Abstract
Freshwater has a significant role in determining the ecological environment, public health and socio-economic development. This study analyzed the spatio-temporal variation in surface water quality of the tropical reservoir Mettur, India, to determine whether its water is suitable for human consumption and aquatic ecosystem. Thus, 21 water quality parameters were monitored seasonally (pre-monsoon, monsoon and post-monsoon) at nine sampling sites covering riverine, transitional and lacustrine zones of the reservoir during 2018. The study revealed spatial variation, which was due to input from industrial and domestic wastewater in the lacustrine zone of the reservoir. However, temporal variability often exceeded spatial variability. The factor analysis indicates that basin geology, anthropogenic stress and agricultural applications simultaneously act vital roles in deciding the reservoir water quality. Based on similarity of water quality parameters, cluster analysis grouped all the nine sampling sites into three clusters of high, moderate and less polluted sites. Correlation analysis also indicated that multiple sources influence the reservoir water quality during all the seasons. Based on BIS and WHO values, the calculated water quality index (134–158) indicates that water of the reservoir is not fit for human consumption, while the trophic state of this reservoir is moderately-eutrophic to eutrophic. However, with precaution, this aquatic body seems to be suitable for aquatic life and fish growth. The overall water quality deterioration of this reservoir indicates a strong need for development of a regular monitoring system for implementation of an effective management system to safeguard human and environmental health.
Similar content being viewed by others
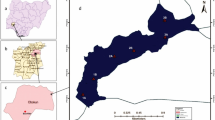
Explore related subjects
Discover the latest articles, news and stories from top researchers in related subjects.Avoid common mistakes on your manuscript.
Introduction
Reservoirs are important sources of freshwater ecosystems with immense economic and ecological value (Mishra et al. 2019). India is bestowed with huge reservoir resources to the tune of 3.42 million ha (19,386 numbers) (Sarkar et al. 2018). Over the last few decades, there has been tremendous progress in India for multi-purpose exploitation of reservoir resources including fisheries development. However, anthropogenic activities like sewage discharge, agricultural runoff and land use pattern cause quality deterioration of freshwater resources all over the world (Teshome 2020; Varol 2020). This results in constant reduction in availability of quality water for drinking purposes, and for other uses like agriculture, fisheries and domestic consumption (Teshome 2020). Whereas the quality of water in terms of drinking water and aquatic life is gaining importance, the need of water quality evaluation is also getting momentum (Ouyang 2005). For development of sustainable water management strategies, it is important to have adequate information about pollution sources that deteriorate water quality. Quality evaluation of freshwater in terms of physical, chemical or biological may help in projecting its best uses and thus help in imposing various restrictions intended to check further quality deterioration (Tibebe et al. 2019). Quality evaluation of water also laid the foundation for its management as by correct characterization of pollution sources. By checking the compliance of water quality parameters as per national and international guidelines, we can develop a reliable monitoring system if required.
The Mettur reservoir (also known as Stanley reservoir), located at Mettur town of Salem district, Tamil Nadu, India, was constructed in 1934 across a gorge where the Cauvery River enters into the plains of South India. This large reservoir was constructed with intention to use for irrigation, power and drinking purposes. Later on, it has been harnessed for its fisheries potential also. However, with passage of time, industrial development led to an increase in pollution of this reservoir water (Anbazhagan 2018), which is of concern. Besides three heavy industry viz. Chemplast, MALCO (Madras Aluminium Company) and Mettur Thermal Power Plant, several small-scale chemical industries are also located on Cauvery River bank at Mettur town (Anbazhagan 2018). The industrial effluents from various industries in and around Mettur town contain pollutant substances, and once they enter into the reservoir, the water quality is affected. Agricultural activities, particularly vegetable cultivation on the banks of this reservoir during summer season when the reservoir shrinks, also form a source of pollutants in the forms of chemical fertilizer and pesticides. Paddy cultivation on the Cauvery delta of Tamil Nadu is totally dependent on Mettur reservoir water and consequently agricultural runoff from this paddy field is another source of contamination.
Although numerous reports on surface water quality of the Mettur reservoir are available, most of the reports are parts of surveys of the Cauvery River (Hema and Subramani 2013; Palaniswami et al. 2015; Sivakumar et al. 2017). All the previous studies for this reservoir were either limited in time or space, or restricted to one season without any spatial data, which are also important (Korkanç et al. 2017; Tibebe et al. 2019). A comprehensive information on the spatio-temporal trend of the Mettur reservoir water quality parameters is not available and this puts a roadblock to the understanding of possible impacts on its water quality and the reason of growing pollution, which leads to potential risks to aquatic life and human health. In addition, for characterization of the aquatic ecosystem, appraisal of spatio-temporal differences of physico-chemical water quality indicators is very much important (Varol 2020) to identify the roots of water quality degradation. Assessing the temporal variations helps in characterizing the seasonal alternation of water quality and thus helps in finding the natural or anthropogenic sources of pollution (Ouyang et al. 2006). Temporal variations in precipitation, surface runoff, interflow, groundwater flow and outflows significantly affect pollution discharge and their concentration (Varol 2020). However, spatial distribution helps in finding the point sources of pollution (Tibebe et al. 2019). The Department of Environment, Govt. of Tamil Nadu, (Anonymous 2005) reported that, from Salem district, about 640 lakh liters of waste water per day are discharged into the Cauvery River. Heavy discharge, agricultural inputs may increase the productivity of a lake or a reservoir, leading to reservoir eutrophication (Varol 2020). Therefore, determination of trophic state of lakes and reservoirs is also important.
Assessment of water quality is associated usually with determination of a large set of physico-chemical and biological parameters and comparing them with threshold values. Although this process is helpful in identifying pollution source, this has little value with respect to the overall water quality status of an aquatic body (Hamlat et al. 2013). Several innovative approaches have evolved to overcome these drawbacks; particularly application of multivariate statistical analysis and evaluation of water quality index (WQI). The latter is a useful way to represent the overall state of water, which will be helpful to end-users and policy-makers (Brown et al. 1972). The Mettur reservoir provides irrigation and drinking water facilities for more than 12 districts of Tamil Nadu and, hence, is revered as the life and livelihood-giving asset of Tamil Nadu. Several previous studies on the Mettur reservoir are mainly focused on, among others, irrigation capacity, water balance and quantity (AlMohseen 2003; Mohan and Ramsundram 2016). However, evaluation of WQI has never been a priority, but mere evaluation of quality parameters, without giving importance by taking consideration of end-users, policy-makers or concerned bodies, is of no practical utility. Additionally, multivariate statistical analysis like principal component analysis / factor analysis (PCA/FA) and cluster analysis (CA) is useful techniques for pollution source identification and, thus, overall quality of water, which helps in management of water bodies (Fan et al. 2010). PCA/FA helps in identifying the main factors controlling the overall quality of water (Ouyang 2005), whereas CA defines natural clustering patterns by making use of similarity in quality parameters (Azhar et al. 2015). The main aim of all these techniques is to assist in identifying the common sources, establish the processes and relations between the variables used for evaluating the water quality, and disclose the basic parameters controlling the overall quality of water.
Studies have not been carried out in the tune of suitability analysis of the Mettur reservoir water with reference to human consumption and fisheries activities. Although this reservoir water is used by a large community, no suitability and safety studies have focused on the use of this reservoir water. Thus, the present study investigated the Mettur reservoir water quality with the aims (1) to appraise the spatio-temporal differences of water quality parameters and use of multivariate analytical technique to identify pollution sources, (2) to assess the trophic state of the reservoir, and (3) to determine the suitability of the reservoir water according to designated use and computation of WQI, which can be used effectively for pollution abatement and for strengthening the management policy for this reservoir. The results of the present investigation will facilitate a decision support tool for aquatic resources manager toward (a) development of effective management module, (b) identification of the most vulnerable areas of a water body, and (c) identification of latent source of water quality deterioration, like uncontrolled effluents from domestic, municipal, industrial and agricultural runoff. Possible policy implications might be the implementation of strict provisions on those who fail to comply with sanitation regulations and standards. Eventually, this investigation will lead to a development of effective management practices to conserve this aquatic resource.
Geography and Geology of the Study Area
The Mettur reservoir is located at Salem district, Tamil Nadu, India, at an altitude of 243 m above mean sea level, with maximum height of 70.4 m and has a length of 1700 m. The maximum capacity of this reservoir is 93.47 tmc ft.Footnote 1* The area of the reservoir is 42.5 km2. The average temperature in Mettur is 27.9 °C, where April is the warmest month (avg. 31.0 °C) and December is the coldest month (avg. 24.8 °C). Precipitation is mainly concentrated during the south-west monsoon (June–September) averaging 181 mm (https://en.climate-data.org/asia/india/tamil-nadu/mettur-24058/). The main source of water for the Mettur reservoir is the Cauvery River, which is also a drinking water source for Salem and 12 other districts in Tamil Nadu. It is one of largest fishing reservoirs in India and of about 5000 fishermen’s livelihood is completely dependent on it.
Geological information of adjoining areas including the Mettur reservoir has been given in various previous work (Jain et al. 2007; Ravikumar et al. 2011; Srinivasamoorthy et al. 2011a, b, 2012; Subramani et al. 2017). The area is located along a fault zone and consists of metamorphosed rocks. The lithology of the entire region is comprised mainly of charnockites with peninsular gneiss and calc gneiss. Little amount of basic granulites and quartzite are also present (Srinivasamoorthy et al. 2011b). Subramani et al. (2017) reported that this region has passed through five phases of deformation, five generations of basic dyke activities and four phases or migmatization and two periods of metallogeny.
Materials and Methods
Site Selection and In situ Sampling for Analysis
For understanding the physical and uniqueness of the reservoir, the Mettur reservoir was divided into three different zones (Fig. 1): (1) riverine zone, the entry point (inflow point) of river into the reservoir where water depth is comparatively lower with aggregation of aquatic weeds and macrophytes; (2) transition zone, largest area between upstream and downstream of reservoir; and (3) lacustrine zone located across the dam of the reservoir with higher depth and sharp slope. Based on the elevation and flow of water in the reservoir, its accessibility, safety, latent pollution sources and sewage disposal activities, the sampling sites in each zone were evenly distributed and water samples were collected from a total of nine sites in the riverine (2 sites), transition (4 sites), and lacustrine (3 sites) zone along the course of reservoir. The whole study period was divided into three different seasons, i.e., pre-monsoon (PRM) (February–May), monsoon (MON) (June–September) and post-monsoon (POM) (October–January) seasons of the year 2018. Sub-surface (0.3 m) water samples were collected through a water sampler and stored immediately in an ice box before transported to laboratory (Fig. 2).
Twenty-one different physico-chemical water quality parameters were measured for quality evaluation of the Mettur reservoir water. Water temperature (WT), pH, electrical conductivity (EC), turbidity (turb), dissolved oxygen (DO) and redox potential (ORP) were measured in situ by a portable multi-parameter water quality instrument (YSI Professional Plus). Transparency (SD) was measured by using the Secchi disk method. TDS (total dissolved solids) were determined gravimetrically, whereas BOD (biochemical oxygen demand) was evaluated by 5-day incubation (20 °C) method. Chlorophyll (Chl-a) was extracted with 90% acetone and analyzed by spectrophotometry. Total alkalinity (TA), total hardness (TH), calcium (Ca–H) and magnesium (Mg–H) hardness and chloride (Cl−) were determined by titration method. In filtered water samples, nutrients like nitrite-N (NO2−–N) and nitrate–N (NO3−–N), phosphate–P (PO43−) and total P (TP), and silicate (SiO2) were analyzed by UV–Vis spectrophotometry. All the protocols for sampling, storage/preservation and analysis were carried out as per the APHA (2005) guidelines.
Trophic Assessment
The trophic state index (TSI) of Carlson (1977) as modified by Lamparelli (2004) for tropical lake/reservoir and wetland was used for determining the trophic state of the Mettur reservoir. These modified equations are:
where TSI (TP), TSI (Chl-a) and TSI (SD) denote the trophic state corresponding to total phosphorus (µg/l), chlorophyll-a (µg/l) content and Secchi disk depth (SD), respectively. TSI (Avg) is average of all three TSI values. Based on the calculated TSI values, tropical wetland/reservoir can be categorized as follows (Lamparelli 2004; Das Sarkar et al. 2019): ultraoligotrophic (< 24), oligotrophic (24 to ≤ 44), mesotrophic (44 to ≤ 54), eutrophic (54 to ≤ 74) and hypereutrophic (> 74).
Computation of Water Quality Index
The weighted arithmetic index method as implemented by many researchers (e.g., Brown et al. 1972; Kumari and Sharma 2019; Saha et al. 2020; Matta et al. 2020a, b) was used for computation of WQI of the Mettur reservoir by considering standard limits as prescribed by BIS (2012) and WHO (2011) (Table 6). WQI was calculated step-by-step by using the following equations.
Step 1
Eleven important parameters, which significantly control water quality (Table 6), were taken in consideration to calculate WQI. The following equation was used:
where qn indicates the quality rating, Vn and Sn are observed and standard prescribed limits, respectively, for the nth water quality parameter, whereas Vi is ideal value that is theoretically 0 for all quality parameters except pH and DO, for which 7.0 and 14.6, respectively, were used as ideal values for WQI calculation.
Step 2
In this step, the unit weight (Wn) of each quality parameter was calculated by a value inversely proportional to the recommended standard value Sn of that parameter. Thus:
where proportionality constant k was calculated as:
Step 3
Finally, WQI was calculated for every water quality parameter by dividing the product of quality rating and unit weight by the summation of all the unit weights, thus:
Statistical Methods
Descriptive statistics were used to determine the overall finding of the investigation and shown in Table 1. Spatial and temporal significant differences were determined by using one-way ANOVA, and means were compared by using Dun-can’s Multiple Range Test (DMRT). Boxplot analysis was carried out for representing the seasonal trend. In this study, Pearson’s correlation was applied to determine the relationship among the different physico-chemical parameters of the Mettur reservoir water samples at different sampling sites in all seasons. Statistical significance was considered at p < 0.05. A correlation matrix was constructed by using mean values of all water quality parameters at different sampling sites over the whole studied period (Belkhiri et al. 2011). To determine the spatial similarity/dissimilarity based on the water quality parameters of the Mettur reservoir, cluster analysis (CA) was performed by using squared Euclidean and Ward’s method as a distance matrix and linkage methods, respectively. Principal component analysis/factor analysis (PCA/FA) was applied for potential pollution source identification. SPSS 20, PAST 3 and the DSAASTAT software packages were used for the data analysis.
Results and Discussion
Mean spatial–temporal values of different physico-chemical parameters are given in Tables 2 and 4 for spatial and seasonal variations, respectively. ANOVA was also performed between various study sites and showed significant correlations with each other (Table 3). In addition, boxplots were constructed (Fig. 4) to represent the temporal variations more accurately.
Discharge Hydrograph of the Mettur Reservoir
The discharge hydrograph of the Mettur reservoir (Fig. 3) represents the variation in daily average water flow into the reservoir in different months for the period of 2018–2020 (http://www.metturdiary.com/p/mettur-dam-water-level-history.html). The maximum flow was observed during July–September because the maximum rainfall occurs during monsoon. Discharge was very low from January to June, which may be due to the summer. However, discharge was medium during October–December, which may be due to north-eastern monsoon shower. Figure 3 also indicates a huge difference between the minimum and maximum discharges, which may be due to seasonal variation and erratic rainfall events. Rainfall and maximum water levels are the important factors that influence the physico-chemical parameters of water.
Detailed Limnochemistry of the Mettur Reservoir
Depth and Secchi Disk Transparency (SD)
The Mettur reservoir depth ranged between 3 and 34 m (avg. 13.77 ± 7.74 m) (Table 1). The depth varied significantly across the sampling sites (Table 2). The riverine zone was the shallowest. Sampling points in the lacustrine zone were the deepest, as water discharged from the river is deposited into this zone. Season also had profound influence on depth. Precipitation during MON and little north-eastern monsoon shower in POM resulted in higher water depth.
The reflection of light from the Secchi disk surface represents transparency. It is a proxy for suspended (dissolved and particulate matter) material present in water, and it is the simplest index for determining the euphotic zone of a reservoir (Wetzel 2001). In whole, reservoir transparency values ranged from 0.34 to 1.6 m with average of 0.81 ± 0.38 m (Table 1). Although the sites were statistically similar with respect to transparency, the lacustrine zone had the highest transparency, which may be due to its shallower depth; and the opposite was true for the riverine zone. Barik et al. (2017) also showed a positive relation between depth and transparency in the Chilika lagoon of India. However, transparency showed a temporal variation, with lowest value in PRM and highest in POM (Fig. 4). Similar temporal trends were also reported for transparency in the Bhindawas (Saluja and Garg 2017) and Akkulam–Veli (Sheela et al. 2011) lakes in India. According to OECD (1982), the Secchi disk depth range of 0.7–1.5 m signifies eutrophication and thus, the data suggest that the Mettur reservoir is on the verge of eutrophication.
Water Temperature
Temperature has profound influence on DO and BOD and thus has direct effects on growth of aquatic life and their biological activity. Temperature at all sites was statistically at par with average values ranging from 27.43 to 28.23 °C (Table 2). The boxplots (Fig. 4) of temperature showed similar patterns for MON and POM but significantly different for PRM, indicating strong influence of seasons on temperature. Seasonal averages were 29.8, 26.1 and 27.6 °C in PRM, MON and POM (Table 4), respectively, which are sufficient to sustain aquatic life except cold water fishes.
Dissolved Oxygen
Dissolved oxygen (DO) values were spatially significant, and the maximum value was found at site 8 (10.56 mg/l) while the minimum at site 1 (5.68 mg/l) (Table 2). The spatial pattern of DO indicates that the inflow point of the reservoir had maximum DO and it decreased toward the transitional zone and decreased again in the lacustrine zone. Sandhya et al. (2019) also found a similar trend of high to low values of DO at riverine and lacustrine zones of the Panchet reservoir, India which led to high diversity of small indigenous fishes in the riverine zone. The Mettur Sewage Treatment Plant and the Mettur Thermal Power Plant discharge outlets are situated in the lacustrine zone, which may have been contributing to low DO values in this zone (TNPCB 2019).
The mean DO values were found to be high in POM and low in MON (Table 4). The DO content of water is highly dependent on salinity, temperature and pressure (Poudel et al. 2013). Low water temperature during POM resulted in high oxygen solubility and thus high DO value (Korkanç et al. 2017). High planktonic growth in POM may be another reason. The long whiskers in the boxplot of DO in PRM (Fig. 4) indicate a skewed distribution toward higher values implying unstable DO conditions during PRM. The uniform boxplot of DO in POM indicates stable reservoir condition when it transits from wet to dry season, and the same was true for MON season also.
Electrical Conductivity and Total Dissolved Solids
Spatially, EC values ranged from 303.27 to 378.13 µS/cm (Table 2) and the values were non-significant across the sites. EC values were found to be higher in the sampling sites situated near the lacustrine zone as compared to all other sites. Wastewater inflows to the lacustrine zone may have contributed to high EC values. Zdanowski (1988) also reported high EC values in Konin Lake (Poland) due to discharge from a power plant, which also affected the habitat condition and trophic diversity. Gaur (1998) also reported similar observations. EC values at sites situated in the riverine zone were comparatively lower than at other sites due to dilution effect. While the sites near the transitional zones showed intermediated values for EC.
Mean EC values were maximum in PRM (482.8 µS/cm) but minimum in MON (183.6 µS/cm) (Table 4). In PRM, water flow rate is reduced and pollution from different (point and non-point) sources get accumulated and concentrated, which resulted in high EC value. The opposite is true during MON as flow rate increases, the concentration gets reduced and the reservoir water gets diluted with rain water. The trend was almost similar for TDS (Tables 2 and 4). In addition, the boxplots of EC and TDS in PRM and POM showed the smallest boxes (Fig. 4), and skewed toward the higher values indicating pollution effect. However, in MON, dilution due to precipitation resulted in a uniform box for EC. The EC values are comparatively higher than in the Krishna Raja Sagar Dam, Karnataka, India, which was constructed along the same Cauvery River but at higher altitude (Gholami and Srikantaswamy 2009). Water input and the extent of evaporation may be the reason for these differences.
Redox Potential
ORP, in association with different ions and compounds, determines the anoxic environment of water bodies. Spatially, ORP variation was not similar to DO variation. ORP had higher values at lacustrine zone where DO was lowest (Table 2). While OPR values were lowest at riverine zone, where DO was highest (Table 2). Spatially, non-significant variation was observed for ORP. However, seasonally, ORP showed a similar trend as DO as shown by the boxplots (Fig. 4). ORP values were maximum during PRM and lowest during MON (Table 4). The seasonal variation of ORP can interfere with water quality for domestic supply because the availability of iron and the solubility of other metals are enhanced/decreased according to changes in ORP (Guarino et al. 2005).
pH
pH significantly influences the activities of aquatic micro-flora and micro-fauna. CO2, CO32− and HCO−3 are the principal ions regulating the water pH. Across the sites, mean pH values varied from 8.89 to 9.14 with highest value at site 5 (Table 2). However, spatially, the pH values were not significant. Seasonal evaluation indicated temporal variation with highest pH in POM (9.8) and lowest in MON (8.3) (Table 4, Fig. 4). Comparatively higher planktonic growth in POM may have utilized CO2 and thus equilibrium is shifted toward the alkaline condition (Nazneen et al. 2019). In the absence of pollution, pH of water bodies is generally neutral to slightly alkaline in nature (Jonnalagadda and Mhere 2001). Ground study indicated that high pH in the Mettur reservoir may be due to heavy discharge of municipal and industrial waste as this reservoir is situated in a highly industrialized area.
Biological Oxygen Demand
With reference to BOD values, maximum (4.42 mg/l) value was recorded at site 9 while minimum (2.85 mg/l) at site 1 (Table 2). Considering the sampling sites, BOD values varied non-significantly. Compared to the lacustrine and riverine zones, the transitional zone showed lower BOD values. Discharges of municipal and industrial wastewater resulted in higher BOD values in the lacustrine zone. BOD values at the entry point of the reservoir, i.e., riverine zone, were also high compared to other sites. Besides, not displaying any significant seasonal differences, highest BOD values were recorded in MON, while POM recorded lowest values (Table 4).
BOD is widely used to indicate organic strength of water. It indicates the concentration of biodegradable organic matter in the water. Usually, BOD is measured for wastewaters and heavily polluted watercourses, because these types of water usually contain high concentrations of organic matter. According to Adakole (2000), surface water can be categorized as unpolluted, moderately polluted and heavily polluted when BOD values are < 1 mg/l, 2–9 mg/l and > 10 mg/l, respectively, and thus the Mettur reservoir was moderately polluted.
Total Alkalinity
Water alkalinity is mainly due the presence of carbonate and bicarbonate ions along with the presence of hydroxide ions. Recorded TA values were higher in POM followed by PRM (Table 4, Fig. 4). Low TA values in MON in all cases are attributed to dilution by rainwater. The seasonal mean values of TA were 99.7, 33.2 and 118.0 mg/l in PRM, MON and POM, respectively. Spatial differences for TA values were not significant (Table 2).
As alkalinity of a solution is a measure of its capacity to neutralize the acid, the higher the pH, higher the basic concentration and its alkalinity are. TA values in this study were consistent with pH, which also showed higher values in POM. Yelavarthy (2002) has studied the hydrobiology of Red Hills reservoir, Tamil Nadu, India, and noticed TA values in the range from 117.3 to 173.3 mg/l and showed close correlation with productivity.
Total Hardness and Calcium and Magnesium Hardness
Water hardness is due to the presence of Ca and Mg. Hardness can be temporary due to carbonates and bicarbonates or it can be permanent due to sulfates and chlorides. Biologically, temporary hardness plays a key role in buffering capacity, thus neutralizing pH due to addition of acidic products. It has a great effect on biotic diversity of an ecosystem. Here, site-wise, there were no significant differences with respect to TH. However, seasonal variation was significant and the minimum TH value was recorded in MON (53.8 mg/l), while the maximum value was recorded in POM (124.2 mg/l) (Table 4).
Ca and Mg are the two most common elements that make water hard (Toma et al. 2018). Sewage is the main source of increased TH levels in the study area. The Ca content in the reservoir increased from PRM to POM in the range of 9.1–27.0 mg/l, whereas the Mg content decreased from PRM (17.1 mg/l) to POM (14.2 mg/l), and became lowest in MON (6.7 mg/l) (Table 4, Fig. 4). Across the sites, there was no difference among the Ca and Mg values.
Chloride
Chloride (Cl−) value increases as mineral content increases, and it is found in water in association with Ca, Mg or Na. Field observation revealed that, across the sampling sites, Cl− values ranged from 23.54 to 29 mg/l without any significant differences (Table 2). Cl− in water arises due to mixing of waste water with natural water. Recorded Cl− values were far lower than in the Krishna Raja Sagar Dam, India, (Gholami and Srikantaswamy 2009), comparable with the shallow wetland in Kashmir Himalaya, India (Shah et al. 2017), but far higher than in the Pandoh lake, a protected water body in Lesser Himalaya, India (Anshumali and Ramanathan 2007). The seasonal average values of Cl− were maximum in PRM (Table 4, Fig. 4). Waste water discharge and high evaporation lead to high and concentrated values of Cl− (Kurunc et al. 2017) in PRM.
Nitrite and Nitrate–N
Nitrite-N (NO2–) was always below the detection limit. Due to unstable in nature, nitrite-N gets converted easily to nitrate-N (NO3–) and thus, it remains for a few times in the environment. NO3– reached a natural water body through industrial discharge, agricultural runoff and through human and animal waste (Nazneen et al. 2019). NO3– plays a pivotal role in quality estimation of aquatic environments. Our study showed that the amount of NO3– varied from 0.003 to 0.04 mg/l with mean of 0.01 mg/l (Table 1). Spatially, NO3– showed non-significant variation (Table 2). The NO3– concentration was highest in MON and lowest in PRM (Table 4, Fig. 4). Compared to other reservoirs [Krishnagiri (Ambujam and Sudha 2016) and Almatti (Hulyal and Kaliwal 2009)] in India, the values of NO3– were lower in the Mettur reservoir.
According to Smith (1995), low ratio of inorganic nitrogen to phosphorus in eutrophic aquatic body may be associated with the dominance of heterocytous cyanobacteria, as they are able to fulfill their nitrogen requirement through ammonium and nitrate-N and then through di-nitrogen. Wood et al. (2010) also suggested to monitor these group of phytoplankton in water bodies when nitrogen is limiting nutrients. This investigation also points toward the regular monitoring of phytoplankton diversity with reference to the heterocytous cyanobacteria blooms.
Total P and Phosphate-P
Phosphorus content in freshwater reservoirs of India is very low (Sugunan 2000), and it is rarely found at high concentration. Higher value of phosphate is an indicator of pollution and eutrophication. Orthophosphate and polyphosphate along with a little amount of organic phosphate are the main forms of phosphorus in a freshwater ecosystem (Markad et al. 2019). Agricultural runoff with phosphatic fertilizer, sewage and detergents are the main source of aquatic phosphorus.
Spatially, TP and PO43−–P content were not significantly different. Highest values for both TP and PO43− were recorded in site 1 and the lowest values at site 6 (Table 2). Higher values for PO43−–P and TP were found during MON (Table 4). Measured concentrations of phosphorus were higher than in the Tiru reservoir (Markad et al. 2019), India. However, the values were lower than in the Almatti Reservoir (Hulyal and Kaliwal 2008), and Bhindawas Lake (Saluja and Garg 2017), of India, Rawar Lake reservoir of Pakistan (Malik and Nadeem 2011), and Volvi Lake of Greece (Gantidis et al. 2007). Nevertheless, when TP concentration reached 0.2 mg/l, it may cause eutrophication along with high algal bloom (Jiang et al. 2010). High phosphorus concentration in the wet season (monsoon) may be due to non-point source loading through precipitation and leaching. Qian et al. (2007) also found a higher nutrient loading in the wet season.
Silicates
Silicates are important nutrient parameters for growth of phytoplankton especially diatoms (Znachor et al. 2013). Seasonal silica concentration in this reservoir varied significantly with highest value in PRM (avg. 16.4 mg/l), and lowest in MON (avg. 3.4 mg/l) (Table 4, Fig. 4). Spatially, silica content varied non-significantly (Table 2). Higher values of silicate in the Mettur reservoir may be due to higher accumulation of sediment/silt. This spatio-temporal differences are due to several factors like physical mixing of silicate and their relative proportion, their sedimentary adsorption, interaction with clay mineral, co-precipitation with humus and iron, or its consumption by phytoplankton specially diatom (Araújo et al. 2011). Silicate concentration up to 0.5 mg/l is considered limiting nutrient for diatom growth and values higher than this, as found in this study, indicate eutrophic condition with higher algal biomass and poor water quality.
Turbidity
Turbidity values were significantly different across the sites with average values ranging from 4.03 to 15.53 NTU (Table 2). While high values at 8th and 9th sites, situated in the riverine zone of this reservoir, this circumstances arises due to riverine load along with eroded sediment particles. Seasonal variation indicates that turbidity values in PRM and MON were significantly higher than in POM (Table 4, Fig. 4). The seasonal differences are in accordance with Kebede et al. (2014) and Teshome (2020). Deposition of undiluted summer discharge resulted in higher value of turbidity in PRM.
Chlorophyll-a
Due to its direct relation to algal biomass, chlorophyll-a (Chl-a) can be considered as an important parameter for indicating the trophic state of aquatic ecosystems (Boyer et al. 2009). It is reliable indicator due its sensitivity to ecosystem changes, easy to monitor and scientifically more logical (Boyer et al. 2009). In the Mettur reservoir, Chl-a contents were non-significant with reference to the sampling sites (Table 2). Seasonally, the Chl-a concentrations ranged from 9 to 28 (avg. 20.0 ± 4.9) µg/l and from 17 to 41 (avg. 29.6 ± 7.7) µg/l in MON and POM, respectively, while elevated values of 52–76.2 µg/l (avg. 67.9 ± 8.6) were recorded during PRM (Table 4). The Chl-a boxplot (Fig. 4) clearly showed high temporal variation during the investigated period. In general, nitrogen and phosphorus controls the concentration of chlorophyll. However, here, Chl-a concentration was highest in PRM, whereas nitrogen and phosphorus values were maximum in MON. However, high silicate content in PRM, which is important for phytoplankton growth, might have resulted in high Chl-a value in PRM. Based on the Chl-a value, the Mettur reservoir can be categorized as a eutrophic reservoir as per the criteria suggested by Nürnberg (1996).
Determination of Trophic State of Mettur Reservoir
Site wise and season wise values for TSI are presented in Table 5 and Fig. 5, respectively. The site wise average values for TSI (TP), TSI (Chl-a) and TSI (SD) were 54.6, 58.0 and 65.3, respectively (Table 5). All the TSI (avg) values were in the range of marginal eutrophic threshold values (Table 5). Overall, TSI (avg) value for the Mettur reservoir was 59.3. These TSI values, based on the modified version of Lamparelli (2004) classification criteria, clearly indicate that the Mettur reservoir is moderately eutrophic to eutrophic during the entire investigation period. However, seasonal difference was significant with highest value during PRM and lowest value during POM.
Carlson’s 2D Approach for Representing the TSI Deviation
As reported by Carlson (1991), any deduction of TSI (Chl-a) value with any other TSI will always be zero or toward zero, and any random variation will result in nonzero value for these differences. Still, in practice there will be always an expected deviation between these TSIs and this can be used for the assessment of degree and type of nutrient limitation. The best way to represent this nutrient limitation in lake or reservoir is through Carlson’s two-dimensional approaches.
Here, four different situations arose due to differences between these three TSIs. TSI (Chl-a) < TSI (TP) indicates the lower algal productivity than it was expected and phosphorus was not the only controlling factor. TSI (Chl-a) < TSI (SD) indicates the dominance of abiotic particles for turbidity with reduction in light intensity. By plotting TSI (Chl-a) − TSI (SD) against TSI (Chl-a) − TSI (TP), we get the TSI 2-D approach graph (Fig. 6) from the data obtained from the Mettur reservoir in different seasons. Data points at different quadrants of Fig. 6 indicate different assumptions. Large particles like blue green algae dominancy are represented by data points in the first quadrant, where influence of particles on transparency is less. Zooplankton grazing, increase in transparency and decrease in chlorophyll are represented by data points in the second quadrant. Non-algal turbidity is represented by data points in the third quadrant, where phosphorus is bound to those particles. Small particle dominance is represented by data points in the fourth quadrant. Data points near the diagonal indicates strong positive correlation between TSI (TP) and TSI (SD) and weak relation between TSI (Chl-a) and TSI (SD), which also indicates the dominance of inorganic seston. The 2D graph for the Mettur reservoir indicates that most of the data points for PRM and POM lie in the first quadrant, signifying large algal bloom dominancy and implies that phosphorus was limiting nutrient. However, the MON data are dispersed almost in all the four quadrants. This large variation may be due to differences in macrophyte coverage, depth and location of the site. Saluja and Garg (2017) also reported dispersion of monsoon data in all the quadrants for Bhindawas Lake of Haryana, India. Kumar et al. (2019) reported that nutrients were the limiting factor for Renuka Lake of Lesser Himalaya, India. Figure 6 also implies the dominance of non-algal particles during the MON. However, due to reduction in non-algal turbidity, there was less difference between TSI (SD) − TSI (Chl-a) as well as between TSI (TP) during PRM and POM (Markad et al. 2019). Previous reports (e.g., An and Park 2003; Markad et al. 2019) also indicate that nutrients were not the only limiting factors for productivity and non-algal particles also reduced the light particularly during monsoon.
Hydrogeochemical Process Involved in the Reservoir Basin
The relation between reservoir water and basin minerals has an important role in controlling reservoir water quality (Cederstorm 1946). To identify the various hydrogeochemical processes involved in aquatic bodies, water quality parameters are frequently subjected to various graphical plots. Based on the parameters studied in the present investigation, the Ca/Mg ratio was chosen as a one of the important indicators to determine the hydrogeochemical processes involved in this reservoir basin (Maya and Loucks 1995). A Ca/Mg ratio above 1 indicates dolomite dissolution. A Ca/Mg ratio of > 2 indicates influence of silicate minerals in contributing Ca and Mg to aquatic systems (Katz et al. 1997; Srinivasamoorthy et al. 2014). The ratio fell below 1 for all the samples during the PRM, indicating precipitation of Ca in the form of CaCO3 and thus resulted in decline of Ca or ion exchange process (Fig. 7). The Ca/Mg ratio for most of the samples in the MON and POM fell above 1, indicating dolomite dissolution. Some of the samples in MON and POM lie above 2, indicating the influence of silicate minerals. Thus, the weathering process in this reservoir basin is influenced by the presence of silicates and carbonates (Mohamed and Zineb 2015).
Water Quality Index
Eleven different physico-chemical water quality parameters were considered for calculating WQI (Table 6) to define the Mettur reservoir water for designated use. The site- and season-wise values of WQI are presented in Table 7. Based on the calculated values of WQI, the potability of water bodies can be categorized as follows: values of 0–25 = “Excellent”; 26–50 = “Good”; 51–75 = “Poor”; 76–100 = “Very poor”; and > 100 = ”Unsuitable” (Etim et al. 2013). Season-wise averages of WQI for the Mettur reservoir were 153.7, 158.2 and 134.1 (Table 7) during PRM, MON and POM, respectively; thus, indicating its unsuitability for human consumption (Brown et al. 1972). Ravikumar et al. (2013) also applied this method and found almost similar WQI values (111.69 to 137.09) for the Mallathahalli Lake in India.
The Mettur reservoir was constructed along Cauvery River, which had higher concentration of major ions than the Himalayan Rivers and streams (Dudgeon 1999). The discharge of domestic sewage into the Mettur reservoir is answerable for the higher WQI values, and the same pattern was also seen in the Cauvery River (Kalavathy et al. 2011). Seasonally, WQI values were higher in the PRM and MON than in POM. This indicates high pollution load along with precipitation during MON, while discharge of concentrated wastewater without dilution caused high values for WQI in PRM. Similar seasonal variation for WQI was also reported by Hemamalini et al. (2017) in India and Khwakaram et al. (2012) in Iraq. Teshome (2020) found very high WQI values (870.3–1313) for Lake Hawassa in Ethiopia (Africa), which is very dangerous for human consumption as compared to the present study.
Spatially, narrow differences were observed for WQI, which may be due to rapid internal water exchange. However, some points in the lacustrine and riverine zones showed comparatively higher value. For example, site 3 in the lacustrine zone showed higher WQI value where discharge is very high as already mentioned. Site 9 in the riverine zone also showed the highest WQI, which may be due to river discharge. Sites in the transitional zone showed intermediate values due to rapid exchange of water between riverine and lacustrine zones. Some parameters are compliant to the permissible limit for human consumption; however, overall, WQI values were very high and may pose a threat for its intended use.
Nevertheless, this study will be very helpful to policy-makers for management decision-making. The WQI is calculated considering the large set of dataset and thus representing the overall quality of water. In contrast, any other index developed based on single or few parameters may not be appropriate for managers to take suitable management decisions and they also do not reflect the true picture of water quality. As already mentioned, pH, EC and turbidity may affect the quality of water and thus local managers should take the appropriate steps to reduce the values of these parameters.
Evaluation of the Mettur Reservoir Water for Aquatic Life and Recreation
For sustaining aquatic life and fish growth, quality can be defined by considering selected physico-chemical parameters in terms of their tolerable limits. Every life function of fish such as breeding, feeding, swimming, digestion, excretion and even disease susceptibility is dependent on the quality of physical support of fish, i.e., water. Sudden changes in these parameters may adversely affect the growth and survival of fish. According to CPCB (2007), water pH from 6.5 to 8.5 is optimum for propagation of wildlife and fisheries. The pH may influence micronutrient availability and thus their consumption by aquatic organisms including fish. Most of the study sites of the present reservoir, pH values were marginally compliant to the standard value for aquatic life and recreation except in MON for which values from all sites were almost compliant with the prescribed range (Table 2). Majumder et al. (2010) suggested that the minimum DO requirement for supporting diverse fish species ranges between 4 and 5 mg/l. The DO values of the present study indicated that the Mettur reservoir water remains well oxygenated and thus is sufficient for survival of biotic community and is suitable for fisheries activities.
However, very high DO (≈ 13–14 mg/l) is considered harmful for aquatic life, as fish may suffer from “gas bubble disease” although it is a rare event (Jiru et al. 2017). The bubbles or emboli block the flow of blood through blood vessels causing death. External bubbles emphysema can also occur and can be seen on fins, skin and on other tissues. Aquatic invertebrates are also affected by “gas bubble disease,” but at levels higher than those lethal to fish (Kumar and Puri 2012). Hence, an optimum level of DO with good quality water is very much important for aquatic life including fish. Turbid water stimulates bacterial growth and the pathogen gets escaped during the application of any kind of disinfectant in water. Thus, consumption of turbid water may pose health threat for aquatic life including fish (Zvikomborero 2005). Regardless of season and site, turbidity values in the Mettur reservoir were above the permissible value (< 5 NTU), which is of concern. For a healthy aquatic ecosystem, recommended BOD values for sensitive fishes range from 0 to 5 mg/l (Woldeab et al. 2018). In the Mettur reservoir, average BOD values at all the sites were in this recommended range, indicating the healthiness of this reservoir for fish growth.
Bhatnagar et al. (2004) suggested that hardness values of 75–150 mg/l are sufficient for fish growth and culture. Hardness values below 20 mg/l develop stress in fishes. In terms of fish growth, the hardness values of the Mettur reservoir water were below the acceptable range (Table 2). According to Kumar et al. (2020), ideally, TA values should be 50–300 mg/l for fish adaptation. The water productivity observed in the Mettur reservoir in terms of alkalinity (Table 2) is ideal for fish growth. Similar values of TA and hardness for the Mettur reservoir were also reported in the previous studies (e.g., Palaniswami et al. 2015; Sivakumar et al. 2017; Hema and Subramani 2013). Fish and aquatic communities cannot survive in high levels of chloride (Kumar and Puri 2012). As the Mettur reservoir water contains moderate levels of chloride, thus may not affect fish growth.
Concentration of nitrate-N in water should be ≤ 0.1 mg/l as it is considered conducive for aquatic life (Murdoch et al. 2001). Hence, the nitrate levels recorded in the present study (Table 2) may be harmless to aquatic life considering nutrient pollution. To maintain optimum aquatic life and their biodiversity, phosphate concentration should be in the range 0.005–0.02 mg/l (WHO 1986). The phosphate values in this study cross the mentioned level and may pose threat in terms of eutrophication. However, for optimum fish growth and culture, there are several opinions about phosphate level in water. Stone and Thomforde (2004) suggested that the desirable level of phosphate for fish is 0.06 mg/l, while Bhatnagar et al. (2004) suggested that phosphate level in the range 0.05–0.07 mg/l is optimum for fish growth. These indicate that phosphate content in the Mettur reservoir was lower than the desirable limit for optimum fish culture. Because there are different opinions regarding the desirable limits suitable for optimum fish growth, effort has to be dedicated to develop a fish-based water quality index system for evaluating the reservoir and lake ecosystem.
Correlation Analysis
Pearson’s correlation matrix was used to determine the relationships among the different variables at all the sites during all the three seasons (Table 8). A positive correlation between depth and transparency signifies that sites with higher depth are having high transparency. Depth is negatively associated with WT, DO, Cl−, Chl-a, and silicate attributing that high content of silicate in lower depth region and thus higher phytoplankton growth and resulted in high Chl-a and DO content. The significant positive correlations between transparency and ions in terms of TH and Ca–H as revealed in this study agree with the findings of Balogun (2015) for Badagry Creek. However, such relationships may be linked to low primary productivity, which may have led to decreased DO concentration (Manson 1991) as can be seen by the negative correlation between DO and transparency. Transparency showed strong negative correlations with BOD, NO3− and non-significant negative correlations with PO43−, TP, SiO2 and Chl-a. These indicate that turbid water contains more nutrients and higher Chl-a due to higher productivity as well as organic load due to BOD. pH is positively correlated with DO indicating that high photosynthesis resulted in increase in water pH (Muduli et al. 2013). pH is positively related with EC, TDS, TA, TH, Ca–H, Mg–H, Cl−, and negatively related with NO3−, PO43− and TP. The positive relation between pH and TA can be attributed to the acid neutralizing power of water (Ustaoglu and Tepe 2019). EC was significantly associated with TA, TH, Mg–H, Cl−, SiO2 and Chl-a but showed inverse relation with NO3−. The perfect correlation between EC and TDS indicates the high content of dissolved ions in the water. The positive correlation of EC with other cations and anions suggests dissolution of evaporates.
Ca and Mg may be from either the weathering of carbonates or the dissolution of evaporates (Xiao et al. 2015). In the semi-arid climate and alkaline condition, dissolution is a common process which determines the water chemistry (Sajil Kumar and James 2016). The studied area also falls under semi-arid environments. These suggest that dissolution of calcite and evaporation are prominent in the geo-hydro environment. Thus, these processes may lead to higher Ca and Mg in water and thus finally contributing to EC. TDS also showed a similar trend. Both TH and TA showed positive correlations with Ca–H, Mg–H, Cl−, but negative relations with nutrients. This indicates the influence of carbonate source and evaporates. Ca is the main contributing factor of TH as they have positive association. High correlation between Ca and Mg indicates a common origin, which is very likely related to the geological characteristics of the sampling locations (Drever 1997; Mahlknecht 2003). Cl− is positively correlated with pH, ORP, EC, TDS, TA, TH, Mg–H, SiO2, and Chl-a, indicating that they have the same origin (Kjeldsen et al. 2002). Cl− has negative relation with organic pollution (NO3− and PO43−) indicating the contribution of land drainage as a source of nutrient pollution. Positive relation between NO3− and PO43− indicates their same source dynamics. Silicate is negatively correlated with NO3− and PO43−. Chl-a has negative relation with NO3− but has a positive relation with silicate attributing to differences in nutrient stoichiometry. The negative correlations of nutrients like NO3− and PO43− with different parameters (TA, TH, Ca–H, Mg–H and Cl−) during all the seasons indicated multiple sources (Gaury et al. 2018). Nutrients like NO3− and PO43− showed comparatively high values in MON, which might be due to surface runoff from farms and storm water runoff into the reservoir during early rain (Gupta et al. 2011). In contrast, maximum values of TA, TH and Ca–H were in POM but for Mg–H and Cl− they were in PRM (Table 4). This indicates that basin geology, weathering process and sewage discharge may be the main source of TA, TH and Ca– Mg–H and Cl−. Thus, multiple sources are operating in this reservoir for controlling the water quality parameters in different seasons.
Cluster Analysis
Cluster analysis was performed for grouping of the nine sampling sites in the Mettur reservoir based on the similarities or dissimilarities of water quality parameters, resulting in a dendrogram (Fig. 8). Based on the results, all the nine sampling sites of the Mettur reservoir were grouped into three statistically significant important clusters, which significantly and distinctly differentiate the three zones of the reservoir, i.e., riverine, transition and lacustrine zones. However, site 7 in the transitional zone was grouped with the sites in the riverine zone. Sites 1, 2 and 3 form cluster 1 and can be considered as high polluted sites, as these sites belong to the lacustrine zone and received pollutants from the Mettur Sewage Treatment plant and the Mettur Thermal Power Plant. The discharge outlets of these plants are situated at the lacustrine zone and likely have contributed to the high pollution status in this zone. Sites 7, 8 and 9 form cluster 2, which correspond to moderate pollution sites. Sites 8 and 9 belong to the riverine zone and receive inputs mostly from non-point sources (agricultural and catchment runoff) along with the riverine input as these inflow to the reservoir. However, site 7 in the transitional zone is also clubbed to this cluster, which may be due to some point sources of pollution. Cluster 3 includes sampling sites 4, 5 and 6 in the transitional zone, which comprises low polluted sites due to rapid exchange of water between riverine and lacustrine zone.
Cluster analysis differentiated each zone based on the water quality parameters. The three groups correspond with natural background features and with water quality character that is affected by different environmental effects/pollutants the sites were exposed to. The results of cluster analysis revealed clearly that the different zones of the Mettur reservoir, i.e., lacustrine, transitional, and riverine zones, fall under highly polluted moderately polluted and less polluted categories, respectively, and this can be correlated with the physico-chemical properties of water. This signifies that sites within a particular zone showed more or less similar characteristics. The results also indicate that during rapid survey, selection of few sites is imperative to represent the spatial variation of water quality parameters of the reservoir. It is clear from the results that cluster analysis is a useful tool for reliable classification of the whole Mettur reservoir water quality and it may also help in designing future sampling strategies for monitoring of reservoir water in an optimum manner. Thus, in future, sampling strategies should start with less number of sampling sites without compromising the outcome of the study. This method has been successfully used by several researchers (e.g., Parashar et al. 2008; Gholami and Srikantaswamy 2009; Najar and Khan 2012; Varol and Sen 2012) in their water quality monitoring programs.
Principal Component Analysis /Factors Analysis (PCA/FA)
Factor analysis was applied to the large dataset to convert it into a meaningful interpretation to disclose the association of measured variables and to establish the ecosystem processes that operated in the reservoir. Here, we have taken only those factors with eigenvalues of > 1. Based on the loading values, the factors loadings were considered ‘weak,’ ‘moderate’ and ‘strong,’ when values of absolute loadings are 0.50–0.30, 0.75–0.50 and > 0.75, respectively. Four factors with eigenvalues of > 1 were considered, which explain > 87.56% data variability (Table 9).
Factor-1 account for > 39% data variability (Fig. 9) with the dominance of WT, ORP, EC, TDS, Cl−, Mg–H, SiO2 and Chl-a, signifying the impact of both rock weathering and anthropogenic activities. Positive loading on Chl-a may be related to high growth of phytoplankton and thus high consumption of CO2 during photosynthesis, which results in higher pH (Barik et al. 2017). Moderate negative loading on NO3− in Factor-1 indicates fertilizer runoff due to agricultural activities, which are very common in the dry season.
Factor-2 account for > 21% of data variance with the dominance of pH, DO, TA, TH, and Ca–H, indicating both natural and anthropogenic activities. High pH values also indicate catchment areas that feed into the Mettur reservoir are polluted or degraded (Tran et al. 2010). Factor-3 account for > 15% of data variance with the dominance of DO, BOD and turbidity, suggesting an unhealthy state of the reservoir water. In addition, the opposite loadings on transparency and turbidity re-affirmed the correlation analysis results, which indicate that low transparency is due to high turbidity. It seems that changes in depth plays negative roles in the hydrological features of this reservoir because depth is negatively related with Factor-3. However, Factor-4 accounts for only 12.15% of data variance with higher contributions of PO43− and TP, indicating the major anthropogenic activities. The factor analysis indicates that basin geology, anthropogenic stress and agricultural applications simultaneously play important roles in defining the water quality of the Mettur reservoir.
Conclusions and Recommendation
In this investigation, spatio-temporal differences for selected physico-chemical properties in the Mettur reservoir water were evaluated to assess water quality and to identify the possible pollution sources. In addition, the suitability of water in terms of drinking water, human health, and domestic use along with protection of aquatic life including fish growth was discussed. Spatial variation indicates that surface runoff and sewage mixing have a definite role in defining the reservoir water quality, and it helps in identifying the possible sources of pollution. However, temporal variation was more pronounced as some of the physico-chemical properties of water showed dilution effect during monsoon and post-monsoon while in terms of selected quality parameters, water remain poorest in summer, which may be due to low discharge and entry of undiluted sewage waste. High WQI in all the seasons indicated slow deterioration of reservoir water quality, and water is unsuitable for drinking. Hence, an immediate precaution has to be taken for those communities who are using this reservoir as drinking water source.
Based on TSI values, this water body can be categorized as moderately-eutrophic to eutrophic. With reference to the protection of aquatic life and fish growth, this water body can be considered as moderately suitable with some values higher than the requirement for optimum fish growth and thus pose a threat for aquatic biodiversity loss. PCA/FA indicated that multiple sources including natural, anthropogenic and agricultural activities influence the physico-chemical variation in water quality. This revealed that emphasis should be given for pollution control as effective management strategies as waste water discharge is the main source of pollution in the Mettur reservoir. Cluster analysis results of this study can be effectively used in formulating sampling strategies for future monitoring programs and may also helps to identify critically affected areas in the reservoir in terms of both natural and anthropogenic pollution. Managers of water quality and water monitoring programs are often faced with constraints in terms of budget, time and laboratory capacity for sample analysis. In such a situation, results of cluster analysis can be used to reduce the number sampling sites without losing any vital information. To protect the quality of water in the Mettur reservoir as well as to restore its suitability for designated use, the following steps have to be taken: (i) minimization of disposal of industrial effluents; (ii) implementation of advance and effective treatment technology before discharge of waste water into this reservoir; (iii) control fertilizer use in the catchment area and buffering of riparian zones from agricultural runoff is needed; (iv) enforcement of strict water quality regulation measures; and (v) regular monitoring of reservoir water to check its quality deterioration and to formulate and implement effective management strategies.
This study thus revealed that multivariate statistical techniques can be effectively used for converting a large water quality dataset into a simple interpretation for identifying pollution sources along with spatio-temporal variation determination for effective management practices. The results obtained in this work can be useful in future management of the reservoir, its water and the activities in the catchment area. The outcome of this study can be a helping hand to the researchers to extend it further and for the policy-makers to address it in their plans.
Notes
1 tmc ft = 28,316,846.592 m3.
References
Adakole, J.A. (2000). The effects of domestic, agriculture and industrial effluents on the water quality and biota of Bindare stream, Zaria – Nigeria. Ph.D. Thesis, Department of Biological Sciences, Ahmadu Bello University, Zaria, Nigeria.
AlMohseen, K.A. (2003). Optimal operation of multi-purpose multi-reservoir water resources system. Ph.D. Thesis, Department of Civil Engineering, IIT, Delhi, India.
Ambujam, N. K., & Sudha, V. (2016). Environmental impacts of point and non-point source pollution in Krishnagiri reservoir: A case study in South India. International Journal of Environmental and Ecological Engineering, 10(4), 443–449.
An, K. G., & Park, S. S. (2003). Influence of seasonal monsoon on the trophic state deviation in an Asian reservoir. Water, Air, and Soil Pollution, 145(1–4), 267–287.
Anbazhagan, V. (2018). Analysis and assessment of heavy metals in soils around the industrial areas in Mettur, Tamilnadu India. Environmental monitoring and assessment, 190(9), 519.
Anonymous. (2005). State of Environment of Tamil Nadu, Dr. S. Balaji, IFS, Department of Environment, Government of Tamil Nadu. Undated. Release date: 2005. http://www.environment.tn.nic.in/soe/soe_report.htm. Accessed 3 April 2020.
Anshumali, & Ramanathan, A. L. (2007). Seasonal variation in the major ion chemistry of Pandoh Lake, Mandi district, Himachal Pradesh India. Applied Geochemistry, 22(8), 1736–1747.
APHA. (2005). Standard methods for the examination of water and wastewater (21st ed.). Washington, DC: American Public Health Association.
Araújo, F. G., Costa de Azevedo, M. C., & Lima Ferreira, M. D. N. (2011). Seasonal changes and spatial variation in the water quality of a eutrophic tropical reservoir determined by the inflowing river. Lake and Reservoir Management, 27(4), 343–354.
Azhar, S. C., Aris, A. Z., Yusoff, M. K., Ramli, M. F., & Juahir, H. (2015). Classification of river water quality using multivariate analysis. Procedia Environmental Sciences, 30, 79–84.
Balogun, K. J. (2015). Effects of abiotic and biotic factors on fish productivity in Badagry creek, Nigeria. Ph.D. Thesis, University of Ibadan, Nigeria.
Barik, S. K., Muduli, P. R., Mohanty, B., Behera, A. T., Mallick, S., Das, A., et al. (2017). Spatio-temporal variability and the impact of Phailin on water quality of Chilika lagoon. Continental Shelf Research, 136, 39–56.
Belkhiri, L., Boudoukha, A., & Mouni, L. (2011). A multivariate statistical analysis of groundwater chemistry data. International Journal Environmental Research, 5(2), 537–544.
Bhatnagar A., Jana S. N., Garg S. K. Patra B. C., Singh G., & Barman U. K. (2004). Water quality management in aquaculture. In: Course Manual of summer school on development of sustainable aquaculture technology in fresh and saline waters (pp. 203–210) CCS Haryana Agricultural, Hisar, India.
BIS:10500. (2012). Indian standard: Drinking water-Specification. New Delhi, India: Second Revision Bureau of Indian Standard.
Boyer, J. N., Kelble, C. R., Ortner, P. B., & Rudnick, D. T. (2009). Phytoplankton bloom status: Chlorophyll a biomass as an indicator of water quality condition in the southern estuaries of Florida, USA. Ecological Indicators, 9(6), S56–S67.
Brown, R. M., McClelland, N. I., Deininger, R. A., & O’Connor, M. F. (1972). A water quality index—crashing the psychological barrier. In W. A. Thomas (Ed.), Indicators of environmental quality (pp. 173–182). Boston, MA: Springer.
Carlson, R. E. (1977). A trophic state index for lakes. Limnology and Oceanography, 22(2), 361–369.
Carlson, R. E. (1991). Expanding the trophic state concept to identify non-nutrient limited lakes and reservoirs. In L. Carpenter (Ed.), Proceedings of a national conference on enhancing the states’ lake management programs (pp. 59–71). Chicago: USEPA.
Cederstorm, D. J. (1946). Genesis of groundwater in the coastal plain of Virginia. Environmental Geology, 41, 218–245.
CPCB (Central pollution control board) (2007). Guidelines for water quality monitoring, Ministry of Environment, Forest and Climate Change, Government of India, New Delhi, India.
Das Sarkar, S., Gogoi, P., Sarkar, U. K., Das Ghosh, B., & Mishal, P. (2019). Trophic state index to assess aquatic ecosystem health. Indian Farming, 69(03), 63–66.
Drever, J. I. (1997). The geochemistry of natural waters (3rd ed., p. 436). NJ: Prentice-Hall.
Dudgeon, D. (1999). Tropical Asian streams: Zoobethos, ecology and conservation. Hong Kong SAR, China: Hong Kong University Press.
Etim, E. E., Odoh, R., Itodo, A. U., Umoh, S. D., & Lawal, U. (2013). Water quality index for the assessment of water quality from different sources in the Niger Delta Region of Nigeria. Frontiers in Science, 3(3), 89–95.
Fan, X., Cui, B., Zhao, H., Zhang, Z., & Zhang, H. (2010). Assessment of river water quality in Pearl River Delta using multivariate statistical techniques. Procedia Environmental Sciences, 2, 1220–1234.
Gantidis, N., Pervolarakis, M., & Fytianos, K. (2007). Assessment of the quality characteristics of two lakes (Koronia and Volvi) of Northern Greece. Environmental Monitoring and Assessment, 125, 175–181.
Gaur, R. K. (1998). Limnology of a leachate reservoir receiving effluents from a thermal power plant. Ph.D. Thesis, Aligarh Muslim University, Aligarh, India.
Gaury, P. K., Meena, N. K., & Mahajan, A. K. (2018). Hydrochemistry and water quality of Rewalsar Lake of Lesser Himalaya, Himachal Pradesh India. Environmental Monitoring and Assessment, 190(2), 84.
Gholami, S., & Srikantaswamy, S. (2009). Statistical multivariate analysis in the assessment of river water quality in the vicinity of KRS Dam, Karnataka India. Natural Resources Research, 18(3), 235–247.
Guarino, A. W. S., Branco, C. W. C., Diniz, G. P., & Rocha, R. (2005). Limnological characteristics of an old tropical reservoir (Ribeira˜o das Lajes Reservoir, RJ, Brazil). Acta Limnologica Brasiliensia, 17(2), 129–141.
Gupta, P., Rawtani, P. M., & Vishwakarma, M. (2011). Study and interpretation of physico-chemical characteristic of Kerwa dam water quality in Bhopal city (India). International Journal of Theoretical and Applied Science, 3(1), 28–34.
Hamlat, A., Tidjani, A.E.-B., Yebdri, D., Errih, M., & Guidoum, A. (2013). Water quality analysis of reservoirs within Western Algeria catchment areas using Water Quality Index CCME WQI. Journal of Water Supply: Research and Technology—AQUA, 63, 311–324.
Hema, S., & Subramani, T. (2013). Study of physico-chemical characteristics of surface water using regression analysis of Cauvery River and its tributaries in Tamilnadu India. Asian Journal of Chemistry, 25(6), 3199–3203.
Hemamalini, J., Mudgal, B., & Sophia, J. D. (2017). Effects of domestic and industrial effluent discharges into the lake and their impact on the drinking water in Pandravedu village, Tamil Nadu, India. Global Nest Journal, 19, 225–231.
Hulyal, S. B., & Kaliwal, B. B. (2008). Water quality assessment of Almatti reservoir of Bijapur (Karnataka State, India) with special reference to zooplankton. Environmental Monitoring and Assessment, 139(1–3), 299–306.
Hulyal, S. B., & Kaliwal, B. B. (2009). Dynamics of phytoplankton in relation to physico-chemical factors of Almatti reservoir of Bijapur District Karnataka State. Environmental Monitoring and Assessment, 153(1–4), 45–59.
Jain, S. K., Agarwal, P. K., & Singh, V. P. (2007). Cauvery and Pennar basins. Hydrology and water resources of India (pp. 701–741). Dordrecht: Springer.
Jiang, C., Zhu, L., Hu, X., Cheng, J., & Xie, M. (2010). Reasons and control of eutrophication in new reservoirs. In A. A. Ansari, G. S. Singh, & G. R. Lanza (Eds.), Eutrophication: Causes, consequences and control (pp. 325–340). Dordrecht: Springer.
Jiru, M., North-Kabore, J., & Roth, T. (2017). Studying water quality using socio-environmental synthesis approach: A case study in Baltimore’s Watershed. Hydrology, 4(2), 32.
Jonnalagadda, S., & Mhere, G. (2001). Water quality of the Odzi River in the Eastern Highlands of Zimbabwe. Water Research, 35, 2371–2376.
Kalavathy, S., Sharma, T. R., & Sureshkumar, P. (2011). Water quality index of river Cauvery in Tiruchirappalli district, Tamilnadu. Archives of Environmental Science, 5, 55–61.
Katz, B. G., Coplen, T. B., Bullen, T. D., & Davis, J. H. (1997). Use of chemical and isotopic tracers to characterize the interactions between ground water and surface water in mantled karst. Ground Water, 35(6), 1014–1028.
Kebede, W., Tefera, M., Habitamu, T., & Alemayehu, T. (2014). Impact of land cover change on water quality and stream flow in Lake Hawassa watershed of Ethiopia. Agricultural Sciences, 5, 647–659.
Khwakaram, A. I., Majid, S. N., & Hama, N. Y. (2012). Determination of water quality index (WQI) for Qalyasan stream in Sulaimani city/Kurdistan region of Iraq. International Journal of Plant, Animal and Environmental Sciences, 2(4), 148–157.
Kjeldsen, P., Barlaz, M. A., Rooker, A. P., Baun, A., Ledin, A., & Christensen, T. H. (2002). Present and long-term composition of MSW landfill leachate: A review. Critical Reviews in Environmental Science and Technology, 32(4), 297–336.
Korkanç, S. Y., Kayıkçı, S., & Korkanç, M. (2017). Evaluation of spatial and temporal water quality in the Akkaya dam watershed (Niğde, Turkey) and management implications. Journal of African Earth Sciences, 129, 481–491.
Kumar, D., Malik, D. S., Kumar, N., Gupta, N., & Gupta, V. (2020). Spatial changes in water and heavy metal contamination in water and sediment of river Ganga in the river belt Haridwar to Kanpur. Environmental Geochemistry and Health, 42, 2059–2079.
Kumar, M., & Puri, A. (2012). A review of permissible limits of drinking water. Indian Journal of Occupational and Environmental Medicine, 16(1), 40.
Kumar, P., Mahajan, A. K., & Meena, N. K. (2019). Evaluation of trophic status and its limiting factors in the Renuka Lake of Lesser Himalaya India. Environmental Monitoring and Assessment, 191(2), 105.
Kumari, R., & Sharma, R. C. (2019). Assessment of water quality index and multivariate analysis of high altitude sacred lake Prashar, Himachal Pradesh, India. International Journal of Environmental Science and Technology, 16(10), 6125–6134.
Lamparelli, M. C. (2004). Grau de Trofia em Corpos D’Água do Estado de São Paulo:Avaliação dos métodos de monitoramento. Ph.D. Thesis, University of São Paulo, São Paulo, Brazil.
Mahlknecht, J. (2003). Estimation of recharge in the Independence aquifer, central Mexico, by combining geochemical and groundwater flow models. Ph.D. Thesis, Institute of Applied Geology, University of Agriculture and Life Sciences (BOKU), Vienna, Austria.
Majumder, M., Dutta, S., Jana, B. K., Barman, R. N., Roy, P., & Mazumdar, A. (2010). Estimation of the spatial variation of water quality by neural models and surface algorithms. In B. Jana & M. Majumder (Eds.), Impact of climate change on natural resource management (pp. 183–201). Dordrecht: Springer.
Malik, R. N., & Nadeem, M. (2011). Spatial and temporal characterization of trace elements and nutrients in the Rawal Lake Reservoir, Pakistan using multivariate analysis techniques. Environmental Geochemistry and Health, 33(6), 525–541.
Manson, C. F. (1991). Biology of freshwater pollution (2nd ed.). New York: Longman scientific and Technical, John Wiley and Sons.
Markad, A. T., Landge, A. T., Nayak, B. B., Inamdar, A. B., & Mishra, A. K. (2019). Trophic state modeling for shallow freshwater reservoir: A new approach. Environmental Monitoring and Assessment, 191(9), 586.
Matta, G., Kumar, A., Nayak, A., Kumar, P., Kumar, A., & Tiwari, A. K. (2020a). Determination of water quality of Ganga River System in Himalayan region, referencing indexing techniques. Arabian Journal of Geosciences, 13, 1027.
Matta, G., Kumar, A., Tiwari, A. K., Naik, P. K., & Berndtsson, R. (2020b). HPI appraisal of concentrations of heavy metals in dynamic and static flow of Ganga River System. Environment, Development and Sustainability, 22, 33–46.
Mayo, A. L., & Loucks, M. D. (1995). Solute and isotopic geochemistry and ground water flow in the central Wasatch Range Utah. Journal of Hydrology, 172(1–4), 31–59.
Mishra, P., Garg, V., & Dutt, K. (2019). Seasonal dynamics of phytoplankton population and water quality in Bidoli reservoir. Environmental monitoring and assessment, 191(3), 130.
Mohamed, C., & Zineb, A. (2015). Geochemistry and hydrogeochemical process of groundwater in the Souf valley of Low Septentrional Sahara, Algeria. African Journal of Environmental Science and Technology, 9(3), 261–273.
Mohan, S., & Ramsundram, N. (2016). Predictive temporal data-mining approach for evolving knowledge based reservoir operation rules. Water Resources Management, 30(10), 3315–3330.
Muduli, P. R., Kanuri, V. V., Robin, R. S., Kumar, B. C., Patra, S., Raman, A. V., et al. (2013). Distribution of dissolved inorganic carbon and net ecosystem production in a tropical brackish water lagoon, India. Continental Shelf Research, 64, 75–87.
Murdoch, T., Cheo, M., & O’Laughlin, K. (2001). Streamkeeper’s field guide: Watershed inventory and stream monitoring methods. Everett, WA: Adopt-A-Stream Foundation.
Najar, I. A., & Khan, A. B. (2012). Assessment of water quality and identification of pollution sources of three lakes in Kashmir, India, using multivariate analysis. Environmental Earth Sciences, 66(8), 2367–2378.
Nazneen, S., Raju, N. J., Madhav, S., & Ahamad, A. (2019). Spatial and temporal dynamics of dissolved nutrients and factors affecting water quality of Chilika lagoon. Arabian Journal of Geosciences, 12(7), 243.
Nürnberg, G. K. (1996). Trophic state of clear and colored, soft-and hardwater lakes with special consideration of nutrients, anoxia, phytoplankton and fish. Lake and Reservoir Management, 12(4), 432–447.
OECD. (1982). Eutrophication of waters: monitoring, assessment and control (p. 147). Paris: Technical Report, Environmental Directorate, OECD.
Ouyang, Y. (2005). Evaluation of river water quality monitoring stations by principal component analysis. Water Research, 39(12), 2621–2635.
Ouyang, Y., Nkedi-Kizza, P., Wu, Q. T., Shinde, D., & Huang, C. H. (2006). Assessment of seasonal variations in surface water quality. Water Research, 40(20), 3800–3810.
Palaniswami, R., Manoharan, S., & Mohan, A. (2015). Characterisation of tropical reservoirs in Tamil Nadu, India in terms of plankton assemblage using multivariate analysis. Indian Journal of Fisheries, 62(3), 1–3.
Parashar, C., Verma, N., Dixit, S., & Shrivastava, R. (2008). Multivariate analysis of drinking water quality parameters in Bhopal. Environmental Monitoring and Assessment, 140, 119–122.
Poudel, D. D., Lee, T., Srinivasan, R., Abbaspour, K., & Jeong, C. Y. (2013). Assessment of seasonal and spatial variation of surface water quality, identification of factors associated with water quality variability, and the modeling of critical nonpoint source pollution areas in an agricultural watershed. Journal of Soil and Water Conservation, 68(3), 155–171.
Qian, Y., Migliaccio, K. W., Wan, Y., & Li, Y. (2007). Trend analysis of nutrient concentrations and loads in selected canals of the Southern Indian River Lagoon Florida. Water Air Soil Pollution, 186, 195–208.
Ravikumar, M., Nagaraju, D., Mahadevaswamy, G., Siddalingamurthy, S., Nagesh, P. C., & Rao, K. (2011). Groundwater pollution sensitivity model for part of Cauvery basin between Mettur dam and Erode town using Remote Sensing and GIS. International Journal of Geomatics and Geosciences, 1(4), 735–757.
Ravikumar, P., Mehmood, M. A., & Somashekar, R. K. (2013). Water quality index to determine the surface water quality of Sankey tank and Mallathahalli Lake, Bangalore urban district, Karnataka India. Applied water science, 3(1), 247–261.
Saha, A., Mol, S. S., Sudheesan, D., Suresh, V. R., Nag, S. K., Panikkar, P., & Das, B. K. (2020). Impacts of a massive flood event on the physico-chemistry and water quality of river Pampa in Western Ghats of India. International Journal of Environmental Analytical Chemistry. https://doi.org/10.1080/03067319.2020.1843026.
Sajil Kumar, P. J., & James, E. J. (2016). Identification of hydrogeochemical processes in the Coimbatore district, Tamil Nadu India. Hydrological Sciences Journal, 61(4), 719–731.
Saluja, R., & Garg, J. K. (2017). Trophic state assessment of Bhindawas Lake, Haryana India. Environmental Monitoring and Assessment, 189(1), 32.
Sandhya, K. M., Lianthuamluaia, L., Karnatak, G., Sarkar, U. K., Kumari, S., Mishal, P., et al. (2019). Fish assemblage structure and spatial gradients of diversity in a large tropical reservoir, Panchet in the Ganges basin India. Environmental Science and Pollution Research, 26(18), 18804–18813.
Sarkar, U. K., Sandhya, K. M., Mishal, P., Karnatak, G., Lianthuamluaia, A., Kumari, S., et al. (2018). Status, prospects, threats, and the way forward for sustainable management and enhancement of the tropical Indian reservoir fisheries: An overview. Reviews in Fisheries Science and Aquaculture, 26(2), 155–175.
Shah, J. A., Pandit, A. K., & Shah, G. M. (2017). Dynamics of physico-chemical limnology of a shallow wetland in Kashmir Himalaya (India). Sustainable Water Resources Management, 3(4), 465–477.
Sheela, A. M., Letha, J., Joseph, S., Ramachandran, K. K., & Sanalkumar, S. P. (2011). Trophic state index of a lake system using IRS (P6-LISS III) satellite imagery. Environmental Monitoring and Assessment, 177(1–4), 575–592.
Sivakumar, P., Senthamizhchelvi, T., Reguananth, R., Anusha, J., & Manivannan, S. (2017). Spatial assessment of water quality in the lower reaches of Cauvery River, Tamil Nadu. International Journal of Fisheries and Aquatic Studies, 5(2), 336–342.
Smith, V. H. (1995). Historical trends in the Lake Okeechobee ecosystem IV. Nitrogen: phosphorus ratios, cyanobacterial dominance and nitrogen fixation potential. Arch Hydrobiol Suppl, 107, 71–88.
Srinivasamoorthy, K., Gopinath, M., Chidambaram, S., Vasanthavigar, M., & Sarma, V. S. (2014). Hydrochemical characterization and quality appraisal of groundwater from Pungar sub basin, Tamilnadu, India. Journal of King Saud University-Science, 26(1), 37–52.
Srinivasamoorthy, K., Nanthakumar, C., Vasanthavigar, M., Vijayaraghavan, K., Rajivgandhi, R., Chidambaram, S., et al. (2011a). Groundwater quality assessment from a hard rock terrain, Salem district of Tamilnadu India. Arabian Journal of Geosciences, 4(1–2), 91–102.
Srinivasamoorthy, K., Vijayaraghavan, K., Vasanthavigar, M., Sarma, V. S., Rajivgandhi, R., Chidambaram, S., et al. (2011b). Assessment of groundwater vulnerability in Mettur region, Tamilnadu, India using drastic and GIS techniques. Arabian Journal of Geosciences, 4(7–8), 1215–1228.
Srinivasamoorthy, K., Vijayaraghavan, K., Vasanthavigar, M., Sarma, S., Chidambaram, S., Anandhan, P., & Manivannan, R. (2012). Assessment of groundwater quality with special emphasis on fluoride contamination in crystalline bed rock aquifers of Mettur region, Tamilnadu India. Arabian Journal of Geosciences, 5(1), 83–94.
Stone, N. M., & Thomforde, H. K. (2004). Understanding Your Fish Pond Water Analysis Report. USA: Cooperative Extension Program University of Arkansas at Pine Bluff Aquaculture/Fisheries.
Subramani, T., Kumar, K. A., Ganesan, A., Senthil, P., & Gunasekar, G. (2017). Design and management of Mettur dam by predicting seepage losses using remote sensing. International Journal of Application or Innovation in Engineering and Management (IJAIEM), 6(5), 327–336.
Sugunan, V. V. (2000). Ecology and fishery management of reservoirs in India. Hydrobiologia, 430(1–3), 121–147.
Tamil Nadu Pollution Control Board (TNPCB). (2019). Action Plan on Rejuvenation of River Cauvery Mettur to Mayiladuthurai Stretch (Priority-I). http://www.tnpcb.gov.in/pdf_2019/PrsCauvery24919.pdf. Accessed 5 April 2020.
Teshome, F. B. (2020). Seasonal water quality index and suitability of the water body to designated uses at the eastern catchment of Lake Hawassa. Environmental Science and Pollution Research, 27(1), 279–290.
Tibebe, D., Kassa, Y., Melaku, A., & Lakew, S. (2019). Investigation of spatio-temporal variations of selected water quality parameters and trophic status of Lake Tana for sustainable management, Ethiopia. Microchemical Journal, 148, 374–384.
Toma, J. J., Shekha, Y. A., & Al-Barzingy, Y. O. (2018). An ecological assessment for water quality of some water bodies in Koysenjaq-Erbil Iraq. Al-Nahrain Journal of Science, 21(2), 119–129.
Tran, C. P., Bode, R. W., Smith, A. J., & Kleppel, G. S. (2010). Land-use proximity as a basis for assessing stream water quality in New York State (USA). Ecological Indicators, 10(3), 727–733.
Ustaoğlu, F., & Tepe, Y. (2019). Water quality and sediment contamination assessment of Pazarsuyu Stream, Turkey using multivariate statistical methods and pollution indicators. International Soil and Water Conservation Research, 7(1), 47–56.
Varol, M. (2020). Spatio-temporal changes in surface water quality and sediment phosphorus content of a large reservoir in Turkey. Environmental Pollution, 259, 113860.
Varol, M., & Şen, B. (2012). Assessment of nutrient and heavy metal contamination in surface water and sediments of the upper Tigris River, Turkey. CATENA, 92, 1–10.
Wetzel, R. G. (2001). Limnology: Lake and river ecosystems. San Diego: Academic Press.
Woldeab, B., Beyene, A., Ambelu, A., Buffam, I., & Mereta, S. T. (2018). Seasonal and spatial variation of reservoir water quality in the southwest of Ethiopia. Environmental Monitoring and Assessment, 190(3), 163.
Wood, S. A., Prentice, M. J., Smith, K., & Hamilton, D. P. (2010). Low dissolved inorganic nitrogen and increased heterocyte frequency: precursors to Anabaena planktonica blooms in a temperate, eutrophic reservoir. Journal of Plankton Research, 32(9), 1315–1325.
World Health Organization (1986) Environmental Health Criteria, No.54. Ammonia. Geneva.
World Health Organization. (2011). Guidelines for drinking water quality (4th ed.). Geneva: World Health Organization.
Xiao, J., Jin, Z., Wang, J., & Zhang, F. (2015). Major ion chemistry, weathering process and water quality of natural waters in the Bosten Lake catchment in an extreme arid region NW China. Environmental Earth Sciences, 73(7), 3697–3708.
Yelavarthy, E. (2002). Hydro-biological studies of Red Hills reservoir, North Chennai, Tamil Nadu. Journal of Aquatic Biology, 17, 13–16.
Zdanowski, B. (1988). Long-term and seasonal changes in the primary production and destruction in heated lakes near Konin (Poland). Ekologia Polska, 36, 1–2.
Znachor, P., Visocká, V., Nedoma, J., & Rychtecký, P. (2013). Spatial heterogeneity of diatom silicification and growth in a eutrophic reservoir. Freshwater Biology, 58(9), 1889–1902.
Zvikomborero, H. (2005). An assessment of water quality of drinking water in rural districts in Zimbabwe. The case of Gpkwe, South, Nkayi, Lupane and Mweezi districts. Physics and Chemistry of the Earth, 30, 859–866.
Acknowledgments
This work has been funded by ICAR- Central Inland Fisheries Research Institute from its institutional research Grant.
Author information
Authors and Affiliations
Corresponding author
Rights and permissions
About this article
Cite this article
Saha, A., Ramya, V.L., Jesna, P.K. et al. Evaluation of Spatio-temporal Changes in Surface Water Quality and Their Suitability for Designated Uses, Mettur Reservoir, India. Nat Resour Res 30, 1367–1394 (2021). https://doi.org/10.1007/s11053-020-09790-5
Received:
Accepted:
Published:
Issue Date:
DOI: https://doi.org/10.1007/s11053-020-09790-5