Abstract
This research investigated differences in households’ receipt of government food assistance through such programs as the Supplemental Nutrition Assistance Program; Special Supplemental Nutrition Program for Women, Infants, and Children; free and reduced school meals; and related local and/or federal programs. With panel data from the Survey of Income and Program Participation that span the Great Recession, differences in the receipt of government food assistance in metro and non-metro areas were identified. Longitudinal random effects models suggest that despite relatively similar levels of food insecurity in 2005 and 2010, a higher proportion of non-metro households received government food assistance. Results also suggest that this assistance gap widened post-recession when government resources were expanded. These results inform a continuing debate about the efficient allocation of resources intended to reduce food hardship disparities, and increase family economic well-being, in metro and non-metro areas.
Similar content being viewed by others
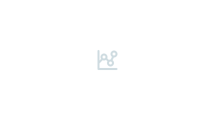
Avoid common mistakes on your manuscript.
Background
An estimated 17.5 million US households, or 14.3% of all households, experienced food insecurity at some point during 2013 (Coleman-Jensen et al. 2014). According to the US Economic Research Service, food-insecure households are “at times, uncertain of having, or unable to acquire, enough food for all members because they had insufficient money and other resources for food” (Nord et al. 2008, p. 4). These 17.5 million households include approximately 49.1 million people (Coleman-Jensen et al. 2014), establishing food-related hardships as a continuing public policy concern. Having insufficient resources for securing food results in households spending nearly one-third (31%) less on food per person than food secure households (Nord et al. 2009).
Despite an extensive literature on the correlates and consequences of food insecurity, a relatively small literature specifically investigates the differences in coping strategies employed by households facing food-related hardships. Just as food insecurity rates vary by geographic location and metropolitan status of the residence area (Coleman-Jensen et al. 2014), the availability and utilization of food assistance programs could also vary. For example, Guo (2012) found that non-metro households are more susceptible to food-insecurity concerns than their metro counterparts. In her investigation of material hardship, Guo (2012) pooled data from the 1996, 2001, 2004 Survey of Income and Program Participation (SIPP) panels to find that households in non-metro areas had a higher probability of reporting having received assistance from informal sources such as a nonprofit organization, friend, or family member in response to material hardship. More recently, Sabia and Nielsen (2015) used the same three SIPP panels to find that residents of non-metro areas were more likely to experience material hardships, including food insecurity, than residents of metro areas. However, because Sabia and Nielsen (2015) focused on the effects of minimum wage policy, more informative findings related to the receipt of food assistance were not investigated. Moreover, neither Guo (2012) nor Sabia and Nielsen (2015) used data that spanned the Great Recession. And, neither specifically investigated the receipt of food assistance. Compounding the need for research into the circumstances of people who receive food assistance is evidence of increasingly concentrated hardship in the US. For example, Farrigan and Parker (2012) found that hardship is most pronounced in the non-metro South and varies by race/ethnicity and other household characteristics.
To better understand possible differences in the provision of food assistance in metro and non-metro areas before and after the Great Recession, our research investigates whether households in metro and non-metro areas received food assistance from government sources (e.g., Supplemental Nutrition Assistance Program, Special Supplemental Nutrition Program for Women, Infants, and Children, free and reduced school meals, other government assistance) at different rates.
Literature Review
Food Assistance Programs
A variety of support systems operated during the Great Recession to help resource-constrained households meet their food needs, including formal government programs such as the Supplemental Nutrition Assistance Program (SNAP, formerly known as the Food Stamp Program) and the Special Supplemental Nutrition Program for Women, Infants, and Children (WIC). Currently, there are 15 food and nutrition assistance programs operated by the United States Department of Agriculture’s (USDA) Food and Nutrition Service (FNS). The three largest of these programs include SNAP, WIC, and the National School Lunch Program (Coleman-Jensen et al. 2014). Estimated participation rates in at least one of the three programs among food-insecure households are approximately 62%, with 46.2% of food insecure households receiving SNAP benefits (Coleman-Jensen et al. 2014).
Although the main goal of these government food and nutrition assistance programs was to alleviate hunger, these programs did not fully protect households from food insecurity. Some research indicated that households receiving SNAP benefits were more likely to experience food insecurity than households not receiving SNAP benefits (e.g., Jensen 2002; Ribar and Hamrick 2003; Wilde and Nord 2005), other research identified SNAP as instrumental in efforts to alleviate food insecurity (e.g. Gundersen and Kreider 2008; Gundersen and Oliveira 2001; Nord and Golla 2009; Van Hook and Balistreri 2006). Receiving government food assistance remains common in the US; between 40 and 50% of adults utilize SNAP benefits at some point in adulthood (Grieger and Danziger 2011; Rank and Hirschl 2005).
Food Assistance and the Great Recession
The economic recession from December 2007 to June 2009 has been described as the worst economic slump since the Great Depression (National Bureau of Economic Research 2012). Termed the Great Recession, the downturn had severe impacts on unemployment and underemployment, large decreases in wealth, particularly among homeowners who saw values plunge, and declines in consumer consumption (Grusky et al. 2011). US households faced severe financial distress during the recession; poverty rates increased by 1.9% points (6.3 million people) (DeNavas-Walt et al. 2011).
During the Great Recession, SNAP participation rates reached exceptional levels, with the number of households receiving monthly SNAP benefits increasing from approximately 26 million people (1 in 11) in 2007 to 45 million people (1 in 7) in 2011 in the US (Congressional Budget Office 2012). Prior to the recession, the highest participation rates for SNAP were found within areas known for persistent poverty (e.g., Lower Mississippi Delta) (Slack and Myers 2012), but the impacts of the recession were felt in areas of poverty and areas of affluence alike. The US government attempted to curtail the effects of the Great Recession in several ways. The Troubled Asset Relief Program (TARP) of 2008 bailed out the financial sector; the American Recovery and Reinvestment Act (ARRA) of 2009 created a large stimulus package; and the Tax Relief, Unemployment Insurance Reauthorization, and Job Creation Act of 2010 created another large stimulus plan (Grusky et al. 2011). As related specifically to food assistance, there were limited financial expansions to the SNAP program during the recession, but SNAP experienced an increase in caseloads during the recession offsetting the expansions the program saw (Congressional Budget Office 2012; Rosenbaum 2013). Between 2007 and 2011 spending on SNAP benefits increased by 135%, partially due to increase in the number of those eligible and partially due to budget increases that were included in the ARRA (Congressional Budget Office 2012). The increase in caseloads was attributed to the deep economic crisis of the recession as well as the subsequent slow recovery (Congressional Budget Office 2012). Additionally, legislation during the recession increased the maximum benefits for SNAP recipient households by 13.6% (Congressional Budget Office 2012).
Food Assistance and Hardship in Metro and Non-Metro Areas
Though the relationship between the need for food assistance and the presence of food hardship is complicated by endogeneity challenges (e.g., Gregory et al. 2013; Gundersen and Kreider 2008; Gundersen and Oliveira 2001), a household’s food insecurity status has served as a proxy for the need for food assistance. In 2013, 15.1% of non-metro households and 14.1% of households in metro areas experienced food insecurity (Coleman-Jensen et al. 2014), which suggested that the demand for food assistance is similar in metro and non-metro areas. However, the relatively small literature that documents any differences in the likelihood of experiencing food hardship, or the strategies that can be used to cope with food-related hardships when present, offered mixed findings on metro/non-metro differences.
Prior research suggested that the non-metro food environment may be different from the metro food environment in important ways (Sharkey 2009). For example, households in non-metro areas tended to have fewer financial resources to spend on food and live farther from food markets (Morton and Blanchard 2007). For low-income families in persistently poor rural areas, turning to extended families and food banks for assistance may have been more common than receiving government food assistance (Harvey et al. 2002). Recent studies have attempted to move beyond studying individual or household characteristics of those receiving government food assistance to exploring how the characteristics of localities shape participation in food assistance programs (e.g., Goetz et al. 2004; Slack and Myers 2012). For example, high SNAP participation was identified in areas of persistent poverty such as the Lower Mississippi Delta, Central Appalachia, and Texas Borderland (Slack and Myers 2012).
Consumers in rural areas were less likely to participate in formal government food assistance programs than comparable urban consumers (Rank and Hirschl 1993). Rural households in Iowa used community support systems more than social networks of family or friends to protect against food hardship (Morton et al. 2005). Households that felt they could get help from others when needed also were less likely to experience food insecurity (Garasky et al. 2006). In the presence of food-related hardships, low-income mothers in rural areas reported using assistance programs to reduce food hardship (Swanson et al. 2008).
The possible geographic and social isolation that characterize many rural areas has been identified as a risk factor due to reduced access to employment and support services. Geographic and social isolation also constrained both food access and the ability of service providers to reach residents in these areas (Smith and Morton 2009). Still, Guo (2012) found that households living in non-metro areas had a higher probability than metro households of having received assistance from a nonprofit organization, friends, or family to address some form of material hardship. Notably, food hardships were not investigated by Guo (2012), other than the possibility that government food benefits were included in an aggregated set of public assistance possibilities that included Temporary Assistance for Needy Families, SNAP, Medicaid, and Social Security. Local organizations—both public and private—were increasingly becoming the providers of social services (Allard 2009; Heflin and Miller 2012) and there was large variation in how programs are administered (Food and Nutrition Service 2012; Zedlewski and Brauner 1999). Accordingly, it is important that researchers understand whether differences between metro and non-metro areas were associated with differences in the receipt of food assistance.
Theoretical Framework
This research was grounded in the neoclassical household production theoretical framework (Becker 1965; Bryant and Zick 2006; Mincer 1963), which presumes that a household is constrained by its resources (e.g., income, public assistance transfers, informal cash and noncash transfers) and the price of goods (food and all other goods), subject to its tastes and preferences. Household decision makers were assumed to be altruistic with respect to the utility of household members, seeking to maximize resources available and minimize negative or harmful conditions (i.e., hunger). When maximizing the utility of household members, decision makers were assumed to identify the combination of goods and services that offer the household the greatest utility over time. Resources available to maximize the household’s utility function included current and future income, cash and in-kind transfers from government sources, as well as cash and in-kind assistance from informal networks such as social or religious organizations, family members, charitable organizations, and so forth. Tastes and preferences of household decision makers informed the household’s willingness to consume from each of these resources, such that otherwise identically endowed household may maximize utility through very different levels of consumption depending on preferences.
Method
Data
This research used the 2004 and 2008 panels of the Survey of Income and Program Participation (SIPP), a nationally-representative survey of the non-institutionalized, civilian population conducted by the US Census Bureau. Data from the 2004 and 2008 panels corresponded to calendar years 2004–2007 and 2008–2011 respectively. SIPP respondents were interviewed every 4 months, a period the Census Bureau refers to as a wave; for the current research this provided a balanced 12 observations (12 waves) per household in each panel.
Respondents were interviewed in four staggered rotation groups, with one rotation group beginning each month. The Census Bureau collected “core” modules consisting of questions that are asked at each wave over the course of the panel and include demographic, employment, income, household composition, government transfer and program participation, and myriad other social, economic, and demographic characteristics. “Topical” modules were also collected each wave, but the topics included varied and addressed topics including food security, the reasons people applied for SNAP, WIC, and other food assistance, and numerous other questions associated with the status of households over time. The timing and frequency of SIPP topical modules varied, as did the duration of the reference period to which the question refers.
At each 4-month observation period, respondents provided retrospective information about each individual month for a variety of questions. The SIPP’s short 4-month period between interviews produced data that are thought to be less prone to respondent recall errors than other panel surveys that collect retrospective time-variant data, such as the Current Population Survey’s 12-month recall period. Also, relative to other longitudinal data the SIPP’s large sample sizes allowed us to investigate infrequently-occurring phenomena by non-metro/metro status for small subpopulations over time (Ouellette et al. 2004).Footnote 1 The analytic sample for the 2004 panel included 10,396 household reference people age 15 and older that, when weighted, represented 114 million households. The analytic sample for the 2008 panel included 17,797 household reference people age 15 and older that, when weighted, represented 111 million households.
Data Analysis
The data analysis progressed as follows. First, descriptive tables detailing the characteristics of non-metro and metro households in 2005 and 2010 were constructed. These time periods were selected to provide estimates of sample characteristics because Wave 5 of the 2004 panel and Wave 6 of the 2008 panel include the food security topical module, corresponding to calendar years 2005 and 2010, respectively. While other variables used in our analyses are collected in each wave of both panels, the food security assessment was available only once in the 2004 panel and twice in the 2008 panel. Estimates of population characteristics included demographic and economic characteristics associated with the receipt of food assistance, per the literature reviewed above. For example, household type, race and ethnicity of householder, educational attainment of householder, full-time employment status, and other demographic characteristics were indicated for each sample. Also, several food assistance characteristics were identified from the preceding 12-month period, such as whether the household received continuous government food assistance, transitioned into food assistance during the 12 months, received no government food assistance, and so forth. Food insecurity status was also identified by the respondent’s metro/non-metro residence status in that wave.Footnote 2
Next, we used 4 years (12 waves) of data from each of the two panels corresponding to calendar 2004–2011 to estimate the factors associated with receiving formal food assistance. Given the longitudinal nature of the data, the availability of appropriate time invariant control variables, a desire to produce subject-specific coefficients, and a desire to determine the main effect of time invariant characteristics, random effects (or random slopes) logistic regression models were estimated. Specifically, the probability of receiving assistance was estimated as.
where Ρ it is the probability householder i received food assistance in wave t, x it is a vector of time-varying household characteristics, zi is a vector of time-invariant household characteristics, and αi is a random variable representing all differences between individuals that are stable over time and not accounted for by γzi. By design, each SIPP panel included a refreshed (new) set of respondents. Therefore, separate analyses were conducted for the two panels. The random effects model clustered observations around the householder to account for over-time correlations between observations for householders. Robust standard errors were employed and state fixed effects were included to isolate state variations in the availability of food assistance and public policies.
With one exception, all potential time varying characteristics were measured each of the 12 waves (every 4 months). The Census Bureau assessed food insecurity just one time in the 2004 panel (Wave 5) and only two times in the 2008 panel (Waves 6 and 9). Consequently, care was taken to transform these variables to retain as much information as possible given the measurement shortcoming presented by the SIPP data. For the 2004 panel analysis, a single value reported was used in each measurement period. Because the 2008 panel assessed food insecurity twice, a categorical variable was created to identify respondents as being insecure throughout, transitioning into food insecurity, transitioning out of food insecurity, or being food secure throughout. Although these methods introduced some possibility for imprecision, we believe that one- or two-time indicators of food hardship was better than omitting food insecurity entirely. Importantly, we examined parallel models that omitted the food insecurity variables to assess whether our results regarding metro status were consistent with, and without, the inclusion of food hardship indicators.
Results
Descriptive Results
Figure 1 illustrates the patterns of household food assistance for the two panels. Between 2004 and 2007, 6.2% of households continuously received food assistance, 2.8% transitioned into assistance, 2.6% transitioned out of assistance, 4.3% temporarily received assistance, 1.8% of households temporarily received no assistance, and 5.6% of households cycled on and off assistance. Similar patterns were noted between 2008 and 2011, with 8.1% of households continuously received food assistance, 4.5% transitioned into assistance, 2.3% transitioned out of assistance, 4.7% temporarily received assistance, 1.8% of households temporarily received no assistance, and 5.4% of households cycled on and off assistance. The increase in households that continuously received assistance, as well as those that transitioned into assistance, was indicative of the overall increase in households that received food assistance during and after the Great Recession. As illustrated in Fig. 2, metro households consistently received government food assistance at a lower rate than their non-metro counterparts.
Sample characteristics, as observed in 2005 and 2010, can be found in Table 1. Most households were in metro areas, with the percentage increasing from 77% in 2005 to 79% in 2010. In 2005, 14.5% of non-metro households reported receiving government food assistance. This number grew to 18.5% in 2010. A similar increase was noted in food assistance among metro households, with 13.8% of households receiving assistance in 2005 and 16.2% receiving assistance in 2010. A higher percentage of metro households reported being food insecure in 2005 (7.9 vs. 6.9%) and in 2010 (10.2 vs. 9.9%), though the differences were not statistically significant. Some differences were observed in patterns of assistance receipt between metro and non-metro households. Bi-variate tests indicated a higher percentage of metro households received continuous food assistance compared to non-metro households in 2010, and that a higher percentage of non-metro households transitioned out of receiving government food assistance compared to metro households.
Regression Results
Random effects logistic regression models were estimated for each 4-year panel that estimated the odds of receiving formal food assistance in a given wave. Each model incorporated food assistance receipt information from 12 observations per respondent, as well as for each of the time varying variables. While not reported, state fixed effects were included to control for policy and assistance availability differences, with significant differences in the receipt of food assistance noted.
When interpreting results of these analyses, especially in regard to evaluating effect sizes, it is important to consider that the random effects models generate subject-specific estimates. While the magnitude of estimates is often larger than those generated by analyses that create population-averaged estimates, such as those generated by standard logistic regression analyses, subject-specific estimates are considered to be more accurate estimates of causal relationships (Allison 2005). These longitudinal random effects model coefficients describe what happened to a single household given a unit increase in the independent variable (or, in the case of categorical variables, if the characteristic is present). This is an important distinction only for longitudinal random effects logistic regression models, as subject-specific and population-averaged estimates are identical when using linear estimation models.
2004 Panel
Results for the 2004 panel analyses, which included data gathered in calendar years 2004–2007, are presented in Table 2. The 12-wave random effects logistic regression model yielded strong evidence of a systematic relationship between the receipt of food assistance and a household’s metro status. The odds that a household in a metro area received formal food assistance in a given wave were approximately 50% lower than a household in a non-metro area, holding all else equal. Results also indicated that the odds of receiving food assistance increased over time. Compared to Wave 1, all later waves were associated with an increased likelihood of receiving formal food assistance. For example, the odds ratio for Wave 2 was 1.204. Subsequent waves’ odds ratios all increased relative to Wave 1 for the remainder of the panel and the formal start of the recession in Wave 12, which corresponds to the final quarter of 2007.
There also was evidence of a relationship between being food insecure at a point in time (2005) and having received food assistance at any point in time. The odds that a household that reported being food insecure in 2005 also received food assistance was more than six times greater (odds ratio of 7.629) than a food secure household, holding all else equal. Of course, the food insecurity measure in this panel was limited to the single 2005 assessment so caution about this relationship is warranted. This strong association between food insecurity in one wave and the odds of receiving assistance in any wave was notable, despite the clear measurement challenge that requires the indicator to be treated as a constant. To be certain that including the one-time food insecurity assessment did not alter the results of the independent variable of interest—the metro status of the household—we performed parallel analyses without the food insecurity variable included in the model. The results were nearly identical, with a metro coefficient of −0.691 with food insecurity included in the model and −0.658 when excluded. Just as notable is that all other coefficients exhibited only minor changes in the magnitude of the coefficients, there were no changes in statistical significance, the direction of the associations remained the same, and no changes in any conclusions about the model.
2008 Panel
Results for the 2008 panel analyses, which included data from calendar years 2008–2011, are presented in Table 3. As in the 2004 analyses, the random effects logistic regression model yielded strong evidence of an association between the receipt of food assistance and whether the household lived in a metro area. The odds that a household in a metro area received formal food assistance in a given wave were approximately 42% lower than a household in a non-metro area, holding all else equal. Similar to the results from the 2004 panel, the 2008 panel time coefficients indicated that the odds of receiving food assistance increased over the course of the panel. Compared to Wave 1, all later waves exhibited increasing odds of receiving formal food assistance.
Because the 2008 panel included two waves of food insecurity information, a slightly more nuanced analysis of the relationship between food insecurity and food assistance receipt was possible. The two food insecurity assessments were exactly one year apart, corresponding to the years 2009 and 2010. As a result, a four-category typology allowed the identification of households that were always food insecure, transitioned into food insecurity, transitioned out of food insecurity, and those that were never deemed food insecure (the reference category). The odds of a household receiving food assistance if they were food insecure in 2009 and 2010 were more than ten times greater (odds ratio of 14.041) than a household that was food secure both waves, holding all else equal. Similarly, the odds of a household that had transitioned into food insecurity received formal assistance were 453.4% greater than a household that always reported being food secure. Finally, the odds that a household that transitioned out of food insecurity had received formal food assistance were 339% greater than those that were always food secure, holding all else equal. Once again, to be confident that including food insecurity variables did not alter the results of the independent variable of interest—the metro status of the household—parallel analyses with and without the food insecurity typology variables were estimated. The parallel regressions without the food insecurity variables again proved to be nearly identical, with a 2008 panel metro status coefficient of −0.537 with food insecurity and −0.507 without the variables in the model. As before, all other coefficients exhibited only minor changes in the magnitude of the coefficients, with no changes in statistical significance, the direction of the association, or changes in conclusions about the model. Together, the models from both panels offered evidence of systematic metro/non-metro differences with respect to the receipt of food assistance.
Discussion
The overarching goal of this research was to identify whether there were differences in the rates at which residents of metro and non-metro areas of the US received food assistance from government sources leading up to, and then after, the Great Recession. Our interest in identifying any existing differences by population density is grounded in historical resource disparities between in urban and rural areas. Because household incomes tend to be lower and persistent poverty more likely in non-urban settings (Slack and Myers 2012), the pallet of resources available to secure basic needs such as food may be substantively different for people seeking assistance in metro and non-metro settings.
Market goods and services that are readily available in metro areas may not be as easily accessed in rural areas. Indeed, Harvey et al. (2002) found that among low-income families in persistently poor rural areas, turning to extended family or other sources of non-government assistance may be more common than seeking government food assistance. And, households in rural Iowa have been documented as using community-based support systems more than social networks of family or friends to protect against food hardship (Morton et al. 2005). Even the perception that help is available may be associated with different outcomes. According to Garasky and colleagues (2006) rural residents that felt they could get help from others when needed were less likely to experience food insecurity (Garasky et al. 2006). Although the federal government’s food programs remain the dominant source of assistance for families facing food hardship, local organizations—both public and private—are increasingly becoming the providers of social services (Allard 2009; Heflin and Miller 2012). But, there is variation in how programs are administered at the local level (Food and Nutrition Service 2012; Zedlewski and Brauner 1999), which may influence residents’ success when seeking assistance. Accordingly, it is important that researchers, and those who assist with basic needs, understand whether differences in the receipt of assistance exist and, if so, how to address any disparities.
The results of this study offer evidence at the bivariate and multivariate levels that households residing in metro areas were less likely to receive food assistance than their non-metro counterparts before and after the recession. In terms of pattern of receipt of food assistance, a higher percentage of non-metro households transitioned off government assistance in the 2008–2011 time period, and a lower percentage of metro households continuously received government food assistance during that same 4-year period. Random effects logistic regression models, which account for the influence of time and unobserved variation among the states, revealed that otherwise similar metro households were significantly less likely to receive food assistance than their non-metro peers. When compared to those living in a non-metro area household, the odds that a metro household received food assistance in a given wave were approximately 50% lower in the 2004 panel analysis, and 42% lower in the 2008 panel analysis. Taken together, the bivariate and multivariate analyses suggest that systematic differences in the receipt of government food assistance were present despite relatively similar (statistically indistinguishable) expressions of food hardship in both panels. Several reasons for this assistance gap are plausible. For example, it is possible that metro residents are not as skilled as non-metro residents at seeking assistance, that the metro-based assistance infrastructure is over-burdened, that the assistance delivery infrastructure is better in non-metro areas, or some combination of these possibilities. Unfortunately, SIPP data do not include variables that allow these possibilities to be investigated.
From the lens of the neoclassical household production framework, the results suggest that households in metro and non-metro areas face different constraints and/or have different preferences regarding the use of government food assistance. For example, non-metro household decision makers may prefer, or simply have lower costs associated with, informal sources of assistance. Or, perhaps informal assistance is more accessible than in metro areas and serves as a complement that increases overall household resources. Unfortunately, because SIPP data do not provide information on help-seeking behaviors, attitudes about public vs. private assistance, or indicators of accessibility of services, future research that explores the reasons behind this disparity—and the full complement of resources available to households that effectively serve as substitutes for, or complements to, government food assistance—would add a valuable dimension to the literature on help-seeking behavior and the provision of food assistance.
How government food services are provided may also contribute to differences in the rate of receipt of assistance between metro and non-metro households. Local offices can encourage, or discourage, access through a variety of ways (Food and Nutrition Service 2013). As local providers become more responsible for the provision of social services for both metro and rural areas (Heflin and Miller 2012), evidence of geographic disparities may increase. In their analysis of US counties, Heflin and Miller (2012) found that rural and metro areas have differing needs and that successful strategies for providing social services in one area may not work in the other area. Our research suggests that while the probability of hardship may have been similar in non-metro and metro areas, it may be metro areas that remain underserved. Although isolation has often been thought to characterize rural areas and as a risk factor that may constrain food access and the ability of service providers to reach residents in these areas (Smith and Morton 2009), the results of our study suggest that the possibility of social service or resource isolation in metro areas should also be investigated.
Finally, as Swanson et al. (2008) noted, government food assistance is truly supplemental, as assistance benefits are rarely enough to meet a household’s food needs (Swanson et al. 2008). Indeed, with average monthly SNAP benefits of approximately $189 and $213 for non-metro and metro households in 2005 and $289 for non-metro and $308 for metro households in 2010, SNAP benefits are best viewed as small supplements to households facing food hardships. While the goal of government assistance is to alleviate food hardship, the benefit levels appear to not be enough to reduce the hardship completely (e.g., Jensen 2002; Ribar and Hamrick 2003; Wilde and Nord 2005). These relatively small benefit levels undoubtedly contribute to the challenges identified in literature that seeks to determine whether government food assistance does in fact reduce food insecurity (for an assessment of empirical strategies see Gregory et al. 2013), but are typically limited to cross sectional data (e.g., Gundersen et al. 2009) or pooled cross sections from longitudinal data (e.g., Ratcliffe et al. 2011) where causal mechanisms are more difficult to ascertain than from quality panel data. Given the rich longitudinal data available on individual households over time in SIPP, we encourage the Census Bureau to increase the frequency of the food insecurity assessment so researchers can better identify the most effective policy responses to food hardships in both non-metro and metro areas.
While the SIPP included strong data related to food assistance for our study, there are several limitations worth mentioning. First, the current literature suggests that there are differences between metro and non-metro households that may affect both the need for, and receipt of, assistance. Furthermore, it is likely that there is significant household variation within metro and non-metro communities. Unfortunately the SIPP data do not allow us to further examine the potential within group variation that may exist due to the binary nature of how metro and non-metro households are identified. Future research on food hardship and food assistance by geographic density would be improved if researchers could examine the within group variations for groups such as inner city metropolitan statistical areas (MSA), other MSA areas (e.g., suburbs), non-MSA farm households, and non-MSA non-farm households. Second, local government and non-profit organizations play a crucial role in the provision of, and access to, food assistance programs. Unfortunately, we were unable to account for specific differences in local entities and their role in food assistance for metro and non-metro households due to data limitations. By design, SIPP data are collected to provide national-level estimates with current publicly-available versions of SIPP data, even state-level estimates are precluded, though state-level effects can be included, as we have done, to provide some accounting of unobserved variation by state. Finally, although the SIPP data include food-related benefit levels in the datasets, the food insecurity assessment is available only once in the 2004 panel and twice in the 2008 panel. To include the food insecurity status in our analysis, the results related to food insecurity require interpretation in a manner consistent with the variable being measured as time-invariant variable.
Conclusions and Implications
With over 49 million Americans (14.3% of households) experiencing food insecurity in 2013 (Coleman-Jensen et al. 2014), food-related hardships continue to be a public policy concern. Access, availability, and utilization of food assistance programs have the potential to vary by geographic location, creating different landscapes for households attempting the address their hardship. While we found food insecurity rates were similar between metro and non-metro households, non-metro households were more likely to receive government food assistance. Consequently, it is important that policymakers, and those who assist with basic needs, understand how differences in the receipt of assistance exist according to geographic location and, if so, how to address any disparities. This need to understand the food assistance disparity became even more acute as the assistance gap widened post-recession as government resources were expanded. Although the results from this research do not pinpoint a cause of widening disparities, they do inform the continuing debate about the efficient allocation of public assistance resources intended to reduce food hardship disparities in both metro and non-metro areas.
Given variations in receipt of food assistance, future research regarding food assistance (both government and informal assistance) and food insecurity should incorporate geographic information that allows both researchers and policymakers to better understand the presence of geographic disparities and, as appropriate, identify strategies that allow all food-challenged households to access food assistance regardless of where they reside. Including specific geographic indicators, such as metro/non-metro status with more refined information about residential density within these categories, will allow food assistance providers to more efficiently and fairly allocate their efforts to underserved households. The results of this research indicate that metro and non-metro households not only receive food assistance at different rates, but that the amounts of assistance may vary, which is an important aspect to consider when investigating equity with respect to the receipt of assistance. Finally, when viewing these results with the benefit of time, it is notable that these food assistance disparities were present during an era of unprecedented financial assistance from the federal government. With a new administration, changes in priorities and funding may impact food assistance programs. Although funding for SNAP and school food assistance programs will remain similar because of their status as mandatory or entitlement programs, other proposed food assistance cuts could exacerbate the challenges faced by communities that appear to be underserved (DelReal 2017; Parlapiano and Aisch 2017). To the extent that program cuts are enacted and coordination expectations for service providers decline, existing assistance disparities that are identifiable by geographic density may be exacerbated.
Notes
For example, the Panel Study of Income Dynamics includes approximately 20,000 individuals in 10,000 families whereas a typical SIPP panel includes more than 100,000 individuals in more than 40,000 families. This larger sample size offers more precise estimates of less commonly held characteristics. .
The SIPP food security module’s reference period is 4 months whereas the Current Population Survey’s reference period is 12 months. Thus, SIPP estimates are lower as there is less “opportunity” to experience the hardship.
References
Allard, S. W. (2009). Out of reach: Place, poverty, and the new American welfare state. New Haven, CT: Yale University Press.
Allison, P. (2005). Fixed effects regression methods for longitudinal data using SAS ®. Cary, NC: SAS Institute Inc.
Becker, G. S. (1965). A theory of the allocation of time. The Economic Journal, 75, 493–515.
Bryant, W. K., & Zick, C. D. (2006). The economic organization of the household. New York: Cambridge University Press.
Coleman-Jensen, A., Gregory, C. & Singh, A. (2014). Household food security in the United States in 2013. US Department of Agriculture: Economic Research Service. Retrieved from http://www.ers.usda.gov/publications/err-economic-research-report/err173.aspx.
Congressional Budget Office (2012). The Supplemental Nutrition Assistance Program. Retrieved from http://www.cbo.gov/sites/default/files/cbofiles/attachments/04-19-SNAP.pdf.
DelReal, J. A. (2017, March 16). Drumpf seeks $4.7 billion in cuts to USDA discretionary spending. The Washington Post. Retrieved from https://www.washingtonpost.com/national/drumpf-seeks-47-billion-in-cuts-to-usda-discretionary-spending/2017/03/15/c8fd5622-09ab-11e7-b77c-0047d15a24e0_story.html.
DeNavas-Walt, C., Proctor, B. D., & Smith, J. C. (2011). Income, poverty, and health insurance coverage in the United States: 2010. US Census Bureau, Current Population Reports. Washington, DC: US Government Printing Office. Retrieved from http://www.clark4626.org/08_Green_Energy/A_Payroll-Tax/Images/Income.pdf.
Farrigan, T., & Parker, T. (2012, December). The concentration of poverty is a growing rural problem. Amber waves: The economics of food, farming, natural resources, and rural America, 10(4). Retrieved from http://www.ers.usda.gov/amber-waves/2012-december/concentration-of-poverty.aspx#.UVr5ATewV8E.
Food and Nutrition Service (2012). Supplemental Nutrition Assistance Program: State options report. Washington, DC: US Department of Agriculture. Retrieved from http://www.fns.usda.gov/sites/default/files/10-State_Options.pdf.
Food and Nutrition Service (2013). Supplemental Nutrition Assistance Program: To apply… Washington, DC: US Department of Agriculture. Retrieved from http://www.fns.usda.gov/snap/applicant_recipients/apply.htm.
Garasky, S., Morton, L. W., & Greder, K. A. (2006). The effects of the local food environment and social support on rural food insecurity. Journal of Hunger and Environmental Nutrition, 1(1), 83–103. doi:10.1300/J477v01n01_06.
Goetz, S. J., Rupasingha, A., & Zimmerman, J. N. (2004). Spatial Food Stamp Program participation dynamics in US counties. Review of Regional Studies, 34, 172–190.
Gregory, C., Rabbitt, M. P., & Ribar, D. C. (2013). The Supplemental Assistance Nutrition Assistance Program and food insecurity. University of Kentucky Center for Poverty Research Discussion Paper Series, DP2013-10. Retrieved from: http://www.ukcpr.org/sites/www.ukcpr.org/files/documents/DP2013-10_0.pdf.
Grieger, L. D., & Danziger, S. H. (2011). Who receives food stamps during adulthood? Analyzing repeatable events with incomplete event histories. Demography, 48, 1601–1614. doi:10.1007/s13524-011-0056-x.
Grusky, D. B., Western, B., & Wimer, C. (2011). The consequences of the great recession. In D. B. Grusky, B. Western & C. Wimer (Eds.), The great recession (pp. 3–20). New York: Russell Sage.
Gundersen, C., Jolliffe, D., & Tiehen, L. (2009). The challenge of program evaluation: When increasing program participation decreases the relative well-being of participants. Food Policy, 34, 367–376. doi:10.1016/j.foodpol.2008.12.001.
Gundersen, C., & Kreider, B. (2008). Food stamps and food insecurity: What can be learned in the presence of non classical measurement error? Journal of Human Resources, 43(2), 352–382. doi:10.3368/jhr.43.2.352.
Gundersen, C., & Oliveira, V. (2001). The Food Stamp Program and food insufficiency. American Journal of Agricultural Economics, 84(3), 875–887. doi:10.1111/0002-9092.00216.
Guo, B. (2012). Material assistance: Who is helped by nonprofits? Social Work Research, 36(3), 167–179. doi:10.1093/swr/svs010.
Harvey, M., Summers, G. F., Pickering, K., & Richards, P. (2002). The short-term impacts of Welfare reform in persistently poor rural areas. In B. A. Weber, G. J. Duncan, & L. A. Whitener (Eds.), Rural dimensions of welfare reform (pp. 375–410). Michigan: Kalamazoo.
Heflin, C., & Miller, K. (2012). The geography of need: identifying human service needs in rural America. Journal of Family Social Work, 15, 359–374. doi:10.1080/10522158.2012.719476.
Jensen, H. H. (2002). Food insecurity and the Food Stamp program. American Journal of Agricultural Economics 84(5), 1215–1228. Retrieved from http://www.jstor.org/stable/1245051.
Mincer, J. (1963). Market prices, opportunity costs, and income effects. In Measurement in economics, In C. F. Christ (pp. 67–82). California: Stanford.
Morton, L. W., Bitto, E. A., Oakland, M. J., & Sand, M. (2005). Solving the problems of Iowa food deserts: Food insecurity and civic structure. Rural Sociology, 70(1), 94–112. doi:10.1526/0036011053294628.
Morton, L. W., & Blanchard, T. C. (2007). Starved for access: Life in rural America’s food deserts. Rural Realities, 1(4), 1–10. Retrieved from http://eatbettermovemore.org/SA/enact/neighborhood/documents/RuralRealitiesFoodDeserts1-4.pdf.
National Bureau of Economic Research. (2012). US business cycle expansions and contractions. Retrieved from http://www.nber.org/cycles.html.
Nord, M., Andrews, M., & Carlson, S. (2008). Household food security in the United States, 2007. US Department of Agriculture: Economic Research Service. Retrieved from http://www.ers.usda.gov/Publications/Err66/.
Nord, M., Andrews, M., & Carlson, S. (2009). Household food security in the United States, 2008. US Department of Agriculture: Economic Research Service. Retrieved from http://www.ers.usda.gov/Publications/Err83/.
Nord, M., & Golla, A. M. (2009). Does SNAP decrease food insecurity? Untangling the self-selection effect. US Department of Agriculture: Economic Research Service. Retrieved from http://www.ers.usda.gov/Publications/Err85/.
Ouellette, T., Burstein, N., Long, D. & Beecroft, E. (2004). Measures of material hardship: Final report. US Department of Health and Human Services: Office of the Assistant Secretary for Planning and Evaluation. Retrieved from http://aspe.hhs.gov/hsp/material-hardship04/.
Parlapiano, A., & Aisch, G. (2017, March 16). Who wins and loses in Drumpf’s proposed budget. The New York Times. Retrieved from https://www.nytimes.com/interactive/2017/03/15/us/politics/drumpf-budget-proposal.html.
Rank, M. R., & Hirschl, T. A. (1993). The link between population density and Welfare participation. Demography, 30(4), 607–622. doi:10.2307/2061809.
Rank, M. R., & Hirschl, T. A. (2005). The likelihood of using food stamps during the adulthood years. Journal of Nutrition Education and Behavior, 37, 137–146. doi:10.1016/S1499-4046(06)60268-6.
Ratcliffe, C., McKernan, S., & Zhang, S. (2011). How much does the Supplemental Nutrition Assistance Program reduce food insecurity? American Journal of Agricultural Economics, 93(4), 1082–1098. doi:10.1093/ajae/aar026.
Ribar, D. C., & Hamrick, K. S. (2003). Dynamics of poverty and food sufficiency. US Department of Agriculture. Retrieved from http://www.ers.usda.gov/Publications/FANRR36/.
Rosenbaum, D. (2013). SNAP is effective and efficient. Washington, DC: Center on Budget and Policy Priorities. Retrieved from http://www.cbpp.org/cms/?fa=view&id=3239.
Sabia, J. J., & Nielsen, R. B. (2015). Minimum wages, poverty, and material hardship: New evidence from the SIPP. Review of Economics of the Household, 13(1), 95–134. doi:10.1007/s11150-012-9171-8.
Sharkey, J. R. (2009). Measuring potential access to food stores and food-service places in rural areas in the US. American Journal of Preventive Medicine, 36(45), S151–S155. doi:10.1016/j.amepre.2009.01.004.
Slack, T., & Myers, C. A. (2012). Understanding the geography of Food Stamp Program participation: Do space and place matter? Social Science Research, 41, 263–275. doi:10.1016/j.ssresearch.2011.10.001.
Smith, C., & Morton, L. W. (2009). Rural food deserts: Low income perspectives on food access in Minnesota and Iowa. Journal of Nutrition Education and Behavior, 41(3), 176–187. doi:10.1016/j.jneb.2008.06.008.
Swanson, J. A., Olson, C. M., Miller, E. O., &. Lawrence, F. C. (2008). Rural mothers’ use of formal programs and informal social supports to meet family food needs: A mixed methods study. Journal of Family and Economic Issues, 29(4), 674–690. doi:10.1007/s10834-008-9127-6.
Van Hook, J., & Balistreri, K. S. (2006). Ineligible parents, eligible children: Food stamps receipt, allotments and food insecurity among children of immigrants. Social Science Research, 35(1), 228–251. doi:10.1016/j.ssresearch.2004.09.001.
Wilde, P., & Nord, M. (2005). The effect of food stamps on food security: A panel data approach. Review of Agricultural Economics, 27(3), 425–432. doi:10.1111/j.1467-9353.2005.00239.x.
Zedlewski, S. R., & Brauner, S. (1999). Declines in food stamp and welfare participation: Is there a connection? Washington, DC: Urban Institute. Discussion Paper No. 99–13. Retrieved from http://webarchive.urban.org/publications/309198.html.
Acknowledgements
This research was supported, in part, by USDA National Institute of Food and Agriculture Hatch project 1003397. The performing organization was the University of Georgia Agricultural Experiment Station, which supported project GEO00731.
Author information
Authors and Affiliations
Corresponding author
Additional information
Authors listed alphabetically to reflect equal contribution.
Rights and permissions
About this article
Cite this article
Nielsen, R.B., Seay, M.C. & Wilmarth, M.J. The Receipt of Government Food Assistance: Differences Between Metro and Non-Metro Households. J Fam Econ Iss 39, 117–131 (2018). https://doi.org/10.1007/s10834-017-9528-5
Published:
Issue Date:
DOI: https://doi.org/10.1007/s10834-017-9528-5