Abstract
We propose a cutting-plane approach (namely, Benders decomposition) for a class of capacitated multi-period facility location problems. The novelty of this approach lies on the use of a specialized interior-point method for solving the Benders subproblems. The primal block-angular structure of the resulting linear optimization problems is exploited by the interior-point method, allowing the (either exact or inexact) efficient solution of large instances. The consequences of different modeling conditions and problem specifications on the computational performance are also investigated both theoretically and empirically, providing a deeper understanding of the significant factors influencing the overall efficiency of the cutting-plane method. The methodology proposed allowed the solution of instances of up to 200 potential locations, one million customers and three periods, resulting in mixed integer linear optimization problems of up to 600 binary and 600 millions of continuous variables. Those problems were solved by the specialized approach in less than one hour and a half, outperforming other state-of-the-art methods, which exhausted the (144 GB of) available memory in the largest instances.
Similar content being viewed by others
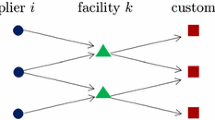
Avoid common mistakes on your manuscript.
1 Introduction
A dynamic facility location problem consists of defining a time-dependent plan for locating a set of facilities in order to serve customers in some area or region. A finite planning horizon is usually considered representing the time for which the decision maker wishes to plan. In a multi-period setting, the planning horizon is divided into several time periods each of which defining specific moments for making adjustments in the system. The most common goal is the minimization of the total cost—for the entire planning horizon—associated with the operation of the facilities and the satisfaction of the demand.
This class of problems extends their static counterparts and emerges as appropriate when changes in the underlying parameters (e.g., demands or transportation costs) can be predicted. The reader can refer to the book chapter [36] for further details as well as for references on this topic.
The study of multi-period facility location problems is far from new. Nevertheless, the relevance of these problems is still quite notable since they are often found at the core of more complex problems such as those arising in logistics (see, e.g., [3, 33]). Accordingly, their study is of major importance. In particular, having efficient approaches for tackling those problems may render an important contribution to the resolution of more comprehensive problems.
The purpose of this paper is to introduce an exact method for a class of multi-period discrete facility location problems. In particular, we consider a pure phase-in setting in which a plan is to be devised for progressively locating a set of capacitated facilities over time. This is the “natural” extension to a multi-period context of the classical capacitated facility location problem. In addition, we specify a maximum number of facilities that can be operating in each time period. This is a means to control the “speed” at which the system changes in case the decision maker finds this necessary. A set of customers whose demand is known for every period is to be supplied from the operating facilities in every period. Nevertheless, we assume that service level is not necessarily 100 %; instead, this will be endogenously defined and a cost is assumed for shortages at the customers. This cost may represent an opportunity loss or simply a penalty incurred due to the shortage. In addition to this cost, we consider operating costs for the facilities and transportation costs from the facilities to the customers. All costs are assumed to be time-dependent. The goal of the problem is to decide where and when to locate facilities in order to minimize the total cost over the planning horizon.
The above problem can be formulated as a mixed integer linear optimization problem with a set of binary variables (associated with the location decisions) and a set of continuous variables (associated with transportation for demand satisfaction and shortage at the customers). Such type of problems are well-known to be particularly suited for decomposition approaches based on cutting planes, namely Benders decomposition [29, 45]. In fact once the binary variables are fixed, the remaining problem is a linear optimization problem which can be dualized for deriving optimality cuts. We explore this structure in order to develop a very efficient Benders decomposition approach for the problem.
1.1 Relation with the existing literature
From a methodological point of view, our work consists of using, extending, and combining several methods in order to obtain an efficient exact solution procedure for the problem that we are investigating. In particular, we make use of a structure-exploiting interior-point method as a cut-generator for a Benders decomposition of the problem. In this section we discuss previous literature on the relevant techniques and their relation with the new methodology proposed.
We start by pointing out that the key idea of our specialized interior-point method differs substantially from that of other existing interior-point based solvers, such as the Object-Oriented Parallel Solver (OOPS) and the suite of parallel solvers PIPS.
The OOPS system, described in [19] and used in several applications (e.g., [13]), is based on partitioned Cholesky factorizations, while our specialized interior-point method eliminates the complicating linking constraints by combining both direct—Cholesky factorizations—and iterative solvers—conjugate gradients. As it will be discussed later, the particular advantage of the iterative solvers resulted to be instrumental for making the overall approach very effective when tackling instances of the problem we are analyzing.
PIPS is an alternative exploiting-structure system, specialized for stochastic optimization, that includes both linear and nonlinear interior-point [6, 39] and simplex solvers [27]. However, again, the interior-point methods of PIPS are significantly different from our approach. Although the block-angular structure of the stochastic optimization problems dealt with by PIPS is similar to ours, PIPS relies on high performance computers that exploit parallel processing, and makes use of state-of-the-art Cholesky solvers. Our approach runs (so far) in single thread mode, it requires much less computing resources, and it is efficient enough if a standard Cholesky solver is considered (and thus, there is room for improvement). From an algorithmic point of view, the most significant difference between [39] and our approach is that we solve the normal equations form of the KKT interior-point conditions, while PIPS considers the augmented system form. This allows us to solve the resulting linear systems by a combination of Cholesky for block constraints and a preconditioned conjugate gradient for linking constraints—using the preconditioner detailed in [7, 10]—whereas in [39] the whole system (including all constraints) is solved by an iterative solver, requiring an expensive factorization to obtain the preconditioner. The approach of [6] also uses an iterative solver, but the preconditioner is tailored to stochastic optimization problems, which is not our case. Compared to PIPS-S [27], our approach can solve our linear optimization subproblems (those obtained after fixing the binary variables) with hundreds of millions of variables using a few Gigabytes of RAM, while the highly efficient and parallel simplex implementation of PIPS-S required about 1000 GB of RAM for stochastic optimization problems of similar sizes, which calls for the use of supercomputers.
A second ingredient of our approach will be the use of suboptimal feasible solutions in the Benders subproblems, obtaining \(\varepsilon \)-subgradients and thus \(\varepsilon \)-cuts. This idea was first used in [20] for the solution of block-angular problems using the general solver HOPDM [18]. The main two differences of that approach when compared to ours are: (i) the problems in [20] were linear, while facility location includes binaries; (ii) the cutting plane was applied in [20] for the solution of the block-angular problem (thus the “master problem” was linear), whereas we use cutting planes for the binary-continuous division (thus the “master problem” is binary), and the block-angular structure is exploited in the subproblems using the specialized interior-point solver. The use of inexact or \(\varepsilon \)-cuts in Benders decomposition was analyzed in [47] for linear problems, confirming its good convergence properties. Its use in integer problems has been recently studied and validated in [30, 44].
Recent improvements have been also achieved in the solution of large facility location problems with quadratic costs [16, 17]. The approach proposed in [17] is also based on an efficient and ad-hoc cut-generator (i.e., subproblem solution), which relies on KKT conditions. However it deals with uncapacitated problems, while we focus on capacitated and multi-period instances which require of an efficient simplex or interior-point method as a cut generator. On the other hand the approach presented in [16] solves instances of a quadratic capacitated facility location problem using a perspective reformulation which, eventually, means solving a quadratically constrained problem with a general purpose interior-point solver. We note that the specialized interior-point solver used in our work could be extended to deal with the type of quadratically constrained problems investigated in [16]—though the extension is nontrivial, and it would mean a significant coding effort. Therefore, we think that combining the subproblem formulation of [16] with an extended version of the specialized interior-point solver we are using in our work, would allow solving extremely large facility location instances with quadratic costs.
It is also worth noting that interior-point methods have already been used in the past for the solution of integer optimization problem using cutting-plane approaches, such as in [34] for linear ordering problems. More recently, primal-dual interior-point methods have shown to be very efficient in the stabilization of column-generation procedures for the solution of problems such as vehicle routing with time windows, cutting stock, and capacitated lot sizing with setup times [21, 35].
One important ingredient for the development of our new methodology has to do with the fact that the Benders subproblems we will be dealing with can be separated into block-angular structured linear programming problems. This same structure has triggered the development of several well-known optimization techniques. Among those, methods based upon Dantzig–Wolfe decomposition, namely column generation approaches ([12, 26]) are possibly the most popular ones. As pointed out in Ref. [43], such approaches can be looked at as a dual method based upon the Lagrangian relaxation of the linking constraints. Alternatively, such Lagrangian relaxation can be tackled directly as a non-smooth concave problem. Subgradient methods ([22, 40]) are one possibility that is quite popular. Another type of methods that have emerged as an alternative to subgradient optimization for non-smooth concave problems are the so-called bundle methods ([25]). Robinson [42] developed a bundle method for tackling block-angular structured convex problems. After dualizing the linking constraints, the resulting non-smooth concave problem can be solved using a bundle-based decomposition method. In Ref. [31] this possibility is studied more deeply and it is applied for tackling large scale block-angular structured linear programming problems. Recently, [38] considered so-called inexact bundle methods to two-stage stochastic programs.
Finally, we refer to the Volume algorithm introduced by [4] as a means for extending the subgradient algorithm so that it also produces primal solutions. Those authors have tested the new approach in linear optimization problems with a special structure including a block-angular one. Recently, in Ref. [15], we observe a successful application of the Volume algorithm in the context of large-scale two-stage stochastic mixed-integer 0–1 problems, namely when it comes to solving the Lagrangian dual resulting from dualizing the non-anticipativity constraints in the splitting variable formulation of the general problem.
1.2 The relevance of the contribution provided by the current work
The novelty in the Benders decomposition we propose has to do with the resolution of the Benders subproblem, for which the specialized interior-point method for primal block-angular structures of [7, 8, 10] will be customized. In short, this is a primal-dual path-following method [46], whose efficiency relies on the sensible combination of Cholesky factorization and preconditioned conjugate gradient for the solution of the linear system of equations to be solved at each interior-point iteration.
This paper amplifies significantly the range of applicability of interior-point methods within the context of combinatorial optimization. This is accomplished by optimally combining existing techniques that result in a new approach yielding remarkable computational results. The methodological novelty can be detailed as follows:
-
Benders subproblems are tackled using a specialized interior-point method, which allows to fully take advantage of some unique factorization properties of the facility location problem matrix structure. This has two main benefits:
-
It becomes possible to efficiently solve very large linear subproblems (that cannot be tackled by state-of-the-art optimization solvers such as IBM CPLEX).
-
Since Benders decomposition does not require an optimal solution to the subproblem, a primal-dual feasible solution (i.e., a point of the primal-dual space which is feasible for both the primal and dual pair of the subproblem) is enough for generating an additional cut. The interior-point method is thus an excellent choice, since it can quickly obtain such a primal-dual feasible point in the earlier iterations, skipping the last ones which focus on reducing the complementarity gap. In particular, avoiding the last interior-point iterations is instrumental for the specialized algorithm considered in this work, since the performance of the embedded preconditioned conjugate gradient solver degrades close to the optimal solution.
-
-
The multi-period capacitated facility location problem that we are investigating is very general—it captures in a single modeling framework several particular cases which are at the core of many real-world logistics network design problems. Accordingly, more than a specific problem, we are in fact investigating a broad class of combinatorial optimization problems.
-
Both from a theoretical and an empirical point of view, we show that the competitive advantage of the proposed approach increases when the number of facilities and customers grows large.
Overall, the new procedure represents a relevant breakthrough in terms of the resolution to optimality of multi-period capacitated facility location problems. In fact, it has been able to solve problems of up to 200 potential locations, one million customers and three periods, resulting in mixed integer linear problems of up to 600 binary and 600 millions of continuous variables. To the best of the authors’ knowledge, the solution of facility location instances of such sizes has never been reported in the literature.
The remainder of this paper is organized as follows. In Sect. 2 the problem is described in detail and formulated. The cutting plane method is presented in Sect. 3, introducing the new approach for solving the subproblems. Computational tests are reported in Sect. 4. The paper ends with an overview of the work done and some conclusions that can be drawn from it.
2 Problem description and formulation
We consider a set of potential locations where facilities can be set operating during a planning horizon divided into several time periods. Additionally, there is a set of customers whose demand in each period is known and that are to be supplied from the operating facilities. Facilities are capacitated and once installed they should remain open until the end of the planning horizon. We specify the maximum number of facilities that can be operating in each time period. Finally, demands are not required to be fully satisfied; instead, we consider a service level not necessarily equal to 100 %; its value is an outcome of the decision making process. We consider costs associated with: (i) the operation of the facilities, (ii) the satisfaction of the demand and (iii) the shortages at the customers. The goal is to decide where facilities should be set operating and how to supply the customers in each time period from the operating facilities in order to minimize the cost for the entire planning horizon.
Before presenting an optimization model for this problem we introduce some notation that will be used hereafter.
Sets:
- \({T}\) :
-
Set of time periods in the planning horizon with \(k=|{T}|\).
- \({I}\) :
-
Set of candidate locations for the facilities with \(n=|{I}|\).
- \({J}\) :
-
Set of customers with \(m=|{J}|\).
Costs:
- \(f_i^t\) :
-
Cost for operating a facility at \(i \in {I}\) in period \(t \in {T}\).
- \(c_{ij}^t\) :
-
Unitary transportation cost from facility \(i \in {I}\) to customer \(j \in {J}\) in period \(t \in {T}\).
- \(h_j^t\) :
-
Unitary shortage cost at customer \(j \in {J}\) in period \(t \in {T}\).
Other parameters:
- \(d_j^t\) :
-
Demand of customer \(j \in {J}\) in period \(t \in {T}\).
- \(q_i\) :
-
Capacity of a facility operating at \(i \in {I}\).
- \(p^t\) :
-
Maximum number of facilities that can be operating in period \(t \in {T}\).
The decisions to be made can be represented by the following sets of decision variables:
\(y_i^t = {\left\{ \begin{array}{ll} 1 &{} \hbox {if a facility is operating at } i \in {I} \hbox { in period } t \in {T}, \\ 0 &{} \hbox {otherwise.} \end{array}\right. }\)
\(x_{ij}^t =\) Amount shipped from facility \(i \in {I}\) to customer \(j \in {J}\) in period \(t \in {T}\).
\(z_j^t =\) Shortage at customer \(j \in {J}\) in period \(t \in {T}\).
The multi-period facility location problem we are working with can be formulated as follows:
In the above model, the objective function (1) represents the total cost throughout the planning horizon, which includes the cost for operating the facilities, the transportation costs from facilities to customers and the costs for shortages at the customers. Constraints (2) ensure that the demand of each customer in each period is divided into two parts: the amount supplied from the operating facilities and the shortage. Inequalities (3) are the capacity constraints for the operating facilities. Constraints (4) define the maximum number of facilities that can be operating in each period. Relations (5) ensure that we are working under a pure phase-in setting, i.e., once installed, a facility should remain open until the end of the planning horizon. Finally, constraints (6)–(8) define the domain of the decision variables.
The above model has several features which are worth emphasizing.
-
(i)
By considering constraints (5) we are capturing a feature of major relevance in many logistics network design problems which has to do with the need for progressively install a system since it is often the case that such systems cannot be setup in a single step (the reader can refer to [32] for a deeper discussion on this aspect).
-
(ii)
Since the facilities are capacitated, the possibility of adjusting the set of operating facilities over time is a way for adjusting the overall capacity of the system, which, in turn, can be looked at as a response to changes in demands and costs. Some authors have explicitly considered capacity adjustments as part of the decision making process (e.g., [23, 24]) within a multi-period modeling framework for facility location problems.
-
(iii)
By specifying the values of \(p^t\), \(t \in T\), we are setting a maximum “speed” for making adjustments in the system in terms of the operating facilities. When such a feature is not relevant, one can simply set \(p^t=n\), \(t \in T\) and the model is still valid. Since we are working with a pure phase-in problem we assume that \(1 \le p^1 \le p^2 \le \cdots \le p^k \le n\).
-
(iv)
In our problem, the service level is not necessarily 100 %; instead, it will be endogenously determined, resulting from a trade-off between the different costs involved. The practical relevance of considering a service level below 100 % in the context of facility location has been discussed by several authors, such as [1, 2, 37]. Since we are working with a multi-period problem, the expression “service level” is rather vague. In fact, we can, for instance, consider a service level per time period or even a global service level for the entire planning horizon:
$$\begin{aligned} \hbox {SL}(t) = \frac{\sum _{j \in J} \sum _{i \in I} x_{ij}^t}{\sum _{j \in J} d_j^t}, \quad \hbox {GSL} = \frac{\sum _{t \in T} \sum _{j \in J} \sum _{i \in I}x_{ij}^t}{\sum _{t \in T} \sum _{j \in J} d_j^t}. \end{aligned}$$In the first case, in order to obtain a “global” service level, we may simply average the service level attained in the different periods yielding
$$\begin{aligned} \hbox {ASL}= \frac{1}{k}\sum _{t \in T} \hbox {SL}(t). \end{aligned}$$ -
(v)
The above model is still valid if some facilities are already operating before the planning horizon and the goal is to expand a system already operating. In such a case we can use the same model if we fix to 1 the location variables associated with the existing facilities.
-
(vi)
In order to present a model that is as general as possible, we are assuming all parameters to be time-dependent. However, in practice this is not always the case. For instance, when the transportation costs are a function of the distance between the facilities and customers we may not observe a significant change from one period to the following and thus we may assume them to be time-invariant.
-
(vii)
Parameters \(f_i^t\) may convey more than the operating costs of the facilities in the different periods. In fact, if we have, say, a fixed cost, \(o_i^t\), for opening a facility at i in period t and we wish to include the corresponding term, \(o_i^t(y_i^t - y_i^{t-1})\), in the objective function, it is easy to conclude that re-arranging the terms associated to the location variables we obtain again each variable \(y_i^t\) multiplied by a “modified” operating cost (the reader can refer to [36] for additional insights).
Considering the problem with \(k=1\) (one period), \(p_1=n\) and shortage costs arbitrarily large (thus ensuring that all z-variables are equal to 0), we obtain the well-known capacitated facility location problem which generalizes the uncapacitated facility location problem that is known to be NP-hard (see, e.g., [14]). Accordingly, the problem we are investigating is also NP-hard. Nevertheless, developing efficient exact approaches that can solve instances with a realistic size is always a possibility worth exploring. This is what we propose next.
3 The cutting-plane approach
The problem described in the previous section is a good candidate for the application of a Benders decomposition approach [5, 28, 41, 45]. In fact, once a decision is made for the binary y-variables, the remaining problem is a linear optimization problem. Therefore, the problem can be projected onto the y-variables space yielding
where \(y=(y_i^t\), \(i \in I\), \(t \in T\)), and Q(y) is defined as
Q(y) is a convex piecewise linear function, so the overall problem can be solved by some nondifferentiable cutting-plane approach. Benders decomposition can be seen as a particular implementation of such an approach, where Q(y) is approximated from below by cutting planes. These planes are obtained by evaluating Q(y) at some particular y values, i.e., solving the (Benders) subproblem induced by those values. The new cuts replace Q(y) and are sequentially added to (9)–(12) leading to an updated (Benders) master problem. Benders master and subproblem provide, respectively, lower and upper bounds to the optimal solution. Such a cutting-plane algorithm is iterated until the gap between the lower and upper bound is either zero or small enough.
Fixing the location variables \(y_i^t\) (\(i \in I\), \(t \in T\)), the linear optimization problem Q(y) is separable in terms of the time periods. A resulting family of k independent linear optimization problems is obtained, which for a particular period \(t=1,\dots ,k\) can be written as:
Therefore, the Benders subproblem can be written as \(Q(y)= \sum _{t \in T} \hbox {SubLP}(y,t)\). Its optimal solution provides the information about the goodness of the designed location decisions. That solution provides an upper bound to the original multi-period problem (1)–(8). It is worth noting that, in theory, a primal-dual feasible suboptimal solution to (18)–(22)—that is, an inexact solution to the subproblem, or an inexact Benders cut—is enough for the Benders decomposition algorithm, though the upper bound obtained may be higher, thus of worse quality. Inexact cuts have been studied and proven to guarantee convergence of the Benders method, for instance, in [47] for linear problems. In the case of mixed integer linear problems, to the best of the authors’ knowledge, the few references existing in the literature exploring the use of inexact cuts are very recent, namely [30] and [44].
Denoting by \(\lambda _j^t\) (\(j \in J\)) and \(\mu _i^t\) (\(i \in I\)) the dual variables associated with constraints (19) and (20), respectively, we can write the dual of \(\hbox {SubLP}(y,t)\) as follows:
Benders decomposition makes use of a cutting-plane method to transfer the information about the goodness of the location decisions specified by the y-variables from the subproblem to the master problem. Suppose that Q(y) was evaluated at a set of points \(y^v, v\in V\). Denote by \(\lambda _j^{t,v}\) (\(j \in J\)) and \(\mu _i^{t,v}\) (\(i \in I\)) the corresponding solution for problem \(\hbox {DualSubLP}\left( y^v,t\right) \). The Benders master problem can be written as follows:
The optimal objective function value of this problem provides a lower bound to the original problem (1)–(8).
In the above model, we present the aggregated cuts (28). In fact, such cuts can be disaggregated by considering one for each time period,
and considering the objective function
In this work we considered the aggregated cuts (28), since some preliminary computational experiments showed that this reduces significantly the size of the master problem, yet producing high quality cuts.
For the particular case of the capacitated multi-period facility location problem we are studying in this paper, the structure of the subproblem allows obtaining a deeper insight into the quality of Benders cuts. In order to see this, consider an \(\xi -\)parameterized version of the problem with \(m = k = 1\) (one period and one customer), where the demand and the capacities are specified as \(d = \xi \) and \(q_i = (1-\xi )\), for \(i \in I\). The corresponding subproblem can be written as follows (we simplify some notation previously introduced since \(m=k=1\)):
Denoting by \(\lambda \), \(\mu _i\) (\(i \in I\)), \(\nu _i\) (\(i \in I\)), and \(\gamma \) the dual variables associated with constraints (33), (34), (35), and (36), respectively, the dual of (32)–(36) can be written as follows:
Proposition 1
In a Benders iteration, let \(I_{A}\) be the subset of \(I\) associated to the active constraints \(x_{i} = (1-\xi ) y_{i}\) of \(\hbox {SubLP}^{\prime }(\xi )\). The corresponding Benders cut is
Proof
The dual feasibility of \(\hbox {SubLP}^{\prime }(\xi )\) implies \(\lambda + \mu _i + \nu _i = c_i\), for \(i \in I\), and \(\lambda + \gamma = h\). Note that, \(\mu _i = c_i + \gamma -h\), for all \(i \in I_{A}\), and \(\mu _i = 0\), for all \(i \in I{\setminus } I_{A}\). (In the special case when \(y_i = 0\), either \(\nu _i\) or \(\mu _i\) can be arbitrarily fixed, and this relation still holds.) Based on (28), we have:

\(\square \)
Proposition 1 suggests two important elements which might substantially effect the goodness of a Benders cut: (i) the relationship between demand and total capacity, captured by \(\xi \), (ii) the shortage cost h. When h is small enough, \(z > 0\) and \(\gamma = 0\), so that \(\theta \ge \xi h - (1 - \xi )\sum _{i \in I_{A}} \left( h - c_i \right) y_{i}\). In particular, when \(h < c_i\), for all \(i = 1 \ldots n\), the Benders cut is \(\theta \ge \xi h\), since \(|I_{A}| = 0\). Similarly, when \(\xi \) approaches either zero (the total capacity widely exceeds the demand) or one (the demand overcomes the total capacity), the two limit cases reduce to \(\theta \ge - \sum _{i \in I_{A}} \left( h - c_i - \gamma \right) y_{i}\) and \(\theta \ge (h - \gamma )\) respectively. It turns out that the information transmitted by the Benders cut reduces when the demand grows large with respect to the total capacity, as reflected by the smaller size of the term \((1-\xi )\sum _{i \in I_{A}} \left( h - c_i - \gamma \right) y_{i}\). Nonetheless, when the demand is too small \(|I_{A}| = 0\) and \((1-\xi )\sum _{i \in I_{A}} \left( h - c_i - \gamma \right) y_{i} = 0\). Thus, both cases give rise to conditions where the decisions of the subproblem poorly affect the decision to be made in the master problem.
3.1 Solving the subproblem by a specialized interior-point method
As we have already shown, the Benders subproblem can be decomposed into k independent linear optimization problems (18)–(22). For each \(t \in T\), the corresponding problem can be written as the following linear problem with primal block-angular constraints:
where matrix \(L = [\mathbb {I}~|~ \mathbf {0}] \in \mathbb {R}^{n \times (n+1)}\) is made up by an identity matrix with a zero column vector on the right; for each \(j \in J\), \(\mathbf {c}_j^t=[c_{1j}^t,\dots ,c_{nj}^t, h_{j}^{t}]^\top \in \mathbb {R}^{n+1}\) and \(\mathbf {x}_j^t=[x_{1j}^t,\dots ,x_{nj}^t, z_j^t]^\top \in \mathbb {R}^{n+1}\) represent, respectively, the shipping and shortage costs involving customer j and the amount of commodity shipped to and shortage of customer j; \(e \in \mathbb {R}^{n+1}\) is a vector of ones; \(\mathbf {x}_{0}^t \in \mathbb {R}^{n}\) are the slacks of the linking constraints; \(\mathbf {q}^{t} = [q_1 y_{1}^{t}, \ldots , q_n y_{n}^{t}]^\top \in \mathbb {R}^{n}\) is the right-hand side vector for the linking constraints which contains the supply capacities of the designed locations. Note that the block constraints \(e^\top \mathbf {x}_j^t = d_j^t\), \(j\in J\), correspond to (19), whereas the linking constraints \(\sum _{j\in J} L \mathbf {x}_j^t + \mathbf {x}_0^t = \mathbf {q}^t\) refer to (20).
Formulation (44)–(46) exhibits a primal block-angular structure, and thus it can be solved by the interior-point method of [7, 10]. This method is a specialized primal-dual path-following algorithm tailored for primal block-angular problems. A thorough description of primal-dual path-following algorithms can be found in [46]. Shortly, these type of methods follow the central path until they reach the optimal solution. The central path is derived as follows. Formulation (44)–(46) can be written in standard form as
where \(c,x \in \mathbb {R}^{(n+1)m+n}\) contain, respectively, all the cost and decision variables vectors \(\mathbf {c}_j^t\), \(\mathbf {x}_j^t\), and \(A\in \mathbb {R}^{(m+n) \times [(n+1)m+n]}\) and \(b\in \mathbb {R}^{m+n}\) are, respectively, the constraints matrix and right-hand-side vector of (44)–(46). Denoting by \(\lambda \) and s the Lagrange multipliers of the equalities and inequalities, and considering a parameter \(\mu >0\), the perturbed Karush–Kuhn–Tucker optimality conditions of (47)–(49) are
where e is a vector of ones, and X and S are diagonal matrices whose (diagonal) entries are those of x and s. The set of unique solutions of (50)–(52) for each \(\mu \) is known as the central path, and these solutions converge to those of (47)–(49) when \(\mu \rightarrow 0\) (see [46]).
Each iteration of a primal-dual path-following method computes a Newton direction for (50)–(52). This requires the solution of the normal equations system \(A\varTheta A^\top \varDelta {\lambda } = g\), where \(\varTheta = XS^{-1}\) is diagonal and directly computed from the values of the primal and dual variables at each interior-point iteration; \(\varDelta {\lambda }\in \mathbb {R}^{m+n}\) is the direction of movement for the Lagrange multipliers \(\lambda \); and \(g\in \mathbb {R}^{m+n}\) is an appropriate right-hand side. Solving the normal equations is the most expensive computational step of the interior-point method. General interior-point solvers usually compute them by a Cholesky factorization, while the specialized method considered in this work combines Cholesky with preconditioned conjugate gradient (PCG). Exploiting the structure of A in (45), and appropriately partitioning \(\varTheta \) and \(\varDelta {\lambda }\) according to the \(m+1\) blocks of variables and constraints, we have
where \({{\mathrm{Tr}}}(.)\) denotes the trace of a matrix, \(\varphi _j= [\varTheta _{j_{11}},\dots ,\varTheta _{j_{nn}}]^\top \), for \(j \in J\), and
is diagonal.
By eliminating \(\varDelta {\lambda _1}\) from the first group of equations, the system (53) reduces to
Systems \(Bu=v\) with matrix B (for some u and v) in (55)–(56) are directly solved as
The only computational effort is thus the solution of system (55)—the Schur complement of (53)—, whose dimension is n, the number of candidate locations.
System (55) is computationally expensive if solved by Cholesky factorization, because (i) it requires computing the matrix \(D-C^\top B^{-1}C\), and (ii) this matrix can become very dense, as shown in [7]. As suggested in [7]—for multicommodity flow problems—and in [8]—for general block-angular problems, this system can be solved by PCG. A good preconditioner is instrumental for the performance of the conjugate gradient. As shown in [7, Prop. 4], the inverse of \(D-C^\top B^{-1}C\) for this kind of block-angular problems can be computed as
The preconditioner, which will be denoted as \(M^{-1}\), is an approximation of \((D-C^\top B^{-1} C)^{-1}\) obtained by truncating the infinite power series (57) at some term \(\phi \). As shown in [9], in many applications the best results are obtained for \(\phi =0\), i.e. the preconditioner is just \(M^{-1}= D^{-1}\). This value, \(\phi =0\), has been successfully used for all the computational results of the paper. In such a case, the solution of (55) by the conjugate gradient only requires matrix-vector products with matrix \((D-C^\top B^{-1} C)\)—computationally cheap because of the structure of D, C and B—and the solution of systems with matrix D—which are straightforward since D is diagonal.
It has been shown in [10] that the quality of the preconditioner depends on the spectral radius (i.e., the maximum absolute eigenvalue) of matrix \(D^{-1}(C^\top B^{-1} C)\), denoted as \(\rho \), which is real and always in [0, 1). The farther from 1, the better is the preconditioner. In practice it is observed that \(\rho \) comes closer to 1 as we approach the optimal solution, degrading the performance of the conjugate gradient. Therefore, since there is no need to optimally solve the Benders subproblem, the interior-point algorithm can be prematurely stopped for some not-too-small \(\mu >0\). The suboptimal primal-dual point will guarantee the primal and dual feasibility conditions (50) and (51), and its optimality gap can be controlled through \(\mu \). This way we can avoid the most expensive conjugate gradient iterations, providing at the same time a good primal-dual feasible point to generate a new cut for the master problem. We note that this cannot be (efficiently) achieved using the simplex algorithm for the Benders subproblem, since in that case the points are either primal feasible (primal simplex) or dual feasible (dual simplex), and primal-dual feasibility is not reached until the optimal solution has been found.
An alternative to the Newton direction is to compute Mehrotra’s predictor–corrector direction (see, for instance, [46, Ch.10] for the details), which in practice significantly reduces the number of interior-point iterations. However, this means to compute two systems with the matrix of the normal equations, for two different right-hand-sides. This is not a main drawback when normal equations are solved by Cholesky, since the factorization—the most expensive part of the solution of the system—is reused for the two backward–forward substitutions. Predictor–corrector directions (even higher-order directions) are the default in state-of-the art interior-point solvers (such as CPLEX). On the other hand, computing the predictor–corrector direction with the specialized interior-point means solving two different systems with PCG, which can drastically increase the solution time. In other applications it was observed [7, 10] that the predictor–corrector direction was not competitive compared to the Newton direction using the specialized interior-point method. However, as it will be seen in Sect. 4.2, in the context of the multi-period facility location problem that we are investigating in this paper, the predictor–corrector direction provided the best results for the largest and most difficult instances. This is explained by the good behaviour of PCG in this particular application.
As stated above, the dimension of the Schur complement system (55) is n, the number of candidate locations. Therefore, we can expect a high performance of this approach when the number of potential facilities is small, even if the number of customers is very large. This assertion is supported by the empirical evidence provided in the next section, where problems of a few hundreds of locations and up to one million of customers are efficiently solved. We should emphasize that this “few locations and many customers” situation is the most usual in practice.
In addition, from a theoretical point of view, the method is also very efficient when the number of candidate locations becomes large. In this case, as stated by the next proposition, in the limit, the diagonal preconditioner \(M^{-1}= D^{-1}\) provides the inverse of the matrix in the Schur complement system (55). We will assume the interior-point (x, s) of the current iteration is not too close to the optimal solution, such that it can be uniformly bounded away from 0 (in fact, at every iteration the current point is known to be greater than 0 [46]).
Proposition 2
Let us assume that there is a \(0< \epsilon \in \mathbb {R}\) such that the current interior-point (x, s) satisfies \(x>\epsilon \) and \(s>\epsilon \). Then, when \(n\rightarrow \infty \) (the number of candidate locations grows larger) we have \(D -C^\top B^{-1}C \rightarrow D\).
Proof
This reduces to showing that matrix \(C^\top B^{-1}C \rightarrow 0\) when \(n\rightarrow \infty \). From the definition of C and B in (53) and since B is diagonal, we have that entry (h, l) of \(C^\top B^{-1}C\) is
Since \(\varTheta _j= X_jS_j^{-1}\) and \(x_j>\varepsilon >0\) and \(s_j>\varepsilon >0\), we get
\(\square \)
A major consequence of this proposition is that for large n, the number of PCG iterations required for the solution of (55) is very small using \(M^{-1}= D^{-1}\) as preconditioner. However, this was also empirically observed when the parameter that grows larger is the number of customers, m, as shown in the next section.
For the computational tests of next section we used the solver BlockIP, which is an efficient C++ implementation of the above specialized interior-point method, including many additional features [9] (among them, the computation of both Newton and Mehrotra’s predictor–corrector directions). Unlike most state-of-the-art solvers, BlockIP does not offer preprocessing capabilites. Because of that, we only considered in (45) the linking constraints of open facilities at period t, since the shipments \(x_{ij}^t\) from the non-open ones are 0. The size of the systems to be solved by PCG is thus the number of open facilities instead of n, which simplifies the solution of the Schur complement system. However, in order to appropriately build the Benders cut we still need the Lagrange multipliers \(\mu _i^t\) of the constraints (20) that are associated to non-open facilities (i.e., those with \(y_i^t= 0\)). Since these Lagrange multipliers have to satisfy constraints (24) and (26) of the dual subproblem, they are computed according to
4 Computational tests
In this section we describe a series of computational experiments designed to empirically validate the efficiency of the proposed cutting-plane approach for capacitated multi-period facility location using the specialized interior-point method for block angular problems. All the runs were carried out on a Fujitsu Primergy RX300 server with 3.33 GHz Intel Xeon X5680 CPUs (24 cores) and 144 GB of RAM, under a GNU/Linux operating system (Suse 11.4), without exploitation of multithreading capabilities, i.e., a single core was used—runs were carried out sequentially. CPLEX branch-and-cut (release 12.4) was used for solving the Benders master problems; Benders subproblems were solved with both the barrier algorithm of CPLEX and BlockIP. The CPLEX barrier—which will be denoted as “BarOpt”—was used since it resulted more efficient than simplex variants for these large subproblems. For running the CPLEX barrier we considered one thread, and no crossover (otherwise the CPU time would significantly increase). For BlockIP again a single thread was used. Both for the interior-point method and for the overall cutting plane approach the gap was computed according to \((UB-LB)/UB\), where UB and LB denote respectively an upper and lower bound for the optimal value either of the subproblem or of the overall multi-period facility location problem. For the very large-scale instances the optimality tolerance in the subproblems was the same for CPLEX and for BlockIP: either \(10^{-3}\) or \(10^{-2}\) depending on the particular type of instances.
4.1 The effect of parameter specification
Consider a capacitated multi-period facility location problem of the form (1)–(8) and the demands, capacities and costs reported in Table 1. Geometrically, this parameter specification can be looked at as resulting from a setting where facilities and customers are distributed along two (possibly piecewise) lines with a random perturbation \(\zeta \sim uniform(\ell ,1)\) in a two-dimensional plane. This is not far from real-world, where population is mostly concentrated around coastlines. Parameter \(\vartheta \in [0,1]\) is responsible for the angle between the two lines: customers and facilities are collinear or orthogonal when \(\vartheta = 0\) or \(\vartheta = 1\) respectively. Instances with \(\vartheta = 0\) will be referred to as one-dimensional or 1D instances; for \(\vartheta > 0\) the instances will be called two-dimensional or 2D instances. The tuning parameter \(\eta \) controls the extent to which the distance measures are heterogeneous. Hence, the transportation costs reflect some distance measure between the facilities and the customers, whereas the cost for operating a facility increases along a line. Discount factors \(0 < \delta _c \le 1\) and \(0 < \delta _f \le 1\) are included to compute the present value of transportation and building costs respectively—thus discounting future costs back to the present values. Concerning customer demands, a similar increasing pattern along a line is considered, so that more expensive locations are geometrically closer to customers with higher demand. The capacity of a location grows linearly with respect to its cost and is time-invariant. The tuning parameters \(\alpha \) and \(\beta \) presented in Table 1 allow (un)balancing the relation between the total demand and the total capacity in the system. Parameter \(\alpha \) is used for defining the capacities, while \(\beta \) controls the maximum number of facilities that can be open in each period.
The first computational tests performed involved 150 instances of problem (1)–(8) divided into six groups of 25 instances. These instances were generated according to the parameter specification of Table 1. For these instances we considered \(\vartheta = 0\) (1D instances with collinear customers and facilities), \(\ell = 1\) (no random perturbation), and \(\eta = 20\). Each group of 25 instances is associated with a specific combination of m, n and k. The 25 instances in each group correspond to different combinations of \(\alpha \) and \(\beta \)—which have been chosen to take the values \(0.1, \, 0,3, \, 0.5, \, 0.7\) and 0.9, resulting in 25 possible combinations. The first two groups instantiate the static problem (a single period in the planning horizon—\(k = 1\)); the third and fourth groups correspond to instances with a 3-period planning horizon (\(k = 3\)); the fifth and sixth groups correspond to instances with a 6-period planning horizon (\(k = 6\)). Next, we summarize the results obtained. The detailed results are presented in “Appendix”. All the instances have been solved by the cutting-plane algorithm that we are proposing in this paper using both CPLEX BarOpt and BlockIP.
Figure 1 depicts the CPU time (seconds)—averaged over 25 instances—for each of the six groups. We computed the arithmetic average since each group of 25 instances is associated with the same number of potential locations, customers and time periods. Accordingly, the order of magnitude of the results within groups does not call for the use of other average or aggregation measure. The vertical axis shows the CPU time (seconds), whereas the horizontal axis presents the five different values of \(\alpha \) (for the left plots) and \(\beta \) (for the right plots). The straightforward interpretation of these results is that, for almost all values of m, n, k, \(\alpha \) and \(\beta \), BlockIP significantly outperformed BarOpt when solving the Benders subproblems. Another interesting and relevant fact is the non-linear effect of \(\alpha \), which is consistent with what we claimed when discussing the implications of Proposition 1: extreme values of \(\alpha \) are associated to a poor effect of the second stage decision and transportation costs (subproblem solution) upon the goodness of the first stage decisions (master problem solution).
Comparisons of the CPU times of Benders-with-BarOpt (dashed-black) and Benders-with-BlockIP (continuous-grey) for different values of \(\alpha \) and \(\beta \), corresponding to the parameter specification of Table 1. Each plot averages values for each \(\beta \) when \(\alpha \) varies, and for each \(\alpha \) when \(\beta \) varies. a \(m = n = 500\), \(k = 1\). b \(m = n = 500\), \(k = 1\). c \(m = n = 1000\), \(k = 1\). d \(m = n = 1000\), \(k = 1\). e \(m = n = 500\), \(k = 3\). f \(m = n = 500\), \(k = 3\). g \(m = n = 1000\), \(k = 3\). h \(m = n = 1000\), \(k = 3\). i \(m = n = 500\), \(k = 6\). j \(m = n = 500\), \(k = 6\). k \(m = n = 1000\), \(k = 6\). l \(m = n = 1000\), \(k = 6\)
The aggregated results for the six groups of instances (averaged over 25 single problems) are presented in Table 2. In addition to the values of n, m and k, the table reports the number of constraints (“const.”), binary variables (“bin. var”) and continuous variables (“cont. var”) of the resulting optimization problems. Columns “BarOpt” and “BlockIP” report the average CPU time (seconds) and, within parentheses, the average number of Benders iterations. The column “Branch-and-cut” reports the average CPU time (seconds) and the average number of simplex iterations required by the CPLEX branch-and-cut solver for the solution of the monolithic formulation (1)–(8). It should be noted that the larger the instances, the more efficient the cutting-plane method—with either BarOpt or BlockIP—compared to branch-and-cut. BlockIP seems to be approximately two times faster than BarOpt for all the instances sizes.
Since the CPU times were obtained for different combinations of \(\alpha \), \(\beta \), n, m and k, a full factorial experiment was performed allowing the estimation of the effect of each parameter on the CPU time as well as on the number of Benders iterations. A linear regression was applied to the collection of 150 numeric observations reported in “Appendix”. The two response variables are given by the CPU time and either the number of Benders iterations—for Table 3—or the number of simplex iterations—for Table 4. Based on the non-linear effect of \(\alpha \), observed in Fig. 1, the regression model includes the linear effect \(|\alpha - 0.5|\) (which is related to the excess of demand or excess of capacities), rather than \(\alpha \).
From Table 3 we conclude that the length of the planning horizon is the main feature responsible for the number of Benders iterations (0.44965), but its effect is comparatively reduced when the CPU time is taken into account. This is consistent with the fact that the size of the subproblems per each time period is exclusively determined by the number of potential locations and customers and this is the reason why the effect of m and n plays the strongest role (0.39638). Another interesting insight that can be deduced from the regression analysis performed is the fact that the excess of demand or capacities (captured by parameter \(|\alpha - 0.5|\)) gives rise to two different outcomes in the computational performance of the Benders decomposition and the branch-and-cut algorithm. In fact, reinterpreting Proposition 1, high values of \(|\alpha - 0.5|\) should result in a poor dependency between the second stage and first stage decisions. Clearly, the same reasoning does not apply to the branch-and-cut algorithm, whose generation of valid inequalities follow a completely different logic.
4.2 Solution of very large-scale instances
In addition to the instances analyzed in the previous section, we generated a collection of very large-scale instances to test the efficiency of the proposed approach. These additional instances were obtained by considering all the combinations for \(n \in \{100,200\}\), \(m \in \{100000, 500000, 1000000\}\) and \(k \in \{1,2,3\}\). The parameters \(\alpha \) and \(\beta \) were set to 0.9999 and to 1, respectively, for all the instances, in order to avoid problems with large shortages resulting from lack of capacity. Due to the large number of customers, in these instances, transportation costs were divided by a scaling factor to reduce their “weight” in the objective function. A time limit of 7200 s was used in those executions, although it was never reached.
The dimensions of these instances are inspired by real-world location problems that may be faced, for instance, by internet-based retailer multinational companies. Such problems call for a few dozens or hundreds of locations spread around the world for the warehousing activities, and hundreds of thousands of “customers” related, for instance, to cities over some threshold population. To the best of the authors’ knowledge, the resolution of facility location problems with such dimensions have never been reported in the literature.
The first set of experiments corresponds to large-scale 1D instances (\(\vartheta = 0\)) with no random perturbation (\(\ell = 1\)). We ran those instances with the cutting-plane approach using an optimality tolerance \(10^{-3}\) for the interior-point solver in the subproblems. As we pointed out in the previous sections, inexact solutions to subproblems save the last and thus often the most “expensive” interior-point iterations with BlockIP since the performance of PCG degrades near the optimal solution. For a fair comparison with the off-the-shelf solver in use, these same optimality tolerances were set for CPLEX BarOpt, although its performance should not be significantly affected by this tolerance since it does not rely on PCG. The master problems were also suboptimally solved with CPLEX branch-and-cut by using a positive optimality tolerance to avoid too expensive solutions (from the perspective of the CPU time required); this tolerance was reduced in each Benders iteration multiplying it by a factor in the interval (0, 1) (in particular, we used 0.95). A positive small optimality gap was also used for the global Benders decomposition; Benders iterations stop when the relative difference between the best lower and upper bounds falls below this tolerance.
Table 5 reports the results obtained. The information contained in the columns headed by “m”, “n”, “k”, “const.”, “bin. var” and “cont. var” is the same as in Table 2. Columns “iter.”, “gap” and “CPU” contain the number of interior-point iterations, the achieved Benders optimality gap, and the CPU time (seconds), respectively, for both BlockIP and BarOpt. In column “rel. diff” we report the relative difference in terms of the best solutions (i.e., best Benders upper bounds) obtained by BlockIP and BarOpt. A negative value indicates that the upper bound obtained when using BlockIP was smaller (and thus better) than that obtained when using BarOpt. Although these values are omitted in the table, it is worth noting that, as it was mentioned in Sect. 3.1, BlockIP required in average only two PCG iterations for the solution of system (55) in the largest instances. (Analogous results for primal block-angular problems with the form (44)–(46) have been also observed in the field of complex network problems [11].) Finally, the last column in Table 5 (headed by “open”) contains the number of facilities operating in each period.
The results presented in Table 5 require some extra explanation: although subproblems were solved with an optimality tolerance of \(10^{-3}\), the Benders cuts generated in those instances were good enough to obtain a solution with a sufficiently small optimality gap. In fact, if Benders cuts were not accurate enough, the current solution could not be properly separated, and the master problem would have reported the same binary solution in two consecutive iterations (that is, the inexact solution of the Benders subproblem would be providing just a valid inequality for the master problem, not really a cut). In such a case, the Benders subproblem would generate the same new constraint for the master and the algorithm would iterate forever. We also see that, in general, optimality gaps were smaller—thus better—with BlockIP than with CPLEX, though both solvers used the same subproblem tolerance.
Regarding efficiency, from Table 5 we see that Benders with BlockIP outperformed Benders with CPLEX BarOpt in all the instances. Concerning the memory requirements we conclude that BlockIP was far superior to CPLEX BarOpt. Benders with BlockIP was able to provide a good solution to the largest instances, while CPLEX with BarOpt ran out of memory. Remarkably, Benders with BlockIP was able to solve the largest cases, namely those with a number of opened facilities equal to 181 in period 3. This means that the dimension of the subproblems solved by BlockIP was up to 181 million of continuous variables. CPLEX exhausted the 144 GB of RAM of the computer in the largest instances (executions marked with\(^{\dag }\)), while BlockIP just required a small fraction of the available memory.
The second set of experiments performed corresponds to large-scale 2D instances obtained with \(\vartheta = 0.5\), keeping unchanged all the previously defined parameterizations. We tested instances with a deterministic distribution of customers (\(\ell = 1\)) as well as instances with a random distribution (\(\ell = 0.5\)). The corresponding results are reported in Tables 6 and 7 respectively, where the competitive advantage of combining Benders decompositions with the specialized interior-point method appears once again. The number of open facilities in each period remains almost the same as in the case of 1D instances, due to the unchanged demand requirements and location capacities.
From Tables 6 and 7 we see that 2D instances were in general more difficult than 1D ones, requiring more CPU time. This is clearly seen in Table 7 which reports results for 2D instances with a random distribution of customers along the line. In fact, the random parameter considered (\(\ell = 0.5\)) means that customers may be located very far from the line. Those instances could not be solved with BlockIP using the standard Newton direction, and we were forced to use Mehrotra’s predictor–corrector direction (see the discussion in Sect. 3.1) with a loose optimality tolerance of \(10^{-2}\) for the subproblems; tighter optimality gaps reported long execution times. However, even in those unfavorable circumstances, Benders using BlockIP was able to compute solutions with small enough gaps for these big instances. Looking into these results we can also conclude that random instances listed in Table 7 are more difficult than the deterministic ones in Table 6 due to the average number of PCG iterations required at each interior-point iteration: two are required for the deterministic instances (as for the 1D instances reported in Table 5) while 4–6 are needed for those of Table 7. As it was observed for 1D instances, CPLEX could not solve the largest ones since the 144 GB of RAM available were exhausted.
5 Conclusions
In this work we exploited the use of a specialized interior-point method for solving the Benders subproblems associated with the decomposition of large-scale capacitated multi-period discrete facility location problems. This was accomplished by taking advantage from the primal block-angular structures of the underlying constraints matrices. The computational tests performed and reported in the paper show that this led to a substantial decrease in the computational effort for the overall Benders procedure. The effect of different modeling conditions on the computational performance was also investigated, which provided a deeper understanding of the significant factors influencing the overall efficiency.
The extensive computational results reported in Sect. 4 show that in all the instances tested, a Benders decomposition approach embedding BlockIP clearly outperformed other approaches, such as branch-and-cut or Benders using a generic interior-point method, even when the latter makes use of the full strength of an off-the-shelf solver such as IBM CPLEX. Furthermore, the specialized interior-point method was able to solve the Benders subproblems of the largest instances, namely, those in which the number of open facilities in the last period is 181 and thus with subproblems involving up to 181 million of continuous variables.
The research presented in this paper opens new possibilities for solving exactly large instances of more comprehensive multi-period facility location problems, A straightforward extension that can be considered is the combined phase-in/phase-out problem in which, in addition to the features considered in this paper, it is assumed that some facilities are already operating before the beginning of the planning horizon, which can be closed in any period. Another challenging area in which the developments proposed in this work may have a strong impact concerns stochastic single- and multi-period discrete facility location problems.
Our results show that the new methodology proposed in this paper works extremely well for problems with a structure such as the one we are considering. It is worth noting that the approach is relevant even if the Benders decomposition does not converge in a few iterations. In fact, even if some spatial distributions called for a larger number of Benders iterations, the benefits of using this specialized interior-point solver are still valid: (i) it may be early stopped with a suboptimal but feasible primal-dual point; (ii) it allows computing valid cuts even for huge subproblems, providing a solution with a given duality gap.
References
Albareda-Sambola, M., Fernández, E., Hinojosa, Y., Puerto, J.: The multi-period incremental service facility location problem. Comput. Oper. Res. 36, 1356–1375 (2009)
Albareda-Sambola, M., Alonso-Ayuso, A., Escudero, L., Fernández, E., Hinojosa, Y., Pizarro-Romero, C.: A computational comparison of several formulations for the multi-period incremental service facility location problem. TOP 18, 62–80 (2010)
Alumur, S., Kara, B.Y., Melo, T.: Location and logistics. In: Laporte, G., Nickel, S., Saldanha-da-Gama, F. (eds.) Location Science. Springer, Berlin (2015)
Barahona, F., Anbil, R.: The volume algorithm: producing primal solutions with a subgradient method. Math. Program. 87, 385–399 (2000)
Benders, J.F.: Partitioning procedures for solving mixed-variables programming problems. Comput. Manag. Sci. 2, 3–19 (2005). (English translation of the original paper appeared in. Numerische Mathematik 4(238–252), 1962)
Cao, Y., Laird, C.D., Zavala, V.M.: Clustering-based preconditioning for stochastic programs. Comput. Optim. Appl. 64, 379–406 (2016)
Castro, J.: A specialized interior-point algorithm for multicommodity network flows. SIAM J. Optim. 10, 852–877 (2000)
Castro, J.: An interior-point approach for primal block-angular problems. Comput. Optim. Appl. 36, 195–219 (2007)
Castro, J.: Interior-point solver for convex separable block-angular problems. Optim. Methods Softw. 31, 88–109 (2016)
Castro, J., Cuesta, J.: Quadratic regularizations in an interior-point method for primal block-angular problems. Math. Program. A 130, 415–445 (2011)
Castro, J., Nasini, S.: Mathematical programming approaches for classes of random network problems. Eur. J. Oper. Res. 245, 402–414 (2015)
Chvátal, V.: Linear Programming. W.H. Freeman and Company, New York (1983)
Colombo, M., Gondzio, J., Grothey, A.: A warm-start approach for large-scale stochastic linear programs. Math. Program. 127, 371–397 (2011)
Cornuéjols, G.P., Nemhauser, G.L., Wolsey, L.A.: The uncapacitated facility location problem. In: Mirchandani, P.B., Francis, R.L. (eds.) Discrete Location Theory. Wiley-Interscience, New York (1990)
Escudero, L.F., Garín, M.A., Pérez, G., Unzueta, A.: Lagrangian decomposition for large-scale two-stage stochastic mixed 0–1 problems. TOP 20, 347–374 (2012)
Fischetti, M., Ljubić, I., Sinnl, M.: Benders decomposition without separability: a computational study for capacitated facility location problems. Eur. J. Oper. Res. 253, 557–569 (2016)
Fischetti, M., Ljubić, I., Sinnl, M.: Redesigning Benders decomposition for large scale facility location. Manag. Sci. doi:10.1287/mnsc.2016.2461 (2016)
Gondzio, J.: Multiple centrality corrections in a primal-dual method for linear programming. Comput. Optim. Appl. 6, 137–156 (1996)
Gondzio, J., Sarkissian, R.: Parallel interior-point solver for structured linear programs. Math. Program. 96, 561–584 (2003)
Gondzio, J., Vial, J.-P.: Warm start and \(\epsilon \)-subgradients in the cutting plane scheme for block-angular linear programs. Comput. Optim. Appl. 14, 17–36 (1999)
Gondzio, J., González-Brevis, P., Munari, P.: New developments in the primal-dual column generation technique. Eur. J. Oper. Res. 224, 41–51 (2013)
Held, M., Wolfe, P., Crowder, H.: Validation of subgradient optimization. Math. Program. 6, 62–88 (1974)
Jena, S., Cordeau, J.-F., Gendron, B.: Modeling and solving a logging camp location problem. Ann. Oper. Res. 232, 151–177 (2015)
Jena, S., Cordeau, J.-F., Gendron, B.: Dynamic facility location with generalized modular capacity. Transp. Sci. 49, 484–499 (2015)
Lemaréchal, C., Strodiot, J.J., Bihain, A.: On a bundle algorithm for nonsmooth optimization. In: Mangasarian, O.L., Meyer, R.R., Robinson, S.M. (eds.) Nonlinear Programming 4, pp. 245–282. Academic Press, New York (1981)
Lübbecke, M.E.: Column generation. In: Cochran, J.J., Cox, L.A., Keskinocak, P., Kharoufeh, J.P., Smith, J.C. (eds.) Wiley Encyclopedia of Operations Research and Management Science, Wiley Online Library, Chichester (2011)
Lubin, M., Hall, J.A., Petra, C.G., Anitescu, M.: Parallel distributed-memory simplex for large-scale stochastic LP problems. Comput. Optim. Appl. 55, 571–596 (2013)
Magnanti, T.L., Wong, R.T.: Accelerating Benders decomposition: algorithmic enhancement and model selection criteria. Oper. Res. 29, 464–484 (1981)
Magnanti, T.L., Wong, R.T.: Decomposition methods for facility location problems. In: Mirchandani, P.B., Francis, R.L. (eds.) Discrete Location Theory. Wiley, New York (1990)
Malick, J., de Oliveira, W., Zaourar, S.: Nonsmooth optimization using uncontrolled inexact information. In: Technical Report, INRIA Grenoble (2013). http://www.optimization-online.org/DBHTML/2013/05/3892.html
Medhi, D.: Bundle-based decomposition for large-scale convex optimization: error estimate and application to block-angular linear programs. Math. Program. 66, 79–101 (1994)
Melo, M.T., Nickel, S., Saldanha-da-Gama, F.: Dynamic multi-commodity capacitated facility location: a mathematical modeling framework for strategic supply chain planning. Comput. Oper. Res. 33, 181–208 (2006)
Melo, M.T., Nickel, S., Saldanha-da-Gama, F.: Facility location and supply chain management—a review. Eur. J. Oper. Res. 196, 401–412 (2009)
Mitchell, J.E., Borchers, B.: Solving real-world linear ordering problems using a primal-dual interior point cutting plane method. Ann. Oper. Res. 62, 253–276 (1996)
Munari, P., Gondzio, J.: Using the primal-dual interior point algorithm within the branch-price-and-cut method. Comput. Oper. Res. 40, 2026–2036 (2013)
Nickel, S., Saldanha-da-Gama, F.: Multi-period facility location. In: Laporte, G., Nickel, S., Saldanha-da-Gama, F. (eds.) Location Science. Springer, Berlin (2015)
Nickel, S., Saldanha-da-Gama, F., Ziegler, H.-P.: A multi-stage stochastic supply network design problem with financial decisions and risk management. Omega 40, 511–524 (2012)
Oliveira, W., Sagastizábal, C., Scheimberg, S.: Inexact bundle methods for two-stage stochastic programming. SIAM J. Optim. 21, 517–544 (2011)
Petra, C.G., Schenk, O., Lubin, M., Gaertner, K.: An augmented incomplete factorization approach for computing the Schur complement in stochastic optimization. SIAM J. Sci. Comput. 36, C139–C162 (2014)
Polyak, B.T.: Subgradient method: a survey of Soviet research. In: Lemaréchal, C., Mifflin, R. (eds.) Nonsmooth Optimization, pp. 5–28. Pergamon Press, Oxford (1978)
Rei, W., Cordeau, J.-F., Gendreau, M., Soriano, P.: Accelerating Benders decomposition by local branching. INFORMS J. Comput. 21, 333–345 (2009)
Robinson, S.M.: Bundle-based decomposition: description and preliminary results. In: Prékopa, A., Szelezsfin, J., Strazicky, B. (eds.) System Modelling and Optimization, Lecture Notes in Control and Information Sciences, vol. 84. Springer, Berlin (1986)
Ruszczyński, A.: An augmented Lagrangian decomposition method for block diagonal linear programming problems. Oper. Res. Lett. 8, 287–294 (1989)
van Ackooij, W., Frangioni, A., de Oliveira, W.: Inexact stabilized Benders’ decomposition approaches with application to chance-constrained problems with finite support. Comput. Optim. Appl. doi:10.1007/s10589-016-9851-z (2016)
Wentges, P.: Accelerating Benders decomposition for the capacitated facility location problem. Math. Methods Oper. Res. 44, 267–290 (1996)
Wright, S.J.: Primal-Dual Interior-Point Methods. SIAM, Philapelphia (1996)
Zakeri, G., Philpott, A.B., Ryan, D.M.: Inexact cuts in Benders decomposition. SIAM J. Optim. 10, 643–657 (2000)
Acknowledgments
The first author has been supported by MINECO/FEDER Grants MTM2012-31440 and MTM2015-65362-R of the Spanish Ministry of Economy and Competitiveness; the second author has been supported by the European Research Council-ref. ERC-2011-StG 283300-REACTOPS; the third author has been supported by the Portuguese Science Foundation (FCT-Fundação para a Ciência e Tecnologia) under the Project UID/MAT/04561/2013 (CMAF-CIO/FCUL). The authors would like to thank the two anonymous reviewers for their valuable comments, suggestions and insights that helped improving the manuscript.
Author information
Authors and Affiliations
Corresponding author
Appendix: Tables of numerical experiments of Sect. 4.1
Appendix: Tables of numerical experiments of Sect. 4.1
Tables 8, 9 and 10 contain the CPU times (seconds) required by the Benders decomposition and the branch-and-cut to solve instances of (1)–(8), with one, three and six time periods respectively. The parameter specification has been defined in Table 1, with different combinations of \(\alpha \) and \(\beta \) and for two sizes \(m = n = 500\) and \(m = n = 1000\).
1.1 One period
See Table 8.
1.2 Three periods
See Table 9.
1.3 Six periods
See Table 10.
Rights and permissions
About this article
Cite this article
Castro, J., Nasini, S. & Saldanha-da-Gama, F. A cutting-plane approach for large-scale capacitated multi-period facility location using a specialized interior-point method. Math. Program. 163, 411–444 (2017). https://doi.org/10.1007/s10107-016-1067-6
Received:
Accepted:
Published:
Issue Date:
DOI: https://doi.org/10.1007/s10107-016-1067-6
Keywords
- Mixed integer linear optimization
- Interior-point methods
- Multi-period facility location
- Cutting planes
- Benders decomposition
- Large-scale optimization