, 2011). For example, we could provide patients who exhibit inhibitory control deficits with specific forms of cognitive training designed to target behavioral disinhibition (Verdejo-Garcia, 2016; Wiers, 2018).
It is worth noting that other neurocognitive functions theoretically linked to impulsive-compulsive behaviors, such as incentive salience attribution, error processing, temporal discounting, habit learning, and reward prediction error, have not been systematically reviewed in any addiction population to date despite the key roles these functions play in relation to addictive behaviors (Franken & van de Wetering, 2015). This is not surprising given these paradigms are typically more laboratory-based, and there is a clear paucity of studies utilizing these tasks consistently within a single addiction population. The systematic evaluation of other neurocognitive functions evidently warrants more urgent, concerted research focus.
Methodological Considerations for Future Research
There are a few qualifications worth noting. It is clear from the current meta-review that the role of certain neurocognitive functions across addictive behaviors are varied and heterogeneous, particularly for compulsivity-related constructs, where heterogeneity analyses were consistently significant. Unfortunately, most meta-analyses did not specifically seek to disentangle the effects of methodological, clinical or demographic characteristics regarding the significant heterogeneity found across studies, for example, lower educational attainment or poorer socioeconomic status in clinical samples versus controls, different substance use severities or comorbid substance use. The meta-analyses that have employed meta-regression approaches have not been able to identify any significant relationships, suggesting that researchers have not been focusing on the right factors when trying to determine why the results may have varied systematically from study to study. Alternatively, it may have been premature to conduct a meta-regression given the small number of studies included in some pooled analyses (e.g. k = 3) and the effects of factors such as age and gender may emerge as important predictors when meta-analyses become better powered, statistically, with better designed studies. Moreover, infrequent consideration of risk of biases and methodological quality of included studies may have inflated the magnitude of effects identified in the current reviews. Methodologically, we were unable to disentangle with confidence the differential contribution of impulsive-compulsive functions to vulnerability and chronicity of addictions, which will require more prospective, longitudinal investigations using repeated panel designs. We also note that only six meta-analyses were included in the current meta-review and the findings stand to be corroborated as more meta-analytic evidence comes to light and may additionally be enhanced by insights from meta-analysis of patient-level data.
Notwithstanding these caveats, impulsivity and compulsivity appear to be core processes underlying addictive behaviors across the substance and behavioral spectrums. Further research is needed to examine compulsivity-related functions across addictive disorders and in the longitudinal trajectories and clinical outcomes of individuals with addictive behaviors. The current meta-review highlighted the need to consider how research evidence is synthesized within the field of addiction, given the majority of reviews to date have either been selective and narrative, or qualitative, in nature.
References
Anderson, S. W., Damasio, H., Jones, R. D., & Tranel, D. (1991). Wisconsin card sorting test performance as a measure of frontal lobe damage. Journal of Clinical and Experimental Neuropsychology, 13(6), 909–922.
Assadi, S. M., Yucel, M., & Pantelis, C. (2009). Dopamine modulates neural networks involved in effort-based decision-making. Neuroscience and Biobehavioral Reviews, 33(3), 383–393.
Bechara, A. (2005). Decision making, impulse control and loss of willpower to resist drugs: A neurocognitive perspective. Nature Neuroscience, 8(11), 1458–1463.
Bechara, A., Damasio, A. R., Damasio, H., & Anderson, S. W. (1994). Insensitivity to future consequences following damage to human prefrontal cortex. Cognition, 50(1-3), 7–15.
Broos, N., Schmaal, L., Wiskerke, J., Kostelijk, L., Lam, T., Stoop, N., … Goudriaan, A. E. (2012). The relationship between impulsive choice and impulsive action: A cross-species translational study. PLoS One, 7(5), e36781.
Burgess, P. W., & Shallice, T. (1996). Response suppression, initiation and strategy use following frontal lobe lesions. Neuropsychologia, 34(4), 263–272.
Carter, A., Hendrikse, J., Lee, N., Yücel, M., Verdejo-Garcia, A., Andrews, Z., & Hall, W. (2016). The neurobiology of “food addiction” and its implications for obesity treatment and policy. Annual Review of Nutrition, 36(1), 105–128.
Chamberlain, S. R., Fineberg, N. A., Blackwell, A. D., Robbins, T. W., & Sahakian, B. J. (2006). Motor inhibition and cognitive flexibility in obsessive-compulsive disorder and trichotillomania. The American Journal of Psychiatry, 163(7), 1282–1284.
Chamberlain, S. R., Stochl, J., Redden, S. A., & Grant, J. E. (2018). Latent traits of impulsivity and compulsivity: Toward dimensional psychiatry. Psychological Medicine, 48(5), 810–821.
Chesney, E., Goodwin, G. M., & Fazel, S. (2014). Risks of all-cause and suicide mortality in mental disorders: A meta-review. World Psychiatry, 13(2), 153–160.
Chowdhury, N. S., Livesey, E. J., Blaszczynski, A., & Harris, J. A. (2017). Pathological gambling and motor impulsivity: A systematic review with meta-analysis. Journal of Gambling Studies, 33(4), 1213–1239.
Comalli, P. E., Wapner, S., & Werner, H. (1962). Interference effects of Stroop color-word test in childhood, adulthood, and aging. The Journal of Genetic Psychology, 100(1), 47–53.
Cuthbert, B. N. (2014). The RDoC framework: Facilitating transition from ICD/DSM to dimensional approaches that integrate neuroscience and psychopathology. World Psychiatry, 13(1), 28–35.
Cuthbert, B. N., & Insel, T. R. (2013). Toward the future of psychiatric diagnosis: The seven pillars of RDoC. BMC Medicine, 11(1), 126.
Dalley, J. W., Everitt, B. J., & Robbins, T. W. (2011). Impulsivity, compulsivity, and top-down cognitive control. Neuron, 69(4), 680–694.
Dalley, J. W., & Robbins, T. W. (2017). Fractionating impulsivity: Neuropsychiatric implications. Nature Reviews. Neuroscience, 18(3), 158–171.
Daruna, J. H., & Barnes, P. A. (1993). A neurodevelopmental view of impulsivity. In The impulsive client: Theory, research, and treatment (pp. 23–37). Washington, DC, US: American Psychological Association.
de Wit, H. (2009). Impulsivity as a determinant and consequence of drug use: A review of underlying processes. Addiction Biology, 14(1), 22–31.
Denys, D. (2011). Obsessionality & compulsivity: A phenomenology of obsessive-compulsive disorder. Philosophy, Ethics, and Humanities in Medicine : PEHM, 6(1), 3–3.
Eagle, D. M., & Robbins, T. W. (2003). Inhibitory control in rats performing a stop-signal reaction-time task: Effects of lesions of the medial striatum and d-amphetamine. Behavioral Neuroscience, 117(6), 1302–1317.
Fineberg, N. A., Chamberlain, S. R., Goudriaan, A. E., Stein, D. J., Vanderschuren, L. J., Gillan, C. M., … Potenza, M. N. (2014). New developments in human neurocognition: Clinical, genetic, and brain imaging correlates of impulsivity and compulsivity. CNS Spectrums, 19(01), 69–89.
Francke, A. L., Smit, M. C., de Veer, A. J., & Mistiaen, P. (2008). Factors influencing the implementation of clinical guidelines for health care professionals: A systematic meta-review. BMC Medical Informatics and Decision Making, 8(1), 38.
Franken, I. H. A., & van de Wetering, B. J. (2015). Bridging the gap between the neurocognitive lab and the addiction clinic. Addictive Behaviors, 44, 108–114.
Franzen, M. D., Paul, D. S. & Price, G. (1990). Alternate form reliability of Trails A, B, C, and D. In Ninth Annual Convention of the National Academy of Neuropsychology: Reno, NY.
Gomez, P., Ratcliff, R., & Perea, M. (2007). A model of the go/no-go task. Journal of Experimental Psychology. General, 136(3), 389–413.
Goudriaan, A. E., Oosterlaan, J., De Beurs, E., & Van Den Brink, W. (2006). Neurocognitive functions in pathological gambling: A comparison with alcohol dependence, Tourette syndrome and normal controls. Addiction, 101(4), 534–547.
Hester, R., Lubman, D. I., & Yücel, M. (2010). The role of executive control in human drug addiction. In D. W. Self & J. K. Staley Gottschalk (Eds.), Behavioral neuroscience of drug addiction (pp. 301–318). Berlin, Heidelberg: Springer Berlin Heidelberg.
Koob, G. F., & Le Moal, M. (2001). Drug addiction, dysregulation of reward, and allostasis. Neuropsychopharmacology, 24(2), 97–129.
Kovacs, I., Richman, M. J., Janka, Z., Maraz, A., & Ando, B. (2017). Decision making measured by the Iowa gambling task in alcohol use disorder and gambling disorder: A systematic review and meta-analysis. Drug and Alcohol Dependence, 181, 152–161.
Lee, R. S. C., Dore, G., Juckes, L., De Regt, T., Naismith, S. L., Lagopoulos, J., … Hermens, D. F. (2015). Cognitive dysfunction and functional disability in alcohol dependent adults with or without a comorbid affective disorder. Cognitive Neuropsychiatry, 20(3), 222–231.
Lee, R. S. C., Hermens, D. F., Scott, J., Redoblado-Hodge, M. A., Naismith, S. L., Lagopoulos, J., … Hickie, I. B. (2014). A meta-analysis of neuropsychological functioning in first-episode bipolar disorders. Journal of Psychiatric Research, 57, 1–11.
Lezak, M. D., Howieson, D. B., Bigler, E. D., & Tranel, D. (2012). Neuropsychological assessment. New York: Oxford University Press.
Lipszyc, J., & Schachar, R. (2010). Inhibitory control and psychopathology: A meta-analysis of studies using the stop signal task. Journal of the International Neuropsychological Society, 16(06), 1064–1076.
Lubman, D. I., Cheetham, A., & Yücel, M. (2015). Cannabis and adolescent brain development. Pharmacology & Therapeutics, 148, 1–16.
Lubman, D. I., Yücel, M., & Pantelis, C. (2004). Addiction, a condition of compulsive behaviour? Neuroimaging and neuropsychological evidence of inhibitory dysregulation. Addiction, 99(12), 1491–1502.
Marhe, R., & Franken, I. (2014). Error-related brain activity as a biomarker for cocaine relapse. Neuropsychopharmacology Reviews, 39(1), 241.
Marhe, R., Luijten, M., van de Wetering, B. J., Smits, M., & Franken, I. H. A. (2013). Individual differences in anterior cingulate activation associated with attentional bias predict cocaine use after treatment. Neuropsychopharmacology, 38(6), 1085–1093.
Newman, J. P., Patterson, C. M., & Kosson, D. S. (1987). Response perseveration in psychopaths. Journal of Abnormal Psychology, 96(2), 145–148.
O'Brien, C. (2011). Addiction and dependence in DSM-V. Addiction, 106(5), 866–867.
Perlis, R. H. (2011). Translating biomarkers to clinical practice. Molecular Psychiatry, 16(11), 1076–1087.
Prochazkova, L., Parkes, L., Dawson, A., Youssef, G., Ferreira, G. M., Lorenzetti, V., … Yücel, M. (2018). Unpacking the role of self-reported compulsivity and impulsivity in obsessive-compulsive disorder. CNS Spectrums, 23(1), 51–58.
Robbins, T. W., Gillan, C. M., Smith, D. G., de Wit, S., & Ersche, K. D. (2012). Neurocognitive endophenotypes of impulsivity and compulsivity: Towards dimensional psychiatry. Trends in Cognitive Sciences, 16(1), 81–91.
Sahakian, B. J., & Owen, A. M. (1992). Computerized assessment in neuropsychiatry using CANTAB: Discussion paper. Journal of the Royal Society of Medicine, 85, 399–402.
Smith, J. L., Mattick, R. P., Jamadar, S. D., & Iredale, J. M. (2014). Deficits in behavioural inhibition in substance abuse and addiction: A meta-analysis. Drug and Alcohol Dependence, 145, 1–33.
Stavro, K., Pelletier, J., & Potvin, S. (2013). Widespread and sustained cognitive deficits in alcoholism: A meta-analysis. Addiction Biology, 18(2), 203–213.
Stephan, R. A., Alhassoon, O. M., Allen, K. E., Wollman, S. C., Hall, M., Thomas, W. J., … Grant, I. (2017). Meta-analyses of clinical neuropsychological tests of executive dysfunction and impulsivity in alcohol use disorder. The American Journal of Drug and Alcohol Abuse, 43(1), 24–43.
Sussman, S., & Sussman, A. N. (2011). Considering the definition of addiction. International Journal of Environmental Research and Public Health, 8(10), 4025–4038.
Torregrossa, M. M., Xie, M., & Taylor, J. R. (2012). Chronic corticosterone exposure during adolescence reduces impulsive action but increases impulsive choice and sensitivity to yohimbine in male Sprague-Dawley rats. Neuropsychopharmacology, 37(7), 1656–1670.
van Timmeren, T., Daams, J. G., van Holst, R. J., & Goudriaan, A. E. (2018). Compulsivity-related neurocognitive performance deficits in gambling disorder: A systematic review and meta-analysis. Neuroscience and Biobehavioral Reviews, 84, 204–217.
Verdejo-Garcia, A. (2016). Cognitive training for substance use disorders: Neuroscientific mechanisms. Neuroscience and Biobehavioral Reviews, 68, 270–281.
Verdejo-Garcia, A., Lawrence, A. J., & Clark, L. (2008). Impulsivity as a vulnerability marker for substance-use disorders: Review of findings from high-risk research, problem gamblers and genetic association studies. Neuroscience and Biobehavioral Reviews, 32(4), 777–810.
Verdejo-Garcia, A., Lubman, D. I., Schwerk, A., Roffel, K., Vilar-Lopez, R., Mackenzie, T., & Yucel, M. (2012). Effect of craving induction on inhibitory control in opiate dependence. Psychopharmacology, 219(2), 519–526.
Whelan, R., Watts, R., Orr, C. A., Althoff, R. R., Artiges, E., Banaschewski, T., … Garavan, H. (2014). Neuropsychosocial profiles of current and future adolescent alcohol misusers. Nature, 512(7513), 185–189.
Wiers, R. W. (2018). Cognitive training in addiction: Does it have clinical potential? Biological Psychiatry: Cognitive Neuroscience and Neuroimaging, 3, 101–102.
Yücel, M., & Fontenelle, L. F. (2012). Compulsivity as an endophenotype: The search for a hazy moving target. Addiction, 107(10), 1735–1736.
Yucel, M., Fornito, A., Youssef, G., Dwyer, D., Whittle, S., Wood, S. J., … Allen, N. B. (2012). Inhibitory control in young adolescents: The role of sex, intelligence, and temperament. Neuropsychology, 26, 347–356.
Yucel, M., Oldenhof, E., Ahmed, S. H., Belin, D., Billieux, J., Bowden-Jones, H., Carter, A., Chamberlain, S. R., Clark, L., Connor, J., Daglish, M., Dom, G., Dannon, P., Duka, T., Fernandez-Serrano, M. J., Field, M., Franken, I., Goldstein, R. Z., Gonzalez, R., Goudriaan, A. E., Grant, J. E., Gullo, M. J., Hester, R., Hodgins, D. C., Le Foll, B., Lee, R. S. C., Lingford-Hughes, A., Lorenzetti, V., Moeller, S. J., Munafo, M. R., Odlaug, B., Potenza, M. N., Segrave, R., Sjoerds, Z., Solowij, N., van den Brink, W., van Holst, R. J., Voon, V., Wiers, R., Fontenelle, L. F. & Verdejo-Garcia, A. (2018). A transdiagnostic dimensional approach towards a neuropsychological assessment for addiction: an international Delphi consensus study. Addiction. https://doi.org/10.1111/add.14424.
Zalesky, A., Solowij, N., Yucel, M., Lubman, D. I., Takagi, M., Harding, I. H., … Seal, M. (2012). Effect of long-term cannabis use on axonal fibre connectivity. Brain, 135(7), 2245–2255.
Author information
Authors and Affiliations
Corresponding author
Additional information
Publisher’s Note
Springer Nature remains neutral with regard to jurisdictional claims in published maps and institutional affiliations.
Rights and permissions
About this article
Cite this article
Lee, R.S.C., Hoppenbrouwers, S. & Franken, I. A Systematic Meta-Review of Impulsivity and Compulsivity in Addictive Behaviors. Neuropsychol Rev 29, 14–26 (2019). https://doi.org/10.1007/s11065-019-09402-x
Received:
Accepted:
Published:
Issue Date:
DOI: https://doi.org/10.1007/s11065-019-09402-x