Abstract
We tested two models to identify the genetic and environmental processes underlying longitudinal changes in depression among adolescents. The first assumes that observed changes in covariance structure result from the unfolding of inherent, random individual differences in the overall levels and rates of change in depression over time (random growth curves). The second assumes that observed changes are due to time-specific random effects (innovations) accumulating over time (autoregressive effects). We found little evidence of age-specific genetic effects or persistent genetic innovations. Instead, genetic effects are consistent with a gradual unfolding in the liability to depression and rates of change with increasing age. Likewise, the environment also creates significant individual differences in overall levels of depression and rates of change. However, there are also time-specific environmental experiences that persist with fidelity. The implications of these differing genetic and environmental mechanisms in the etiology of depression are considered.
Similar content being viewed by others
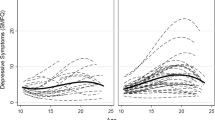
Avoid common mistakes on your manuscript.
Introduction
Rates of depression change markedly through adolescence in boys and girls but few studies (if any) have addressed the underlying genetic and environmental processes that account for individual differences in the developmental trajectory of adolescent depression. Several studies have shown that individual differences in liability to juvenile depression are moderately heritable and that the relative contribution of genetic differences increases with age (e.g. Eley and Stevenson 1999; Rice et al. 2002; Silberg et al. 1999, 2001; Thapar and McGuffin 1994). Furthermore, such longitudinal twin data as have been explored (e.g. Bergen et al. 2007; Eley and Stevenson 1999; Kendler et al. 2008; Lau and Eley 2006; Thapar and McGuffin 1994) suggest that there is some partial cross-temporal consistency in the genetic contribution to depression, possibly reflecting the stable contribution of the same genes across development. In spite of this overall consistency, however, there are also apparently genetic factors whose expression is age-specific.
Such studies, however, have significant weaknesses. Although they describe the pattern of longitudinal change, they do not attempt to resolve the underlying developmental genetic process that generates the empirical patterns of twin resemblance across time. There are two principal shortcomings of these earlier analyses. Firstly, although they involve repeated measures, measures on different occasions are pooled over a relatively large developmental time-span, limiting the precision with which age-dependent genetic and environmental influences can be resolved. Secondly, the model that is used to account for the pattern of genetic variation and covariation over time is the atheoretical “Cholesky decomposition” that merely describes patterns of change but does not attempt to account for them in terms of one or more simpler and, potentially, more informative theoretical possibilities. A simple example of the problem with the conventional approach is that the same basic genetic covariances admit series of more-or-less arbitrary explanations beyond the one that is advanced in the literature. For example, the Cholesky decomposition that is often employed assumes that genetic variation increases over time due to the progressive accumulation of novel age-specific genetic differences at each age that, once “switched on” are expressed at subsequent ages. Formally, this published account is indistinguishable from the radically different explanation that some sets of genes expressed at earlier stages in development are progressively “switched off” as development proceeds.
Different models for the roles of genes and environment in development can lead to different predictions about the patterns of correlation in longitudinal data on family members. For example, the variances and covariances of repeated measures in longitudinal twin data may depend on individual genetic and/or environmental differences in inherent growth patterns unfolding with age (“random growth curves”). Alternatively, or concurrently, they may arise when time-specific random genetic or environmental effects are more of less persistent over time (“autoregressive effects”). As an example, (Gillespie et al. 2004a) fitted auto-regresive models to longitudinal neuroticism data from adolescent twins and found that additive genetic variance observed at ages 14 and 16 could be explained by genetic effects at age 12 years. There was also evidence for smaller but significant genetic innovations at 14 and 16 years. The data were limited to three time points and they did not test competing ‘latent growth’ or combinatory ‘dual change’ models. Among adults, found that genetic determinants of anxiety and depression appear to be relatively stable across the lifespan for males and females (Gillespie et al. 2004b). There is some evidence to support additional mid-life and late age gene action in females for depression (Gillespie et al. 2004b).
These findings illustrate how different developmental processes may apply to the genetic and environmental components of developmental change. It is conceivable, for example, that genetic influences account for individual differences in levels and rates of change, while autoregressive effects account for the remembering or forgetting of time-dependent non-genetic influences. Efforts to combine the feature of autoregressive (Eaves et al. 1986) with those from standard latent growth models (McArdle 1994; McArdle and Anderson 1989; McArdle and Hamagami 1991; Nesselroade and Baltes 1974), which are mathematically and statistically equivalent to random coefficient, multilevel or hierarchical linear models (Bryk and Raudenbush 1987; McArdle 1988; McArdle and Hamagami 1992; McArdle et al. 1991; Mehta and West 2000; Miyazaki and Raudenbush 2000), have been applied to cognition (Finkel et al. 2007; McArdle 1986) and drug availability (Gillespie et al. 2007). One recent report has fitted a dynamic model to depression (Hishinuma et al. 2012) but not within a genetically informative framework. The current paper attempts to unravel such a series of contrasting models for the unfolding patterns of genetic and environmental differences in depression in a longitudinal study of juvenile twins.
Methods
Data
The data used for this analysis were derived from repeated measures of depression symptomatology of twins taking part in the longitudinal Virginia Twin Study of Adolescent Behavioral Development (“VTSABD”). The overall composition of the sample by age, sex and zygosity at the time of initial assessment and up to four follow-ups is summarized in Tables 1, 2. Pairs were studied at approximately 2-year intervals until they reached age 18 at which point they became eligible for a young adult follow-up (“YAFU”) using a different protocol, not considered further in this study. Data were organized by year based on the ages at which twins were assessed. This varied between 1 and 4 occasions between the ages of 8 and 18, and as a result, the sample sizes used to estimate correlations between measurement occasions also varied.
Details of ascertainment, demographics, zygosity diagnosis, assessment and prevalence rates for key adolescent psychiatric outcomes are provided elsewhere (Meyer et al. 1996; Hewitt et al. 1997; Eaves et al. 1997; Simonoff et al. 1997). In this application, depressive symptomatology was assessed by the sum of keyed responses to items for the long form of the Mood and Feelings Questionnaire (Angold et al. 1995; Costello and Angold 1988; see also http://devepi.duhs.duke.edu/mfq.html). Item response theoretical (IRT) modeling of the MFQ items from the VTSABD is described in detail elsewhere (Eaves et al. 2005). The scale comprised 33 three category items, coded 0, 1, 2 yielding raw depression scores in the range 0–66 where 0 denotes completely asymptomatic individuals.
Cross-temporal patterns in genetic and environmental effects
The starting point for any attempt to elucidate the developmental processes that characterize cross-temporal change in genetic and environmental components is the basic structure of genetic and environmental variation and covariation as a function of age. We obtained maximum-likelihood (ML) estimates of the cross-temporal additive genetic (A) and within-family, non-shared, environmental (E) covariance matrices on the assumption that mating was random and any effects of the shared family environment (C) are negligible. Previous genetic analysis of depression in adults and juveniles suggest that these assumptions are appropriate to a reasonable approximation. The cross-temporal phenotypic covariances are therefore:
Given the assumptions of this model, the matrix of covariances between the repeated measures across first and second twins is T MZ = A. The corresponding covariances for the first and second twins of DZ pairs is expected to be T DZ = ½A. Therefore, the entire covariance structure within and between MZ pairs is expected to be the partitioned matrix:
Whereas for DZ pairs, the expected partitioned matrix is:
Using the Cholesky decomposition, the genetic and environmental covariance matrices were constrained to be positive definite by decomposing them into the products A = a × a′ and E = e × e′ where a and e are lower triangular matrices. Since we are primarily interested in the random genetic and environmental differences between subjects and not in the average trends in depression as a function of age, we treat the mean depression scores at each age as nuisance variables, and allow each to take its own value by age and sex without imposing any structure on the means. Note that this model was only fitted to provide a baseline model against which other theoretical models were compared.
ML estimation of the parameters of the basic model was implemented using “classic” Mx (Neale and Cardon 1992) using six groups of twins: male MZ, female MZ, male DZ, female DZ, male–female DZ and female–male DZ. The covariance structure was assumed to be the same in boys and girls. Tests of heterogeneity of parameter values in the saturated Cholesky across male and female like-sex pairs suggested that the assumption of homogeneity across sexes of genetic and environmental variances is generally justified by the data (∆χ 2(120) = 109.89, P = 0.73).
Figure 1 shows the estimates of the total variance and the genetic and environmental components of variance as a function age. Table 3 shows the means of self-report depression scores at each age by sex. The figure confirms the published findings of a modest contribution of genetic influences on liability to depression that increases with age. The contribution of unique environmental influences at each age is substantially larger initially than that of genetic effects but may tend to attenuate somewhat with advancing age. Linear interpolation from the estimates in Fig. 1 yields an approximate heritability on 0.39 at age 8 years, rising to 0.46 at age 18. Both these estimates are consistent with values reported in the literature for single occasions of measurement.
Figure 2 illustrates the changes in the age-lagged genetic and environmental correlations estimated from the ML genetic (A) and environmental covariance matrices (E). Results are presented for the trends in genetic and environmental correlations between measures made at intervals of 2 and 4 years as a function of the age of the first occasion of measurement. Unweighted linear trends are superimposed on the point estimates.
The graphs show three principal trends. First, cross-temporal genetic correlations are higher than the corresponding environmental correlations implying greater stability of genetic influences during adolescent development than environmental differences. That is, effects of the environment are more age-specific than effects of the genes. This finding is consistent with longitudinal data on the genetic control of adult depression (Gillespie et al. 2004b). Second, both genetic and environmental cross-temporal correlations increase with age implying that there is some accumulating stability of both effects with increasing age during adolescence. Finally, both genetic and environmental correlations decrease as a function of increasing lag between repeated measures. Taken together, these findings imply that there may be a dynamic mechanism underlying the development of depression that increases the apparent contribution of differences with progress through adolescence, results in increased genetic and environmental stability over time yet shows a developmental pattern that leads to a progressive decay (“forgetting”) of earlier genetic and environmental influences as adolescence unfolds.
Model for the covariance structure of genetic and environmental effects on repeated measures
The results in Figs. 1, 2 are purely descriptive and call for further analysis in order to elucidate the possible underlying processes of developmental change in the effects of genes and environment on the patterns of twin resemblance within and between ages. The broad qualitative findings summarized above may arise from either or both of two different conceptions of how development proceeds (see Gillespie et al. 2007).
Autoregression models
As described by Eaves (Eaves et al. 1986, see also Boomsma et al. 1989; Boomsma and Molenaar 1987) the first “autoregressive” feature of the model assumes that the observed covariance structure arises because random, age-specific genetic and/or environmental effects arise at each time t. The “innovations” reflect novel, time-specific genetic effects or environmental influences. Genetic and/or environmental differences at time t are partly a function of new random effects on the phenotype that arise at time t and partly a (linear) function of differences expressed at an earlier time, t−1. The cross temporal correlations within subjects arise because the innovations have a more or less persistent effect over time and may, under some circumstances accumulate during development, giving rise to a developmental increase in genetic and/or environmental variance and increased correlation between adjacent measures. Examples of autoregressive genetic and environmental effects have been described for a variety of human traits (Boomsma et al. 1989; Boomsma and Molenaar 1987; Cornes et al. 2007; Eaves et al. 1986; Gillespie et al. 2004a, b). One consequence of the autoregressive model is the tendency of cross-temporal correlations to decay as a function of increasing separation in time. Depending on the magnitude of the innovation and their relative persistence, the observed variances and cross-temporal covariances may increase during development towards a stable asymptotic value (see e.g. Eaves et al. 1986, for some graphical examples of an application to longitudinal cognitive data).
Growth curve models
The second component of the model is conceptually different from the autoregressive model, although in some forms may lead to very similar predictions about the covariance structure of repeated measures. This model regards developmental change as the unfolding of inherent, random genetic and environmental differences in the level and rates of change in behavior over time. “Change” may involve both linear and non-linear components. In different contexts, such models have been referred to as “random regression” models, “hierarchial mixed models” or “latent growth curve models (Duncan and Duncan 1991; Duncan et al. 1994; McArdle 1994; McArdle and Epstein 1987; Nesselroade and Baltes 1974). These models correspond to special cases of the factor model in which factor loadings from the level (intercept) and change factors are functions of the coefficients of a priori contrasts on the levels of age at which the repeated measures were taken. In the simplest model, random effects may only affect the overall level of trait expression rather than any predicted rate of change. This model will yield a single common factor with equal loadings across all occasions of measurement and predicts equal variances over occasions and equal cross-temporal correlations.
Combine autoregressive and growth curve modeling
Figure 3 summarizes the principal features of these two models diagrammatically. For simplicity, the figure only considers the elements of the model without distinction between genetic and environmental components, although our analysis treats both independently to allow for the possibility that different processes underlie genetic and environmental components of developmental change (McArdle and Hamagami 2003; McArdle et al. 2004).
Structural model of developmental change attributable to auto-regressive and growth curve components. Note The diagram includes both constant and “change” random effects on growth. The Model is easily elaborated to reflect higher order components of growth and can be applied to genetic or environmental components of developmental change or both
If we let Σ be a covariance matrix (P, A or E) in (1) above, then the model for the covariance structure is:
where \({\varvec{\Lambda}}\) is the orthonormal matrix of cross-temporal contrasts or the factor loadings (λ) from the constant (\(\uplambda\) = 1) and linear slope (\(\uplambda\) 8 − \(\uplambda\) 18), and which are shown in Table 4. \({\varvec{\Gamma}}\) is the variance–covariance matrix for the constant and change (linear, quadratic etc.) components of growth. \({\varvec{\Theta}}\) is a diagonal matrix of error (\(\upepsilon\)) variances for the latent depression factors at each age (Age8–Age18) not explained by the constant and change factors. Likewise, \({\varvec{\Psi}}\) is a diagonal matrix for the item-specific or residual (\(\uppsi\)) variances in the observed depression scores (P8–P18). Finally, \(\varvec{\upbeta}\) is the matrix of first-order, freely estimated autoregressive coefficients (β) that represent the accumulation of risks explained by the constant and change factors including latent residual error over time.
The model allows for different \({\varvec{\Gamma}}\), \({\varvec{\Theta}}\), \({\varvec{\Psi}}\) and \(\varvec{\upbeta}\) when estimating the expected genetic (A) and environmental (E) covariance matrices. The matrix of cross-temporal factor loadings (λ) remain constant. The covariance matrix (\({\varvec{\Gamma}}\)) between the components of growth may be parameterized in terms of a diagonal matrix of variances, \(\Delta\), and the correlations, R, between the random effects on the constants, linear and quadratic components of growth, thus \(\Delta^{1/2} {\mathbf{RP}} \Delta^{1/2}\).
Again, the model parameters may be defined separately for the genetic and environmental components of growth to allow for different processes underlying the genetic and environmental components of individual differences in development. The parameters for the different components of the model are constrained to be the same for different ages in the model.
Model-fitting method
Various constellations of the parameters of the developmental model were fitted to the raw data by full ML (see Lange et al. 1976) using the classical Mx package (Neale et al. 2004). Since the principal focus of the analysis was elucidation of the covariance structure of individual differences in change, the means were allowed to take their own values as a function of age and sex, with the constraint that the means were equated across zygosity groups and between the fist and second members of twin pairs, except in the case of unlike-sex pairs where the mean vectors appropriate for males and females were substituted as appropriate.
Results
Model comparison statistics are shown in Table 5. Three models were fitted initially. Model 1 assumes the standard Cholesky decomposition whereby the genetic and environmental covariances are unstructured, apart from the requirement that they are positive definite. Model 2 assumes that there is no genetic or environmental covariance across ages i.e. that neither genes nor environment show any characteristics of random growth effects or autoregression. This model allows only for occasion-specific genetic and environmental effects. Elements of the genetic and environmental specific variances were allowed to vary across time. Models 1 and 2 represent reasonable extremes of good and bad fit against which other models may be compared. Model 3 is the most complex developmental model that can be fitted to these data: 2nd order random growth curves with linear and quadratic rates of changes; combined with first-order autoregressive effects for both genes and environment. In all models the means were estimated as free parameters so that mean effects of age, sex and the interaction of age and sex were allowed to take their own values. Therefore, comparisons of different models for development are based only on their relative abilities to account for the covariance structure of the repeated measures of depressive symptomatology in twins.
As expected, the fit of Model 2 provided an extremely bad fit compared with the most general Model 1 (\(\Delta \upchi^{2}_{{\left( {76} \right)}}\) = 964.47, P < 10−5) reflecting the significant cross-temporal consistency of the causes of depression in adolescence. By comparison, the fit of the basic developmental model (Model 3), combining random second order genetic and environmental effects on growth, together with autoregressive effects on both genetic and environmental influences gives a good fit in comparison with the general model (\(\Delta \upchi^{2}_{{\left( {102} \right)}}\) = 116.68, P = 0.15).
We then compared a variety of models nested within the fairly general but saturated developmental model. Our approach was to identify broad simplifications, judged by the Akaike information criterion (AIC) that could be accomplished without sacrificing goodness of fit. Before proceeding, tests of heterogeneity of parameter values in this saturated developmental model across male and female like-sex pairs suggested that the assumption of homogeneity across sexes of genetic and environmental variances was again justified by the data (\(\Delta \upchi^{2}_{{\left( {18} \right)}}\) = 21.18, P = 0.27). Given no support for sex differences in the covariance structure all models were based on the same covariance structure for boys and girls in Table 5. It is conceivable that larger samples might require models that account for heterogeneity of the covariance structure across sexes.
Models 4, 5 and 6 (Table 5) removed sequentially the random quadratic (Q), linear (L) and constant (C) differences from both the genetic and environmental covariance structures while retaining autoregressive components (β) in both. Dropping both the genetic and environmental quadratic components of growth jointly (Model 4) resulted in a marginally significant change in fit (\(\Delta \upchi^{ 2}_{\left( 6\right)}\) = 14.04, P = 2.9 × 10−2). The data resisted attempts to remove the random genetic and environmental differences in overall elevation and linear change (Models 5 and 6) and resulted in much larger AIC.
Models 7, 8 and 9 examined the effect of removing autoregressive effects from the developmental Model 3. Removing any autoregressive effects from the genetic covariance structure (Model 7) resulted in no appreciable loss of fit (\(\Delta \upchi^{2}_{\left( 2 \right)}\) = 0.20, P = 0.74) while providing the lowest AIC (−91.28) achieved in this analysis. By comparison, dropping the non-genetic autoregressive component (Model 8) made the fit appreciably worse (\(\Delta \upchi^{2}_{\left( 2 \right)}\) = 8.53, P = 1.4 × 10−2) suggesting that a significant component of environmental correlation in depression across adolescence is due to the relative persistence of individual, time-specific, environmental experiences whose effects gradually decay across time. This appears to be in contrast to the genetic components that change significantly across adolescence, but reflect the temporal unfolding of inherited genetic differences in developmental trajectory.
The best model (Model 7) assumes 2nd order random growth effects on both genetic and environmental covariance structure, with the addition of autoregressive effects on the environmental structure. Models 10 and 11 represent post hoc attempts to simplify this structure further. Model 10 drops the environmental component of quadratic change. The quadratic genetic component is removed as a further step in Model 11. Removing the environmental quadratic component resulted only in a marginal deterioration in fit and increase in AIC. Attempting to remove the quadratic genetic component leads to a relatively bad fit and a much larger increase in AIC. Model 10 was subsequently judged the best fitting. ML estimates of the parameters of this best fitting model (Model 10) are given in Table 6.
Figure 4 shows the patterns of change in the environmental, genetic and phenotypic variances predicted from the best fitting model. Figures 5, 6 show the pattern of predicted cross-temporal correlations derived from the expected environmental and additive genetic covariance matrices respectively.
The estimates in Fig. 4 depict the trends in the components of variance obtained for the unconstrained genetic and environmental Model 10. There is a slight tendency for the total variance to increase with age but the effect is not marked. The average contribution of the within-family environment is somewhat larger than that of genetic effects across adolescence with a modest overall tendency for environmental differences to increase with age. Overall, however, the total variance with the genetic and environmental components show relatively small changes with age.
Figure 5 shows the predicted cross-temporal non-shared environmental correlations based on the best fitting Model 10 as a function of initial age and interval between measurements ranging from 1 through 5 years. Though significant, these environmental correlations were not large, and this is indicative of the small variance due to differences in elevation (“constant” differences over time) in the estimates of non-shared environmental parameters in Table 6. Instead of changing markedly as a function of age, as indicated by the variance attributable to “linear” increases in differences over time (see Table 6), the cross-temporal non-shared environmental correlations tended to decay as a function of the increasing interval between assessments. Thus, measures separated by 1 year are approximately twice as correlated as measures separated by 5 years. As shown in Table 6, compared to additive genetic effects, the variance attributable to the environmental constant and linear rate of change were smaller. The significant autoregressive component (β) in Model 10 over 1 year intervals nevertheless suggests that at least some individual environmental experiences are enduring.
As shown in Fig. 6, the pattern for additive genetic effects is quite different from that emerging for the environment. Firstly, the overall level of genetic correlation across time is much higher (>0.7 for measures separated by 1 year) compared with that for environmental effects (~0.4). These effects are reflected in the large variance due to differences in elevation (“constant” differences over time) in the estimates of genetic parameters in Table 6. The variation in cross-temporal genetic correlations as a function of initial age at measurement is greater for younger children (see initial age 8 years in Fig. 6) and gradually decreases in older children.
Discussion
Our combined autoregressive and growth curve modeling using multi-wave data on adolescent depression provides compelling support for distinct mechanisms underlying genetic effects on adolescent development compared with environmental effects.
Considering the environmental effects first, it is apparent that juveniles experience two types of environment during adolescence. The first are generally more or less depressogenic environments that operate throughout this part of the lifespan. These differences contribute to the random differences in the overall levels (C) and linear (L) rates of change in the environmental component of Model 10. However, compared with the variances of the latent genetic effects on growth, these initial and unfolding contributions of the environment in depression throughout adolescence are small. Indeed, the genetic variance on differences in the average levels during adolescence is 6.649 compared with 1.346, almost a five-fold difference.
The second and much larger source of environmental effects are those arising from all short-term influences including those contributing to measurement error, rather than to any persistent, unfolding and long-term external adversity. These short-term effects are indicated by the small cross-lagged environmental correlations which point to a much higher degree of age-specificity in the relative protective and depressogenic experiences of adolescents. Thus a child, even an identical twin, who is exposed to an environmental insult at one time might, at another, have the benefit of a protective effect not shared with his or her cotwin. Note however, that the relatively rapid decay in cross-temporal correlations that would follow if all environmental experiences were transient and forgotten over time is not borne out by our data—the small non-shared environmental autoregressive component (Model 10) remained significant over 1 year intervals (β = ~0.4). This suggests that at least some individual environmental experiences are enduring, carry forward, and contribute significantly to future random effects on overall depression levels in the model for environmental differences. So although we expect some experiences of individual twins to remain persistent and accumulate throughout development, perhaps pointing to long-term developmental effects of experiences that precede the onset of measurement, there is much stronger support instead for large time-specific environmental effects whose effects are mostly and rapidly forgotten.
We now consider the genetic effects. The cross-lagged structure of genetic effects on depression cannot simply be ascribed to differences in juveniles’ initial average (“Constant”) levels of genetic liability. Compared to the environment, there remain larger and significant genetic effects on both types (linear and quadratic) of developmental change. Genetic variance in linear rates of change with age (1.335) is approximately twice that seen for the contributions of the environment to change (0.658). Also, the small but significant quadratic relationship between genetic variability and age is commensurate with a slight burst in genetic variability observed in correlated measures of neuroticism in other adolescent pubertal samples, (Gillespie et al. 2004a). Once differences in the level and rates of genetic change are accounted for, any age-specific (Θ), residual (Ψ) and enduring (β) genetic effects are relatively small or completely absent. The absence of any significant autoregressive effects in the model suggests a relative static role for the genetic causes of developmental change which, apart from quite modest age-specific effects, have no accumulative impact. Instead, our results strongly suggest that the bulk of genetic differences are due to overall levels of genetic liability at the start of adolescence and which unfold over time.
Thus, the patterns of developmental changes in genetic variance and covariance cannot be ascribed to the long- term persistence and accumulation of age-specific random genetic effects. Any apparent age changes in genetic variance simply represent the successive expression of differences in the inherent unfolding developmental trajectory. These effects will largely be confounded with any that arise because of the developmental transaction between the organism’s genetic liability and the exposure to depressogenic environments (active and evocative genotype-environment correlation). Such a finding is important for gene discovery, if such be possible, because it implies that the same genes may be expressed at different developmental stages, even if some of their effects increase with age. Or, discovery of genes that affect adult depression may also assist the discovery of genes that explain differences among juveniles.
In contrast, the impact of the environment is, at least in part, dynamic and cumulative whereas the genes affect inherent levels and rates of change. This model does not make explicit provision for genotype × environment interaction (G × E). However, in so far as G × E involves interaction with individual and time-specific environments, it is expected that the effects of G × E are confounded with those of the unique environment, E, in our model.
We note that the original 11 × 11 genetic covariance matrix and its Cholesky decomposition has 76 free parameters. Our best fitting model has only seven genetic parameters (four genetic variances and three correlations, Table 5) derived from a parsimonious developmental theory yet reproduces the covariance pattern very well, including that implied by the Cholesky decomposition that is characteristic of any longitudinal data set under several contrasting theories of the underlying developmental process. Our model thus captures the descriptive genetic findings of previous studies of repeated measures of juvenile depression, but offers the additional insight that the genetic covariance structure described in terms of the Cholesky decomposition in earlier studies may be attributed to random genetic effects on the overall liability to depression and genetic differences in the rates of developmental change.
Limitations
The data and model have significant limitations that are shared with many other studies. The principal weaknesses of the data are the small samples and the relatively coarse-grained longitudinal assessment. The former limitation reduces the power of statistical tests of heterogeneity, such as those due to sex effects on the contributions of genes and environment. The limited number of widely-separated assessments reduces the ability of the design to resolve subtleties of the developmental process beyond the relatively crude effects assumed in this model. We note that, in this study, any effects of G × E interaction will be confounded with those of the (unmeasured) unique environment and any effects of the environment correlated with genotype will be confounded with those of genotype (see Jinks and Fulker 1970). Thus, one plausible explanation of the increase of genetic effects during development is the accumulation of positively or negatively reinforcing environments correlated with those of variation in genetic liability to depression.
In conclusion, although our model reveals significant differences in the way genes and environment contribute to developmental change in adolescent depression it does not exhaust alternative theoretical possibilities such as the role of the developmental genetic timing of milestones in the expression of genetic differences (e.g. Eaves and Silberg 2003). Such models demand alternate conceptual models and analytical approaches.
References
Angold A, Costello EJ, Messer SC, Pickles A, Winder F, Silver D (1995) The development of a short questionnaire for use in epidemiological studies of depression in children and adolescents. Int J Methods Psychiatr Res 5:237–249
Bergen SE, Gardner CO, Kendler KS (2007) Age-related changes in heritability of behavioral phenotypes over adolescence and young adulthood: a meta-analysis. Twin Res Hum Genet 10:423–433
Boomsma DI, Molenaar PC (1987) The genetic analysis of repeated measures I. Simplex models. Behav Genet 17:111–123
Boomsma DI, Martin NG, Molenaar PC (1989) Factor and simplex models for repeated measures: application to two psychomotor measures of alcohol sensitivity in twins. Behav Genet 19:79–96
Bryk AS, Raudenbush SW (1987) Application of hierarchical linear models to assessing change. Psychol Bull 101:147–158
Cornes BK, Zhu G, Martin NG (2007) Sex differences in genetic variation in weight: a longitudinal study of body mass index in adolescent twins. Behav Genet 37:648–660
Costello AJ, Angold A (1988) Scales to assess child and adolescent depression: checklists, screens, and nets. J Am Acad Child Adolesc Psychiatry 27(6):726–737
Duncan TE, Duncan SC (1991) A latent growth curve approach to investigating developmental dynamics and correlates of change in children’s perceptions of physical competence. Res Q Exerc Sport 62:390–398
Duncan TE, Duncan SC, Hops H (1994) The effects of family cohesiveness and peer encouragement on the development of adolescent alcohol use: a cohort-sequential approach to the analysis of longitudinal data. J Stud Alcohol 55:588–599
Eaves LJ, Silberg JL (2003) Modulation of gene expression by genetic and environmental heterogeneity in timing of a developmental milestone. Behav Genet 33:1–6
Eaves LJ, Long J, Heath AC (1986) A theory of developmental change in quantitative phenotypes applied to cognitive development. Behav Genet 16:143–162
Eaves LJ, Silberg JL, Meyer JM, Maes HH, Simonoff E, Pickles AR, Rutter ML, Neale MC, Reynolds CA, Erickson MT, Heath AC, Loeber R, Truett KR, Hewitt JK (1997) Genetics and developmental psychopathology: 2. The main effects of genes and environment on behavioral problems in the Virginia Twin Study of Adolescent Behavioral Development. J Child Psychol Psychiatry 38:965–980
Eaves L, Erkanli A, Silberg J, Angold A, Maes HH, Foley D (2005) Application of Bayesian inference using Gibbs sampling to item-response theory modeling of multi-symptom genetic data. Behav Genet 35:765–780
Eley TC, Stevenson J (1999) Exploring the covariation between anxiety and depression symptoms: a genetic analysis of the effects of age and sex. J Child Psychol Psychiatry 40:1273–1282
Finkel D, Reynolds CA, Mcardle JJ, Pedersen NL (2007) Age changes in processing speed as a leading indicator of cognitive aging. Psychol Aging 22:558–568
Gillespie NA, Evans DE, Wright MM, Martin NG (2004a) Genetic simplex modeling of Eysenck’s dimensions of personality in a sample of young Australian twins. Twin Res 7:637–648
Gillespie NA, Kirk KM, Evans DM, Heath AC, Hickie IB, Martin NG (2004b) Do the genetic or environmental determinants of anxiety and depression change with age? A longitudinal study of Australian twins. Twin Res 7:39–53
Gillespie NA, Kendler KS, Prescott CA et al (2007) Longitudinal modeling of genetic and environmental influences on self-reported availability of psychoactive substances: alcohol, cigarettes, marijuana, cocaine and stimulants. Psychol Med 37:947–959
Hewitt JK, Silberg JL, Rutter ML, Simonoff E, Meyer JM, Maes HH, Pickles AR, Neale MC, Loeber R, Erickson MT, Kendler KS, Heath AC, Truett KR, Reynolds CA, Eaves LJ (1997) Genetics and developmental psychopathology:1. Phenotypic assessment in The Virginia Twin Study of Adolescent Behavioral Development. J Child Psychol Psychiatry 38:943–963
Hishinuma E, Chang J, McArdle JJ, Hamagami A (2012) Potential causal relationship between depressive symptoms and academic achievement in the Hawaiian High Schools Health Survey using contemporary longitudinal latent variable change models. Dev Psychol 48(5):1327–1342
Jinks JL, Fulker DW (1970) Comparison of the biometrical genetical, MAVA and classical approaches to the analysis of human behavior. Psychol Bull 73:511–549
Kendler KS, Gardner CO, Lichtenstein P (2008) A developmental twin study of symptoms of anxiety and depression: evidence for genetic innovation and attenuation. Psychol Med 38(11):1567–1575
Lange K, Westlake J, Spence MA (1976) Extensions to pedigree analysis III. Variance components by the scoring method. Ann Hum Genet 39:485–491
Lau JY, Eley TC (2006) Changes in genetic and environmental influences on depressive symptoms across adolescence and young adulthood. Br J Psychiatry 189:422–427
McArdle JJ (1986) Latent variable growth within behavior genetic models. Behav Genet 16(1):163–200
McArdle JJ (1988) Dynamic but structural equation modeling of repeated measures data. In: Cattell RB, Nesselroade J (eds) Handbook of multivariate experimental psychology, 2nd edn. Plenum Press, New York, pp 561–614
McArdle JJ (1994) Appropriate questions about causal inference from “Direction of Causation” analyses. Genet Epidemiol 11:477–482
McArdle JJ, Anderson ER (1989) Latent growth models for research on aging. In: Biren LE, Schaie KW (eds) The handbook of the psychology of aging, vol 3. Academic Press, San Diego, pp 21–44
McArdle JJ, Epstein D (1987) Latent growth curves within developmental structural equation models. Child Dev 58:110–133
McArdle JJ, Hamagami F (1991) Modeling incomplete longitudinal and cross-sectional data using latent growth structural models. In: Collins L, Horn JL (eds) Best methods for the analysis of change. APA Press, Washington, pp 276–304
Mcardle JJ, Hamagami F (1992) Modeling incomplete longitudinal and cross-sectional data using latent growth structural Models. Exp Aging Res 18:145–166
McArdle JJ, Hamagami F (2003) Structural equation models for evaluating dynamic concepts within longitudinal twin analyses. Behav Genet 33:137–159
Mcardle JJ, Hamagami F, Elias MF, Robbins MA (1991) Structural modeling of mixed longitudinal and cross-sectional data. Exp Aging Res 17:29–52
McArdle JJ, Hamagami F, Jones K, Jolesz F, Kikinis R, Spiro A 3rd, Albert MS (2004) Structural modeling of dynamic changes in memory and brain structure using longitudinal data from the normative aging study. J Gerontol B 59:294–304
Mehta PD, West SG (2000) Putting the individual back into individual growth curves. Psychol Methods 5(1):23–43
Meyer JM, Silberg JL, Simonoff E, Kendler KS, Hewitt JK (1996) The Virginia Twin-Family Study of Adolescent Behavioral Development: assessing sample biases in demographic correlates of psychopathology. Psychol Med 26:1119–1133
Miyazaki Y, Raudenbush SW (2000) A test for linkage of multiple cohorts from an accelerated longitudinal design. Psychol Methods 5(1):44–63
Neale MC, Cardon LR (1992) Methodology for genetic studies of twins and families, NATO ASI Series, 1st edn. Kluwer Academic Publishers, Dordrecht
Neale MC, Boker SM, Xie G, Maes HH (2004) MX: statistical modeling. Virginia Institute for Psychiatric and Behavioral Genetics, Richmond
Nesselroade JR, Baltes PB (1974) Adolescent personality development and historical change: 1970-1972. Monogr Soc Res Child Dev 39:1–80
Rice F, Harold GT, Thapar A (2002) Assessing the effects of age, sex and shared environment on the genetic aetiology of depression in childhood and adolescence. J Child Psychol Psychiatry 43:1039–1051
Silberg JL, Pickles A, Rutter M, Hewitt JK, Simonoff E, Maes H, Carbonneau R, Murrelle L, Foley DL, Eaves LJ (1999) The influence of genetic factors and life stress on depression in adolescent girls. Arch Gen Psychiatry 56:225–232
Silberg JL, Rutter M, Eaves L (2001) Genetic and environmental influences on the temporal association between earlier anxiety and later depression in girls. Biol Psychiatry 49:1040–1049
Simonoff E, Pickles AR, Meyer JM, Silberg JL, Maes HH, Loeber R, Rutter ML, Hewitt JK, Eaves LJ (1997) The Virginia Twin Study of Adolescent Behavioral Development: influences of age, gender and impairment on rates of disorder. Arch Gen Psychiatry 54:801–808
Thapar A, McGuffin P (1994) A twin study of depressive symptoms in childhood. Br J Psychiatry 165:259–265
Conflict of Interest
All authors declare that they have no conflict of interest.
Human and Animal Rights and Informed Consent
This article does not contain any studies with animals performed by any of the authors. Informed consent was obtained from all individual participants included in the study.
Author information
Authors and Affiliations
Corresponding author
Additional information
Edited by Matt McGue.
Rights and permissions
About this article
Cite this article
Gillespie, N.A., Eaves, L.J., Maes, H. et al. Testing Models for the Contributions of Genes and Environment to Developmental Change in Adolescent Depression. Behav Genet 45, 382–393 (2015). https://doi.org/10.1007/s10519-015-9715-9
Received:
Accepted:
Published:
Issue Date:
DOI: https://doi.org/10.1007/s10519-015-9715-9