Abstract
The Campbell CSAT3 sonic anemometer is one of the most popular instruments for turbulence measurements in basic micrometeorological research and ecological applications. While measurement uncertainty has been characterized by field experiments and wind-tunnel studies in the past, there are conflicting estimates, which motivated us to conduct a numerical experiment using large-eddy simulation to evaluate the probe-induced flow distortion of the CSAT3 anemometer under controlled conditions, and with exact knowledge of the undisturbed flow. As opposed to wind-tunnel studies, we imposed oscillations in both the vertical and horizontal velocity components at the distinct frequencies and amplitudes found in typical turbulence spectra in the surface layer. The resulting flow-distortion errors for the standard deviations of the vertical velocity component range from 3 to 7%, and from 1 to 3% for the horizontal velocity component, depending on the azimuth angle. The magnitude of these errors is almost independent of the frequency of wind speed fluctuations, provided the amplitude is typical for surface-layer turbulence. A comparison of the corrections for transducer shadowing proposed by both Kaimal et al. (Proc Dyn Flow Conf, 551–565, 1978) and Horst et al. (Boundary-Layer Meteorol 155:371–395, 2015) show that both methods compensate for a larger part of the observed error, but do not sufficiently account for the azimuth dependency. Further numerical simulations could be conducted in the future to characterize the flow distortion induced by other existing types of sonic anemometers for the purposes of optimizing their geometry.
Similar content being viewed by others
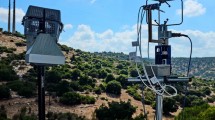
Avoid common mistakes on your manuscript.
1 Introduction
The eddy-covariance method has been well established to measure the energy and matter exchange between the surface and the atmosphere. Sonic anemometers are at the core of those micrometeorological measurement systems, whose accuracy is, therefore, crucial for any research on biosphere-atmosphere interactions. Fluxes of energy, water, and \(\hbox {CO}_{2}\) are currently measured at several hundred long-term FLUXNET sites around the world (Baldocchi 2014) for which the CSAT3 anemometer (Campbell Scientific Inc., Logan, Utah, USA) is one of the most popular instruments within this network. The CSAT3 anemometer has been commercially available for more than 20 years, and has become the standard instrument of several national networks, e.g., ChinaFLUX (Yu et al. 2006), TERENO (Zacharias et al. 2011) and NEON (SanClements et al. 2014).
The accuracy of commonly used sonic anemometers is nevertheless still discussed, because a reference calibration is not readily available. Transducer-shadow effects or more general probe-induced flow distortion are suspected to be larger than what was previously thought (Kochendorfer et al. 2012), where non-orthogonal sonic arrays are found to be particularly prone to flow distortion (Frank et al. 2013). However, the magnitude of the error found for the CSAT3 anemometer differs considerably between different studies, with reports ranging from 3% to 14% for the vertical component of velocity fluctuations (Kochendorfer et al. 2012; Mauder 2013; Frank et al. 2013; Horst et al. 2015). As this situation is unsatisfactory, we wish to revisit older theoretical analyses to shed more light on this issue.
Wyngaard (1981) developed the first theory on probe-induced flow-distortion effects in which linear effects dominate, with second-order effects neglected. The theory was validated by wind-tunnel experiments, which showed that transducer-shadow effects are directly proportional to the ratio of the transducer diameter d to the sonic path length L (Kaimal 1978; Wyngaard and Zhang 1985), indicating that for \(d{/}L<0.05\), the resulting attenuation <10%. The increased theoretical understanding led to the development of improved instruments that minimize flow distortion. For example, the transducer diameter d is to be as small as possible, with its shape aerodynamically optimized (Hanafusa et al. 1982), the sensor should be as vertically symmetrical as possible (Wyngaard 1988), and the support structure should be minimized (Dyer 1981; Kaimal et al. 1990). Wyngaard (1981) also pointed out that great care should be taken to avoid cross talk between the three velocity components because a potential-flow correction during post-processing is not possible. Kaimal (2013) gives a chronological account of the conception of sonic anemometry and the development of non-orthogonal anemometers.
These considerations led to the design of an optimized sonic-anemometer array, the so-called UW probe developed at the University of Washington (Zhang et al. 1986), which has three non-orthogonal intersecting acoustic paths tilted \(60{^{\circ }}\) from the horizontal plane, while supported only from behind. The \(60{^{\circ }}\) angle was chosen because it facilitates a more accurate determination of the vertical velocity component than does a \(45{^{\circ }}\) angle. This novel geometry became a model for the CSAT3-anemometer design, resulting in less flow distortion for than for previously available sonic anemometers, while being particularly optimized for the measurement of vertical fluxes (Foken and Oncley 1995).
Two possibilities to evaluate the error of sonic anemometers have included wind-tunnel studies (e.g. Kaimal and Gaynor 1983; Christen et al. 2001; Wieser et al. 2001), and side-by-side field intercomparison experiments (e.g. Dyer et al. 1982; Mauder et al. 2007; Tsvang et al. 1985), both of which have advantages, but also weaknesses. While wind-tunnel tests are conducted with steady flow under quasi-laminar conditions, the instruments are typically deployed in the atmospheric boundary layer, where they experience a wide spectrum of turbulent fluctuations. As flow distortion depends on the turbulence intensity (Wyngaard 1981), wind-tunnel-based flow-distortion corrections tend to overestimate the true magnitude of flow distortion (Högström and Smedman 2004).
Field intercomparisons are conducted under real-world conditions. Sonic anemometers have been available for about 50 years, and it was the initial interest of the pioneers of this technique to compare the instruments under natural conditions within the so-called international turbulence comparison experiments (Miyake et al. 1971; Tsvang et al. 1973; Dyer et al. 1982; Tsvang et al. 1985), where the optimal anemometer design was the focus of research. Because of influences of the sensor and nearby devices on the degree of flow distortion (Dyer 1981), it was decided that the instruments should be installed perpendicular to the mean flow at a suitable distance from each other to help minimize this problem. Experimental sites at Tsimlyansk (Russia, 1970 and 1981) and Conargo (Australia, 1976) were considered to be ideal for such studies due to their horizontal homogeneity. The basic assumption for such comparisons was a homogeneous measuring field to ensure nearly identical turbulent characteristics for all instruments. Unfortunately, the anemometers compared then are no longer actively used. Hence, a comparison experiment following these assumptions with most of the available sonic anemometers was realized in California in the summer of 2000 (Mauder et al. 2007). Another possibility is the comparison of differently oriented sonic-anemometer arrays to detect flow distortion (Kaimal et al. 1990; Horst et al. 2015). Traditionally, only turbulence statistics are compared between different instruments, because sensors do not sample the same realization of the turbulence field due to the separation between sensors necessary to avoid cross-contamination. Recently, different sonic anemometers were placed within one integral length scale, i.e. with a separation of less than 2 m (Kochendorfer et al. 2012), to compare even instantaneous turbulence.
In any event, field intercomparisons always have the difficulty of defining an absolute reference or etalon, since it is only possible to compare one instrument with another. Nevertheless, good agreement in different statistics measured by two instruments independently over the same surface increases the confidence in the measurements. Such experiments are, therefore, very useful in characterizing the precision of sonic measurements. For example, if sonic anemometers are to be deployed at different sites at a later date, it is important to know at what scale differences are able to be detected. However, even with good agreement between two instruments, both could potentially be equally inaccurate (Horst et al. 2015).
A third possibility will be explored here, which returns to the idea of an etalon having well-known characteristics, while being stable for long time periods. For past field intercomparison experiments, the etalon was realized by using the same instrument in different comparison experiments (Mauder et al. 2007; Goodrich et al. 2016). In the following, the characteristics of a sonic anemometer are determined numerically by large-eddy simulation (LES), where a series of numerical simulations of the flow around a sonic anemometer with oscillating winds will be conducted. Within this model set-up, the undisturbed wind velocities can also be determined. The results aim to answer the following questions:
-
How large are the measurement errors that arise from probe-induced flow distortion?
-
Are the measurements of the vertical velocity component w and horizontal velocity component u equally affected by flow distortion?
-
To what extent does the flow distortion error differ with varying azimuth and angle-of-attack?
-
How does the frequency of wind-speed fluctuations affect the flow-distortion error?
We consider such a numerical experiment as an important step towards a true comparability between different anemometers, and thus complementing the findings from experiments in the real world. For this study, the CSAT3 sonic anemometer (Campbell Scientific Inc., Logan, Utah) was selected, because the construction details and all software calculations in the firmware were readily provided upon request by the manufacturer. Figure 1 shows a CSAT3 sonic anemometer deployed at a field site. However, the same analysis can be conducted for any other sonic-anemometer model in principle. As a first step, the flow-distortion issue is addressed for fixed mean wind speeds and fixed frequencies for the horizontal and vertical wind components. Here, the focus is on the frequency dependence of the flow distortion. In a planned second study, the simulation will be extended to natural turbulence spectra.
2 Methods
2.1 LES in OpenFOAM\(^\circledR \)
OpenFOAM\(^\circledR \) is an open source C++ library for solving the partial differential equations commonly encountered in continuum mechanics (Weller et al. 1998). The LES models in OpenFOAM\(^\circledR \) have also been employed to simulate atmospheric boundary-layer flows (Schlegel et al. 2012). The OpenFOAM\(^\circledR \) version 2.3 is used here to solve the governing equations on a co-located unstructured mesh by the finite-volume method. An algebraic Smagorinsky model is employed to model the subgrid-scale fluxes (Fureby et al. 1997). The discretized equations are integrated in time by an implicit second-order backward scheme. As the fluid is assumed to be incompressible, the PISO (Pressure Implicit with Splitting of Operator) algorithm is used for handling the velocity-pressure coupling (Issa 1985).
For each case, the total simulated time is 70 s. The velocity field in the flow domain is initialized with a uniform value of zero, with a prescribed time-varying inlet velocity. The spin-up time of 10 s corresponds to one period of oscillation of u for the X and Y cases described below, which is sufficient for the initial wake around the anemometer to develop. The data required for computing the turbulent statistics are recorded for the next 60 s of the simulation. The discretized equations are integrated in time in fixed steps of \(2\times 10^{-4}\) s such that the maximal Courant–Friedrichs–Lewy number is always maintained below a value of 1.
2.2 Mesh Generation
The mesh generation is a critical step in the analysis that directly influences the accuracy of the simulation. While the domain size and the resolution of the mesh are the key factors, the use of a larger domain and smaller mesh spacing results in a higher computational cost. To make this investigation computationally feasible, we neglect the fact that the anemometer is usually situated within the inertial sublayer, which relaxes the need for a larger domain typically required for a fully-developed wall-bounded flow. Furthermore, not having to resolve the wall reduces the number of grid points. Consequently, the flow in vertical (z) and spanwise (y) directions is assumed to be periodic. The numerical wind tunnel is 1 m long in all three directions, and the origin of the domain is set to coincide with the intersection point of the three transducer path lengths. For cases with a \(90{^{\circ }}\) azimuth angle, the domain is extended by 0.2 m on one side of the spanwise direction to maintain the distance between the anemometer structure and the periodic boundary. The input signals for u and w are prescribed at the inlet for the streamwise (x) direction. A constant pressure boundary condition is applied at the outlet. The domain extent in the streamwise direction is sufficient as the magnitude of the velocity at the inflow is always positive, and an appropriate boundary condition is applied at the outlet. The domain extent in the vertical and spanwise directions is also sufficient, since the streamwise flow dominates and the periodic boundaries are placed sufficiently far away from the anemometer structure.
The smallest spatial resolution required is dictated by the diameter of the transducer pin. Since the pin is only 6.4 mm in diameter, a mesh spacing of at most 3 mm is needed in the vicinity of that surface to satisfactorily resolve the geometry. In the coarsest region, the mesh spacing is 12.5 mm, followed by the first refinement region, where the spacing is 6 mm. The finest resolution at the surface of the anemometer is between 1 mm and 2.5 mm. A constant-flux layer is assumed in the first cell adjacent to the anemometer surface. The refinement regions in the domain and the resolution around the transducer surface are shown in Fig. 2. Four different meshes were generated for different azimuth angles. Additional meshes for angle-of-attacks of \(5{^{\circ }}\) and \(25{^{\circ }}\) at a \(0{^{\circ }}\) azimuth angle were also generated. The unstructured mesh containing mostly hexahedral cells and some polyhedral cells is generated using a mesh generation tool in OpenFOAM\(^\circledR \) (the so-called snappyHexMesh utility). The meshes meet the common quality requirements for cell aspect ratio and non-orthogonality.
Mesh cross-section at the centre of the domain for the anemometer at a zero azimuth angle. Regions of domain refinement (left), and the mesh near the surface of the transducer (right), are shown. Only two of the six transducer pins are visible in the left sub-panel. While creating a cross-section of the unstructured three-dimensional mesh, some spurious lines are formed in the resulting two-dimensional mesh, although these artefacts do not exist in the computational domain
2.3 Simulation Set-Up
The wind velocity and the frequency for the horizontal and vertical components were selected to be close to typical values found in the field. Therefore, the model spectra by Kaimal et al. (1972) were used to find values in the inertial subrange close to the energy maximum. For an arbitrary measurement height of 2 m, the mean horizontal wind speed \(\bar{u}\) was chosen as 2 m s\(^{-1}\) with a sinusoidal variation of peak amplitude \(u_\mathrm{amp}=1\) m s\(^{-1}\) and a frequency of \(u_\mathrm{freq}=0.1\) Hz; the mean vertical wind speed \(\bar{w}\) was set to zero with a sinusoidal variation of peak amplitude \(w_\mathrm{amp}=0.5\) m s\(^{-1}\) and a frequency of \(w_\mathrm{freq}=1.0\) Hz (case Y). The relevant positions within the turbulence spectra are shown in Fig. 3. In addition, we also conducted simulations where both u and w have the same frequency of oscillation (0.1 Hz, case X), as well as a set of simulations with a constant wind speed and no oscillations, which is similar to wind-tunnel studies (case Z). The oscillating velocity components of case Y are shown in Fig. 4.
Position of the chosen simulation characteristics (black filled circle) for the horizontal component of the velocity u and the vertical component of the velocity w in comparison with the model spectra (modified after Kaimal et al. 1972)
Simulations for all three cases X, Y and Z were conducted for four different azimuth angles (\(0{^{\circ }}\), \(30{^{\circ }}\), \(60{^{\circ }}\) and \(90{^{\circ }})\). For case Z, we also varied the angle-of-attack three times (\(0{^{\circ }}\), \(5{^{\circ }}\) and \(25{^{\circ }})\), together with the angle-of-attack that is changing constantly due to the imposed oscillations for the X and Y cases. The input parameters for the 28 simulations are listed in Table 1. The cases are coded such that the first character indicates the sinusoidal input signal, with the following two digits signifying the azimuth angle, and the last two digits representing the angle-of-attack.
The spectra derived from the virtual sonic anemometer measurements are shown in Fig. 5. The peaks of the respective input signals at 0.1 and 1 Hz can clearly be identified. However, it is interesting to note that part of the turbulent kinetic energy has already been transferred to smaller eddies along the turbulence cascade, even though the distance from the left boundary of the simulation domain is only 0.5 m. This is indicated by an inertial subrange starting at frequencies of about 0.5 Hz for the X cases (Fig. 5a, b) and at about 2 Hz for the Y cases (Fig. 5c, d). For the Y cases, harmonics in the turbulence cascade region of the horizontal component can also be observed. One can also see that there are some minor differences in the spectra depending on the azimuth angle, which is \(0{^{\circ }}\) for the two left subpanels (Fig. 5a, c) and \(90{^{\circ }}\) for the two right subpanels (Fig. 5b, d).
In fact, when rotating the set of three transducer pins \(60{^{\circ }}\) in the xy-plane, and turning the anemometer upside down (\(z\rightarrow -z\)), the latter effectively exchanging the orientation of the upper three pins for that of the lower three pins, the configuration of the transducer is identical to the orientation before rotation. Therefore, we expect the response of the flow to behave similarly for azimuth angles that differ by \(60{^{\circ }}\), at least if the inflow does not change under the operation \(z\rightarrow -z\). For the Y cases, the input velocity has no preferred directionality along the z-axis, because the w-frequency is 10 times higher than the u-frequency and the inflow u and w signals are effectively decoupled. Note that the locus of the input velocity shown in Fig. 6b remains unchanged when the sign of w is reversed. For the X cases, however, w and u have the same frequency and vary in phase, which means that a positive w is correlated with higher wind speeds, and negative w with lower wind speeds. Therefore, the locus of the input velocity for the X cases becomes different when the sign of w is reversed under the operation \(z\rightarrow -z\). Hence, we cannot expect the results for \(\varphi \) and \(\varphi +60{^{\circ }}\) to behave similarly for the X cases.
2.4 Virtual Measurement
The flow distortion by the sonic anemometer is studied by analyzing the velocity fluctuations recorded by a virtual probe inside the simulation domain. The vertical distance between the tips of the lower and upper transducers is 0.1 m. The distance between a matching pair of transducers, i.e. the path length, is 0.12 m. For each pair of transducers, velocity measurements are collected at 11 points along the acoustic path over a length of 0.115 m. In the first cell adjacent to the surface of the anemometer the turbulence is parameterized in an LES, due to the lack of resolved turbulence in this region of the flow, the data collected there are unreliable. The virtual path length is accordingly shortened by 2.5 mm on either side. The 33 values from the three virtual paths are averaged to arrive at a single instantaneous value for u, v and w. The virtual probe collects data at 100 Hz, which is then re-sampled by block averaging to 20 Hz during post-processing, corresponding to the typical frequency for recording field measurements.
The reference measurement for the velocity (i.e. the measurement of the undisturbed flow) is assessed by three different methods. The first is by replicating the virtual probe, but offset by 0.3 m from the anemometer in the spanwise direction (AddProb). The data are presumed to be reliable there since the grid spacing in the reference region is approximately the same as in the transducer path length. One alternative method is to perform separate reference simulations on a structured grid without the anemometer being present in the domain (RefSim). Another alternative method is to directly take the input signal as the reference with which to compare the virtual measurements (InFlow). The influence of the different measurement concepts was tested (see Sect. 4.2) and the InFlow reference was found to be the most appropriate here.
Instantaneous u-velocity component at the cross-section in the middle of the domain for the case Y0000, time = 63 s, as in Fig. 7; the wind velocity components are \(u=2.95\) m s\(^{-1}\) and \(w=0\). Only two of the six transducer pins are visible. A stagnation area in front of the transducers and a recirculation area to the rear side are observed. The wake behind the anemometer is inclined downwards as a result of negative values of w prescribed in the earlier timesteps
3 Results
3.1 Qualitative Analysis
The wake behind the transducers and the supporting structure in the top part of the anemometer is illustrated in Fig. 7, where for a dominant streamwise velocity component, the wakes of the transducers and the supporting structure do not interact. The pulsating velocity of the inflow means that the flow strikes the anemometer at a different angle-of-attack at each timestep, as under naturally turbulent conditions. In Fig. 8, the wake behind the anemometer is tilted downwards due to the non-zero angle-of-attack of the flow, where a recirculation region immediately behind the transducer is also seen. Just above the bottom transducer pin, there is a region of increased flow speed, which will affect the anemometer measurements. Although the instantaneous w-velocity component in the measurement volume of the sonic anemometer is exactly zero in Fig. 8, the wake downwind of the instrument moves below the instrument, because this area of the simulation domain is still influenced by the negative w values applied earlier as the input signal. The downwind wake, which is clearly resolved by our LES, can be considered as being fully turbulent. The second largest structural elements, i.e. the supporting arm along with the spherical joint that connects the three transducers at the top and bottom respectively, cause vortex shedding. However, the wakes generated by the transducer pins, which are much smaller in diameter than the rest of the anemometer structure, appear to be at least partly of a laminar character far downwind, because no fluctuations are visible there. However, the wake region immediately near the pins is certainly turbulent. These observations are confirmed by the vorticity plot in Fig. 9, where the swirls are distorted to some extent by the oscillating input signal. It is noteworthy that the flow in the actual measurement volume appears to be largely undisturbed, with flow distortion only visible in those grid cells of the measurement path that are very close to the transducer pin.
Isosurface (at 66 Hz) of the magnitude of instantaneous vorticity coloured by the velocity magnitude \(\left| U \right| \) in m \(s^{-1}\) (blue to red). The background image shows the vorticity magnitude \(\left| \omega \right| \) in Hz (white to red, but only the yellow part is visible outside of the isosurface). This figure shows the case Y0000 at time = 63 s, as in Fig. 7. Only one measurement path is fully visible (upper-left transducer to lower-right transducer); the other two visible transducers are both frontal parts belonging to two different measurement paths. The corresponding transducers at the back are not visible at this viewing angle. The structure of the vortices is discussed in Sect. 4.1
3.2 Quantitative Analysis
As expected, the mean vertical velocity component is measured accurately for almost all simulated cases, as depicted in Fig. 10. Furthermore, the mean u-component agrees well with the input signal for all the X and Y cases, and those Z cases with zero angle-of-attack. Unexpectedly, the error of the mean u-component is larger for constant flow speeds with a \(5{^{\circ }}\) angle-of-attack than with a \(25{^{\circ }}\) angle-of-attack.
Mean flow speed measured by the virtual sonic anemometer for the u-component (\(\bar{u}\)) and for the w-component (\(\bar{w}\)). The triangles indicate the corresponding component of the input signal for reference. The x-axis shows the different simulated cases listed in Table 1
For flux measurements, the accuracy of the mean velocities is almost irrelevant because the average is always subtracted when calculating a covariance. Only turbulent fluctuations must be captured accurately as is evident from the analysis of the standard deviations of the velocity components (Fig. 11). In our simulations, the relative error of the u-component is generally less than 4% and less than 7% for the w-component, but varying with the azimuth angle. While part of the difference between the relative errors of u and w arises from their different normalizations, because the standard deviation of u is larger than that of w, the absolute error of the standard deviation of w is also larger than that of u (not shown). The smallest errors of the w-component, which is critical for measurements of vertical fluxes, occurs for azimuth angles of \(0{^{\circ }}\), becoming only 3% for the X case with w-oscillations of 0.1 Hz, and 5% for the Y case with 1-Hz oscillations.
In the top subpanel, the virtual CSAT3-measurements of velocity fluctuations are compared with the input signal for reference by plotting the standard deviations of u (\(\sigma _u\)) and w (\(\sigma _w\)). In the bottom subpanel, the relative error of the virtual standard deviation measurements (\(\textit{RE}\sigma _u\) and \(\textit{RE}\sigma _w\)) is shown. Labels X and Y denote cases with a different frequency of oscillation; the trailing digits in the label indicate the azimuth angle of the anemometer
4 Discussion
We align our discussion of the results along the concepts of internal and external validity (Campbell and Stanley 1963), which were originally proposed for experiments in the area of social sciences, but may also be useful here to emphasize the significance of the numerical experiment. If an experiment is not internally valid, we cannot say that the observed results have a causal relationship with the input parameters. If an experiment is not externally valid, results cannot be said to hold outside of the experimental setting, and thus, even if internally valid, they are still irrelevant outside the experiment (Jiménez-Buedo and Miller 2010). The relationship between internal and external validity is often described as a trade-off in which laboratory experiments typically have the highest internal validity, but lowest external validity, and pure observational data collected in the field have the lowest internal validity, but highest external validity (Roe and Just 2009).
4.1 Internal Validity of the Wake Regions
We investigate, firstly, the degree to which the wake regions have been resolved. The anemometer consists of different structural components, each of which leads to the generation of a wake. As seen in Fig. 8, the wake of the transducer pin has a different appearance compared with the wake of the support arm and the wake of the central structure on the right. To discuss the wake behind an immersed structure, we need to first introduce the Reynolds numbers (Re) related to the individual components, while restricting our analysis to one transducer pin, the support arm on the top, and the central structure on the right side, with diameters (D) of 6.4, 15.9 and 100 mm, respectively. With a kinematic viscosity of \(\nu =1.5\times 10^{-5}\,\hbox {m}^{2}\,\hbox {s}^{-1}\) and the mean wind speed of \(\bar{u}=2\,\hbox {m}\,\hbox {s}^{-1}\), the Reynolds numbers are 850, 2100 and 13,333, respectively, where \(Re=\bar{u}D/\nu \). The latter value is above the critical Reynolds number for turbulent flow. Following Williamson (1996), for the two larger values (\(\textit{Re}>1000\)), the turbulent wake region behind the cylinder consists of von Kármán vortices interspersed with many other turbulent elements. For the transducer pin, we, therefore, expect a regular von Kármán vortex street. Hence, the pulsating flow in the x-direction may lead to vortex shedding, depending on the orientation of the structural element. However, we have to take into account that the central structure on the right is not entirely cylindrically shaped, and the support arm is actually aligned with the mean flow direction in the case of a zero mean angle-of-attack. From the oscillating flow in the z-direction, we expect no vortex shedding because of the zero mean vertical velocity.
In addition, the pulsating inflow leads to the further complication that, in theory, the vortex street interacts with the harmonic perturbation on the mean inflow. For oscillating cylinders in steady flow, Karniadakis and Triantafyllou (1989) found that the interaction between the vortex street and the oscillation depends on the ratio of the oscillation frequency to the vortex-shedding frequency (\(f_s\)), and the ratio between the amplitude of the oscillation and the diameter of the cylinder. The oscillation frequency at the inflow is 1 Hz at most here, where the frequency of vortex shedding is estimated from the Strouhal number, \(St=Df_{s} /\bar{u}\), which is approximately 0.2 for \(\textit{Re}<10^{4}\) (Sakamoto and Haniu 1990), leading to \(f_s=50\) Hz for the anemometer pins. Therefore, the ratio of the frequencies here is \(1{/}50\). To determine the ratio of the length scales, we adapt the case of the oscillating cylinder to our case of a pulsating inflow. Changing the frame of reference, the amplitude of the oscillating cylinder is obtained from the amplitude of the velocity fluctuation (\(1\,\hbox {m}\,\hbox {s}^{-1}\)) divided by the angular frequency (6 Hz) of the pulsating inflow, yielding the ratio of the length scales of about 0.15 / 0.01. As both the frequency and length-scale ratios are significantly different from unity, there cannot be a significant alteration of the wake by the low-frequency unsteady inflow.
By taking a closer look at Fig. 9, we notice the vortices detaching from the turbulent wake at the upper arm, while we do not observe a von Kármán street in the wake of the transducer pin, which also appears smoothed in Fig. 8. Therefore, although it is possible that the mesh in front of the supporting structure is not fine enough to resolve the individual von Kármán vortices in the wake behind the transducers, the average behaviour of the wake has been adequately resolved, even though the individual turbulent elements are not. Furthermore, the wake from the transducer pins only crosses the measurement paths for large angles-of-attack. Although the drag coefficient could alter slightly when the von Kármán vortices are resolved, this cannot significantly change the conclusions of the study, especially since the drag coefficient actually drops when a laminar boundary layer develops into a von Kármán street. Hence, for the same mean velocity, the drag will be even smaller in the case of the resolved von Kármán street due to its lower drag coefficient.
4.2 Internal Validity of the Virtual Measurements
Naturally, the internal validity of the presented virtual measurements is high, because the experiment is conducted completely under controlled conditions as it is purely numerical. Any unwanted external disturbances common to experiments in the field are excluded, with measurement errors thereby reduced to the numerical precision of the simulation. Nevertheless, the design of the virtual sonic anemometer measurement, i.e. the exact definition of the sampling points and the sampling interval, may have an influence on the resulting flow-distortion error. Moreover, while the choice of the reference measurement will influence the resulting error estimate, it is uncertain by how much.
We first consider the importance of the exact location of the virtual measurements within the acoustic paths. As described above, we have placed 11 virtual probes in each of the three sonic paths of the anemometer to mimic the actual volume-averaging sampling of the real anemometer. The space between two virtual probes is defined to be larger than the local grid resolution to avoid the same grid cell being sampled twice, resulting in the sampling of every second grid cell within the measurement paths, whereby there are more than 20 grid cells along a path of 0.115 m at a grid resolution of 0.006 m. Since the qualitative analysis showed that the flow in the measurement volume is rather homogeneous, the 11 virtual probes are sufficient because the sampling points are equidistant and the area in the direct vicinity of the transducer pin is well represented. However, if the two sampling points at the ends of each virtual measurement path were to not be taken into account appropriately, the resulting error in the measurement of the standard deviation would even have the opposite sign (data not shown). The opposite sign appears due to mass conservation as the flow slightly speeds up at the centre of the measurement volume as it circumvents the bluff body of the anemometer. Directly next to the transducer pin, the flow is reduced due to drag.
The other question concerns the best reference for calculating the measurement error, or in other words, the exact definition of the etalon, for which we tested three different possibilities:
-
(a)
a separate reference simulation (RefSim),
-
(b)
additional virtual probes (AddProb),
-
(c)
the prescribed input signal (InFlow).
The difference between a, b, and c are quite small (Table 2), and each has advantages and disadvantages. The RefSim reference has the advantage that the position of the virtual measurement in the simulation domain is the same, but the mesh is inevitably slightly different, with high computational costs. The AddProb reference has advantages in that the distance from the left domain boundary is the same and no additional simulation is necessary, but it is uncertain to what extent the addition probes are really undisturbed. This is especially a problem for the \(90{^{\circ }}\) azimuth angle, since a larger computational domain is required to prevent the wake penetrating the periodic spanwise boundary. The InFlow reference also does not require any additional simulation; the signal is certainly undisturbed, but the turbulent cross-talk between the u and w velocity components along the 0.5-m distance between the left boundary and the anemometer position are not reflected. We chose the InFlow reference for the presentation of the results in Sect. 3, because the comparison with the RefSim results shows that the mismatch in measurement position of 0.5 m in the flow direction is not critical.
4.3 External Validity
Our LES simulation can be assumed to have a higher external validity than wind-tunnel studies, because we applied a pulsating inflow with fluctuations oriented in both frequency and amplitude at conditions typical for the inertial sublayer of the atmospheric surface layer, where flux measurements are usually conducted. To assess the external validity of our simulations further, our results are compared with other studies with the aim of characterizing the probe-induced flow distortion of the CSAT3 anemometer.
Friebel et al. (2009) show that the CSAT3 anemometer has no significant error of the mean wind speed with the exception of the wind sector of \(160{^{\circ }}\)–\(200{^{\circ }}\), which is in very good agreement with our results. Furthermore, the error of u-fluctuations is found to be about twice as large for the zero azimuth as for the other flow angles, which is also reported by, e.g., Li et al. (2013), who, as a consequence, propose a different planar fit for the wind sector corresponding to the \(\pm 30{^{\circ }}\) azimuth.
From a field experiment with two horizontally-mounted RM Young 81000 sonic anemometers as reference instruments, Kochendorfer et al. (2012) determine an error estimate of 14% for the standard deviation of the w-component as measured by a tilted CSAT3 anemometer, which is much larger than both our findings, as well as that from all previous field intercomparison experiments, where the CSAT3 anemometer was even chosen as the reference on account of its minimal flow distortion (Foken and Oncley 1995; Loescher et al. 2005; Mauder et al. 2006, 2007). Therefore, we interpret this discrepancy between the error estimate of Kochendorfer et al. (2012) and our results as an indication that the experimental design of the former study was flawed, as previously pointed out by Mauder (2013).
Frank et al. (2013) compared two vertically-mounted CSAT3 anemometers with two horizontally-mounted CSAT3 anemometers to conclude that the horizontally-mounted CSAT3 is less influenced by flow distortion for measuring vertical fluxes. This is confirmed by our results also showing the relative error of the standard deviation of w being approximately twice as large as the relative error for the standard deviation of u. While we agree with this aspect of their study, it is not clear how the error of \(\overline{w^{\prime }T_s^{\prime }}\) can be larger than the error of standard deviation of w (\(\sigma _{w}\)). While the error of \(\overline{w^{\prime }T_s^{\prime }}\) may theoretically be smaller than that of \(\sigma _{w}\), provided the time series of the w-component is affected by spikes uncorrelated with the time series of \(T_s\), a larger error of the flux implies an additional error in the measurement of \(T_s\). Indeed, Horst et al. (2015) demonstrate that flow distortion can also affect the sonic temperature measurement, which may explain these differences in \(\sigma _{Ts}\). Nevertheless, the experimental design of Frank et al. (2013) has the disadvantage that the sensors are placed within the roughness sublayer over a forest, where the turbulence is heavily affected by coherent structures and the instantaneous angle-of-attack can be very large, especially as their site is also sloped. Moreover, the flow is often dominated by coherent structures during stable stratification, resulting in larger angles-of-attack than that tested here.
For the standard deviation of the w-component, Horst et al. (2015) report a relative error of between 3 and 5%, which is almost the same as our error. We suspect the error from our numerical experiment is slightly larger because the turbulence intensity is not quite as large as in the field, where more intense turbulence tends to weaken flow-distortion effects. Moreover, it is interesting that Horst et al. (2015) find the error to be independent of measurement height and stability, implying that the frequency of turbulent fluctuations does not influence measurement error, at least within the range usually found in atmospheric boundary-layer flows. Hence, Horst et al. (2015) also confirm our finding that the flow-distortion error is not systematically different between the X and Y cases, although the w-frequency differs by one order of magnitude.
4.4 Flow-Distortion Correction
The concept of transducer-shadow effects in sonic anemometry actually goes back to the ground-breaking work of Kaimal (1978), see also Kaimal et al. (1990), where a linear dependence of the transducer-shadow effect on the ratio between the path length and transducer diameter was found (which is about 18 for the CSAT3), and
Here, \(v_\mathrm{m}\) is the measured velocity component obtained from each acoustic path separately, and \(\theta \) is the instantaneous angle between the velocity vector and the respective acoustic path. Furthermore, Horst et al. (2015) similarly suggested
which depends on the sine of the flow angle. We applied both corrections (Eqs. 1, 2) to the high-frequency data of our virtual measurements and the results are presented in Table 2. Accordingly, the error of the standard deviation of the w-component indeed becomes smaller after applying the corrections proposed in the literature. Both corrections reduce the error of \(\sigma _w \) by about 1–3%, while the Horst-correction is slightly larger and, therefore, closer to the reference value of our simulations. However, neither of the two corrections fully compensates for the error in \(\sigma _w \).
In the fundamental literature concerning the optimal design of the sonic anemometer (Wyngaard and Zhang 1985; Zhang et al. 1986; Wyngaard 1988), the importance of minimizing flow distortion of the vertical component of the wind velocity is stressed, because the spectrum of the vertical component has its maximum typically at frequencies of about one magnitude larger than the horizontal component (Fig. 3). It is especially important to minimize artificial cross-contamination between these two wind velocity components because such an error cannot be compensated by wind-tunnel-based corrections (Wyngaard 1988). Such problems can be diagnosed by analyzing the correlation coefficient between the horizontal and vertical wind velocity components. However, this correlation coefficient for the momentum flux is in general much lower than for scalar fluxes (Kaimal and Finnigan 1994; Arya 2001), which underlines the statistical independence between the low-frequency fluctuations of the horizontal wind speed and the high-frequency fluctuations of the vertical wind speed. Therefore, the instantaneous wind velocity changes very rapidly in time, which disagrees with the angle-of-attack concept originating from experiments with a constantly tilted instrument in a steady-state flow (van der Molen et al. 2004; Nakai et al. 2006; Nakai and Shimoyama 2012). Our results also show that angles-of-attack larger than \(5{^{\circ }}\) indeed lead to large flow-distortion errors for the mean wind speed, but this error becomes much smaller as soon as oscillations are superimposed on the flow. Obviously, the latter case is closer to conditions in the field. Moreover, no angle-of-attack dependent correction is found to be necessary for the measurement of fluctuations, which is in agreement with Horst et al. (2015).
Finally, in considering the different values of \(\textit{RE}\sigma _{w}\) for the different azimuth angles, the Y-cases, Y0000 and Y6000 behave similarly, as do Y3000 and Y9000, while the X cases do not show the \(60{^{\circ }}\) symmetry. As mentioned in the methods section, this can be expected from the symmetry of the transducer structure and the inflow signal, because the input velocity has no preferred directionality along the z-axis for the Y-cases. Hence, \(\textit{RE}\sigma _{w}\) for a Y case with azimuth angle \(\varphi \) is comparable with \(\textit{RE}\sigma _{w}\) for \(\varphi +60{^{\circ }}\). Grare et al. (2016) also report a similar azimuth dependence of the flow-distortion error for the CSAT3 anemometer based on field and wind-tunnel data.
5 Conclusions
As expected, our study confirms that probe-induced flow distortion of the CSAT3 anemometer causes a dampening of both horizontal and vertical wind velocity components for all the azimuth angles and mean angles-of-attack that were tested. This overall dampening effect is caused by the drag in the first few millimetres of the measurement path in the direct vicinity of the sonic transducer, while the velocity magnitude in the centre of the measurement path is often even increased. Furthermore, our experiment confirms that probe-induced flow distortion is much smaller for unsteady wind velocities than under constant flow conditions.
Whether these fluctuations occur at frequencies of 1 or 0.1 Hz is of minor importance. Hence, the errors reported here can be considered as being spectrum-independent to some extent, for wind fluctuations of a magnitude typical for surface-layer turbulence (\(\mathcal{O}(1\,\hbox {m}\,\hbox {s}^{-1})\)). Hence, neither stability nor measurement height influences the flow-distortion error significantly, which is in agreement with the Horst et al. (2015). Our findings also explain why wind-tunnel-based experiments with quasi-laminar flow conditions typically result in larger flow-distortion errors than field intercomparisons, and why wind-tunnel-based corrections are not transferable to turbulence measurements in the field.
We found that the CSAT3 anemometer has an improved accuracy for the measurement of fluctuations of the u-component than of the w-component, implying that the flow distortion could be reduced if the instrument were rotated by \(90{^{\circ }}\), which is in agreement with the results from a field comparison of vertically- and horizontally-mounted CSAT3 anemometers (Frank et al. 2013). When mounted vertically, the probe-induced dampening effect is of 3–7% for the w-fluctuations, depending on the azimuth angle, while it is only 1–3% for the u-fluctuations. However, the regular vertical orientation probably results in a higher precision of the w-measurement.
The results for \(\textit{RE}\sigma _{w}\) are larger than the difference between different instruments of the same model found during field intercomparisons (Mauder et al. 2007). Hence, if the numerical experiment is chosen as the etalon, then a systematic error of about 5% would need to be added to the results of in situ comparisons. Reconsidering the findings of Mauder et al. (2007) for \(\sigma _{w}\), the agreement of the CSAT3 anemometer with some models would improve (ATI-K, R.M. Young), but become worse with others (Solent-HS, USA-1, NUW).
The transducer-shadowing correction proposed by Horst et al. (2015) reduces the magnitude of the observed probe-induced dampening effect, but it does not reflect the azimuth dependence of this error. A remaining flow-distortion error translates directly into an underestimation of scalar fluxes, such as the fluxes of sensible heat, latent heat, and \(\hbox {CO}_{2}\), and this error will be as large as the error for \(\sigma _{w}\), assuming that the scalar measurement does not produce any additional flow distortion due to the presence of gas analyzers.
Large-eddy simulation has been successfully applied to characterize the flow distortion of a popular sonic anemometer. Similar studies may be conducted for other sonic-anemometer models in the future. Moreover, large-eddy simulation may also be used to optimize the structural design of sonic anemometers or to develop new correction algorithms.
References
Arya SP (2001) Introduction to micrometeorology. Academic Press, San Diego, 415 pp
Baldocchi D (2014) Measuring fluxes of trace gases and energy between ecosystems and the atmosphere—the state and future of the eddy covariance method. Glob Change Biol 20:3600–3609. doi:10.1111/gcb.12649
Campbell DT, Stanley JC (1963) Experimental and quasi-experimental designs for research. NIDA Res Monogr 107:140–158
Christen A, van Gorsel E, Vogt R, Andretta M, Rotach MW (2001) Ultrasonic anemometer instrumentation at steep slopes: wind tunnel study—field comaprison measurements. MAP Newsl 15:164–167
Dyer AJ (1981) Flow distortion by supporting structures. Boundary-Layer Meteorol 20:363–372
Dyer AJ, Garratt JR, Francey RJ, McIlroy IC, Bacon NE, Bradley EF, Denmead OT, Tsvang LR, Volkov YA, Koprov BM, Elagina LG, Sahashi K, Monji N, Hanafusa T, Tsukamoto O, Frenzen P, Hicks BB, Wesely M, Miyake M, Shaw W (1982) An international turbulence comparison experiment (ITCE-76). Boundary-Layer Meteorol 24:181–209
Foken T, Oncley SP (1995) Workshop on instrumental and methodical problems of land surface flux measurements. Bull Am Meteorol Soc 76:1191–1193
Frank JM, Massman WJ, Ewers BE (2013) Underestimates of sensible heat flux due to vertical velocity measurement errors in non-orthogonal sonic anemometers. Agric For Meteorol 171–172:72–81. doi:10.1016/j.agrformet.2012.11.005
Friebel HC, Herrington TO, Belinov AY, Benilov AY, Belinov AY, Benilov AY (2009) Evaluation of the flow distortion around the Campbell Scientific CSAT3 sonic anemometer relative to incident wind direction. J Atmos Ocean Technol 26:582–592. doi:10.1175/2008JTECHO550.1
Fureby C, Tabor G, Weller H, Gosman A (1997) A comparative study of subgrid scale models in homogeneous isotropic turbulence. Phys Fluids 9:1416–1429. doi:10.1063/1.869254
Grare L, Lenain L, Melville WK (2016) The influence of wind direction on Campbell Scientific CSAT3 and Gill R3-50 sonic anemometer measurements. J Atmos Ocean Technol 33:2477–2497. doi:10.1175/JTECH-D-16-0055.1
Goodrich JPP, Oechel WCC, Gioli B, Moreaux V, Murphy PCC, Burba G, Zona D (2016) Impact of different eddy covariance sensors, site set-up, and maintenance on the annual balance of CO2 and CH4 in the harsh Arctic environment. Agric For Meteorol 228–229:239–251. doi:10.1016/j.agrformet.2016.07.008
Hanafusa T, Fujitani T, Kobori Y, Mitsuta Y (1982) A new type sonic anemometer–thermometer for field operation. Pap Meteorol Geophys 33:1–19
Högström U, Smedman AS (2004) Accuracy of sonic anemometers: laminar wind-tunnel calibrations compared to atmospheric in situ calibrations against a reference instrument. Boundary-Layer Meteorol 111:33–54. doi:10.1023/B:BOUN.0000011000.05248.47
Horst TW, Semmer SR, Maclean G (2015) Correction of a non-orthogonal, three-component sonic anemometer for flow distortion by transducer shadowing. Boundary-Layer Meteorol 155:371–395. doi:10.1007/s10546-015-0010-3
Issa R (1985) Solution of the implicitly dsicretized fluid flow equations by operator-splitting. J Comput Phys 62:40–65
Jiménez-Buedo M, Miller LM (2010) Why a trade-off? The relationship between the external and internal validity of experiments. Theoria 69:301–321. doi:10.1387/theoria.779
Kaimal J (1978) Sonic anemometer measurement of atmospheric turbulence. In: Hanson BW (ed) Proceedings of the dynamic flow conference 1978 on dynamic measurements in unsteady flows. Springer, Dordrecht, pp 551–565
Kaimal JC (2013) Advances in meteorology and the evolution of sonic anemometry. http://www.apptech.com/wp-content/uploads/2016/08/Evolution-of-Sonic-Anemometry.pdf. Accessed 4 Feb 2017
Kaimal JC, Gaynor JE (1983) The boulder atmospheric observatory. J Appl Meteorol 22:863–880
Kaimal JC, Finnigan JJ (1994) Atmospheric boundary layer flows: their structure and measurement. Oxford University Press, New York
Kaimal JC, Wyngaard JC, Izumi Y, Cote OR (1972) Spectral characteristics of surface layer turbulence. Q J R Meteorol Soc 53:103–115
Kaimal JC, Gaynor JE, Zimmerman HA, Zimmerman GA (1990) Minimizing flow distortion errors in a sonic anemometer. Boundary-Layer Meteorol 53:103–115. doi:10.1007/BF00122466
Karniadakis GE, Triantafyllou GS (1989) Frequency selection and asymptotic states in laminar wakes. J Fluid Mech 199:441–469. doi:10.1017/S0022112089000431
Kochendorfer J, Meyers TP, Heuer MW, Frank JM, Massman WJ, Heuer MW, Frank JM, Massman WJ (2012) How well can we measure the vertical wind speed? Implications for the fluxes of energy and mass. Boundary-Layer Meteorol 145:383–398. doi:10.1007/s10546-012-9738-1
Li M, Babel W, Tanaka K, Foken T (2013) Note on the application of planar-fit rotation for non-omnidirectional sonic anemometers. Atmos Meas Tech 6:221–229. doi:10.5194/amt-6-221-2013
Loescher HW, Ocheltree T, Tanner B, Swiatek E, Dano B, Wong J, Zimmerman G, Campbell J, Stock C, Jacobsen L, Shiga Y, Kollas J, Liburdy J, Law BE (2005) Comparison of temperature and wind statistics in contrasting environments among different sonic anemometer-thermometers. Agric For Meteorol 133:119–139. doi:10.1016/j.agrformet.2005.08.009
Mauder M (2013) A comment on “How well can we measure the vertical wind speed? Implications for fluxes of energy and mass” by Kochendorfer et al. Boundary-Layer Meteorol 147:329–335. doi:10.1007/s10546-012-9794-6
Mauder M, Liebethal C, Göckede M, Leps JP, Beyrich F, Foken T (2006) Processing and quality control of flux data during LITFASS-2003. Boundary-Layer Meteorol 121:67–88. doi:10.1007/s10546-006-9094-0
Mauder M, Oncley SP, Vogt R, Weidinger T, Ribeiro L, Bernhofer C, Foken T, Kohsiek W, Bruin HaR, Liu H (2007) The energy balance experiment EBEX-2000. Part II: intercomparison of eddy-covariance sensors and post-field data processing methods. Boundary-Layer Meteorol 123:29–54. doi:10.1007/s10546-006-9139-4
Miyake M, Stewart RW, Burling HW, Tsvang LR, Koprov BM, Kuznetsov OA (1971) Comparison of acoustic instruments in an atmospheric turbulent flow over water. Boundary-Layer Meteorol 2:228–245
Nakai T, Shimoyama K (2012) Ultrasonic anemometer angle of attack errors under turbulent conditions. Agric For Meteorol 162–163:14–26. doi:10.1016/j.agrformet.2012.04.004
Nakai T, van der Molen MK, Gash JHC, Kodama Y (2006) Correction of sonic anemometer angle of attack errors. Agric For Meteorol 136:19–30. doi:10.1016/j.agrformet.2006.01.006
Roe BE, Just DR (2009) Internal and extrernal validity in economics research: tradeoffs between experiments and field data. Am J Agric Econ 91:1266–1271. doi:10.1111/j.1467-8276.2009.01295.x
Sakamoto H, Haniu H (1990) A study on vortex shedding from spheres in a uniform flow. J Fluid Eng 112:386–392. doi:10.1115/1.2909415
SanClements MD, Metzger S, Luo H, Pingintha-Durden N, Zulueta R, Loescher HW (2014) The National Ecological Observatory Network (NEON): providing free long-term ecological data on a continental scale. iLEAPS Newsletter. Issue Spec Issue Environ Res Infrastruct 23–26
Schlegel F, Stiller J, Bienert A, Maas HG, Queck R, Bernhofer C (2012) Large-eddy simulation of inhomogeneous canopy flows using high resolution terrestrial laser scanning data. Boundary-Layer Meteorol 142:223–243. doi:10.1007/s10546-011-9678-1
Tsvang LR, Koprov BM, Zubkovskii SL, Dyer AJ, Hicks B, Miyake M, Stewart RW, McDonald JW (1973) A comparison of turbulence measurements by different instruments; Tsimlyansk field experiment 1970. Boundary-Layer Meteorol 3:499–521
Tsvang LR, Zubkovskij SL, Kader BA, Kallistratova MA, Foken T, Gerstmann W, Przandka Z, Pretel J, Zelenny J, Keder J (1985) International turbulence comparison experiment (ITCE-81). Boundary-Layer Meteorol 31:325–348
van der Molen M, Gash JH, Elbers J (2004) Sonic anemometer (co)sine response and flux measurement. Agric For Meteorol 122:95–109. doi:10.1016/j.agrformet.2003.09.003
Weller HG, Tabor G, Jasak H, Fureby C (1998) A tensorial approach to computational continuum mechanics using object-oriented techniques. Comput Phys 12:620–631. doi:10.1063/1.168744
Wieser A, Fiedler F, Corsmeier U (2001) The influence of the sensor design on wind measurements with sonci anemometer systems. J Atmos Ocean Technol 18:1585–1608
Williamson CHK (1996) Vortex dynamics in the cylinder wake. Annu Rev Fluid Mech 28:477–539. doi:10.1146/annurev.fl.28.010196.002401
Wyngaard JC (1981) The effects of probe-induced flow distortion on atmospheric turbulence measurements. J Appl Meteorol 20:784–794. doi:10.1175/1520-0469(1988)045<3400:TEOPIF>2.0.CO;2
Wyngaard JC (1988) Flow-distortion effects on scalar flux measurements in the surface layer: implications for sensor design. Boundary-Layer Meteorol 42:19–26. doi:10.1007/BF00119872
Wyngaard JC, Zhang S-FF (1985) Transducer-shadow effects on turbulence spectra measured by sonic anemometers. J Atmos Ocean Technol 2:548–558. doi:10.1175/1520-0426(1985)002<0548:TSEOTS>2.0.CO;2
Yu GR, Wen X-F, Sun X-M, Tanner BD, Lee X, Chen J-Y (2006) Overview of ChinaFLUX and evaluation of its eddy covariance measurement. Agric For Meteorol 137:125–137
Zacharias S, Bogena H, Samaniego L, Mauder M, Fuß R, Pütz T, Frenzel M, Schwank M, Baessler C, Butterbach-Bahl K, Bens O (2011) A network of terrestrial environmental observatories in Germany. Vadose Zone J 10:955–973
Zhang SF, Wyngaard JC, Businger JA, Oncley SP (1986) Response characteristics of the U.W. sonic anemometer. J Atmos Ocean Technol 3:315–323. doi:10.1175/1520-0426(1986)003<0315:RCOTUS>2.0.CO;2
Acknowledgements
This work was conducted within the Helmholtz Young Investigator Group “Capturing all relevant scales of biosphere-atmosphere exchange—the enigmatic energy balance closure problem”, which is funded by the Helmholtz-Association through the President’s Initiative and Networking Fund, and by KIT. We also gratefully acknowledge Michael Manhart (Technical University of Munich) for fruitful discussions about the simulation set-up, and we thank Campbell Scientific for providing a CAD model of the CSAT3 geometry.
Author information
Authors and Affiliations
Corresponding author
Rights and permissions
About this article
Cite this article
Huq, S., De Roo, F., Foken, T. et al. Evaluation of Probe-Induced Flow Distortion of Campbell CSAT3 Sonic Anemometers by Numerical Simulation. Boundary-Layer Meteorol 165, 9–28 (2017). https://doi.org/10.1007/s10546-017-0264-z
Received:
Accepted:
Published:
Issue Date:
DOI: https://doi.org/10.1007/s10546-017-0264-z