Abstract
European Alpine landscapes are facing marked land-use changes. On the one hand, outdoor winter recreation is spreading, with ski infrastructure degrading fragile mountain habitats, and snowsports causing disturbance and stress to wildlife. On the other hand, the abandonment of traditional grazing practices on timberline grasslands is leading to their encroachment by shrubs and forest, which decreases habitat heterogeneity and negatively affects biodiversity. We used the black grouse, a declining key indicator species of the Alpine timberline ecosystem, to assess optimal breeding habitat characteristics, with the goal of providing guidelines for appropriate restoration. Using Mixed Effects Logistic Regression analyses, we compared habitat features both at visited and at pseudo-absence locations within individual home ranges in order to determine the optimal habitat for males and females. Horizontal and vertical structural heterogeneity within all vegetation layers was the best predictor of occurrence for both sexes. In contrast, vegetation composition affected the presence of females, but not that of males. Females preferred a diverse, complex mosaic consisting of isolated mature coniferous trees and scattered small regenerating trees, associated with shrub cover (Ericacea) and Alpine meadows (Nardion). Chick-rearing females furthermore avoided roads, forest tracks and walking paths. The optimal predicted proportions of habitat types obtained from the model provide guidelines for the restoration of timberline ecosystems through corrective forestry measures and/or adapted grazing practices. These measures are likely to also benefit other Alpine timberline biodiversity.
Similar content being viewed by others
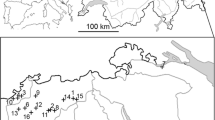
Avoid common mistakes on your manuscript.
Introduction
In contrast to many lowland ecosystems, negative anthropogenic impacts on Alpine ecosystems are relatively new, but they have significantly increased over the past decades (Körner 2000). The constantly growing winter and summer tourism industry has resulted in an expansion of infrastructure, especially ski-lift installations, and an increasing human penetration into vulnerable, formerly relatively untouched ecosystems outside ski resorts (Arlettaz et al. 2007; Delgado et al. 2007). In addition, the progressive abandonment of traditional farming practices, such as extensive cattle grazing and meadow mowing, also threatens Alpine biodiversity (Maurer et al. 2006).
In mountainous regions, the effects of land-use changes on native flora and fauna are complex, variable in space and time (Körner 2000; Tasser et al. 2007) and remain poorly understood. Negative anthropogenic impacts on Alpine wildlife population dynamics may operate through changes in habitat quality caused by e.g. modifications of vegetation, or direct human disturbance (Watson and Moss 2004; Ingold 2005; Arlettaz et al. 2007; Patthey et al. 2008). The maintenance or restoration of high-quality habitats in semi-natural and anthropogenic landscapes is a key issue in conservation biology, as habitat quality is one of the main determinants of reproductive output and survival (Pärt 2001; Vickery et al. 2001).
In farmland ecosystems, spatial and temporal habitat heterogeneity, which operates at different scales, has been shown to be essential for biodiversity (Benton et al. 2003). The same probably holds true for Alpine grasslands, which have for long been heavily grazed by cattle and sheep. Among Alpine ecosystems, timberline habitats, which are situated at the interface between subalpine coniferous forests, adjacent dwarf shrub formations and alpine meadows, are a naturally diverse ecotone that harbours a high diversity of plant and animal species (Camarero and Gutiérrez 2002). Many of these species are specialised and therefore restricted to this ecotone (Sippola et al. 2002), rendering them especially vulnerable to habitat modifications. Timberline ecosystems are also the favourite areas for the development of snow sports such as skiing or snowshoeing. Compared to sites without ski infrastructure, ski runs and their surroundings feature lower plant productivity and species richness, reduced abundance and cover of woody plants, as well as diminished arthropod abundance and bird species diversity (Wipf et al. 2005; Rolando et al. 2007). Moreover, any increase of human disturbance within recreation areas may decrease individual fitness and, consequently, wildlife abundance (Watson and Moss 2004; Patthey et al. 2008).
Grouse species are viewed as indicators of ecosystem integrity (Storch 2007). The black grouse Tetrao tetrix L., an emblematic representative of the timberline fauna, is likely to play the role of an indicator species, if not of an umbrella species, for timberline ecosystems. Black grouse populations are declining in most Central European countries, especially in peripheral areas situated at species’ distribution edge, including external Alpine massifs (Storch 2007). Habitat loss, degradation and/or fragmentation (Kurki et al. 2000; Storch 2007), climatic variation (Lindström et al. 1997; Zbinden and Salvioni 2004) and disturbance caused by human activities (Arlettaz et al. 2007; Patthey et al. 2008) are regarded as the main factors leading to population shrinkage. Reproductive success is the principal determinant of black grouse population dynamics, and is most likely to depend on weather variation (Zbinden and Salvioni 2004) and habitat quality (Ludwig et al. 2008). Using radio-tracking, we investigated fine-grained habitat selection of black grouse in the Alps. Given the indicator, and presumed umbrella, functions of black grouse, and the role of reproductive success in determining population dynamics, we assumed that the habitat preferences shown by breeding females, compared to non-breeding females and males, would provide the essential information needed to define adequate habitat management measures, which, in turn, would promote overall biodiversity.
Methods
Study area
This study was conducted in the upper subalpine forests and the adjacent timberline at seven different sites in SW Switzerland (canton of Valais, 46°12′ N; 7°20′ E) and at two sites in N Italy close to the Swiss border (Piemonte, Verbania Province, 46°06′ N; 8°18′ E) between 1,400 and 2,300 m above sea level. In most study sites, the dominant tree species are larch Larix decidua and arolla pine Pinus cembra, but Norway spruce Picea abies is more common in the West. Dwarf shrubs (e.g. alpenrose Rhododendron ferrugineum, bilberry Vaccinium myrtillus, northern bilberry Vaccinium uliginosum, dwarf juniper Juniperus communis nana, heather Calluna vulgaris, bearberry Arctostaphylos uva-ursi and crowberry Empetrum nigrum) and grasses (e.g. matgrass Nardus stricta, Calamagrostis villosa) represent a characteristic fieldlayer in all areas (Signorell et al. 2010). The study areas are characterised by a subcontinental to continental climate, with warm, dry summers and cold, relatively wet winters (Reisigl and Keller 1999).
Radio-locations and pseudo-absence points
Birds were captured from snow burrows between January and March in 2003–2006. We placed a 2 × 2.5-m mist-net stretched between 2- and 6-m long telescopic fishing rods on the snow surface above visually pre-located igloos and flushed the birds into the mesh while approaching closer to them (Marti 1985). Birds were also mist-netted at leks during April–June in the same years. They were tagged with neck-laced radio-transmitters with an activity sensor (12 and 15 g for females and males, respectively). In July and August, i.e. during the chick-rearing period, females were radio-located every day and males every second day for a period of 30 consecutive days. In every study area, radio-tracking, irrespective of sex or female breeding status, started on the day the first chicks hatched. Radio-locations were obtained by triangulation and homing-in onto the animal but without approaching closer than 10–15 m in order to avoid inadvertently flushing the grouse. We made sure that the birds did not move during our approach by listening to the signal emitted by the activity sensor. A pole, indicating the distance and direction to the expected bird position, was placed at each bearing position, and information noted. At the end of the breeding season, locations were retrieved from these poles and notes, and exact coordinates estimated using a global positioning system. These locations are henceforth referred to as visited points.
To analyse habitat selection, we compared habitat features between visited points (radio-locations) and pseudo-absence points. First, we delineated individual home ranges as the minimum convex polygon (MCP) encompassing all radio-locations for a given bird. A 15-m buffer was added around the MCP (extended MCP), to account for localisation error. We also added a 30-m buffer around each radio-location within the MCP, again to account for localisation error. As visited points are often clumped together in space, we delineated the 70% Kernel density surface. The coordinates of the pseudo-absence points were then randomly generated so as to lie inside the extended MCP, but outside the 70% Kernel and 30 m radius buffered areas. A total of 30 and 15 pseudo-absence points, equivalent to the number of radio-locations per sex, were randomized for females and males, respectively. We assumed that these pseudo-absence points were unsuitable or at least less suitable than visited points (Arlettaz 1999).
Predictors of occurrence
At the end of the radio-tracking season, predictors of occurrence were mapped at each point, within a 15-m radius circle, by the same observer (NS). This scale of measurement was a compromise between the accuracy of the radio-location (given the error) and the minimal adequate surface for a proper assessment of forest stand structure (500 m2, Bollmann et al. 2005). Occurrence predictors were grouped into six categories (vegetation structure, plant species cover, plant community cover, topography, human infrastructure and tourism and food abundance; Fig. 1, Online Resource 1).
Model selection design, with arrows indicating the main modelling steps. The main categories (vegetation structure, plant species and plant community covers) consist of five to six sub-categories each. Categories of predictors are indicated in bold. Variables are described in Online Resource 1
Vegetation structure
Horizontal and vertical vegetation heterogeneity (e.g. Signorell et al. 2010) was described through a simple visual ordinal estimate of structural heterogeneity with three levels (low, middle and high, see Online Resource 1). Predictors of vegetation heterogeneity were estimated for mature trees (>3 m height), small trees (<3 m), shrub, herbaceous, bare ground and rock habitat features. A virtual, 30-m long horizontal transect line centred on each point was set. Horizontal heterogeneity was considered to be high when corresponding to the maximum heterogeneity observable in our study area. Low and middle levels were assessed in the same way. The number of habitat features (low, 0–1 habitat features; middle, 2–4; high, >4) along the transect line were used to estimate vertical heterogeneity. Moreover, average habitat feature height (0–3, ascending), number of stumps and grazing activity (presence–absence) were also considered as structural predictors.
We hypothesized that the higher the vegetation heterogeneity the more likely would be the occurrence of black grouse, so horizontal and vertical heterogeneity were analysed as linear functions. The same held for stumps, since they are an indicator for richly structured habitats and high biodiversity (Humphrey et al. 2002). For vegetation height, however, we expected a quadratic relation with the occurrence of birds: if vegetation was low, it would increase predation risk, whereas if it were too tall, it could impede the mobility of the birds and the accessibility of ground-dwelling arthropods for young chicks (Signorell et al. 2010).
Plant species cover
The percentage cover of each plant species was estimated visually, within each vegetation layer to the nearest 1% for cover between 0% and 10% cover, and to the nearest 5% for cover greater than 10% (Online Resource 1). We included the linear and quadratic functions for all predictors, except for megaforbs (i.e. tall herb associations such as Adenostylion and Filipendulion), and rock cover, since they do not represent typical black grouse habitat features and are likely to be avoided (Klaus et al. 1990).
Plant community cover
Percentage cover of a given plant community was visually estimated as for plant species cover (Online Resource 1). Plant communities were classified according to Delarze et al. (1999), and included plants, as well as other ground cover information such as bare ground, rocks, screes, etc. The classification by Delarze et al. (1999) was applied to each vegetation layer. The linear and quadratic terms for all plant community predictors, except rocks and screes were included in the analysis.
Topography
Slope, southing (cosine of aspect) and easting (sinus of aspect) and convexity were used to describe the relief (Online Resource 1). Since these predictors affect the microclimatic conditions and influence the vegetation, they may play a role in habitat selection.
Human infrastructure and tourism
We selected two predictors for human infrastructure and tourism, based on the presence–absence or Euclidean distance to the nearest infrastructure (road, forest track or walking trail). These predictors integrated human disturbance as well as vegetation modifications (Online Resource 1). We hypothesized that sites near human facilities are avoided, whilst site quality increases with the distance from human facilities.
Food abundance
Ants and grasshoppers (Ponce and Magnani 1988; Ponce 1992a) are considered to be crucial food sources for grouse chicks during their first weeks of life. We used the number of anthills as an estimate of ant abundance. Grasshopper abundance can be assumed to be already approximated through the estimation of alpine meadow cover (Signorell et al. 2010).
Modelling and model selection process
To enable the simultaneous analysis of habitat selection in all birds pooled together, we used Mixed Effects Logistic Regressions, with study sites, and individuals nested within study site, being treated as random effects. The predictors of occurrence were modelled as fixed effects. To identify which habitat descriptor best explained the occurrence of birds, and to avoid drawbacks inherent to stepwise regression (Whittingham et al. 2006), we applied an information theoretic approach (Burnham and Anderson 1998; Johnson and Omland 2004). We first excluded those predictors (n total = 91) that occurred only marginally (e.g. E. nigrum, descriptor # 39, Online Resource 1) and the less biologically meaningful variables for each pair of correlated (r s ≥ |0.7|) variables. For some predictors, the quadratic term was used in addition to the linear term in order to model possible curvilinear preferences. Variables expressing structural heterogeneity (vegetation structure) and variables within the habitat categories plant species cover and plant community cover were logically grouped into sub-categories according to the specific vegetation layer in which they occurred (Online Resource 1). The overall modelling and model selection procedure is shown in Fig. 1.
Model selection within sub-categories
First, we looked at single and combined contributions of the various habitat predictors within the sub-categories of the three main categories, namely vegetation structure, species plant cover and plant community cover (up to six variables per sub-category, Online Resource 1). We then retained the best model for subsequent analyses.
Model selection within categories
Second, each of the variables from the best model for each of the categories (vegetation structure, plant species and plant community covers) were modelled singly, as well as in every possible combination of the variables retained within each sub-category. The model with the lowest Akaike’s Information Criterion corrected for small sample sizes (AIC C ) value was retained for further modelling. The variables within the categories topography, human infrastructure and tourism and food abundance were tested singly, as well as in combination. Again, the model with the lowest AIC C value within each of these three categories was retained for further modelling.
Combining plant species cover and plant community cover into vegetation composition
Third, we grouped the categories plant cover and plant community cover, as they both describe the cover structure of the mapping locations. This new group was called vegetation composition. The model selection process was the same as that mentioned above.
Best overall model
Finally, the set of explanatory variables of the single best model obtained for each category was used to build a new set of candidate models, in order to select the best overall model. Model performance was estimated by using the Kappa statistic (Vaughan and Ormerod 2005). Model performance was considered poor when Kappa was lower than 0.4, good when Kappa was between 0.4 and 0.75 and excellent when Kappa was above 0.75 (Fielding and Bell 1997).
Our approach allowed the number of predictors to be reduced and the most likely explanatory predictors to be selected at each stage (e.g. Johnson et al. 2005). This also avoided testing all possible combinations of predictors, which would have dramatically inflated the number of candidate models. Candidate models (each combination of habitat predictor categories) were ranked based on Akaike’s Information Criterion corrected for small sample sizes and Akaike weights (w i ) (Burnham and Anderson 1998; Johnson and Omland 2004). The selection probability of a given habitat category was calculated as the sum of the AIC C weights of those candidate models in which that category was included (Burnham and Anderson 1998). Finally, we used model averaging to calculate a general model and to estimate coefficients and 95% confidence intervals (CI) for each habitat predictor by averaging the models that had ΔAIC C < 2 compared to the best candidate model. Model performance was again estimated with the Kappa statistic (Vaughan and Ormerod 2005). All statistical analyses were performed using the software R 2.4.1 (lme4 and PresenceAbsence libraries, R Development Core Team 2006).
Results
Thirty black grouse, distributed across nine study sites, were radio-tracked during the chick-rearing period. Our sample was biassed towards males (n = 15) and unsuccessful breeding females (n = 11), compared to successful chick-rearing females (n = 4). Although 80% of the radio-tracked hens began incubation each year (2003–2006), only 28.6% of the incubating females successfully raised their chicks. Failures were due either to nest failure (62.5%) or chick mortality (37.5%) that occurred during the first 10 days of chicks’ life. All unsuccessful females stayed within the perimeter of the study site after losing their brood. Home range sizes were between 9.8 and 18.5 ha (mean ± SD, 13.5 ± 3.6) for successful breeding females, 9.8–48.8 ha (18.1 ± 13.0) for unsuccessful breeding females and 4.0–80.0 ha (18.0 ± 19.2) for males.
Model selection
Among the set of candidate models, vegetation structure presented the most likely ecological correlation for black grouse occurrence, showing a selection probability of nearly 100% for all three bird classes (Table 1). Vegetation composition and human infrastructure and tourism were also likely predictors of the occurrence of unsuccessful and successful chick-rearing females (selection probability [AIC C weights] > 77%), but not for males (<59%). The probability of linking topography with bird occurrence was high for males (83%) and low for females (<46%). Ant abundance, on the other hand, had a very low selection probability for all three bird classes (25% to 27%). The best candidate models (ΔAIC C < 2) used to estimate the averaged model for each bird class all had good to very good predictive power (Kappa, 0.59–0.83).
Averaged occurrence models
Averaged models showed that female and male black grouse selected the most heterogeneous locations, mainly described as having horizontal and vertical heterogeneity of the different vegetation layers (Table 2). The probability of the presence of breeding females was higher when mean grass height was low (1–10 cm), and decreased further with increasing grass height.
Variables describing vegetation composition were only retained in averaged models for successful and unsuccessful breeding females (Table 1). The averaged models for hens consisted of a complex assemblage of predictors. Although many predictors appeared in both models, there was no clear common link with bird occurrence for any of these fine-grained habitat predictors, except for the negative correlation with rocky habitats (Table 2, negative coefficients). To better understand the habitat composition correlating with bird occurrence, we used a reduced simple vegetation composition model that included shrubs, Alpine meadow and small and mature tree covers. We plotted predicted occurrence probability as a function of each vegetation cover by holding constant the other cover predictors at their averaged values (Fig. 2). The probability of the occurrence of brood-rearing hens was higher with a small cover of alpine meadow (10–50%) mixed with small tree cover (20–60%), mature tree cover (10–50%) and a cover of shrubs (>50%). Unsuccessful breeding hens selected the same broad habitat as chick-rearing hens, except that they showed a preference for an even higher alpine meadow cover (>50%).
Predicted probability of occurrence of successful (bold lines) and unsuccessful (thin lines) breeding black grouse females, during the chick-rearing season, in the Swiss and Italian Alps, with respect to various vegetation covers (alpine meadow, mature trees and small trees, shrub; 95% CI). Occurrence probability was allowed to vary with the species vegetation cover under consideration, while other vegetation covers were held constant at their mean values. Observed cover values are shown as small bars for successful (along lower horizontal axis) and unsuccessful (upper horizontal axis) breeding females
The probability of occurrence of successful and unsuccessful females was lower when tourism infrastructure (roads, forest tracks and walking trails) was present within a 30-m diameter circle (Table 2), and the occurrence of chick-rearing females was negatively correlated with proximity to roads (Table 2). Black grouse males have a tendency to select steeper sites (Table 2). Our ant abundance index was not correlated with any of our bird class occurrences (Table 2).
Discussion
The present study demonstrates that both male and female black grouse show a high preference for patchy, heterogeneous microhabitats, consisting of a field layer of mixed shrubland, interspersed with alpine meadow, within a matrix dominated by both isolated mature and young, regenerating coniferous trees. Habitat heterogeneity has been shown to be a key element for sustaining high biodiversity in ecosystems in general (Benton et al. 2003; Hamer et al. 2003; Tews et al. 2004), particularly in Alpine timberline ecosystems (Dufour et al. 2006). Here, we have quantitatively established that vegetation heterogeneity in timberline ecotones is essential for the occurrence of black grouse, an emblematic element of the Alpine fauna. This indirectly confirms the presumption that black grouse may play the role of an umbrella species within timberline ecosystems, similar to that of the capercaillie Tetrao urogallus in subalpine woodland (Suter et al. 2002). Promoting optimal conditions for their survival would therefore be beneficial to Alpine biodiversity in general.
In contrast to males, females showed a preference for a specific vegetation composition pattern. These different selection patterns may be linked to sex and/or breeding status. The pronounced sexual dimorphism (body mass of hens, 960 g; cocks, 1,311 g; Marti and Pauli 1985) combined with a completely different plumage coloration (males, black and white; females, cryptic brown) may be related to different eco-physiological adaptations (body size) and/or anti-predator behaviour (cryptic plumage) (Wolf and Walsberg 2000; Signorell et al. 2010). Although eco-physiological adaptations mediated through plumage structure and coloration may be essential in mountainous ecosystems subjected to marked microclimatic variation, only black grouse cocks might afford a conspicuous dark, sun-capturing plumage because they feed twice a day, mostly at dusk and dawn, always remaining close to vegetation cover. In contrast, brood-rearing hens face the challenge of providing sufficient protein-rich food for their chicks throughout the entire day. Their best source of food comes in the form of invertebrates, which are most abundant and accessible in open meadow, where the concealment from predators is lowest (Signorell et al. 2010). Diverging sex-specific selection pressures in terms of optimal habitat selection constraints may explain these plumage differences, in addition to sexual-selection mechanisms.
A comparison of vegetation composition preferences of successful and unsuccessful breeding hens suggests that these two classes differ little in their habitat selection pattern. However, successful chick-rearing females appear to have a much narrower niche than unsuccessful breeding females, the former being typically restricted to a more specific range of open and semi-open vegetation cover. This difference is best explained by the need to not only provide chicks with a good food supply, as mentioned above, but also to ensure adequate shelter, such as regenerating trees and dense shrubland, in order to decrease exposure to predators (Signorell et al. 2010). Broodless females feed mainly on plant material, and in the absence of chicks, they would thus have less specific requirements.
Our results suggest that hens avoided roads, forest tracks and walking paths. Roads may impair habitat quality directly, by degrading the vegetation, and indirectly through increased human disturbance. We do not know which of these factor(s) are linked to the occurrence of breeding females, but both factors could negatively affect reproductive success and, ultimately, black grouse density (Patthey et al. 2008).
We were unable to establish any association between the occurrence of chick-rearing females and the number of anthills. Either the index of ant abundance is too crude, or ants do not play such an important role for black grouse broods (Wegge and Kastdalen 2008). In effect, grasshoppers may be a more crucial resource for chicks in the Alps (Ponce 1992b; Signorell et al. 2010).
Recommendations for the management of Alpine timberline habitats
Guidelines for an optimal chick-rearing matrix can be derived from our model projections concerning occurrence probability in relation to the most important environmental predictors. These guidelines may be applied where habitat composition and food resources are the same as in our study area, i.e. in the Internal Alps.
Ericaceae shrubland (mainly R. ferrugineum and Vaccinium spp., >50% cover) and alpine meadow (mainly Nardion strictae and Festucion variae, 10–50% cover) must constitute the field vegetation layer, combined with scattered small (<3 m) regenerating coniferous trees (spruce, larch and arolla pine, density ca. 30 individuals per hectare, 10–50% cover). All these should be interspersed so as to form a spatially diversified mosaic. The upper layer should consist of isolated mature trees (ca. 10 trees per hectare, 10–50% cover). These elements should be available regularly along the timberline belt. As the latter is on average 300 m broad, depending on slope steepness, we suggest ensuring a repetition of the mosaic at least every 400 m along the timberline. Such an area (12 ha) roughly corresponds to the average home range for a successful chick-rearing hen (13.5 ha in this study) and may thus constitute a good minimal reference area for restoration management.
Vegetation heterogeneity, which plays such a central role for both females and males, must be promoted wherever possible. Such a varied mosaic is threatened in the European Alps due to shrub and forest encroachment after abandonment of traditional land-use by farmers (e.g. forest pasturing) and, more locally, too intensive grazing. The destruction of the field layer is also pronounced in tourist (especially ski) resorts. In the latter areas, retaining patches of shrubland and allowing natural seed rain from surrounding areas (Urbanska and Fattorini 2000) could be a first measure in preventing artificial homogenization of vegetation along ski slopes; re-vegetation could also be envisaged in areas damaged by heavy machinery. In timberline meadows, vegetation encroachment should be counteracted by traditional extensive farming practices, such as cattle grazing or moderate goat grazing (Storch 2007), but intensive pasturing resulting in monotonous open grassland should be discouraged. In localised areas where spontaneous reforestation is very advanced, some initial forestry work may even be necessary in order to establish favourable conditions for reinstating grazing (Muller et al. 1998; Tasser et al. 2007).
It was not possible to dissociate the effects of human infrastructure from the effects of human disturbance on the occurrence of breeding females. We nevertheless recommend, as a safety measure, the restriction of human access to sensitive chick-rearing areas, as well as the implementation of visitor-steering measures, such as the canalisation of tourist movement along designated paths, during the chick-rearing season.
The habitat model developed here may be used in the future as a basis for mapping habitat suitability across the Alps using GIS and satellite images. This may enable delineation of the areas where corrective management measures would provide the greatest return on investment, for instance where forest or shrub encroachment is more advanced. If appropriately applied, the above management recommendations are likely to benefit also other elements of biodiversity within Alpine timberline ecosystems.
References
Arlettaz R (1999) Habitat selection as a major resource partitioning mechanism between the two sympatric sibling bat species Myotis myotis and Myotis blythii. J Anim Ecol 68:460–471
Arlettaz R, Patthey P, Baltic M, Leu T, Schaub M, Palme R, Jenni-Eiermann S (2007) Spreading free-riding snow sports represent a novel serious threat for wildlife. Proc R Soc B Biol Sci 274:1219–1224
Benton TG, Vickery JA, Wilson JD (2003) Farmland biodiversity: is habitat heterogeneity the key? Trends Ecol Evol 18:182–188
Bollmann K, Weibel P, Graff RF (2005) An analysis of central Alpine Capercaillie spring habitat at the forest stand scale. For Ecol Manage 215:307–318
Burnham KP, Anderson DR (1998) Model selection and inference: a practical information-theoretic approach. Springer, New York
Camarero JJ, Gutiérrez E (2002) Plant species distribution across two contrasting treeline ecotones in the Spanish Pyrenees. Plant Ecol 162:247–257
Delarze R, Gonseth Y, Galland P (1999) Lebensräume der Schweiz. Ott Verlag (ed). Thun, pp 413
Delgado R, Sánchez-Marañón M, Martín-García JM, Aranda V, Serrano-Bernardo F, Rosúa JL (2007) Impact of ski pistes on soil properties: a case study from a mountainous area in the Mediterranean region. Soil Use Manage 23:269–277
Dufour A, Gadallah F, Wagner HH, Guisan A, Buttler A (2006) Plant species richness and environmental heterogeneity in a mountain landscape: effects of variability and spatial configuration. Ecography 29:573–584
Fielding AH, Bell JF (1997) A review of methods for the assessment of prediction errors in conservation presence/absence models. Environ Conserv 24:38–49
Hamer KC, Hill JK, Benedick S, Mustaffa N, Sherratt TN, Maryati M, Chey VK (2003) Ecology of butterflies in natural and selectively logged forests of northern Borneo: the importance of habitat heterogeneity. J Appl Ecol 40:150–162
Humphrey JW, Davey S, Peace AJ, Ferris R, Harding K (2002) Lichens and bryophyte communities of planted and semi-natural forests in Britain: the influence of site type, stand structure and deadwood. Biol Conserv 107:165–180
Ingold P (2005) Freizeitaktivitäten im Lebensraum der Alpentiere. Haupt, Bern
Johnson JB, Omland KS (2004) Model selection in ecology and evolution. Trends Ecol Evol 19:101–108
Johnson CJ, Boyce MS, Case RL, Cluff HD, Gau RJ, Gunn A, Mulders R (2005) Cumulative effects of human developments on arctic wildlife. Wildl Monogr 160:1–36
Klaus S, Bergmann HH, Marti C, Müller F, Vitovic OA, Wiesner J (1990) Die Birkhühner. A. Ziemsen, Lutherstadt Wittenberg
Körner C (2000) The alpine life zone under global change. Gayana Bot 57:1–17
Kurki S, Nikula A, Helle P, Lindén H (2000) Landscape fragmentation and forest composition effects on grouse breeding success in boreal forests. Ecology 81:1985–1997
Lindström J, Ranta E, Lindén M, Lindén H (1997) Reproductive output, population structure and cyclic dynamics in Capercaillie, Black Grouse and Hazel Grouse. J Avian Biol 28:1–8
Ludwig GX, Alatalo RV, Helle P, Nissinen K, Siitari H (2008) Large-scale drainage and breeding success in boreal forest grouse. J Appl Ecol 45:325–333
Marti C (1985) Differences in the winter ecology between cock and hen black grouse Tetrao tetrix in the Swiss Alps. Ornithol Beob 82:31–54
Marti C, Pauli HR (1985) Wintergewicht, Masse und Altersbestimmung in einer alpinen Population des Birkhuhns Tetrao tetrix. Ornithol Beob 82:231–241
Maurer K, Weyand A, Fischer M, Stöcklin J (2006) Old cultural traditions, in addition to land use and topography, are shaping plant diversity of grasslands in the Alps. Biol Conserv 130:438–446
Muller S, Dutoit T, Alard D, Grévilliot F (1998) Restoration and rehabilitation of species-rich grassland ecosystems in France: a review. Restor Ecol 6:94–101
Pärt T (2001) The effects of territory quality on age-dependent reproductive performance in the northern wheatear, Oenanthe oenanthe. Anim Behav 62:379–388
Patthey P, Wirthner S, Signorell N, Arlettaz R (2008) Impact of outdoor winter sports on the abundance of a key indicator species of alpine ecosystems. J Appl Ecol 45:1704–1711
Ponce F (1992a) Régime alimentaire du Tétras lyre Tetrao tetrix dans les Alpes françaises. Alauda 60:260–268
Ponce FMJ (1992b) Régime et sélection alimentaires des poussins de Tétras lyre (Tetrao tetrix) dans les Alpes françaises. Gibier Faune Sauvage 9:27–51
Ponce F, Magnani Y (1988) Régime alimentaire des poussins de Tétras lyre (Tetrao tetrix) sur deux zones des Alpes françaises: méthodologie et résultats préliminaires. In: Office National de la Chasse (ed) Colloque Galliformes de montagne. Paris, pp225–228
Reisigl H, Keller R (1999) Lebensraum Bergwald, 2nd edn. Spektrum Akademischer, Heidelberg
Rolando A, Caprio E, Rinaldi E, Ellena I (2007) The impact of high-altitude ski-runs on alpine grassland bird communities. J Appl Ecol 44:210–219
Signorell N, Wirthner S, Patthey P, Schranz R, Rotelli L, Arlettaz R (2010) Concealment from predators drives foraging habitat selection in brood-rearing Alpine black grouse Tetrao tetrix hens: habitat management implications. Wildl Biol 16:249–257
Sippola AL, Siitonen J, Punttila P (2002) Beetle diversity in timberline forests: a comparison between old-growth and regeneration areas in Finnish Lapland. Ann Zool Fenn 39:69–86
Storch I (2007) Grouse. Status survey and conservation action plan 2006–2010. IUCN, the world pheasant association. Gland, Switzerland
Suter W, Graf RF, Hess R (2002) Capercaillie (Tetrao urogallus) and avian biodiversity: testing the umbrella-species concept. Conserv Biol 16:778–788
Tasser E, Walde J, Tappeiner U, Teutsch A, Noggler W (2007) Land-use changes and natural reforestation in the Eastern Central Alps. Agric Ecosyst Environ 118:115–129
Tews J, Brose U, Grimm V, Tielbörger K, Wichmann MC, Schwager M, Jeltsch F (2004) Animal species diversity driven by habitat heterogeneity/diversity: the importance of keystone structures. J Biogeogr 31:79–92
Urbanska KM, Fattorini M (2000) Seed rain in high-altitude restoration plots in Switzerland. Restor Ecol 8:74–79
Vaughan IP, Ormerod SJ (2005) The continuing challenges of testing species distribution models. J Appl Ecol 42:720–730
Vickery JA, Tallowin JR, Feber RE, Asteraki EJ, Atkinson PW, Fuller RJ, Brown VK (2001) The management of lowland neutral grasslands in Britain: effects of agricultural practices on birds and their food resources. J Appl Ecol 38:647–664
Watson A, Moss R (2004) Impacts of ski-development on ptarmigan (Lagopus mutus) at Cairn Gorm, Scotland. Biol Conserv 116:267–275
Wegge P, Kastdalen L (2008) Habitat and diet of young grouse broods: resource partitioning between Capercaillie (Tetrao urogallus) and Black Grouse (Tetrao tetrix) in boreal forests. J Ornithol 149:237–244
Whittingham MJ, Stephens PA, Bradbury RB, Freckleton RP (2006) Why do we still use stepwise modelling in ecology and behaviour? J Anim Ecol 75:1182–1189
Wipf S, Rixen C, Fischer M, Schmid B, Stoeckli V (2005) Effects of ski piste preparation on alpine vegetation. J Appl Ecol 42:306–316
Wolf BO, Walsberg GE (2000) The role of the plumage in heat transfer processes of birds. Am Zool 40:575–584
Zbinden N, Salvioni M (2004) Bedeutung der Temperatur in der frühen Aufzuchtzeit für den Fortpflanzungserfolg des Birkhuhns Tetrao tetrix auf verschiedenen Höhenstufen im Tessin, Südschweiz. Ornithol Beob 101:307–318
Acknowledgements
We would like to thank S. Mettaz, S. Wirthner, G. Obrist, N. Reusser, F. Bancala, J. Beguin, R. Egli, U. Kormann, P. Nyffeler, P.-L. Obrist and C. Buchli for assistance in the field; G. Wittwer, J. Zbinden, M. Häusler, N. Zbinden and two anonymous reviewers who provided helpful suggestions on an earlier version of this manuscript; M. Schaub and F. Abadi Gebreselassie for providing advice on statistics. The Parco Naturale Veglia Devero, Italy, and the Naturschutzzentrum Aletsch provided free accommodation, and the ski resorts of Verbier and Les Diablerets provided free access to their facilities. A. and P. Buhayer corrected the language. This research was funded by a grant from the Swiss National Science Foundation to R.A. Additional funding was obtained from the Swiss Federal Office for the Environment, the Cantons of Valais, Vaud and Ticino, as well as a European Interreg IIIa grant.
Author information
Authors and Affiliations
Corresponding author
Additional information
Communicated by C. Gortázar
Patrick Patthey and Natalina Signorell contributed equally to the study.
Electronic supplementary material
Below is the link to the electronic supplementary material.
Online Resource 1
Explanatory variables (n = 91) mapped in the field, regrouped in main categories (in bold) and sub-categories (roman numerals), with format (continuous, ordinal, categorical), function (quadratic or linear, for both continuous and ordinal variables) and levels (categorical variables, see nomenclature in the respective column; ordinal variables, 0–3, or 0–4). Variables belonging to the same sub-category are marked with vertical bars. A variable followed by brackets indicates that this variable has been eliminated in favour of the variable number given in parentheses. See text for more details (DOC 145 kb)
Rights and permissions
About this article
Cite this article
Patthey, P., Signorell, N., Rotelli, L. et al. Vegetation structural and compositional heterogeneity as a key feature in Alpine black grouse microhabitat selection: conservation management implications. Eur J Wildl Res 58, 59–70 (2012). https://doi.org/10.1007/s10344-011-0540-z
Received:
Revised:
Accepted:
Published:
Issue Date:
DOI: https://doi.org/10.1007/s10344-011-0540-z