Abstract
Context
Golden-cheeked warblers (Setophaga chrysoparia), an endangered wood-warbler, breed exclusively in woodlands co-dominated by Ashe juniper (Juniperus ashei) in central Texas. Their breeding range is becoming increasingly urbanized and habitat loss and fragmentation are a main threat to the species’ viability.
Objectives
We investigated the effects of remotely sensed local habitat and landscape attributes on point occupancy and density of warblers in an urban preserve and produced a spatially explicit density map for the preserve using model-supported relationships.
Methods
We conducted 1507 point-count surveys during spring 2011–2014 across Balcones Canyonlands Preserve (BCP) to evaluate warbler habitat associations and predict density of males. We used hierarchical Bayesian models to estimate multiple components of detection probability and evaluate covariate effects on detection probability, point occupancy, and density.
Results
Point occupancy was positively related to landscape forest cover and local canopy cover; mean occupancy was 0.83. Density was influenced more by local than landscape factors. Density increased with greater amounts of juniper and mixed forest and decreased with more open edge. There was a weak negative relationship between density and landscape urban land cover.
Conclusions
Landscape composition and habitat structure were important determinants of warbler occupancy and density, and the large intact patches of juniper and mixed forest on BCP (>2100 ha) supported a high density of warblers. Increasing urbanization and fragmentation in the surrounding landscape will likely result in lower breeding density due to loss of juniper and mixed forest and increasing urban land cover and edge.
Similar content being viewed by others
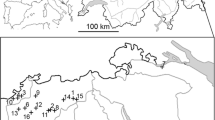
Avoid common mistakes on your manuscript.
Introduction
Habitat loss and fragmentation due to increasing urbanization is a leading cause for endangered and threatened species listing (Wilcove et al. 1998; Czech et al. 2000). Urban sprawl is an increasingly important source of land use change as human populations become more urban (Marzluff 2001). Urban sprawl often progresses as exurban development, or low-density residential development. This results in loss or fragmentation of native vegetation and habitat structure, depending on the severity of the land use changes. The impact to wildlife populations is difficult to generalize because some species thrive while others languish in response to increasingly urbanized landscapes (Marzluff 2001; Rodewald et al. 2013). However; native, forest-interior, insectivorous, and ground-nesting birds tend to respond negatively to greater urban land cover (Marzluff 2001; Chace and Walsh 2006) and there is evidence that forest birds are negatively affected by urbanization even within forest remnants (Rodewald et al. 2013; Suarez-Rubio et al. 2013). Urban areas are increasingly likely to encroach upon or surround protected areas as they expand and undermine the capability of these preserves to buffer effects of human disturbance (McDonald et al. 2009; Wade and Theobald 2010). Conservation planning for endangered species requires reliable knowledge of distribution, abundance, and habitat and landscape associations and should include impacts of land use change caused by human disturbance and development.
The golden-cheeked warbler (Setophaga chrysoparia) is a federally endangered Neotropical migrant songbird that breeds exclusively in the Ashe juniper (Juniperus ashei) and oak (Quercus spp.) woodlands of central Texas (USFWS 1992; Ladd and Gass 1999). Greater than 90 % of the global population of golden-cheeked warblers breeds on private land (Collier et al. 2010; North American Bird Conservation Initiative 2013), which renders this species especially vulnerable to increasing land use change, habitat loss, and fragmentation. The entire breeding range is rapidly becoming urbanized and substantial amounts of potential habitat have been converted to other land uses since 2000 (Duarte et al. 2013). The human population of the Austin metropolitan area, where we conducted our study, has more than doubled since the golden-cheeked warbler was listed under the Endangered Species Act in 1990 (U. S. Census Bureau 2012). These changes emphasize the need to understand relationships between abundance and landscape features affected by urbanization. Despite substantial research on this species since its listing, there is a dearth of information regarding the response of golden-cheeked warblers to urbanization. Coldren (1998) investigated golden-cheeked warblers’ response to various habitat edges and found that territory size and distance to edge were greatest for territories closest to human disturbance (compared to other habitat features); within small patches (<32 ha), golden-cheeked warblers selected against edges of medium- to high-human use, including residential development. Reidy et al. (2009) documented negative effects of open edge but not urban land cover, road density, or building density on nest survival. Robinson (2013) reported a greater minimum patch size threshold for territory establishment, pairing success, and territory success in an urban landscape than a rural landscape. DeBoer and Diamond (2006) reported higher occupancy rates of golden-cheeked warblers in wooded patches away from human development.
Previous studies investigating habitat associations of golden-cheeked warblers have typically focused on coarse landscape measures such as patch size and total amount of woodland cover (Magness et al. 2006; Butcher et al. 2010; Mathewson et al. 2012; Collier et al. 2013), likely because these data are remotely sensed, readily updateable, and cover large spatial scales. However, coarse-scale landscape attributes may fail to identify important habitat relationships at finer scales and may result in erroneous density estimates if substantial within-patch heterogeneity exists that is not addressed through sampling or modeling. Recent work has shown golden-cheeked warblers respond to differences within forest types and forest structure broadly considered to be golden-cheeked warbler habitat. Golden-cheeked warbler density and productivity were greater in mixed juniper–oak forest than in juniper-dominated forest on Fort Hood, Texas (Peak and Thompson 2013, 2014). Golden-cheeked warbler density on Balcones Canyonlands National Wildlife Refuge, Texas, peaked at a local ~70:30 ratio of juniper to broadleaf canopy cover (Steve Sesnie, USFWS, unpublished data). Additionally, golden-cheeked warbler occupancy was better predicted by coarse-scale forest structure measures that were refined by inclusion of LiDAR data (Farrell et al. 2013). DeBoer and Diamond (2006) reported golden-cheeked warbler occupancy was positively related to slope steepness, forest interior, and canopy height. Of the few published studies reporting density of golden-cheeked warblers, none has focused on density–habitat relationships in an urban landscape. On Fort Hood, situated in a rural landscape, golden-cheeked warbler predicted density was reported as 0.39 males ha−1 (95 % CI 0.34–0.45) within wooded vegetation types co-dominated by Ashe juniper (Peak and Thompson 2013), whereas Collier et al. (2013) reported an average predicted density of 0.14 males ha−1 (95 % CI 0.08–0.23) for the live-fire region within Fort Hood across all vegetation types sampled. Mathewson et al. (2012) predicted an average range-wide density of 0.23 males ha−1 (95 % CI 0.20–0.25) based on relationships between occupancy and coarse landscape attributes within wooded habitat patches delineated across the known breeding range. Our understanding of density distribution across habitats within the breeding range is hindered by the differences in sampling and analytical approaches employed by these studies.
Obtaining unbiased and broadly applicable estimates of density and habitat associations of golden-cheeked warblers, and songbirds in general, has been hampered by the difficulty in applying models to counts of breeding birds (Peak 2011; Hunt et al. 2012; Warren et al. 2013). Monitoring forest songbirds often requires counting primarily acoustic signals from individual territorial breeding males to estimate male density in an area (Brewster and Simons 2009; Hunt et al. 2012). Counts based on point-count surveys are considered an index of the actual population present at a given site because some portion of the population is not present on the breeding grounds during the time of the survey (i.e., probability of presence), is present but does not signal its presence during a survey (i.e., availability bias), or signals but is not detected by the surveyor (i.e., perceptibility bias) (Rosenstock et al. 2002; Elphick 2008; Nichols et al. 2009). This “imperfect detection” may not affect the interpretation of results as long as the ratio of detection and counts are unbiased or relatively constant (Johnson 2008), but several studies have shown that detection probability often varies by observers (Diefenbach et al. 2003; Alldredge et al. 2007; Simons et al. 2007; Reidy et al. 2014), by habitat (Pacifici et al. 2008; Amundson et al. 2014), with weather conditions (Simons et al. 2007; Amundson et al. 2014), and through time (Selmi and Boulinier 2003; Diefenbach et al. 2007). Further, if detection probability differs between habitats but is not accounted for, differences in detection may be misattributed to species habitat associations (Amundson et al. 2014). Monitoring programs are increasingly incorporating various methods to account for detection bias (e.g., double observer, distance sampling, repeated surveys; Nichols et al. 2000; Buckland et al. 2001; Royle 2004; Nichols et al. 2009), and recent analytical advancements, namely hierarchical N-mixture modeling (Royle 2004), have facilitated simultaneous estimation of detection probability and population density from spatially replicated wildlife surveys. However, evaluation of model performance is typically non-existent or based on simulated data because the underlying quantity being estimated (i.e., true abundance or density) is usually not known (Cassey and McArdle 1999; Royle 2004; Amundson et al. 2014). Peak (2011) and Hunt et al. (2012) demonstrated point-count surveys may be unreliable predictors of actual observed density of golden-cheeked warblers. Thus, any estimate of density should be interpreted with caution and careful attention should be paid to model validation.
We assessed effects of local habitat and landscape factors on the distribution and density of golden-cheeked warblers on the Balcones Canyonlands Preserve (BCP), Texas, which is located in a rapidly urbanizing landscape. Our objectives were to: (1) determine relationships between occupancy and density of golden-cheeked warblers with remotely sensed habitat and landscape attributes; and (2) produce a spatially explicit density map for the BCP to provide baseline data for population modeling. Our approach was novel and an improvement over previous studies because we accounted for multiple sources of detection bias and compared predicted density with actual density determined from re-sighting color-banded birds on intensively monitored plots as part of our model validation.
Methods
Study area and study species
We conducted this study across the BCP, situated along the eastern edge of the Edwards Plateau (Fig. 1). The BCP is a system of preserves located in western Travis County, Texas, and encompasses approximately 12,294 ha (based on ownership as of May 2014). The BCP is the result of a multi-agency conservation effort operating to preserve and protect habitat of endangered and rare species while allowing for urban expansion and development on surrounding unprotected land. The BCP represents the largest protected area and ~18 % of total protected potential habitat in the golden-cheeked warbler recovery region 5 (USFWS 1992; Fig. 1), which covers the area west of Austin, Texas (Groce et al. 2010). This region experienced the greatest rate of habitat fragmentation range-wide from 2000 to 2010, resulting in increased edge and smaller forest patches (Duarte e al. 2013). While much of the BCP is closed to the public, some areas allow public access and trails. Land use history across the BCP includes extensive logging with subsequent goat and cattle ranching (Travis County and City of Austin 2015). Currently, management for the golden-cheeked warbler within the BCP includes protecting and restoring soils and plant diversity, hog and deer control, invasive plant removal, preventing the spread of oak wilt, and protecting and monitoring boundaries and internal infrastructure corridors (Travis County and City of Austin 2015). The preserve consists primarily of Ashe juniper and juniper–oak woodlands. Dominant tree species include Ashe juniper, Texas red oak (Q. buckleyi), plateau live oak (Q. fusiformis), shin oak (Q. sinuata var. breviloba), cedar elm (Ulmus crassifolia), escarpment black cherry (Prunus serotina var. exima), and Texas ash (Fraxinus texensis). Common understory species include Carolina buckthorn (Frangula caroliniana), yaupon holly (Ilex vomitoria), red buckeye (Aesculus pavia var. pavia), Mexican buckeye (Ungnadia speciosa), Lindheimer silk-tassel (Garrya ovata var. lindheimeri), and elbowbush (Forestiera pubescens) (City of Austin et al. 2014).
a Point count locations (black circles) and intensive monitoring plots (black outline) surveyed for male golden-cheeked warblers across Balcones Canyonlands Preserve (BCP; color-coded to show 9 patches used for spatially balanced sampling) during April and May 2011–2014. The BCP is located in golden-cheeked warbler recovery region 5 (asterisk in inset of Texas with recovery regions outlined), along the eastern edge of the Balcones escarpment; b dominant land cover categories in and around the BCP; and c predicted density across the BCP. Scale bar in km
Golden-cheeked warblers breed in the juniper and juniper–oak (mixed) forests found in central Texas (USFWS 1992; Ladd and Gass 1999) and spend the winter in pine-oak woodlands in montane areas of Central America (Rappole et al. 2000; Komar et al. 2011). Females build nests primarily composed of peeling bark from mature Ashe junipers, hence the presence of mature junipers is required for nesting habitat. However, golden-cheeked warblers are not found across the range of Ashe junipers, which extends into Oklahoma, Arkansas, and Missouri in discrete populations. They are confirmed to breed in only 27 counties in the Edwards Plateau and Cross Timbers ecoregions (Groce et al. 2010). Golden-cheeked warblers forage in a variety of tree species, including junipers and a variety of oaks (Ladd and Gass 1999). Golden-cheeked warbler occupancy is highest in the eastern and southern portion of their range (Collier et al. 2013), and this area experienced the highest human growth rate in the United States since 2010 (US Census Bureau 2012).
Avian surveys
We randomly placed a point grid with 250-m spacing over the entire BCP in ArcMap (ver. 9.3, ESRI, Redlands, CA, USA), resulting in random-systematic coverage of the study area. We eliminated points that were <50 m from major roads because the survey plot would be partly composed of roadway and this proximity decreased our ability to hear singing birds over road noise. We did not exclude points based on a priori expectations of habitat suitability or occupancy. We divided our point grid into non-overlapping transects consisting of 8–15 points based on topography and access. We divided the BCP into nine patches based on patch connectivity (patches were defined by watersheds and highways; Fig. 1) and randomly selected a similar portion of transects each year from each patch to ensure spatially balanced sampling across BCP occurred each year. Each transect was surveyed only once during the study period.
We conducted 5-min, unlimited-radius point count surveys from mid-April to mid-May, 2011–2014. We chose this sampling period because it allowed the entire population to arrive and settle on territories and males were still singing frequently. It also minimized potential population fluctuations that occur within the breeding season due to intraseasonal movement (J. Reidy, University of Missouri, and City of Austin, unpublished data), which would have violated the assumption of population closure needed for estimating density (Nichols et al. 2009). Surveys were conducted from 10 min post-sunrise until ~1100 CDT when temperatures were >10 °C, winds <19 kph, and there was no or light precipitation. We recorded weather information (wind speed using the Beaufort scale, temperature, cloud cover, and an index of precipitation) and point locations in Universal Transverse Mercator (UTM) coordinates prior to starting surveys; this brief delay served as a settling down period for any observer-induced movement. We recorded time of, distance to, and type of (e.g., song, call, visual) initial detections of male golden-cheeked warblers during surveys. We measured distances to each detected bird with laser range-finders (Bushnell Yardage Pro, Bushnell, Overland Park, Kansas, USA), but occasionally estimated distance due to dense vegetation or steep topography. Points were surveyed once by one of eight observers (two per year). All observers were trained in species identification and distance estimation. Observers recorded multiple individuals at a point only if they were confident they were unique individuals (e.g., simultaneous detections) to avoid double-counting.
Landscape and habitat metrics
We derived covariates from two remotely sensed products, the Texas Ecological Systems Phase 1 Vegetation Classification (TESP1) and light detection and ranging imagery (LiDAR), because we needed spatially continuous maps of covariate values and we desired products that could be readily updated and easily replicated for future comparisons. TESP1 provides detailed vegetation classification for central Texas; it was derived from 10-m resolution satellite imagery from 2005 and 2006 by refining land cover categories with ancillary data such as slope and aspect, landscape position, hydrology, soil, roads, and cities data (Texas Parks and Wildlife 2012). LiDAR is a remote sensing technology that measures distance by illuminating a target with a laser and analyzing the reflected light (Sexton et al. 2009) and can be used to determine vegetation height (Hill and Thomson 2005; Sexton et al. 2009). We obtained 2-m resolution LiDAR data acquired during 206 flight lines of standard density (1.4 m ground sample distance) from 20 missions in January 2012. The overlap between flight lines was removed to provide a homogeneous coverage and the coverage was classified to extract a bare earth digital elevation model (DEM). Canopy height was calculated by subtracting the minimum elevation values (at 2.5 m resolution) from the maximum elevation values (at 1 m resolution) (W. Simper, Travis County Natural Resources, unpublished data).
We calculated landscape metrics in ArcMap (ver. 10.0, ESRI, Redlands, CA, USA). We intersected the LiDAR and TESP1 data layers to create a higher resolution (2 m) and more current map (2012) of land cover. We considered a 10-m pixel to be wooded or tree canopy if it was classified as a wooded habitat in the TESP1 layer and ≥50 % of 2-m pixels in the LiDAR layer had vegetation height ≥3 m. We chose 3 m because habitat on the preserve is typified by trees averaging ~5 m tall (Table 1), but across the breeding range, suitable canopy may be shorter; thus, based on our knowledge, we considered 3-m to be the minimum height used as breeding habitat. We calculated the proportion of land cover types within a 100-m and 1-km radius of each point. We evaluated variables in a 100-m radius (local habitat factors) to correspond with the area surveyed by a point count and because it is approximately the size of a birds’ territory and at a 1-km radius (landscape factors) to measure landscape-level habitat composition and structure. We also chose these scales to correspond with a similar study conducted on Fort Hood (Peak and Thompson 2014). We computed mean canopy cover, mean canopy height, and open edge density within the 100-m radius around points, and urban edge density within the 1-km radius around points. We considered urban edge to be the interface between urban land cover and all wooded habitats; we defined open edge as the interface between all wooded and all non-wooded habitat types (excluding urban). We chose to evaluate urban edge density at the 1-km scale only because there was very little urban edge within 100 m of points. We chose to examine open-edge density at the 100-m scale because we were interested in evaluating local effects of fragmentation within wooded habitat, and preliminary examination of the aggregation index, a measure that describes the connectedness of landscape classes (He et al. 2000), indicated that at large scales our forests were continuous rather than patchy or fragmented.
Actual density
We intensively monitored golden-cheeked warblers on 18 plots distributed across the BCP and that represented a diversity of habitat types within the BCP. Most plots (67 %) were ~40 ha and established as part of a long-term monitoring program prior to this study. Six additional plots, ranging in size from 27 to 180 ha, were surveyed to capture additional variation in landscape composition and habitat structure; these plots were mostly constrained by property boundaries. We captured territorial adult golden-cheeked warblers in 6-m mist-nets using pre-recorded playback of golden-cheeked warbler songs. We placed 2–3 colored bands and a numbered aluminum band issued by the U.S. Geological Survey on legs of unbanded individuals. We re-sighted color-banded birds, recorded locations of banded and unbanded birds, and documented reproductive activity at least twice a week from 15 March through 25 May, and at least once per week from 25 May through 15 June 2011–2014. Survey effort was ~5.37 h/ha (range 0.4–9.0 h/ha) and survey intensity was based on plot density such that similar effort was expended per territory. We considered a male territorial if it was present on the plot for ≥3 weeks and delineated territories by bounding territorial observations as minimum convex polygons in ArcMap 10.0 (City of Austin et al. 2014). The number of territorial males present on each plot reached an asymptote by the first week of April; therefore, we have high confidence in the number of territorial males present on our plots. We calculated plot density as the number of territories fully within the plot boundaries plus one-half of the territories partially within the plot boundaries divided by the area of the plot (Verner 1985; City of Austin et al. 2014). We compared the mean actual density for each plot from the 4 years (2011–2014) to model-based predicted density for each plot to inform model selection and base inference.
Data analyses
Amundson et al. (2014) created a hierarchical extension of a combined distance-sampling and time-removal model (Farnsworth et al. 2005) that can simultaneously estimate detection probability and density, and incorporate covariates for each model component. We used this approach to create a hierarchical Bayesian N-mixture model to evaluate the effects of landscape factors on golden-cheeked warbler density and predict density across the BCP (cf., Royle 2004; Amundson et al. 2014). We included distance from the observer to each bird detection (i.e., distance sampling; Buckland et al. 2001) and time elapsed between the beginning of the survey and each unique detection (i.e., time removal; Farnsworth et al. 2002, 2005) to adjust counts for multiple sources of detection bias. The ability of an observer to hear a bird song decreases as distance to the bird increases and this heterogeneity in detection is referred to as perceptibility bias (p d; Nichols et al. 2009). Perceptibility bias can lead to severe underestimation of actual density and often varies by the hearing ability of the observer, habitat at the survey point, and wind and weather conditions during the survey (Alldredge et al. 2007; Simons et al. 2007; Pacifici et al. 2008; Amundson et al. 2014). Traditional distance sampling assumes each individual is available to be detected, i.e., all males present within the focal area sing at some point within the count period. However, there is ample evidence that availability (p a), the probability a male sings during a survey, is less than one, is not constant across time and space, and should therefore be included in models predicting density (Wilson and Bart 1985; Scott et al. 2005; Diefenbach et al. 2007; Amundson et al. 2014). We accounted for availability bias using Farnsworth et al.’s (2002) time-removal method that divides the survey period into discrete time intervals and counts the number of unique individuals that initially sing during each interval. Similar to the population removal model of Zippin (1958), counts in each time interval are fit in a regression; thus, the proportion of the population that never sang during the survey can be estimated. We modeled density (\(\widehat{D}\); abundance per point divided by area surveyed) adjusted for detection (p a × p d) using a Poisson regression. We incorporated a zero-inflation term, z, to reduce bias that can result from a large number of zero counts (Martin et al. 2005; Kéry and Schaub 2012; Amundson et al. 2014). Zero counts can arise if the species did not occur at the point during the study (i.e., true zeros) or if the species occurs at the point but was not present or detected during the study (i.e., false zeros; Martin et al. 2005). Zero-inflation first models counts as a Bernoulli draw to estimate the proportion that are true zeros. Counts not assigned as true zeros are then modeled using a Poisson distribution and may take a zero value. This approach allowed us to separate zero counts arising from surveys in areas likely unsuitable for golden-cheeked warblers and model the density related to our variables of interest (Rathbun and Fei 2006).
We truncated bird observations at a maximum distance of 100 m and binned remaining observations into 4 unequal distance bins (40, 60, 80, 100 m) such that each bin would have a similar number of detections (Buckland et al. 2001; Amundson et al. 2014). We modeled the scale parameter σ, and shape parameter γ, using a hazard rate function because preliminary analyses indicated greater support for the hazard function than a uniform or half-normal function for estimating p d (Buckland et al. 2001). We included each observer (OBS) as a random effect, and fixed effects for wind (WIND; ≤ or >2 on the Beaufort scale) and the proportion of the surveyed area that contained juniper woodland within a 100-m radius of the point (JUN100m) because we hypothesized increasing wind or juniper density (due to the thickness of the foliage) decreased p d. We modeled availability using 5 equal 1-min time intervals and included time of day at the start of the survey (TOD), day of year (DOY), and temperature at the start of the survey (TEMP) as potential effects on p a.
To address the issue that two points along the same transect are more likely to have similar densities than points on different transects, we partially accounted for spatial autocorrelation among points within transects within sites using transect-specific intercepts nested within sites. We originally evaluated random and fixed year effects on N, but found that including these effects resulted in poor model fit (Bayesian P > 0.9). Each year, two novel observers conducted surveys, thus, because the model is hierarchical, observer effects on the perceptibility portion of the model were confounded with temporal variation in density. We omitted year effects on density, but recognize that some temporal variation accounted for in the observation components of the model (i.e., temporally varying covariates) is likely due to unaccounted for variation in density from year to year.
We constructed six candidate models that included landscape and local habitat covariates for the zero-inflation and density portions of the model that we hypothesized were important and that received support in other studies (DeBoer and Diamond 2006; Magness et al. 2006; Farrell et al. 2013; Peak and Thompson 2013) and in our preliminary analyses (City of Austin et al. 2014). We evaluated multi-collinearity of covariates and did not include variables in individual models that resulted in tolerance values <0.4 (Allison 1999). We included two linear covariates that represented generic forest characteristics to evaluate factors influencing point occupancy in all six candidate models: the proportion of area within a 1-km radius composed of juniper and mixed woodland/forest (TOTFOR1km) and the percent of forest canopy cover in a 100-m radius (CC100m). We chose these broad metrics because point occupancy is likely more associated with general forest cover whereas density may be related to the relative proportion of forest types. We included different combinations of landscape and local effects on density in the six candidate models. We evaluated linear and quadratic effects of proportion of area in juniper forest (JUN1km) and mixed forest (MIX1km), and linear effects of proportion of area in urban land cover (URBAN1km) or urban edge density (URBANEDGE1km) in a 1-km radius. We considered linear effects of open edge density (OPENEDGE100m), proportion of area in juniper (JUN100m) and mixed woodland (MIX100m), and mean canopy height (HT100m) in a 100-m radius (Table 1). We a priori predicted that density may peak at some intermediate value of each forest type at the landscape scale because golden-cheeked warblers require junipers for nesting material and oaks for foraging; therefore, we chose to include quadratic effects of JUN1km and MIX1km at the landscape scale. We predicted that golden-cheeked warbler density would respond linearly to the other variables (positively to JUN100m, MIX100m, and HT100m, and negatively to URBAN1km, URBANEDGE1km, and OPENEDGE100m) based on previous studies (DeBoer and Diamond 2006; Peak and Thompson 2013).
We implemented the models using JAGS version 3.4.0 (Plummer 2003) called remotely from the JagsUI package in R (vers. 3.1.1 Patched, R Core Team 2014). We standardized all continuous variables to a mean = 0 and SD = 1 to facilitate model convergence. We assigned uninformative priors to random and fixed effects and assessed convergence of model parameters similar to Amundson et al. (2014). We assigned the hazard function shape parameter γ to a uniform prior distribution between 0.01 and 20. Models reached convergence after 50,000–100,000 iterations from 3 Markov chains with the first 80 % discarded as burn-in. We retained every 10th remaining iteration (i.e., thin). We evaluated fit of the p d and p a portions of the model using Bayesian P values (Gelman et al. 1996) where values near 0 or 1 indicate poor fit (Kéry 2010). We present mean parameter estimates ±95 % credible intervals (CI).
Density distribution map
We compared our model-based predictions of density to mean actual densities on the 18 plots for which we mapped territories of color-banded birds to inform selection of the best model to produce a spatially explicit density map for the BCP. We resampled the 10-m resolution GIS layers for each covariate to represent mean values of covariates for 180 × 180 m pixels (3.2 ha). These pixels approximated the area surveyed during point counts and the average golden-cheeked warbler territory size (City of Austin, unpublished data) and therefore avoided any bias that could result from applying models derived from the point counts to finer resolution pixels. We generated density estimates for each pixel using the model of interest, intersected pixel estimates with the boundaries of the 18 plots, and calculated mean predicted densities and 95 % confidence intervals for each plot. We evaluated models by comparing predicted densities to mean actual densities based on R 2, mean squared error, and mean absolute error for each model and then selected a best model for inference based on these multiple criteria. We used the best model to predict density for all pixels in the BCP and mapped density values to demonstrate geographic patterns. We plotted loess-smoothed lines (α = 1.0, λ = 1; Jacoby 2000) representing the relationships of predicted occupancy or density and observed values of supported covariates across all pixels in the BCP to visually demonstrate landscape relationships.
Results
We conducted point count surveys at 1507 points and censused 18 plots annually from 2011 to 2014 (Fig. 1). We surveyed a wide range of local and landscape conditions with total forest cover ranging from 4 to 100 % within 100 m of points and 12–72 % within 1 km of points. Our survey points and plots were generally representative of the BCP landscape with similar means and ranges among survey points, monitoring plots, and the 3766 180 × 180 m pixels that made up the entire BCP (Table 1). The intensive plots had slightly greater canopy cover and canopy height, and less open edge on average than the survey points and the entire BCP.
We detected 603 singing male golden-cheeked warblers (n2011 = 122, n2012 = 134, n2013 = 135, n2014 = 212) within a 100-m radius of all points. At least one golden-cheeked warbler male was detected at 500 points and zero males were detected at the remaining 1007 points. We banded 70 % (range 0–100 %) of known territorial males on 18 plots from 2011 to 2014. We mapped 0–28 territories/plot and a total of 247, 191.25, 180.5, and 181 territories in 2011, 2012, 2013, and 2014, respectively. Mean actual plot densities across the 4 years were 0.01–0.48 males ha−1 (Fig. 2). Mean actual density across all 18 plots ranged from 0.22 to 0.23 (coefficient of variation = 1.5 %) while variation within plots across years was more substantial (mean coefficient of variation = 31 %); nevertheless the rank order of plots ranked by density did not change among years.
The fit of the models varied but all six models generally fit the data adequately (Bayesian P values = 0.66–0.90). Our comparison of model-predicted densities for pixels within the 18 plots and actual plot densities resulted in R 2 >0.67, mean absolute error <0.105, and mean squared error <0.012 across the six models (Table 2). Parameter estimates from all six models generally represented the same landscape and habitat effects on golden-cheeked warbler density; density was positively associated with mature forest habitats and negatively associated with open edge density within 100 m, had mixed relationships with forest habitats (mixed forest = positive, juniper forest = negative), and was negatively associated with measures of urban edge and total urban area within 1-km of survey points (Table 3). We chose model 3 for further inference because it had the greatest R 2 with actual densities, lower than average prediction error, and inclusion of JUN100m, MIX100m, and HT100m made it the most relevant of the top three models to managers (the other models did not include all three of these variables; Table 2). Credible intervals for densities derived from model 3 overlapped mean actual densities at 15 of the 18 plots and predicted densities within 0.05 males ha−1 for eight plots; predicted densities for three plots were higher than mean actual densities (Fig. 2). There was a tendency to overestimate density more at lower density plots than higher density plots; mean absolute error based on plots below and above the median observed density was 0.11 and 0.04, respectively.
Mean survey point occupancy was 0.83 (95 % CI 0.78, 0.90), indicating the majority of our survey points were considered suitable for golden-cheeked warblers. Point occupancy was positively associated with the amount of CC100m (β = 1.93, 95 % CI 1.14, 2.96; Fig. 3a) and TOTFOR1km (β = 3.73; 95 % CI 2.10, 5.98; Fig. 3b). Mean availability (p a) was 0.90 (95 % CI 0.87, 0.93) and did not vary by TOD (β = −0.04; 95 % CI −0.22, 0.15) or TEMP (β = 0.12; 95 % CI −0.08, 0.32). Availability increased marginally with DOY (β = 0.18; 95 % CI −0.01, 0.36). Mean detectability (p d) was 0.60 (95 % CI 0.53, 0.65) and varied by OBS (Table 4), but not JUN100m (β = 0.02, 95 % CI −0.01, 0.06) or WIND (β = 0.03, 95 % CI −0.05, 0.10).
Predicted density was strongly influenced by local habitat features, increasing with a greater amount of JUN100m (β = 0.24, 95 % CI 0.07, 0.43; Fig. 4a) and MIX100m (β = 0.24, 95 % CI 0.06, 0.44; Fig. 4b), and greater HT100m (β = 0.19, 95 % CI 0.04, 0.33; Fig. 4c), and decreasing with OPENEDGE100m (β = −0.14, 95 % CI −0.27, −0.01; Fig. 4d). Relationships between predicted density and landscape scale factors were weaker. Density had a weak positive relationship with the amount of MIX1km (β = 0.07, 95 % CI −0.15, 0.30), and a weak negative relationship with JUN1km (β = −0.17, 95 % CI −0.39, 0.05) and URBAN1km (β = −0.18, 95 % CI −0.42, 0.06; Fig. 5) at the 1-km scale.
Loess-smoothed trends in predicted densities (solid black line) of male golden-cheeked warblers in relation to a proportion of juniper forest cover, b proportion of mixed forest cover, c canopy height, and d open edge density; all measures are at the 100-m scale. Dashed lines represent 95 % credible intervals
Mean predicted golden-cheeked warbler density was 0.23 males ha−1 (95 % CI 0.19, 0.28) for all pixels in the BCP but averaged 0.32 males ha−1 for pixels dominated (>70 %) by mixed and juniper woodland. Predicted densities were greatest in the northeastern portion of the BCP and lowest in the southwestern (Fig. 1). Mean golden-cheeked warbler density on BCP for 2011–2014 based on the actual observed plot densities, weighted by plot area, was 0.17 (95 % CI 0.14, 0.20), which was similar to our predicted density by model 3 minus mean absolute error, or 0.23–0.07 = 0.16 males ha−1.
Discussion
We provided a rigorous evaluation of landscape and habitat relationships for predicted density of golden-cheeked warblers and demonstrated a hierarchical pattern of habitat use in an urban landscape. Our estimate of point occupancy was high (83 %), indicating the majority of our points, and hence the BCP, were considered suitable for golden-cheeked warblers. However, we only detected singing males at approximately one-third (33 %) of our points, leaving 50 % of our points as suitable but not known to be occupied. Our overall detection probability was ~54 % (p d × p a), so it is likely some unoccupied sites had undetected males present. This result suggests that habitat within the BCP is not be saturated, and either could support more golden-cheeked warblers or that the population is being limited by something other than the local and landscape features we evaluated.
We found strong support for effects of landscape composition and local structure on point occupancy. The total amount of juniper and mixed woodlands at the landscape scale and canopy cover at the local scale were highly predictive of habitat occupancy. Local canopy cover and total landscape forest cover averaged 79 and 48 %, respectively for survey points with at least 0.5 probability of occupancy. Conversely, the probability of golden-cheeked warbler occupancy in the southern portion of the breeding range did not exceed 0.5 until forest cover was at least 80 % at multiple spatial scales (Magness et al. 2006). Golden-cheeked warbler occupancy at Fort Hood was similarly related to local canopy cover derived from LiDAR, although they used 1-m high to define canopy cover because they were modelling golden-cheeked warbler and black-capped vireo (Vireo atricapilla; an endangered shrub-nesting songbird) occupancy (Farrell et al. 2013).
Predicted density was influenced most strongly by forest composition and fragmentation at the local scale. Although the amount of mixed woodland and juniper woodland had similar estimated effects on density, we found that density showed a much stronger positive response to increasing amounts of mixed woodlands than juniper across the landscape (Fig. 4). The differential response to the two forest types was likely a result of the community composition of habitats dominated by each forest type. For example, juniper woodlands generally had lower canopy height and more open edge than mixed woodlands. Canopy height also exerted a strong influence on golden-cheeked warbler density, indicating more mature woodlands attract more males. We also documented a strong negative effect of open edge density on golden-cheeked warbler density. This may be due to perceived lower habitat quality, fewer nest sites, foraging trees or optimal trees to sing from, or lower nest success because of increased abundance of edge-adapted predators (Reidy et al. 2008; Peak and Thompson 2014).
We were unable to estimate annual variation in density with our model due to confounding with observer effects. Despite variation in density in individual plots among years (CV = 31 %), we found little variation in mean actual density (1–3 % change in mean density per year). This suggests that there was movement of birds within the BCP from year to year, but that annual variation in population size during our study was relatively low. Because sampling intensity was similar across all 4 years of our study, golden-checked warbler density likely responded, on average, to the landscape and habitat factors we studied. Regardless, seasonal and annual factors affecting warbler density warrant further consideration.
We provide further evidence that golden-cheeked warblers benefit from large, contiguous patches of mature woodlands, which corroborates the strong effect of forest types and edge documented on Fort Hood (Peak and Thompson 2013). We expect that large-scale landscape composition was less influential on golden-cheeked warbler density because our model accounted for landscape effects on point occupancy. We did find a trend for golden-cheeked warbler density to decrease with greater urban land cover and this effect is likely to become more pronounced as the area around the BCP continues to develop. As the human population within the Austin, Texas, metropolitan area continues to expand west into the area surrounding the BCP, juniper and mixed woodlands are rapidly being replaced by urban development and USFWS (1996) projects a loss of >70 % of the golden-cheeked warbler’s habitat in western Travis County. Our results suggest the large intact patches of juniper and mixed forest in the BCP currently buffer the effects of landscape-level urbanization on golden-cheeked warbler density, but as urbanization and fragmentation intensify, negative impacts to breeding density will likely increase.
Our observed habitat associations and range of predicted densities were similar to those documented in Peak and Thompson (2013), which reported golden-cheeked warbler density was positively related to mixed forest cover and juniper forest cover in the 100-m radius and total forest cover in the 1-km radius and negatively related to edge density around points. Despite differences in design and analysis between our study and theirs, we believe strong inference can be made at broad spatial scales between golden-cheeked warbler density and landscape metrics based on the patterns that emerged from these different landscapes. Our mean predicted density for wooded habitat within BCP was lower than predicted density for wooded habitat within Fort Hood (Peak and Thompson 2013). The woodland composition at Fort Hood was also characterized by greater amounts of mixed forest cover (68 %) and less juniper cover (16 %) at the local scale and more total forest cover (73 %) at the landscape scale than our study area (Peak and Thompson 2013), so we might expect their landscape to support more birds. However, it is difficult to make a direct comparison due to differences in data collection and analysis.
Density estimation accounting for detection probability has become increasingly common over the past decade, and model development has resulted in a novel framework that allows simultaneous modeling of availability, perceptibility, occupancy, and density and allows covariates to be modelled on each portion (cf., Royle 2004; Amundson et al. 2014). However, for most studies it is unknown if density estimates accounting for detection probability approximate actual breeding population size better than raw counts. Our estimate of availability was high and not affected by day of year, time of day, or temperature. Our sampling window was designed to ensure the golden-cheeked warbler population had arrived on the breeding grounds (takes approx. 1 month), was during the period of high site fidelity (territorial behavior is reduced beginning in mid-May), and occurred during optimal singing conditions (moderate weather and morning hours). However, our estimate of p a is calculated from the 5-min survey data, which may not give a true, unbiased estimate if singing probability is not a random process. Availability of golden-cheeked warblers based on singing within a 5-min sample was 0.64 (SD = 0.26) in a separate study that recorded per-minute singing probability of territorial males for 30–120 min periods, which is much lower than 0.90 arrived at from decomposition of the survey min in this study; further, singing occurred in bouts, or strings, of singing and silence (J. Reidy, unpublished data). Perceptibility was not affected by wind speed (which we also controlled for in our sampling design) or total amount of juniper cover at the local scale, but was influenced by the observer, a well-documented source of detection bias (Simons et al. 2007; Reidy et al. 2014). Our results suggest careful study design reduced several sources of detection bias (e.g., day of year, wind), but spatio-temporal variation in detection still occurred due to surveys being conducted by multiple observers across multiple years and individual heterogeneity in singing rates. Thus, surveys should be designed to minimize sources of detection bias (e.g., conducting surveys at peak breeding so that 100 % of the breeding population is likely present at the point), but for large-scale, multi-year studies utilizing many observers, employing methods that account for one or more sources of detection bias may further improve accuracy of density estimates (Hefley et al. 2013; Kellner and Swihart 2014).
Studies rarely test model performance against sites with known abundance. Although mean predicted density was ~35 % greater than mean actual density measured on 18 plots, actual densities fell within the credible intervals of our predicted densities for 83 % of the intensively monitored plots. Our model performed well at predicting densities in plots within large, contiguous patches of woodland that supported >0.20 males ha−1. Our model validation revealed an interesting trend to overestimate at plots with low densities (<0.20 males ha−1). Overestimation could have occurred because birds are not saturating the habitat at the level expected given landscape relationships or because we were unable to model biological phenomena such as conspecific attraction (Farrell et al. 2012) or forest health. For example, two plots with inflated model-predicted densities supported a network of trails and one of these experienced substantial tree mortality as a result of extreme drought from 2011 to 2012. While we attempted to update land use changes that occurred after the 2005–2006 land cover classification and create a finer-resolution of woodland composition by combining it with the 2012 LiDAR layer, we still used relatively coarse-level metrics in our model. Additional urban effects that we did not model included public access, trail density, trail use, and effects of non-native vegetation. Plots that were overestimated the most tended to be small, isolated, internally fragmented by trails and other canopy gaps, and externally surrounded by low- to medium-density human development. While we identified some plot-specific characteristics that may explain some of the observed overestimation of density, we believe there may be some more general factors that explain differences in intensive plot-based territory mapping versus model-based estimates of pixel densities summarized over a large landscape. Overestimation could partly be because points or pixels that are not suitable are always assigned some non-zero density estimate due to the uncertainty in the Bernoulli realization of the probability of occupancy. While these non-zero estimates are typically very small at the point or pixel-level, estimates are non-trivial when summed across a larger area. Similarly, the model could predict very low densities for pixels that are actually unsuitable habitat and that become non-trivial when summed over a large area. O’Donnell et al. (in press) similarly compared densities estimated from three independent point count studies to actual densities from territory mapping at the plot level within BCP and found all three studies tended to overestimate density, particularly at low density plots. There are a number of factors that could contribute to differences in model-based estimates of density from point counts compared to territory counts from intensive monitoring that are not the result of poor model fit and we consider this is an area for future investigation. Additionally, we believe incorporating finer-scale measures of landscapes and vegetation structure may improve model performance for low density areas.
References
Alldredge MW, Simons TR, Pollock KH, Pacifici K (2007) A field evaluation of the time-of-detection method to estimate population size and density for aural avian point counts. Avian Cons Ecol 2:13
Allison PD (1999) Logistic regression using SAS®: theory and application. SAS Institute, Cary
Amundson CL, Royle JA, Handel CM (2014) A hierarchical model combining distance sampling and time removal to estimate detection probability during avian point counts. Auk 131:476–494
Brewster JP, Simons TR (2009) Testing the importance of auditory detections in avian point counts. J Field Ornithol 80:178–182
Buckland ST, Anderson DR, Burnham KP, Laake JL, Borchers DL, Thomas L (2001) Introduction to distance sampling. Oxford University Press, New York
Butcher JA, Morrison ML, Ransom D, Slack RD, Wilkins RN (2010) Evidence of a minimum patch size threshold of reproductive success in an endangered songbird. J Wildl Manag 74:133–139
Cassey P, McArdle BH (1999) An assessment of distance sampling techniques for estimating animal abundance. Environmetrics 10:261–278
Chace JF, Walsh JJ (2006) Urban effects on native avifauna: a review. Landsc Urban Plan 74:46–69
City of Austin, Travis County, and US Forest Service (2014) 2014 annual report: golden-cheeked warbler (Setophaga chrysoparia) monitoring program, Balcones Canyonlands Preserve. Prepared by City of Austin Water Utility Wildland Conservation Division, Travis County Department of Transportation and Natural Resources, US Forest Service Northern Research Station, Department of Fisheries & Wildlife Sciences, and University of Missouri, Balcones Canyonlands Preserve, Austin, Texas
Coldren CL (1998) The effects of habitat fragmentation on the golden-cheeked warbler. Dissertation. Texas A&M University
Collier BA, Morrison ML, Farrell SL, Campomizzi AJ, Butcher JA, Hays KB, Mackenzie DI, Wilkins RN (2010) Monitoring golden-cheeked warblers on private lands in Texas. J Wildl Manag 74:140–147
Collier BA, Farrell SL, Long AM, Campomizzi AJ, Hays KB, Laake JL, Morrison ML, Wilkins RN (2013) Modeling spatially explicit densities of endangered avian species in a heterogeneous landscape. Auk 130:666–676
Czech B, Krausman PR, Devers PK (2000) Economic associations among causes of species endangerment in the United States. Bioscience 50:593–601
DeBoer TS, Diamond DD (2006) Predicting presence-absence of the endangered golden-cheeked warbler (Dendroica chrysoparia). Southwest Nat 51:181–190
Diefenbach DR, Brauning DW, Mattice JA (2003) Variability in grassland bird counts related to observer differences and species detection rates. Auk 120:1168–1179
Diefenbach DR, Marshall MR, Mattice JA, Brauning DW (2007) Incorporating availability for detection in estimates of bird abundance. Auk 124:96–106
Duarte A, Jensen JLR, Hatfield JS, Weckerly FW (2013) Spatiotemporal variation in range-wide golden-cheeked warbler breeding habitat. Ecosphere 4:152
Elphick CS (2008) How you count counts: the importance of methods research in applied ecology. J App Ecol 45:1313–1320
Farnsworth GL, Pollock KH, Nichols JD, Simons TR, Hines JE, Sauer JR (2002) A removal model for estimating detection probabilities from point-count surveys. Auk 119:414–425
Farnsworth GL, Nichols JD, Sauer JR, Fancy SG, Pollock KH, Shriner SA, Simons TR (2005) Statistical approaches to the analysis of point count data: a little extra information can go a long way. In: Ralph CJ, Rich TD (eds), Bird conservation implementation and integration in the Americas: Proceedings of the third international Partners in Flight conference. US Serv Gen Tech Rep PSWGTR-191, pp 736–743
Farrell SL, Morrison ML, Campomizzi AJ, Wilkins RN (2012) Conspecific cues and breeding habitat selection in an endangered woodland warbler. J Anim Ecol 81:1056–1064
Farrell SL, Colllier BA, Skow KL, Long AM, Campomizzi AJ, Morrison ML, Hays KB, Wilkins RN (2013) Using LiDAR-derived vegetation metrics for high-resolution, species distribution models for conservation planning. Ecosphere 4:42
Gelman A, Meng XL, Stern HS (1996) Posterior predictive assessment of model fitness via realized discrepancies (with discussion). Stat Sinica 6:733–807
Groce JE, Mathewson HA, Morrison ML, Wilkins RN (2010) Scientific evaluation for the 5-year state review of the golden-cheeked warbler. Texas A&M Institute of Renewable Natural Resources, College Station
He HS, DeZonia BE, Mladenoff DJ (2000) An aggregation index (AI) to quantify spatial patterns of landscapes. Landscape Ecol 15:591–601
Hefley TJ, Tyre AJ, Blankenship EE (2013) Fitting population growth models in the presence of measurement and detection error. Ecol Model 263:244–250
Hill RA, Thomson AG (2005) Mapping woodland species composition and structure using airborne spectral and LiDAR data. Int J Rem Sens 26:3763–3779
Hunt JW, Weckerly FW, Ott JR (2012) Reliability of occupancy and binomial mixture models for estimating abundance of golden-cheeked warblers (Setophaga chrysoparia). Auk 129:105–114
Jacoby WG (2000) Loess: a nonparametric, graphical tool for depicting relationships between variables. Electoral Stud 19:577–613
Johnson DH (2008) In defense of indices: the case of bird surveys. J Wildl Manag 72:857–868
Kellner KF, Swihart RK (2014) Accounting for imperfect detection in ecology: a quantitative review. PLoS One 9:e111436
Kéry M (2010) Introduction to WinBUGS for ecologists. Academic Press, New York
Kéry M, Schaub M (2012) Bayesian population analysis using WinBUGS: a hierarchical perspective. Academic Press, New York
Komar O, McCrary JK, Van Dort J, Cobar AJ, Castellano EC (2011) Winter ecology, relative abundance and population monitoring of golden-cheeked warblers (Dendroica chrysoparia) throughout the known and potential winter range. https://tpwd.texas.gov/business/grants/wildlife/section_6/projects/birds/e69_final_report.pdf. Accessed Feb 2015
Ladd C, Gass L (1999) Golden-cheeked warbler (Dendroica chrysoparia). In: Poole A, Gill F (eds) The birds of North America. Cornell Lab of Ornithology, New York
Magness DR, Wilkins RN, Hejl SJ (2006) Quantitative relationships among golden-cheeked warbler occurrence and landscape size, composition, and structure. Wildl Soc Bull 34:473–479
Martin TG, Wintle BA, Rhodes JR, Kuhnert PM, Field SA, Low-Choy SJ, Tyre AJ, Possingham HP (2005) Zero tolerance ecology: improving ecological inference by modelling the source of zero observations. Ecol Lett 8:1235–1246
Marzluff JM (2001) Worldwide urbanization and its effects on birds. In: Marzluff JM, Bowman R, Donnelly R (eds) Avian ecology and conservation in an urbanizing world. Kluwer Academic Publishers, Boston, pp 19–48
Mathewson HA, Groce JE, McFarland TM, Morrison ML, Newnam JC, Snelgrove RT, Collier BA, Wilkins RN (2012) Estimating breeding season abundance of golden-cheeked warblers in Texas, USA. J Wildl Manag 76:1117–1128
McDonald RI, Forman RTT, Kareiva P, Neugarten R, Salzer D, Fisher J (2009) Urban effects, distance, and protected areas in an urbanizing world. Landsc Urban Plan 93:63–75
Nichols JD, Hines JE, Sauer JR, Fallon FW, Fallon JE, Heglund JE (2000) A double-observer approach for estimating detection probability and abundance from point counts. Auk 117:393–408
Nichols JD, Thomas L, Conn PB (2009) Inferences about landbird abundance from count data: recent advances and future directions. In: Thomson DL, Cooch EG, Conroy MJ (eds) Environmental and ecological statistics, vol 3. Springer, New York, pp 201–235
North American Bird Conservation Initiative, U.S. Committee (2013) The State of the Birds 2013 report on private lands. US Depart of Interior: Washington, p 48
O’Donnell L, Farquhar CC, Hunt JW, Nesvacil K, Reidy JL, Reiner W, Scalise JL, Warren CC. Breeding density influences accuracy of model-based estimates for a forest songbird. J Field Ornithol (in press)
Pacifici K, Simons TR, Pollock KH (2008) Effects of vegetation and background noise on the detection process in auditory avian point-count surveys. Auk 125:600–607
Peak RG (2011) A field test of the distance sampling method using Golden-cheeked Warblers. J Field Ornithol 82:311–319
Peak RG, Thompson FR (2013) Amount and type of forest cover and edge are important predictors of golden-cheeked warbler density. Condor 115:659–668
Peak RG, Thompson FR (2014) Seasonal productivity and nest survival of golden-cheeked warblers vary with forest type and edge density. Condor 116:546–559
Plummer M (2003) JAGS: a program for analysis of Bayesian graphical models using Gibbs sampling. Proceedings of the third international workshop on distributed statistical computing, Vienna
R Core Team (2014) R: a language and environment for statistical computing. R Foundation for Statistical Computing, Vienna
Ralph CJ, Geupel GR, Pyle P, Martin TE, DeSante DF (1993) Handbook of field methods for monitoring landbirds. U S For Serv Gen Tech Rep PSWGTR-144
Rappole JH, King DI, Leimgruber P (2000) Winter habitat and distribution of the endangered golden-cheeked warbler (Dendroica chrysoparia). Anim Conserv 2:45–59
Rathbun SL, Fei S (2006) A spatial zero-inflated poisson regression model for oak regeneration. Environ Ecol Stat 13:409–426
Reidy JL, Stake MM, Thompson FR (2008) Golden-cheeked warbler nest mortality and predators in urban and rural landscapes. Condor 110:458–466
Reidy JL, Thompson FR, Peak R (2009) Factors affecting golden-cheeked warbler nest survival in urban and rural landscapes. J Wildl Manag 73:407–413
Reidy JL, Thompson FR, Kendrick SW (2014) Breeding bird response to habitat and landscape factors across a gradient of savanna, woodland, and forest in the Missouri Ozarks. For Ecol Manag 313:34–46
Robinson DH (2013) Effects of habitat characteristics on occupancy and productivity of a forest-dependent songbird in an urban landscape (Thesis). Texas A&M University, Texas
Rodewald AD, Kearns LJ, Shustack DP (2013) Consequences of urbanizing landscapes to reproductive performance of birds in remnant forests. Biol Cons 160:32–39
Rosenstock SS, Anderson DR, Giesen KM, Leukering T, Carter MF (2002) Landbird counting techniques: current practices and an alternative. Auk 119:46–53
Royle JA (2004) N-mixture models for estimating population size from spatially replicated counts. Biometrics 60:108–115
Scott TA, Lee P-Y, Greene GC, McCallum DA (2005) Singing rate and detection probability: an example from the Least bell’s vireo (Vireo belli pusillus). In: Ralph CJ, Rich TD (eds), Bird conservation implementation and integration in the Americas: proceedings of the third international Partners in Flight conference. U S For Serv Gen Tech Rep PSWGTR-191, pp 845–853
Selmi S, Boulinier T (2003) Does time of season influence bird species number determined from point-count data? A capture-recapture approach. J Field Ornithol 74:349–356
Sexton JO, Bax T, Siqueira P, Swenson JJ, Hensley S (2009) A comparison of lidar, radar, and field measurements of canopy height in pine and hardwood forests of southeastern North America. For Ecol Manag 257:1136–1147
Simons TR, Alldredge MW, Pollock KH, Wettroth JM (2007) Experimental analysis of the auditory detection process on avian point counts. Auk 124:986–999
Suarez-Rubio M, Wilson S, Leimgruber P, Lookingbill T (2013) Threshold responses of forest birds to landscape changes around exurban development. PLoS One 8:e67593
Texas Parks and Wildlife Department (2012) Texas ecological systems classification project. https://www.tpwd.state.tx.us/landwater/land/maps/gis/tescp/index.phtml. Accessed Sept 2014
Travis County and City of Austin (2015) Balcones Canyonlands Conservation Plan 2014 annual report (October 1, 2013–September 30, 2014), USFWS regional section 10(a)(1)(B) permit no. TE-788841-2. Available from https://www.traviscountytx.gov/images/tnr/Docs/2014/annual-report-2014.pdf. Accessed Jan 2015
US Census Bureau (2012) Large metropolitan statistical areas—population: 1990 to 2010. Available from http://www.census.gov/compendia/statab/2012/tables/12s0020.pdf. Accessed Feb 2015
USFWS (Fish and Wildlife Service) (1992) Golden-cheeked warbler (Dendroica chrysoparia) recovery plan, Albuquerque
USFWS (Fish and Wildlife Service) (1996) Final environmental impact statement/habitat conservation plan for proposed issuance of a permit to allow incidental take of the golden-cheeked warbler, black-capped vireo, and six karst invertebrates in Travis County, Texas. Prepared by Regional Environmental Consultants (RECON) and USFWS, Albuquerque
US Census Bureau (2012) Large metropolitan statistical areas—population: 1990 to 2010. Available from http://www.census.gov/compendia/statab/2012/tables/12s0020.pdf. Accessed Feb 2015
Verner J (1985) Assessment of counting techniques. Curr Ornithol 2:247–302
Wade A, Theobald DM (2010) Residential development encroachment on U S protected areas. Conserv Biol 24:151–161
Warren CC, Veech JA, Weckerly FW, O’Donnell L, Ott JR (2013) Detection heterogeneity and abundance estimation in populations of golden-cheeked warblers (Setophaga chrysoparia). Auk 130:677–688
Wilcove DS, Rothstein D, Dubow J, Phillips A, Losos E (1998) Quantifying threats to imperiled species in the United States. Bioscience 48:607–615
Wilson DM, Bart J (1985) Reliability of singing bird surveys: effects of song phenology during the breeding season. Condor 87:69–73
Zippin C (1958) The removal method of population estimation. J Wildl Manag 22:82–90
Acknowledgments
We thank W. Dijak, U.S. Forest Service, and W. Simper, Travis County Natural Resources, for assisting with GIS analyses; P. Bullard, J. Edwardson, N. Flood, M. Frye, G. Geier, J. Halka, S. Stollery, and C. Weyenberg for assistance with data collection; the many BCP staff, partners, and volunteers, for collecting the territory mapping data; and G. Connette, C. Handel, R. Peak, J. Pierce, W. Reiner and two anonymous reviewers for comments on a draft of this manuscript. Funding for this research was provided by the City of Austin and USDA Forest Service Northern Research Station. Any use of trade, product, or firm names in this publication is for descriptive purposes only and does not imply endorsement by the U.S. Government.
Author information
Authors and Affiliations
Corresponding author
Ethics declarations
Conflict of interest
The authors have no conflict of interest to report.
Additional information
Birds were banded under Bird Banding Lab Permit Number 23615 and University of Missouri ACUC Number 8383. Other activities were covered under U.S. Federal Permit TE798920-4 and Texas State Permit SPR1111378.
Rights and permissions
About this article
Cite this article
Reidy, J.L., Thompson, F.R., Amundson, C. et al. Landscape and local effects on occupancy and densities of an endangered wood-warbler in an urbanizing landscape. Landscape Ecol 31, 365–382 (2016). https://doi.org/10.1007/s10980-015-0250-0
Received:
Accepted:
Published:
Issue Date:
DOI: https://doi.org/10.1007/s10980-015-0250-0