Abstract
The assessment of aquifer vulnerability to pollution is crucial for planning a sound management strategy of groundwater quality protection and farmland fertilizer use. This study establishes a reliable model for aquifer vulnerability assessment with an excellent performance for predicting groundwater nitrate-N contamination in the Choushui River alluvial fan, Taiwan based on the DRASTIC method. To promote the prediction performance of aquifer vulnerability assessment, discriminant analysis (DA) was applied to determine the weights of factors in the DRASTIC model by comparing the model results with the observed nitrate-N data. Key factors influencing the presence of groundwater nitrate-N pollution were characterized for different concentration thresholds. The results of analysis reveal that the modified DRASTIC model using DA significantly improves prediction performance for aquifer vulnerability assessment, and groundwater protection zones can be determined correctly based on the modified DRASTIC index. Furthermore, the sensitivity of the factors in the modified DRASTIC model indicates that the depth to the groundwater and aquifer media are critical when the nitrate-N concentration is less than 3 mg/L, while the impact of the vadose zone plays a vital role in controlling nitrate-N pollution of over 5 mg/L.
Similar content being viewed by others
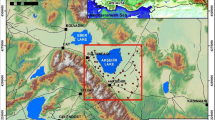
Explore related subjects
Discover the latest articles, news and stories from top researchers in related subjects.Avoid common mistakes on your manuscript.
1 Introduction
As a consequence of finite surface water resources, groundwater is frequently used to supply various water demands in many agricultural regions in Taiwan. However, agricultural activities easily contaminate and affect the quality of groundwater. Polluted groundwater may impact various types of water utilization, such as for irrigation, aquaculture, and drinking purposes (Militino et al. 2008; Jang et al. 2012, 2013a). Nitrate-N (NO3 −-N) in groundwater, which mainly stems from the application of fertilizer and manure compost to farmlands, is a common pollutant that typically appears in natural recharging zones (Chen and Liu 2003; García-Díaz 2011). In Taiwan, nitrate-N pollution in groundwater has increased markedly during the past several decades (Chen and Liu 2003; Jang and Liu 2005). A long-term groundwater quality survey revealed that NO3 −-N concentrations in some of the monitoring wells exceeded the Taiwanese standards for drinking water quality of 10 mg/L (Agriculture Engineering Research Center 2009). Moreover, there is much epidemiological evidence demonstrating that long-term NO3 −-N exposure may result in the occurrence of several diseases, such as blue baby syndrome, gastric cancer, thyroid disease, and diabetes (Knobeloch et al. 2000). Yang et al. (1998) also reported a relationship between gastric cancers and long-term drinking of low-dose nitrate-polluted water in Taiwan. In short, the increasing NO3 −-N pollution in groundwater poses a potential threat to human health.
Assessment of aquifer vulnerability is a common approach requiring analysis of the occurrence of groundwater nitrate-N and the mapping of potential contamination under uncertainty conditions (Saidi et al. 2011). Three methods for assessing aquifer vulnerability include (1) an overlay and index method; (2) a method employing process-based simulation models; and (3) a statistical method (National Research Council 1993). The DRASTIC model of aquifer vulnerability developed by the U.S. EPA (U.S. EPA 1985; Aller et al. 1987) is such an overlay and index method, and has been comprehensively used to evaluate potentials of groundwater pollution in the United States and other countries (Aller et al. 1987; Babiker et al. 2005; Baalousha 2006; Assaf and Saadeh 2009; Leone et al. 2009). For example, Babiker et al. (2005) used the GIS-based DRASTIC model to evaluate aquifer vulnerability in central Japan. Their study indicated that net recharge and hydraulic conductivity were more effective than the DRASTIC index for aquifer vulnerability assessment. Assaf and Saadeh (2009) and Leone et al. (2009) adopted the DRASTIC model to analyze risks and vulnerability of agricultural potential nitrogen pollution. Baalousha (2010) established the DRASTIC vulnerability map to plan a nitrate monitoring network in New Zealand. However, many studies also indicated that the DRASTIC model has the low prediction performance for assessing aquifer vulnerability in relation to nitrate-N pollution compared to data of observation (Baalousha 2006; Panagopoulos et al. 2006; Antonakos and Lambrakis 2007; Assaf and Saadeh 2009). Therefore, Antonakos and Lambrakis (2007) developed a modified DRASTIC method, which determined rating scales and factor weights of factors using logistic regression and weights of evidence based on observed nitrate-N data, in attempt to improve the prediction accuracy of actual nitrogen pollution in groundwater. Saidi et al. (2011) modified the effective weights of factors in the DRASTIC model using sensitivity analysis to determine hazard zones for agricultural nitrogen pollution. Huan et al. (2012) employed a modified DRASTIC model to assess the aquifer vulnerability to nitrate in Jilin City of northeast China. In order to reduce the subjectivity of the overlay index method, the model was optimized by rebuilding the index system, adjusting the rating scale of each index, reassigning the index weights, and comparing grading methods for groundwater vulnerability to nitrate.
The multivariate statistical technique is an effective tool for exploring the relationship among hydrogeological, hydrological, and hydrochemical data, and as a consequence is frequently adopted as classification approaches. Discriminant analysis (DA) is one of the classification approaches to forecast optimal categories using hydrogeological, hydrological, and hydrochemical parameters according to two or more occurrence categories. For instance, Lambrakis et al. (2004) investigated the NO3 − distribution in aquifers using factor, cluster, and discriminant analyses on hydrogeological, hydrochemical, and environmental data. Papatheodorou et al. (2007) adopted DA to examine the evolution of groundwater using several data samples, and to explore the main hydrochemical evolution processes. Huang et al. (2011) used DA to determine principal sources for coastal water quality deterioration based on spatiotemporal variations. DA is rather different to other multivariate statistical techniques because hydrogeological, hydrological, and hydrochemical characteristics of variables need to be grouped in advance. In this work polluted and unpolluted groups were determined based on observed nitrate-N data, and the weights of model factors were characterized using DA.
This study aims to develop a reliable DRASTIC-based model of aquifer vulnerability assessment which offers excellent prediction performance of groundwater nitrate-N contamination in the Choushui River alluvial fan in Taiwan. To enhance the prediction performance for aquifer vulnerability assessment, DA is applied to determine the weights of factors in the modified DRASTIC model via the comparison between the observed nitrate-N data and the results of the modified DRASTIC model. The critical factors influencing groundwater nitrate-N pollution are characterized in terms of low, medium, and high concentrations. The research findings offer government administrators sound guidelines for establishing groundwater protection zones and fertilizer management strategies, which should help decrease potential risks to human health due to drinking nitrate-N-polluted water.
2 Hydrogeology of the study area
The Choushui River alluvial fan is located in the western Taiwan and enclosed by the Taiwan Strait to the west, the Wu River to the north, the Dulliu Hill and Baguah Mount to the east, and the Peikang River to the south (Fig. 1). The alluvial fan occupies an area of about 2500 km2, and can be mainly classified into the proximal-fan, mid-fan, and distal-fan areas. The quaternary unconsolidated sediments underlying the alluvial fan are abundant in groundwater. The proximal-fan is the primary zone for natural aquifer recharging. Agricultural landscapes, such as paddy fields and dry farmlands, are a common sight in this plain. According to climate statistics between 1998 and 2012, the average annual precipitation is approximately 1572 mm in the area.
Geological and hydrogeological drilling analyses were conducted from 1992 to 1998 to evaluate the subsurface characteristics of the alluvial fan. A network of 77 hydrogeological investigation stations and 185 monitoring wells drilled to aquifers of various depths was simultaneously established. The results of the hydrogeological analysis to a depth of approximately 300 m divided the fan deposits in the distal-fan and mid-fan areas into four marine deposits and four terrestrial deposits (Taiwan Central Geological Survey (CGS) 1999). Terrestrial deposits ranging from medium sand to gravel with high permeability are considered to be aquifers, whereas marine deposits ranging from clay to fine sand with low permeability are regarded as aquitards (Liu et al. 2006) (Fig. 2). The proximal-fan formation is an unconfined aquifer. Aquitards are primarily located in the distal-fan and mid-fan areas. The four aquifers from top to bottom are named “aquifer 1”, “aquifer 2”, “aquifer 3”, and “aquifer 4”. The depths of aquifers 1, 2, 3, and 4 vary from 19–66 m, 47–148 m, 122–243 m, and 200–317 m, respectively.
Hydrogeological profile A–B marked in the Fig. 1
3 DRASTIC model
The DRASTIC model of aquifer vulnerability considers seven hydrological and hydrogeological factors that influence and dominate the unsaturated and saturated groundwater flow, and pollutant transport processes into, via, and out of a region (U.S. EPA 1985; Aller et al. 1987). The seven factors of the DRASTIC model are the depth to groundwater (D), net recharge (R), aquifer media (A), soil media (S), topography (T), impact of the vadose zone (I), and hydraulic conductivity (C). The DRASTIC model adopts an index derived from ratings and weights assigned to the seven factors. The several levels of each factor are measured and rated with scores from 1 to 10 (i.e., the ratings), and the seven factors are assigned weights ranging from 1 to 5 according to the significance to pollution. Table 1 lists the weights of the factors used in the traditional DRASTIC model. The DRASTIC index is quantified by using a linear combination of the products of ratings and weights for the seven factors, and is expressed as follows.
where the subscripts w and r of the factors refer to the corresponding weights and ratings, respectively.
4 Data preparation of the DRASTIC model
The seven factors in the DRASTIC model are related to several types of data. Table 2 reports data types and sources used in this study. This work used data on the average monthly groundwater levels between 1999 and 2005 recorded by the Taiwan Water Resources Agency (WRA) (2012). The depth to groundwater was obtained by subtracting the average monthly groundwater level from the top elevation in each well. Because the DRASTIC model highlights the groundwater movement and pollution processes from the ground to the uppermost saturated groundwater, only 46 data for the groundwater levels located in aquifer 1 were considered to acquire the depth to groundwater (shown in Fig. 1). The data on average rainfall and evapotranspiration between 1999 and 2005 observed by the Taiwan WRA (2012) and Taiwan Central Weather Bureau (CWB) (2012), respectively, were adopted to calculate the net recharge. The net recharge at each rainfall station was determined according to the following formula (Babiker et al. 2005):
Ten rainfall stations and two evapotranspiration stations were situated in the study area (Fig. 1). To simplify the evapotranspiration calculation, the spatial variability of evapotranspiration data was not considered. The average of the two evapotranspiration data readings was adopted in Eq. (2). Moreover, the net recharges for various land use types were estimated with the reference to the recharge ratios proposed by Chow et al. (1988) and shown in Table 3. Furthermore, the borehole data on soil textures published by the Taiwan CGS (2012) were used to analyze the factors of aquifer media, soil media, and impact of the vadose zone. Before analyzing the borehole data, the average monthly groundwater level was utilized to determine unsaturated zones and saturated aquifers in each borehole (Jang et al. 2013b). The factor of soil media related to the texture of the soil from the ground surface to the 1 m depth. The factor of the impact of the vadose zone represented the soil texture from the 1 m depth to the uppermost saturated aquifer. The factor of the aquifer media denoted the soil texture within the saturated aquifer. Analysis of the three factors obtained from borehole data required prior knowledge of groundwater levels and location of the unsaturated zones and saturated aquifers; therefore, only 46 borehole data were used in this study (Fig. 1). Although the geological and hydrogeological conditions in this study area frequently present a complicated pattern of several overlapping soil layers, dominant soil textures were selected to represent the three factors. The slope for the topography factor was estimated from the elevation obtained using the digital terrain model (DTM). Forty-six data on hydraulic conductivity in aquifer 1, published by the Taiwan CGS (1999), were used in this work (Fig. 1). Additionally, the average observed nitrate-N concentrations between 1999 and 2003 documented by the Taiwan Sugar Company (TSC) (1999, 2000, 2001, 2002, 2003) were used to determine the polluted and unpolluted groups in this study area according to different concentration thresholds. The observed nitrate-N concentrations at 46 monitoring wells ranged from 0 to 12.7 mg/L. This study individually used the nitrate-N thresholds of 0.5, 3, and 5 mg/L to establish groundwater protection zones. Polluted and unpolluted groups in the modified DRASTIC model were determined based on each nitrate-N threshold. Observed nitrate-N data exceeding or equaling the threshold belonged to the polluted group, while those less than the threshold were regarded as the unpolluted group. Nitrate-N concentrations exceeding 0.5, 3, and 5 mg/L were found for 14, 11, and 7 wells (Fig. 1), respectively.
5 Discriminant analysis
Discriminant analysis (DA) is a multivariate statistical approach that is extensively used to characterize several parameters into mutually exclusive and exhaustive categories according to independent variables (Wiggine et al. 1999). DA has two functions—classification and prediction. DA is used to partition cases into the values of a categorical dependent variable. If DA is effective, the classification of correct and incorrect estimates gives rise to a high percentage of correct ratios. A discriminant function is a latent variable established by using a linear combination of independent variables. Linear combinations of independent variables using DA discriminate among the categories and minimize misclassification. In DA, a discriminant function is established for the known category g and is expressed as
where k is the constant; n represents the number of parameters; and w j denotes the weight assigned to a parameter p j by DA (Sharma 1996). DA is based on prior knowledge of separated samples by various categories in advance. Classification using DA objectively characterizes the relationship between the main parameters via the linear combinations of the hit ratio which is defined as the ratio of the correctly classified number to the total number. A larger hit ratio stands for a more excellent classification.
This study classified monitoring wells into two groups—pollution and no pollution—in advance based on observed nitrate-N data. DA was employed to establish the discrimination model with all factors of the DRASTIC model. SPSS (SPSS Inc. 1998) was used to execute DA. Additionally, the area under the curve (AUC) of the receiver operating characteristic (ROC) was adopted to quantify the classification results (Swets 1995). The correct classification ratio, which is defined as a ratio of the true positive and true negative to the total number (i.e., (a + d)/N in Table 4), was also used to gauge the prediction accuracy.
6 Ratings of factors and index in the traditional DRASTIC model
This work determined ratings of the factors D, R, T, and C according to the standards of the U.S. EPA (1985) and Aller et al. (1987). Meanwhile, because the factors A, S, and I are categorical variables, and the hydrogeological settings in the U.S. are considerably different from those in the alluvial fan in Taiwan, this study used eight categories of soil textiles proposed by the Taiwan CGS (1999)—coarse gravel (cG), gravel (G), fine gravel (fG), coarse sand (cS), medium sand (mS), fine sand (fS), mud (M), and clay (C)—to gauge ratings of the factors. Table 5 lists the ratings of the factors in the DRASTIC model. Figure 3 shows the box-and-whisker plots of the ratings of each factor for observed nitrate-N. The analyzed results account for the high ratings of factors R, A, S, and I having the wide distributions of observed nitrate-N concentrations. Meanwhile, the low ratings of factors D and T possess the broad distributions of observed nitrate-N concentrations. The distributions of observed nitrate-N concentrations are wide for the high and low ratings of factor C, while the medium ratings of factor C possess low observed nitrate-N concentrations. Figure 4 maps the spatial distributions of the ratings of the factors. The ratings of factors R, A, S, I, and C are high in the proximal-fan and eastern regions. High factor D ratings are mainly distributed in the mid-fan and distal-fan zones, while factor T has low ratings in the plains, but high in the eastern foothills.
The traditional DRASTIC index was calculated with Eq. (1) using the aforementioned ratings and weights of the factors. Moreover, the kriging method of the SURFER software was used for spatial interpolation of the ratings of each factor based on well data. A 65 × 85 grid was discretized with a spacing of 1000 m. Figure 5 displays the spatial distributions of the traditional DRASTIC index ranging between 61 and 215. The high DRASTIC indexes (>145), which denote high contamination potentials of nitrate-N, are present primarily in the proximal-fan and southeastern and central mid-fan regions.
7 Modified DRASTIC model using DA to determine weights of factors
It has been pointed out in several studies that the index of the traditional DRASTIC model is poorly correlated with the observed nitrate-N concentrations (Baalousha 2006; Antonakos and Lambrakis 2007; Assaf and Saadeh 2009). In this study, the correlation coefficient between them was 0.15. To enhance the prediction performance of aquifer vulnerability assessment, this work adopted DA to determine the weights of the factors in the DRASTIC model based on the observed nitrate-N concentrations. Nitrate-N concentration thresholds of 0.5, 3, and 5 mg/L were used to characterize low, medium, and high levels of pollution, respectively. Polluted and unpolluted groups were categorized for each aforementioned concentration threshold.
The discriminant functions for nitrate-N concentration levels of 0.5, 3, and 5 mg/L are as follows.
The analyzed results indicate that factors R, A, and I positively contribute to the DRASTIC index, whereas factor D is negatively correlated with the DRASTIC index for the three thresholds. Chen and Liu (2003) documented that because a thick gravel layer is present in the proximal-fan of the Choushui River alluvial fan, nitrate-N can quickly move downward to deep aquifers (more than 200 m). Thus, the depth to groundwater is negatively correlated with the nitrate-N concentrations in this study. The positive or negative contributions of factors S, T, and C to the DRASTIC index vary with various thresholds. Antonakos and Lambrakis (2007) also presented a negative weight for factor I in the modified DRASTIC model using logistic regression for the nitrate concentration level exceeding 50 mg/L. Additionally, the DRASTIC index was newly obtained from the discriminant functions and the ratings of the factors. The ratings of the factors in the modified DRASTIC model were the same as those in the traditional one. A cut-off value of the DRASTIC index was calculated from the averages of the polluted and unpolluted groups.
The modified DRASTIC model using DA to adjust weights of the factors obtains correct classification ratios of polluted and unpolluted groups ranging from 81 to 87 %, while the AUC ranges from 0.91 to 0.95 for three thresholds. This is indicative of its high prediction performance for aquifer vulnerability assessment. For the traditional DRASTIC model, the correct classification ratios of polluted and unpolluted groups only range between 49 and 67 %, and the AUC is 0.62. The above results illustrate that the prediction performance of the modified DRASTIC model is superior to that of the traditional DRASTIC model. Figure 6 shows the modified DRASTIC indexes for concentration thresholds of 0.5, 3, and 5 mg/L, respectively. Recommended groundwater protection zones were delineated according to cut-off values reported in Table 6. Figure 7 maps the determined protection zones obtained with the traditional and modified DRASTIC models. The determined protection zones in the modified DRASTIC model are smaller than those in the traditional DRASTIC model, indicating that the protection zones delineated by the modified DRASTIC model are more accurate than those determined by the traditional DRASTIC model. A management scheme for farmland fertilizer utilization can be effectively implemented in the groundwater protection zones determined by the modified DRASTIC model.
8 Sensitivity of the Factors
This study attempted to characterize the sensitivity of the factors in the aforementioned discriminant functions, and examine key factors influencing groundwater nitrate-N pollution in the Choushui River alluvial fan. The average values of each factor for polluted and unpolluted groups were individually calculated, and served as inputs into Eqs. (4), (5), and (6) for different nitrate-N thresholds. The absolute difference of each factor between the polluted and unpolluted groups was used to evaluate the sensitivity of factors in the discriminant functions.
Table 7 lists the absolute difference of each factor between the polluted and unpolluted groups for the nitrate-N threshold of 0.5 mg/L. The results of the analysis reveal that terms 0.18A and −0.17D in discriminant function 1 greatly impacts the aquifer vulnerability assessment. Table 8 documents the absolute difference of each factor between the polluted and unpolluted groups for the nitrate-N threshold of 3 mg/L. Term 0.4A in discriminant function 2 has significant influences on the aquifer vulnerability assessment, while the term −0.1D is second. Table 9 reports the absolute difference for each factor between the polluted and unpolluted groups for the nitrate-N threshold of 5 mg/L. Term 0.28I in discriminant function 3 is the most critical factor, dominating the aquifer vulnerability assessment. Accordingly, factors D and A are the key factors impacting the nitrate-N pollution level of less than 3 mg/L (medium and low thresholds), whereas factor I plays the crucial role in controlling nitrate-N pollution of over 5 mg/L (high threshold).
Compared with previous studies related to the DRASTIC models, Babiker et al. (2005) documented that the factor of net recharge is the most important for assessing aquifer vulnerability, Antonakos and Lambrakis (2007) stated that the depth to groundwater, aquifer media, topography, impact of the vadose zone media, and land use are significantly correlated to groundwater nitrate-N pollution, and Saidi et al. (2011) considered that hydraulic conductivity and topography play important roles in assessing aquifer vulnerability. However, our study results differ from their ones, revealing that the causes of groundwater nitrate-N pollution depend on regional hydrological and hydrogeological characteristics. Additionally, previous modified DRASTIC studies using statistical methods, such as Panagopoulos et al. (2006), Antonakos and Lambrakis (2007), and Chen et al. (2013), adopted a single nitrate-N threshold to discuss the relationship between the groundwater pollution and several environmental factors. This work used three nitrate-N thresholds to characterize various levels of pollution and the major factors. Our research findings obtained from the multi-threshold analysis of nitrate-N pollution can give government administrators excellent insights in differentiating management schemes for farmland fertilizer use. For example, in a determined groundwater protection zone, farmland fertilizer use should be more rigorous in the regions with highly permeable vadose zones than in those with deep groundwater and highly permeable aquifer media.
9 Conclusions
This study modified the weights of the factors in the DRASTIC model using DA and determined the DRASTIC index in the Choushui River alluvial fan. According to the research findings, the prediction performance of groundwater nitrate-N pollution in the modified DRASTIC model can be significantly improved compared to that of the traditional DRASTIC model. To avoid great exposure risks which will affect public health, the high index of the modified DRASTIC model can be used to determine groundwater protection zones where farmland fertilizer utilization should be substantially reduced. Additionally, it is found that the depth to groundwater and aquifer media has a substantial impact on the nitrate-N pollution of medium to low concentrations (0.5–3 mg/L), while the impact of the vadose zone remarkably influence nitrate-N pollution in high concentrations (exceeding 5 mg/L). The multi-threshold analysis result is useful for differentiating the management schemes in the determined groundwater protection zones. This research suggests that farmland fertilizer use should be strictly limited in the regions with highly permeable vadose zones.
References
Aller L, Bennett T, Lehr JH, Petty RJ, Hackett G (1987) DRASTIC: a standardized system for evaluating ground water pollution potential using hydrogeologic settings. EPA-600/2-87-035, EPA, Washington, DC
Antonakos AK, Lambrakis NJ (2007) Development and testing of three hybrid methods for the assessment of aquifer vulnerability to nitrates, based on the drastic model, an example from NE Korinthia, Greece. J Hydrol 333:288–304
Agriculture Engineering Research Center (2009) Survey, analysis and assessment of groundwater quality in Taiwan areas in 2009. Water Resources Agency, Ministry of Economic Affairs, Executive Yuan, Taiwan, pp 100–102
Assaf H, Saadeh M (2009) Geostatistical assessment of groundwater nitrate contamination with reflection on DRASTIC vulnerability assessment: the case of the upper Litani basin, Lebanon. Water Resour Manag 23:775–796
Baalousha H (2006) Vulnerability assessment for the Gaza Strip, Palestine using DRASTIC. Environ Geol 50:405–414
Baalousha H (2010) Assessment of a groundwater quality monitoring network using vulnerability mapping and geostatistics: a case study from Heretaunga Plains, New Zealand. Agric Water Manag 97:240–246
Babiker IS, Mohamed AAM, Terao H, Kato K (2005) A GIS-based DRASTIC model for assessing aquifer vulnerability in Kakamigahara Heights, Gifu Prefecture, central Japan. Sci Total Environ 345(1–3):127–140
Chen WF, Liu TK (2003) Dissolved oxygen and nitrate of groundwater in Choushui Fan-Delta, western Taiwan. Environ Geol 44(6):731–737
Chen SK, Jang CS, Peng YH (2013) Developing a probability-based model of aquifer vulnerability in an agricultural region. J Hydrol 486:494–504
Chow VT, Maidment D, Mays LW (1988) Applied hydrology. McGraw Hill, New York, p 498
García-Díaz JC (2011) Monitoring and forecasting nitrate concentration in the groundwater using statistical process control and time series analysis: a case study. Stoch Env Res Risk Assess 25(3):331–339
Huan H, Wang J, Teng Y (2012) Assessment and validation of groundwater vulnerability to nitrate based on a modified DRASTIC model: a case study in Jilin City of northeast China. Sci Total Environ 440:14–23
Huang J, Ho M, Du P (2011) Assessment of temporal and spatial variation of coastal water quality and source identification along Macau peninsula. Stoch Env Res Risk Assess 25(3):353–361
Jang CS, Liu CW (2005) Contamination potential of nitrogen compounds in the heterogeneous aquifers of the Choushui River alluvial fan, Taiwan. J Contam Hydrol 79(3–4):135–155
Jang CS, Chen SK, Kuo YM (2012) Establishing an irrigation management plan of sustainable groundwater based on spatial variability of water quality and quantity. J Hydrol 414–415:201–210
Jang CS, Liang CP, Wang SW (2013a) Integrating the spatial variability of water quality and quantity to probabilistically assess groundwater sustainability for use in aquaculture. Stoch Env Res Risk Assess 27(6):1281–1291
Jang CS, Chen SK, Kuo YM (2013b) Applying indicator-based geostatistical approaches to determine potential zones of groundwater recharge based on borehole data. Catena 101:178–187
Knobeloch L, Salna B, Hogan A, Postle J, Anderson H (2000) Blue babies and nitrate-contaminated well water. Environ Health Perspect 108(7):675–678
Lambrakis N, Antonakos A, Panagopoulos G (2004) The use of multicomponent statistical analysis in hydrogeological environmental research. Water Res 38:1862–1872
Leone A, Ripa MN, Uricchio V, Deák J, Vargay Z (2009) Vulnerability and risk evaluation of agricultural nitrogen pollution for Hungary’s main aquifer using DRASTIC and GLEAMS models. J Environ Manag 90(10):2969–2978
Liu CW, Wang SW, Jang CS, Lin KH (2006) Occurrence of arsenic in groundwater of the Choshui river alluvial fan, Taiwan. J Environ Qual 35:68–75
Militino AF, Ugarte MD, Ibáñez B (2008) Longitudinal analysis of spatially correlated data. Stoch Env Res Risk Assess 22(1):49–57
National Research Council (1993) Ground water vulnerability assessment: predictive relative contamination potential under conditions of uncertainty. National Academy Press, Washington, DC, pp 42–63
Panagopoulos GP, Antonakos AK, Lambrakis NJ (2006) Optimization of the DRASTIC method for groundwater vulnerability assessment via the use of simple statistical methods and GIS. Hydrogeol J 14:894–911
Papatheodorou G, Lambrakis N, Panagopoulos G (2007) Application of multivariate statistical procedures to the hydrochemical study of a coastal aquifer: an example from Crete, Greece. Hydrol Process 21:1482–1495
Saidi S, Bouri S, Ben Dhia H, Anselme B (2011) Assessment of groundwater risk using intrinsic vulnerability and hazard mapping: application to Souassi aquifer, Tunisian Sahel. Agric Water Manag 98(10):1671–1682
Sharma S (1996) Applied multivariate techniques. Wiley, New York, pp 264–273
SPSS Inc. (1998) SPSS BASE 8.0- Application Guide. SPSS Inc, Chicago
Swets JA (1995) Signal detection theory and ROC analysis in psychology and diagnostics: Collected Papers. Lawrence Erlbaum Associates
Taiwan Central Geological Survey (CGS) (1999) Project of groundwater monitoring network in Taiwan during first stage: research report of Choushui River alluvial fan. Water Resources Bureau, Taipei, p 383
Taiwan Central Geological Survey (CGS) (2012) Hydrogeological database. Central Geological Survey, Ministry of Economic Affairs, Executive Yuan, Taiwan. http://hydro.moeacgs.gov.tw/
Taiwan Central Weather Bureau (CWB) (2012) Internet application of climate data. Central Weather Bureau, Ministry of Transportation & Communications, Executive Yuan, Taiwan. http://e-service.cwb.gov.tw/wdps/index_net.jsp
Taiwan Sugar Company (TSC) (1999) Groundwater quality by the Taiwan groundwater monitoring network (1/5)—Part 1: the Choushui River alluvial fan. Water Resources Bureau, Taipei, pp 197–204
Taiwan Sugar Company (TSC) (2000) Groundwater quality by the Taiwan groundwater monitoring network (2/5)—Part 1: the Choushui River alluvial fan. Water Resources Bureau, Taipei, pp 140–148
Taiwan Sugar Company (TSC) (2001) Groundwater quality by the Taiwan groundwater monitoring network (3/5)—Part 3: the Choushui River alluvial fan. Water Resources Bureau, Taipei, pp 68–74
Taiwan Sugar Company (TSC) (2002) Groundwater quality by the Taiwan groundwater monitoring network (4/5). Water Resources Bureau, Taipei, pp 48–51
Taiwan Sugar Company (TSC) (2003) Groundwater quality by the Taiwan groundwater monitoring network (5/5). Water Resources Bureau, Taiwan, pp. 5-31-5-35
Taiwan Water Resources Agency (WRA) (2012) Application network of hydrological data. Water Resources Agency, Ministry of Economic Affairs, Executive Yuan, Taiwan. http://gweb.wra.gov.tw/HydroApplication/index.aspx
U.S. EPA (Environmental Protection Agency) (1985) DRASTIC: A standard system for evaluating groundwater potential using hydrogeological settings. Oklahoma WA/EPA Series, Ada, pp 163
Wiggine BA, Andrew RW, Conway RA, Corr CL, Dobratz EJ, Dougherty DP, Eppard JR, Knupp SR, Limjoco MC, Mettenburg JM, Rinehardt JM, Sonsino J, Torrijos RL, Zimmerman ME (1999) Use of antibiotic resistance analysis to identify nonpoint sources of fecal pollution. Appl Environ Microbiol 65:3483–3486
Yang CY, Cheng MF, Tsai SS, Hsieh YL (1998) Calcium, magnesium, and nitrate in drinking water and gastric cancer mortality. Cancer Sci 89:124–130
Acknowledgments
The authors would like to thank the Ministry of Science and Technology, Taiwan for financially supporting this research under Contract No. NSC 98-2116-M-242-001.
Author information
Authors and Affiliations
Corresponding author
Rights and permissions
About this article
Cite this article
Jang, CS., Lin, CW., Liang, CP. et al. Developing a reliable model for aquifer vulnerability. Stoch Environ Res Risk Assess 30, 175–187 (2016). https://doi.org/10.1007/s00477-015-1063-z
Published:
Issue Date:
DOI: https://doi.org/10.1007/s00477-015-1063-z