Abstract
The tau hypothesis has been raised with regard to the pathophysiology of Alzheimer’s disease (AD). Mild cognitive impairment (MCI) is associated with a high risk for developing AD. However, no study has directly examined the brain topological alterations based on combined effects of tau protein pathway genes in MCI population. Forty-three patients with MCI and 30 healthy controls underwent resting-state functional magnetic resonance imaging (fMRI) in Chinese Han, and a tau protein pathway-based imaging approaches (7 candidate genes: 17 SNPs) were used to investigate changes in the topological organisation of brain activation associated with MCI. Impaired regional activation is related to tau protein pathway genes (5/7 candidate genes) in patients with MCI and likely in topologically convergent and divergent functional alterations patterns associated with genes, and combined effects of tau protein pathway genes disrupt the topological architecture of cortico-cerebellar loops. The associations between the loops and behaviours further suggest that tau protein pathway genes do play a significant role in non-episodic memory impairment. Tau pathway-based imaging approaches might strengthen the credibility in imaging genetic associations and generate pathway frameworks that might provide powerful new insights into the neural mechanisms that underlie MCI.
Similar content being viewed by others
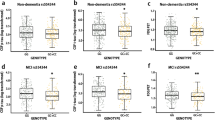
Avoid common mistakes on your manuscript.
Introduction
Alzheimer’s disease (AD) is a progressive neurodegenerative disorder, and brain neurofibrillary tangles (NFTs) are a characteristic neuropathological lesion of this disorder. NFTs consist of hyperphosphorylated forms of the microtubule-associated protein tau (Grundke-Iqbal et al. 1986). Several protein kinases regulate the phosphorylation of tau, and these kinases are associated with MAPT\STH (Chen et al. 2010), GSK3β (Hooper et al. 2008), LRP6 (Small and Duff 2008), CDK5 (Shelton and Johnson 2004), CDC2 (Pei et al. 2002), CAMK2G (Yamamoto et al. 2005) and DDYRK1A (Ryoo et al. 2007) in the brains of patients with AD. Tau protein is a promising diagnostic candidate and therapeutic target for AD (Maccioni et al. 2010).
In the tau hypothesis, intracellular aggregates of tau (either in soluble or insoluble forms) likely disrupt cellular machinery and synaptic function, which ultimately leads to neuronal death (Tolnay and Probst 1999; Marcus and Schachter 2011). Altered and aggregated forms of tau likely act as toxins that contribute to neurofibrillary degeneration in AD-affected brains. This degeneration directly reflects aberrant brain function (Wang et al. 2007). Neurofibrillary degeneration starts at the hippocampal formation, reaches the polymodal association areas, and then successively spreads to the unimodal association areas and the entire cerebral cortex (Braak and Braak 1991; Delacourte et al. 1999), involving frontal and parietal cortex (Leinonen et al. 2010; Guadagna et al. 2012), basal ganglia (Attems et al. 2012), brainstem (Dugger et al. 2011) and cerebellum (Sepulveda-Falla et al. 2011). These regions may be involved in cortico-cerebellar loops (Gomez-Beldarrain and Garcia-Monco 1998; Georgiou-Karistianis et al. 2012).
Advances in the development and application of functional magnetic resonance imaging (fMRI) have allowed us to investigate the topological patterns of brain function in AD (Sperling et al. 2011). Recently, genetic susceptibility occurring at multiple genes and the interactions among them, as well as environmental factors, likely influence the risk of AD (Williamson et al. 2009). Recent imaging genetic studies have suggested that the genes also influence neuroimaging correlates associated with AD, including the APOE ε4 allele (Schuff et al. 2009; Sheline et al. 2010; Vemuri et al. 2010; Frank et al. 2011; Westlye et al. 2011; Koch et al. 2012; Trachtenberg et al. 2012), COMT Val158Met (Lancaster et al. 2011) and the ACE D allele (Zhang et al. 2010). Our recent study also showed gene-brain-behaviour interactions involving a GSK3β polymorphism in patients with mild cognitive impairment (MCI) (Bai et al. 2012). These findings hold promise for understanding the underlying mechanisms using image-derived endophenotypes in the emerging field of imaging genetics. However, it should be noted that single candidate gene-based approach might coherently account for the diversity and lack of independent replications of these associations, and the occurrence of AD might be the result of the integrated effects of multiple genes (Williamson et al. 2009; Liu et al. 2012).
An alternative pathway-based approach has been suggested to address this issue by testing polymorphisms in multiple functionally related genes to increase credibility in imaging genetics and generate a pathway framework (Inkster et al. 2010). However, no study has directly examined the brain topological alterations based on combined effects of tau protein pathway genes, i.e., MCI that is at high risk for AD (Petersen et al. 1999; Petersen and Negash 2008). Resting-state fMRI provides a viable alternative imaging approach for the assessment of brain function in the low-frequency range (<0.1 Hz) of blood oxygenation level dependent (BOLD) fluctuations. Zang et al. (2007) developed an index, amplitude of low-frequency fluctuations (ALFF) in which the square root of power spectrum was integrated in a low-frequency range, for detecting the regional intensity of spontaneous fluctuations in BOLD signal. Specifically, low-frequency fluctuations in BOLD-fMRI signals are closely related to spontaneous neuronal activity (Zang et al. 2007). Furthermore, ALFF has already been applied in a wide range of brain disorders including Parkinson’s disease (Long et al. 2012), schizophrenia (Yu et al. 2014), post-traumatic stress disorder (Bing et al. 2012), Alzheimer’s disease (Wang et al. 2011), MCI (Bai et al. 2012) and so on. Therefore, we used tau protein pathway-based imaging approaches to investigate changes in the topological organisation of brain activation in patients with MCI using ALFF. As described above, we sought to determine whether this pathway-based approach would show (a) detailed topological patterns of brain activation associated with tau protein pathway genes in patients with MCI; and (b) the associations of tau protein pathway genes that are related to architectural disruptions in the cortico-cerebellar loops of the patients group.
Materials and methods
Subjects
In the present study, 73 elderly individuals (right handed), including 43 MCI subjects and 30 healthy controls, were recruited through normal community health screening and newspaper advertisements. The study was approved by the Research Ethics Committee of Affiliated Zhong-Da Hospital, Southeast University, and written informed consent was obtained from all participants. All subjects underwent diagnostic evaluations.
Inclusion criteria
The diagnosis of MCI, including single domain (impairment involving only the memory domain) and multiple domain (impairments in the memory domain plus at least one other cognitive domain), was made following the recommendations of Petersen et al. (1999) and others (Winblad et al. 2004): (a) subjective memory impairment corroborated by the subject and an informant; (b) objective memory performance documented by an AVLT-delayed recall score less than or equal to 1.5 SD of age- and education-adjusted norms (cut-off of ≤4 correct responses on 12 items for patients with ≥8 years of education); (c) MMSE score of 24 or higher; (d) CDR of 0.5; (e) no or minimal impairment in activities of daily living; (f) absence of dementia or insufficient dementia to meet the NINCDS-ADRDA (National Institute of Neurological and Communicative Disorders and Stroke and the Alzheimer’s Disease and Related Disorders Association) Alzheimer’s criteria. In addition, the controls were required to have a CDR of 0, an MMSE score ≥26, and an AVLT-delayed recall score >4 for subjects with 8 or more years of education.
Exclusion criteria
Participants were excluded from the study if they had a history of known stroke, alcoholism, head injury, Parkinson’s disease, epilepsy, major depression or other neurological or psychiatric illness, major medical illness, or severe visual or hearing loss.
SNP genotyping and candidate gene selection of tau protein pathway genes
Investigators blinded to all participant identifiers and information performed the genotype analysis. Blood samples were obtained from 43 patients with MCI and 30 controls. The data were processed and analysed using MassARRAY TYPER 4.0 software (Sequenom). In all, 17 previously suggested tagging SNPs from 7 candidate genes were selected based on the AD tau hypothesis, the Alzgene website and HapMap (Liu et al. 2012): MAPT\STH, GSK3β, LRP6, CDK5, CDC2, CAMK2G and DYRK1A (for details, see Table 1). The Hardy–Weinberg equilibrium was checked using the χ 2 test. SNPs were excluded from the study if (a) they demonstrated complete linkage disequilibrium (LD) with another SNP (i.e., the alleles were completely correlated with the alleles of another SNP) or (b) the minor allele frequency (MAF) was lower than 5 % (Inkster et al. 2010). Consequently, 17 SNPs from seven candidate genes can be detected in all subjects and entered into the following analysis (a flowsheet see Fig. 1).
MRI data acquisition and preprocessing
The subjects were scanned using a General Electric 1.5 Tesla scanner (General Electric Medical Systems, USA) with a homogeneous birdcage head coil. Subjects lay supine with the head snugly fixed by a belt and foam pads to minimize head motion. Conventional axial fast relaxation fast spin echo sequence T2 weighted anatomic MR images were obtained to rule out major white matter changes, cerebral infarction or other lesions: repetition time (TR) = 3500 ms; echo time (TE) = 103 ms; flip angle (FA) = 90°; acquisition matrix = 320 × 192; field of view (FOV) = 240 × 240 mm; thickness = 6.0 mm; gap = 0 mm; no. of excitations (NEX) = 2.0. High-resolution T1-weighted axial images covering the whole brain were acquired using a 3D spoiled gradient echo sequence as follow: TR = 9.9 ms; TE = 2.1 ms; FA = 150; acquisition matrix = 256 × 192; FOV = 240 mm × 240 mm; thickness = 2.0 mm; gap = 0 mm. The functional scans (T2* weighted images) involved the acquisition of 30 contiguous axial slices using a GRE-EPI pulse sequence: TR = 3,000 ms; TE = 40 ms; FA = 90°; acquisition matrix = 64 × 64; FOV = 240 × 240 mm; thickness = 4.0 mm; gap = 0 mm and 3.75 × 3.75 mm2 in-plane resolution parallel to the anterior commissure–posterior commissure line. This acquisition sequence generated 142 volumes in 7 min and 6 s. All subjects have eyes closed during scanning.
Data preprocessing
Data analyses of groups were conducted with SPM5 toolkit (http://www.fil.ion.ucl.ac.uk/spm). The first eight volumes of the scanning session were discarded to allow for T1 equilibration effects. The remaining images were corrected for timing differences and motion effects. No translation or rotation parameters of head motion in any given data set exceeded ±3 mm or ±3°. The resulting images were spatially normalised into the SPM5 Montreal Neurological Institute echo-planar imaging template using the default settings and resampling to 3 × 3 × 3 mm3 voxels, and smoothed with a Gaussian kernel of 8 × 8 × 8 mm. Then, REST software (http://www.restingfmri.sourceforge.net) was used for removing the linear trend of time courses and for temporally band-pass filtering (0.01–0.08 Hz).
ALFF analysis
Separate ALFF analyses were performed for each SNP using REST software following a procedure similar to that used in previous study (Wang et al. 2011; Bai et al. 2012; Bing et al. 2012; Long et al. 2012; Yu et al. 2014). In brief, the time series of the resultant images was transformed to the frequency domain after image preprocessing using a fast Fourier transform (FFT; taper percent = 0, FFT length = shortest). The power spectrum was then obtained. Because spectral power is the square of spectral amplitude, the square root was calculated at each frequency of the power spectrum and averaged between 0.01 and 0.08 Hz at each voxel. The activity in this frequency band was then taken as the ALFF. The current study considered only regions within the brain (i.e., background noise and activity of tissue outside the brain were removed).
Voxelwise-based grey matter volume correction
To control for possible differences in the brain activation results (i.e., the ALFF) that might be explained by differences in between-participant grey matter volume between subjects, we included estimates of a voxel’s likelihood of containing grey matter as a covariate (nuisance variable) in the analysis of the resting-state functional data using standard statistical techniques (Oakes et al. 2007). The purpose of this method is to isolate the functional changes component which cannot be attributed to anatomical difference and is thus likely due to genuine functional differences. Firstly, voxel-based morphometry (VBM) was used to explore grey matter volume maps of every subject. These maps were transformed into the same standard space as the resting-state fMRI images using affine linear registration. As VBM results can be sensitive to the size of the smoothing kernel used to smooth the tissue segment images, the criterion used in this work was to match the smoothness of the grey matter volume map data to that of the corresponding functional data (8 mm). Finally, the resulting voxelwise grey matter volume maps were input as covariates in the analysis of functional data. The voxelwise-based grey matter volume correction was used for each subject. Note that there were no anatomical images for one MCI participant.
Statistical analysis
Mass univariate modelling
A procedure similar to that used in previous studies was applied to the present study (Inkster et al. 2009, 2010). In brief, a general linear model was used to analyse MCI-by-genotype interactions in the ALFF data at each SNP. Groups × genotypes ANOVA was recruited in the present study (groups: MCI and controls; genotypes: 2- or 3-level covariate for genotype status). Briefly, we used a genotypic model parameterised with orthogonal polynomials (and 2 degrees of freedom) for SNPs among the 10 % of participants who showed this rare genotype (i.e., \({\text{MAF}} > \sqrt {0.10} = \;0. 3 1\)). For SNPs with MAF < 0.31, a recessive model that merged the rare homozygous and heterozygous groups was used. In addition, because indisputable genetic neuroimaging data have identified APOE ε4 as a risk allele in AD and showed that patients with this allele present with abnormal resting-state brain function. In detail, MCI group included 13 APOE-ε4 carriers (9 subjects were single ε4 carriers; 4 subjects were double ε4–ε4 carriers) and 30 no APOE-ε4 carriers (including 26 subjects ε3–ε3 carriers and 4 subjects ε2–ε3 carriers), and controls group included 1 subject ε4–ε3 carriers and 29 subjects ε3–ε3 carriers. To control for possible brain functional differences that may be explained by differences of samples, the present study included APOE genotypes, age, gender and education as covariates in the following fMRI analysis. ANOVA statistical thresholds were set at an AlphaSim-corrected P < 0.05 as determined by Monte Carlo simulation (single voxel P value = 0.005, a minimum cluster size of 1,296 mm3, and FWHM = 8 mm with mask, see the AlphaSim program by D. Ward at http://afni.nimh.nih.gov/pub/dist/doc/manual/AlphaSim.pdf).
Cluster-based inference
Non-stationary cluster-size inference (Inkster et al. 2009, 2010) has been used to test for associations between individual SNPs and brain variations while correcting for searching across the entire brain imaging space using a minimum non-stationary AlphaSim-corrected P value as a summary measure to reflect the ALFF of any one SNP within each gene [denoted as P corrected(S) < 0.05]. For cases in which multiple SNPs related to the same gene could be tested, we selected the SNP that had the lowest (significant) P value for final analysis after applying a Bonferroni correction based on the number of SNPs tested for that gene. Thus, the final measure of significance for each gene included corrections for multiple comparisons both within and between genes [denoted as P corrected(S, G) < 0.05].
Network construction and behavioural significance
MCI-by-genotype associations with ALFF differences remained significant after correcting for imaging space for any one SNP [P corrected(S)] and across multiple SNPs per gene [P corrected(S, G)], including GSK3β (rs12630592), LRP6 (rs2302685), CDK5 (rs2069442), CDC2 (rs7919724) and CAMK2G (rs2242255) (also see Results section). To simplify the analysis, only the 11 clusters associated with the highest peak F value in every abovementioned SNPs were extracted as regions of interest (ROIs), including right anterior cingulum (MNI: 12 33 9), bilateral cerebellum posterior lobe (MNI: 0 −57 −45), left lentiform nucleus/putamen/insula (MNI:−18 0 12), right cerebellum anterior lobe/bilateral brainstem (MNI:−3 −36 −30), and bilateral cerebellum posterior Lobe (MNI: 18 −72 −24) (for details, see Table 3). These 11 cluster masks (ROIs) were extracted using a semi-automated imaging analysis program (developed by Dr. Liao W). To evaluate the overall effects of these 11 ROIs as well as interaction with aMCI, these ROIs were marked out a network. In brief, the topological characteristics of the network merged above resulted in regions using the following steps: (a) Using network node definitions, 11 ROIs were extracted based on their significance after correcting for imaging space for any one SNP [P corrected(S)] and across multiple SNPs per gene [P corrected(S, G)]. (b) Network edge definitions were used to individually extract averaged BOLD time from the 11 ROIs for each participant; then, we regressed the six head motion parameters, averaged the signals from the cerebrospinal fluid and white matter, and acquired a global brain signal (Fox et al. 2005). A Pearson’s correlation coefficient (after Fisher’s z-transform) was obtained for each of the 11 ROI time series pairs. Thus, we obtained an 11 × 11 matrix for each participant, and the weight of the edge between any two nodes represented the z-valued strength of the functional connectivity between the two corresponding brain regions. (c) We constructed a complete undirected weighted graph to model the topology of the network for each participant. The degree Si quantifies the extent to which a node is relevant to the network, and is defined as follows:
where w ij denotes the weighted edge that connects node i and node j. In other words, it is the z-value strength of the functional connectivity between brain region i and brain region j in the present study. (d) Finally, Pearson’s correlational analyses between S i and cognitive performance (i.e., episodic memory and non-episodic memory) were performed (P < 0.05).
Results
Participant characteristics
Compared with the controls, participants with MCI showed deficits in CDR, MMSE, episodic memory (i.e., AVLT-delayed recall and Rey-Osterrieth complex figure test-delayed recall) and non-episodic memory (i.e., trail-making test-A, trail-making test-B, the symbol digit modalities test, the clock-drawing test and the digit span test). Significant differences did not exist between these groups with regard to performance on the digit span test (Table 2). In addition, the genotype frequencies did not deviate from the Hardy–Weinberg equilibrium in either group (P > 0.05).
Tau protein pathway genes
Within genes
MCI-by-genotype associations with activation (ALFF) differences were identified for SNPs in 9/17 of the tau protein pathway SNPs after a minimum non-stationary AlphaSim-corrected P value for imaging space for any one SNP [P corrected(S)], including MAPT/STH (rs242557), GSK3β (rs12630592 and rs6438552), LRP6 (rs2284396 and rs2302685), CDK5 (rs2069442), CDC2 (rs7919724), CAMK2G (rs2242255) and DYRK1A (rs8126696) [which had P corrected(S) < 0.05]. The association clusters were widely found in frontal/parietal cortex, basal ganglia, brainstem and cerebellum (details see Table 3). It should be noted that there was no remained statistically significant after correction for imaging space between opposite statistical contrasts (controls <MCI).
Furthermore, this study confirmed the interactions between the SNP associations and brain activation associated with different tau protein pathway genes, showing different patterns among patients with MCI. For GSK3β, the regions associated with the rs12630592 and rs6438552 SNPs converged approximately at the right anterior cingulum cingulum/left precentral gyrus/left middle/superior frontal gyrus/right postcentral gyrus (Fig. 2a). In contrast, the rs2284396 and rs2302685 SNPs of LRP6 completely diverged at the left temporal lobe and the bilateral cerebellum posterior lobe/bilateral thalamus/left brainstem, respectively (Fig. 2b).
a GSK3β (rs12630592 and rs6438552) is associated with the MCI-by-genotype ALFF map. These SNPs demonstrated approximately converging regions in the right anterior cingulum/left precentral gyrus/left middle/superior frontal gyrus/right postcentral gyrus [P corrected(S) < 0.05]. b LRP6 (rs2284396 and rs2302685) is associated with the MCI-by-genotype ALFF map. These SNPs showed completely diverging regions in the left temporal lobe and the bilateral cerebellum posterior lobe/bilateral thalamus/left brainstem, respectively [P corrected(S) < 0.05]
Between genes
For cases in which multiple SNPs related to the same gene could be tested, we selected the SNP that had the lowest (significant) P value for final analysis after applying a Bonferroni correction based on the number of SNPs tested for that gene. Finally, 5/17 tau protein pathway SNPs remained significant after correcting for imaging space for any one SNP [P corrected(S)] and across multiple SNPs per gene [P corrected(S, G)], including GSK3β (rs12630592), LRP6 (rs2302685), CDK5 (rs2069442), CDC2 (rs7919724) and CAMK2G (rs2242255). This statistical approach was used to test the candidate genes of the tau protein pathway as all of these genes contribute to brain activation. In detail, these regions predominate along the cortico-cerebellar loops (i.e., the frontal-parietal-basal and ganglia-brainstem-cerebellum systems) were observed in patients with MCI, including the frontal superior/middle gyrus, the precentral/postcentral gyrus, anterior cingulum, lentiform nucleus, putamen, insula, brainstem, thalamus, and cerebellum posterior/anterior lobe (Table 3; Fig. 3a). None remained statistically significant after correction for imaging space and across multiple SNP per gene (P < 0.05) between opposite statistical contrasts (controls <MCI) in the present study.
Genes-brain-behaviour interactions
To simplify the analysis, the 11 clusters associated with highest peak F value in every abovementioned 5/17 SNPs were further extracted as ROIs, including right anterior cingulum (MNI:12 33 9), bilateral cerebellum posterior lobe (MNI:0 −57 −45), left lentiform nucleus/putamen/insula (MNI:−18 0 12), right cerebellum anterior lobe/bilateral brainstem (MNI:−3 −36 −30) and bilateral cerebellum posterior Lobe (MNI:18 −72 −24) (for details, see Table 3). To evaluate the overall effects of these abovementioned 11 ROIs as well as interaction with MCI, these ROIs were marked out a unidirectional weighted network with 11 nodes and 55 edges that globally described the network connectivity pattern for each participant. The characteristic network associations and behaviours demonstrated that the degree of correlated activity in right postcentral gyrus (R = −0.414, P = 0.006) and right cerebellum posterior lobe (R = −0.468, P = 0.002) were negatively correlated with non-episodic memory performance for all patients with MCI (Fig. 3b). No correlation was found between memory-related performance and network significance in the present study.
a The following genes remained significant after correcting for imaging space for any one SNP [P corrected(S)] and across multiple SNPs per gene [P corrected(S, G)]: GSK3β (rs12630592, red), LRP6 (rs2302685, blue), CDK5 (rs2069442, green), CDC2 (rs7919724, violet) and CAMK2G [rs2242255, yellow; P corrected(S, G) < 0.05]. These regions predominated in the cortico-cerebellar loops, including the frontal superior/middle gyrus, precentral/postcentral gyrus, anterior cingulum, lentiform nucleus, putamen, insula, brainstem, thalamus, and cerebellum posterior/anterior lobe. b The network characteristics and behavioural significance of the degrees of the correlated activity in right postcentral gyrus (R = −0.414, P = 0.006, blue) and right cerebellum posterior lobe (R = −0.468, P = 0.002, yellow) were negatively correlated with non-episodic memory performance in all patients with MCI
Discussion
This present study revealed that the topological alterations of brain activation were associated with tau protein pathway genes in patients with MCI. The two major findings are as follows: (a) impaired regional activation is related to tau protein pathway genes in patients with MCI and likely in topologically convergent and divergent functional alterations patterns within genes; and (b) tau protein pathway genes disrupt the topological architecture of cortico-cerebellar loops. This study was the first to extend our understanding of the tau pathway neuroimaging framework, which increases the information regarding combined genetics and imaging datasets among patients with MCI.
Topologically convergent and divergent functional alterations patterns
An increasing number of independent genetic association studies have found tau protein pathway genes in patients with AD and MCI disorders (Pei et al. 2002; Shelton and Johnson 2004; Yamamoto et al. 2005; Ryoo et al. 2007; Hooper et al. 2008; Small and Duff 2008; Chen et al. 2010). However, little is known regarding the detailed neuroimaging patterns related to these genes. The hyperactivity of GSK3β expressed in neurons causes cognitive impairments, tau hyperphosphorylation, increased β-amyloid production, neuronal death and neuroinflammation (Hooper et al. 2008). Furthermore, this gene is a potential target for the effective treatment of all AD clinical symptoms (Martinez et al. 2011). Kwok et al. (2005) reported that rs6438552 of GSK3β might regulate the selection of splice acceptor sites and enhance tau phosphorylation in patients with Parkinson’s disease. The present imaging genetic study showed that variations in the activation regions of the frontal-parietal lobe are associated with a common intronic polymorphism (rs6438552) at the GSK3β locus in patients with MCI, and a similar association between genotype and local region activation was also detected for rs12630592, which is 136,432 kb away from rs6438552. These findings were highly consistent with rs6438552, which is in high linkage disequilibrium with rs12630592 (i.e., r 2 = 0.95; the C allele of rs6438552 corresponds to the a allele of rs12630592, which has been found in Caucasian individuals’ HapMap data). Interestingly, our previous study observed significant resting-state differences for the GSK3β rs334558 polymorphism, while in the present study no significant changes are found (Bai et al. 2012). Previous study only focused on single SNP (GSK-3β rs334558), therefore, two factors are the possible reasons. One is associated with the method of statistical analysis (i.e., uncorrected for other SNPs vs. corrected for other SNPs), and another is a single SNP-based approach might coherently account for the diversity and lack of independent replications of these associations (i.e., one SNP-based on approach vs. pathway-based approach).
In addition, different alterations of brain regions were observed within the SNPs of other tau protein pathway genes among MCI populations. The LRP family is of high interest due to its key function in cholesterol/APOE uptake (Jaeger and Pietrzik 2008), which links APOE and tau phosphorylation given that APOE binds to cell-surface LDL receptor-related proteins (Small and Duff 2008). Recently, several studies have suggested that GSK3β promotes beta-catenin phosphorylation and degradation, as well as mediates the phosphorylation and activation of LRP6 (Zeng et al. 2005). In contrast to the convergent alterations of GSK3β (rs12630592 and rs6438552) and LRP6 (rs2284396 and rs2302685), however, related brain activation showed completely divergent patterns between the SNPs in the present sample of patients with MCI. Importantly, no linkage disequilibrium existed between rs2284396 and rs2302685, although they were slightly correlated (r 2 = 0.43 from HapMap data). Therefore, we infer that the linkage disequilibrium of the SNPs in the tau protein pathway genes contributes to the underlying target brain patterns in patients with MCI.
Cortico-cerebellar loops associated with tau protein pathways
This study was the first to identify the disrupted cortico-cerebellar loops associated with tau protein pathway genes in patients with MCI. Although none remained statistically significant between opposite statistical contrasts (controls <MCI) in the present study, neural hyperactivation that have been highlighted in the recent studies. For example, some regions with early hyperfunction could be due to compensation for other impairments (Clément and Belleville 2010), indeed neural hyperactivation was also closely associated with synaptic integrity. Bero et al. (2011) suggested that excess activity in several brain regions could be amyloidogenic processing through that neuronal activity increases Aβ production and secretion into interstitial fluid. Namely, increased activity at early stage of disease may be a pathological process that contributes to generating plaques, which then creates a pathological load in the same regions that subsequently causes a rapid decline in connectivity in these regions. This was also consistent with the threshold model of AD (Mortimer 1997).
The cortico-cerebellar loop projections originated throughout the cerebral cortex. In addition, they originated from the dentate nucleus of the cerebellum to the prefrontal and posterior parietal cortices via the brainstem and thalamus (He et al. 2004; Allen et al. 2005). These findings hold promise for understanding the underlying mechanisms of the disease-related increases in the pathological alterations (which may be attributable to functional disruptions in the cortico-cerebellar loops). Interestingly, a recent review has indicated that tauopathy of the AD process begins in the brainstem decades before the appearance of the first clinical AD symptoms (Braak and Del Tredici 2012). Another study showed a significant shortening of rapid eye movement (REM) sleep in MCI subjects relative to healthy elders (Hita-Yañez et al. 2012), while REM is strongly regulated by neuronal populations in the brainstem (Diniz Behn and Booth 2012). The present study observed that the brainstem and other areas comprised a network of regions significantly associated with tau protein pathway genes in MCI subjects. It could be a better understanding of the role of the brainstem in early neurodegeneration.
Furthermore, the present study demonstrated that the network characteristics (i.e., the degree of network) of the cortico-cerebellar loops were strongly negatively correlated with non-memory cognitive performance in patients with MCI. In detail, non-episodic memory tests of the present study includes trail-making test-A/B (assessment of visual attention and task switching), symbol digit modalities test (assessment of executive function), clock-drawing test (assessment of executive function and psychomotor speed), and the digit span test (assessment of memory span). This observation is consistent with the psychological functions of cortico-cerebellar loops, which include attention, psychomotor speed, executive function and visuospatial skills (Habas et al. 2009). Thus, patients with MCI might be able to alleviate or arrest cognitive decline through the increasing use of brain resources. The associations between networks and behavioural significance also implicate direct (or indirect) interactions among tau protein pathway genes. It should be noted that MCI can be sub-divided into two sub-types (Petersen et al. 1999; Winblad et al. 2004), including MCI-single domain that is assumed if the impairment involves only memory domain and MCI-multiple domain that pertains to impairments in the memory domain plus at least one other cognitive domain. Although the present study mainly recruited MCI-multiple domain patients, these findings only demonstrated that the network characteristics were strongly negatively correlated with non-memory cognitive performance. The lack of correlation with memory-associated tests may suggest that tau protein pathway genes do not play a significant role in the initial stages of memory impairment. We could assume that significance between the cortico-cerebellar loops and memory may become more apparent as disease changes worsen.
Methodological issues
Several issues should be addressed. First, brain function might be influenced by additional pathway variants that have not been studied with regard to other AD hypotheses, such as the amyloid cascade, cholinergic and cholesterol metabolism hypotheses. Future studies that focus on more complex pathways are necessary and important to provide a comprehensive picture of the topological alterations of brain networks in patients. Second, the present 17 selected SNPs were previously suggested to be tagging SNPs based on the tau protein hypothesis of AD, the Alzgene website and HapMap. This common, operational definition of a pathway was biased by the information obtained at the time of the analysis (Inkster et al. 2010). A larger sample size (i.e., the number of genes or SNPs) is needed to expand upon these preliminary findings. Third, a considerable amount of clinical and biological heterogeneity existed in the present sample of MCI participants whose recruitment was based on clinical criteria only. Certain participants may not have displayed the underlying AD pathology; thus, the sample was “contaminated” with non-AD cases. This limitation could be overcome by adding biomarker information to better characterise the study groups. This addition would yield the current “MCI due to AD” diagnosis that was recently published in the revised diagnostic criteria for AD (Dubois et al. 2010). Finally, the present perform analyses with samples were not fully matched (not statistically, but one-to-one) in age and gender. Therefore, these data should be interpreted with caution.
In all, the disrupted cortico-cerebellar loops were associated with tau protein pathway genes in patients with MCI. Tau protein pathway-based imaging approaches might strengthen the credibility in imaging genetic associations and generate pathway frameworks that might provide powerful new insights into the neural mechanisms that underlie MCI.
References
Allen G, McColl R, Barnard H, Ringe WK, Fleckenstein J, Cullum CM (2005) Magnetic resonance imaging of cerebellar-prefrontal and cerebellarparietal functional connectivity. Neuroimage 28:39–48
Attems J, Thomas A, Jellinger K (2012) Correlations between cortical and subcortical tau pathology. Neuropathol Appl Neurobiol 38:582–590
Bai F, Shi Y, Yuan Y, Yue C, Zhuang L, Xu X, Liu X, Zhang Z (2012) Association of a GSK-3β polymorphism with brain resting-state function in amnestic-type mild cognitive impairment. J Alzheimers Dis 32:387–396
Bero AW, Yan P, Roh JH, Cirrito JR, Stewart FR, Raichle ME, Lee JM, Holtzman DM (2011) Neuronal activity regulates the regional vulnerability to amyloid-β deposition. Nat Neurosci 14:750–756
Bing X, Ming-Guo Q, Ye Z, Jing-Na Z, Min L, Han C, Yu Z, Jia-Jia Z, Jian W, Wei C, Han-Jian D, Shao-Xiang Z (2012) Alterations in the cortical thickness and the amplitude of low-frequency fluctuation in patients with post-traumatic stress disorder. Brain Res 1490:225–232
Braak H, Braak E (1991) Neuropathological stageing of Alzheimer-related changes. Acta Neuropathol 82:239–259
Braak H, Del Tredici K (2012) Where, when, and in what form does sporadic Alzheimer’s disease begin? Curr Opin Neurol 25:708–714
Chen S, Townsend K, Goldberg TE, Davies P, Conejero-Goldberg C (2010) MAPT isoforms: differential transcriptional profiles related to 3R and 4R splice variants. J Alzheimers Dis 22:1313–1329
Clément F, Belleville S (2010) Compensation and disease severity on the memory-related activations in mild cognitive impairment. Biol Psychiatry 68:894–902
Delacourte A, David JP, Sergeant N, Buée L, Wattez A, Vermersch P, Ghozali F, Fallet-Bianco C, Pasquier F, Lebert F, Petit H, Di Menza C (1999) The biochemical pathway of neurofibrillary degeneration in aging and Alzheimer’s disease. Neurology 52:1158–1165
Diniz Behn C, Booth V (2012) A fast-slow analysis of the dynamics of REM sleep. SIAM J Appl Dyn System 11(1):212–242
Dubois B, Feldman HH, Jacova C, Cummings JL, Dekosky ST, Barberger-Gateau P, Delacourte A, Frisoni G, Fox NC, Galasko D, Gauthier S, Hampel H, Jicha GA, Meguro K, O’Brien J, Pasquier F, Robert P, Rossor M, Salloway S, Sarazin M, de Souza LC, Stern Y, Visser PJ, Scheltens P (2010) Revising the definition of Alzheimer’s disease: a new lexicon. Lancet Neurol 9:1118–1127
Dugger BN, Tu M, Murray ME, Dickson DW (2011) Disease specificity and pathologic progression of tau pathology in brainstem nuclei of Alzheimer’s disease and progressive supranuclear palsy. Neurosci Lett 491:122–126
Fox MD, Snyder AZ, Vincent JL, Corbetta M, Van Essen DC, Raichle ME (2005) The human brain is intrinsically organized into dynamic, anticorrelated functional networks. Proc Natl Acad Sci USA 102:9673–9678
Frank G, Hennig-Fast K, Klünemann HH, Schmitz G, Greenlee MW (2011) Differential impact of ApoE ε4 on cortical activation during famous face recognition in cognitively intact individuals and patients with amnestic mild cognitive impairment. Alzheimer Dis Assoc Disord 25:250–261
Georgiou-Karistianis N, Akhlaghi H, Corben LA, Delatycki MB, Storey E, Bradshaw JL, Egan GF (2012) Decreased functional brain activation in Friedreich ataxia using the Simon effect task. Brain Cogn 79:200–208
Gomez-Beldarrain M, Garcia-Monco JC (1998) The cerebellar cognitive affective syndrome. Brain 121:2202–2205
Grundke-Iqbal I, Iqbal K, Tung YC, Quinlan M, Wisniewski HM, Binder LI (1986) Abnormal phosphorylation of the microtubule-associated protein tau (tau) in Alzheimer cytoskeletal pathology. Proc Natl Acad Sci USA 83:4913–4917
Guadagna S, Esiri MM, Williams RJ, Francis PT (2012) Tau phosphorylation in human brain: relationship to behavioral disturbance in dementia. Neurobiol Aging 33:2798–2806
Habas C, Kamdar N, Nguyen D, Prater K, Beckmann CF, Menon V, Greicius MD (2009) Distinct cerebellar contributions to intrinsic connectivity networks. J Neurosci 29:8586–8594
He Y, Zang Y, Jiang T, Liang M, Gong G (2004) Detecting functional connectivity of the cerebellum using low frequency fluctuations (LFFs). Lect Notes Comput Sci 3217:907–915
Hita-Yañez E, Atienza M, Gil-Neciga E, Cantero JL (2012) Disturbed sleep patterns in elders with mild cognitive impairment: the role of memory decline and ApoE ε4 genotype. Curr Alzheimer Res 9:290–297
Hooper C, Killick R, Lovestone S (2008) The GSK3 hypothesis of Alzheimer’s disease. J Neurochem 104:1433–1439
Inkster B, Nichols TE, Saemann PG, Auer DP, Holsboer F, Muglia P, Matthews PM (2009) Association of GSK3beta polymorphisms with brain structural changes in major depressive disorder. Arch Gen Psychiatry 66:721–728
Inkster B, Nichols TE, Saemann PG, Auer DP, Holsboer F, Muglia P, Matthews PM (2010) Pathway-based approaches to imaging genetics association studies: wnt signaling, GSK3beta substrates and major depression. Neuroimage 53:908–917
Jaeger S, Pietrzik CU (2008) Functional role of lipoprotein receptors in Alzheimer’s disease. Curr Alzheimer Res 5:15–25
Koch W, Teipel S, Mueller S, Benninghoff J, Wagner M, Bokde AL, Hampel H, Coates U, Reiser M, Meindl T (2012) Diagnostic power of default mode network resting state fMRI in the detection of Alzheimer’s disease. Neurobiol Aging 33:466–478
Kwok JB, Hallupp M, Loy CT, Chan DK, Woo J, Mellick GD, Buchanan DD, Silburn PA, Halliday GM, Schofield PR (2005) GSK3B polymorphisms alter transcription and splicing in Parkinson’s disease. Ann Neurol 58:829–839
Lancaster TM, Baird A, Wolf C, Jackson MC, Johnston SJ, Donev R, Thome J, Linden DE (2011) Neural hyperactivation in carriers of the Alzheimer’s risk variant on the clusterin gene. Eur Neuropsychopharmacol 21:880–884
Leinonen V, Koivisto AM, Savolainen S, Rummukainen J, Tamminen JN, Tillgren T, Vainikka S, Pyykkö OT, Mölsä J, Fraunberg M, Pirttilä T, Jääskeläinen JE, Soininen H, Rinne J, Alafuzoff I (2010) Amyloid and tau proteins in cortical brain biopsy and Alzheimer’s disease. Ann Neurol 68:446–453
Liu X, Yue C, Xu Z, Shu H, Pu M, Yu H, Shi Y, Zhuang L, Xu X, Zhang Z (2012) Association study of candidate gene polymorphisms with amnestic mild cognitive impairment in a Chinese population. PLoS One 7:e41198
Long D, Wang J, Xuan M, Gu Q, Xu X, Kong D, Zhang M (2012) Automatic classification of early Parkinson’s disease with multi-modal MR imaging. PLoS One 7:e47714
Maccioni RB, Farías G, Morales I, Navarrete L (2010) The revitalized tau hypothesis on Alzheimer’s disease. Arch Med Res 41:226–231
Marcus JN, Schachter J (2011) Targeting post-translational modifications on tau as a therapeutic strategy for Alzheimer’s disease. J Neurogenet 25:127–133
Martinez A, Gil C, Perez DI (2011) Glycogen synthase kinase 3 inhibitors in the next horizon for Alzheimer’s disease treatment. Int J Alzheimers Dis 2011:280502
Mortimer JA (1997) Brain reserve and the clinical expression of Alzheimer’s disease. Geriatrics 52(Suppl 2):S50–S53
Oakes TR, Fox AS, Johnstone T, Chung MK, Kalin N, Davidson RJ (2007) Integrating VBM into the General Linear Model with voxelwise anatomical covariates. Neuroimage 34:500–508
Pei JJ, Braak H, Gong CX, Grundke-Iqbal I, Iqbal K, Winblad B, Cowburn RF (2002) Up-regulation of cell division cycle (cdc) 2 kinase in neurons with early stage Alzheimer’s disease neurofibrillary degeneration. Acta Neuropathol 104:369–376
Petersen RC, Negash S (2008) Mild cognitive impairment: an overview. CNS Spectr 13:45–53
Petersen RC, Smith GE, Waring SC, Ivnik RJ, Tangalos EG, Kokmen E (1999) Mild cognitive impairment, clinical characterization and outcome. Arch Neurol 56:303–308
Ryoo SR, Jeong HK, Radnaabazar C, Yoo JJ, Cho HJ, Lee HW, Kim IS, Cheon YH, Ahn YS, Chung SH, Song WJ (2007) DYRK1A-mediated hyperphosphorylation of Tau. A functional link between Down syndrome and Alzheimer disease. J Biol Chem 282:34850–34857
Schuff N, Woerner N, Boreta L, Kornfield T, Shaw LM, Trojanowski JQ, Thompson PM, Jack CR Jr, Weiner MW, Alzheimer’s Disease Neuroimaging Initiative (2009) MRI of hippocampal volume loss in early Alzheimer’s disease in relation to ApoE genotype and biomarkers. Brain 132:1067–1077
Sepulveda-Falla D, Matschke J, Bernreuther C, Hagel C, Puig B, Villegas A, Garcia G, Zea J, Gomez-Mancilla B, Ferrer I, Lopera F, Glatzel M (2011) Deposition of hyperphosphorylated tau in cerebellum of PS1 E280A Alzheimer’s disease. Brain Pathol 21:452–463
Sheline YI, Morris JC, Snyder AZ, Price JL, Yan Z, D’Angelo G, Liu C, Dixit S, Benzinger T, Fagan A, Goate A, Mintun MA (2010) APOE4 allele disrupts resting state fMRI connectivity in the absence of amyloid plaques or decreased CSF Aβ42. J Neurosci 30:17035–17040
Shelton SB, Johnson GV (2004) Cyclin-dependent kinase-5 in neurodegeneration. J Neurochem 88:1313–1326
Small SA, Duff K (2008) Linking Abeta and tau in late-onset Alzheimer’s disease: a dual pathway hypothesis. Neuron 60:534–542
Sperling R (2011) The potential of functional MRI as a biomarker in early Alzheimer’s disease. Neurobiol Aging 32:S37–S43
Tolnay M, Probst A (1999) Review: tau protein pathology in Alzheimer’s disease and related disorders. Neuropathol Appl Neurobiol 25:171–187
Trachtenberg AJ, Filippini N, Ebmeier KP, Smith SM, Karpe F, Mackay CE (2012) The effects of APOE on the functional architecture of the resting brain. Neuroimage 59:565–572
Vemuri P, Wiste HJ, Weigand SD, Knopman DS, Trojanowski JQ, Shaw LM, Bernstein MA, Aisen PS, Weiner M, Petersen RC, Jack CR Jr, Alzheimer’s Disease Neuroimaging Initiative (2010) Serial MRI and CSF biomarkers in normal aging, MCI, and AD. Neurology 75:143–151
Wang J, Grundke-Iqbal I, Iqbal K (2007) Kinases and phosphatases and tau sites involved in Alzheimer neurofibrillary degeneration. Eur J Neurosci 25:59–68
Wang Z, Yan C, Zhao C, Qi Z, Zhou W, Lu J, He Y, Li K (2011) Spatial patterns of intrinsic brain activity in mild cognitive impairment and Alzheimer’s disease: a resting-state functional MRI study. Hum Brain Mapp 32:1720–1740
Westlye ET, Lundervold A, Rootwelt H, Lundervold AJ, Westlye LT (2011) Increased hippocampal default mode synchronization during rest in middle-aged and elderly APOE ε4 carriers: relationships with memory performance. J Neurosci 31:7775–7783
Williamson J, Goldman J, Marder KS (2009) Genetic aspects of Alzheimer disease. Neurologist 15:80–86
Winblad B, Palmer K, Kivipelto M, Jelic V, Fratiglioni L, Wahlund LO, Nordberg A, Bäckman L, Albert M, Almkvist O, Arai H, Basun H, Blennow K, de Leon M, DeCarli C, Erkinjuntti T, Giacobini E, Graff C, Hardy J, Jack C, Jorm A, Ritchie K, van Duijn C, Visser P, Petersen RC (2004) Mild cognitive impairment-beyond controversies, towards a consensus: report of the international working group on mild cognitive impairment. J Intern Med 256:240–246
Yamamoto H, Hiragami Y, Murayama M, Ishizuka K, Kawahara M, Takashima A (2005) Phosphorylation of tau at serine 416 by Ca2+/calmodulin-dependent protein kinase II in neuronal soma in brain. J Neurochem 94:1438–1447
Yu R, Chien YL, Wang HL, Liu CM, Liu CC, Hwang TJ, Hsieh MH, Hwu HG, Tseng WY (2014) Frequency-specific alternations in the amplitude of low-frequency fluctuations in schizophrenia. Hum Brain Mapp 35:627–637
Zang YF, He Y, Zhu CZ, Cao QJ, Sui MQ, Liang M, Tian LX, Jiang TZ, Wang YF (2007) Altered baseline brain activity in children with ADHD revealed by resting-state functional MRI. Brain Dev 29:83–91
Zeng X, Tamai K, Doble B, Li S, Huang H, Habas R, Okamura H, Woodgett J, He X (2005) A dual-kinase mechanism for Wnt co-receptor phosphorylation and activation. Nature 438:873–877
Zhang Z, Deng L, Bai F, Shi Y, Yu H, Yuan Y, Wang K, Jiang T, Jia J, Zhang Z (2010) Alteration of resting brain function by genetic variation in angiotensin converting enzyme in amnestic-type mild cognitive impairment of Chinese Han. Behav Brain Res 208:619–625
Acknowledgments
Thanks to all participants. This research was partly supported by the National Natural Science Foundation of China (No. 81201080, 91332104); Program for New Century Excellent Talents in University (No. NCET-13-0117); Key Program for Clinical Medicine and Science and Tochnology: Jiangsu Provence Clinical Medical Research Center (No.BL2013025); Natural Science Foundation of Jiangsu Province (No. BK2012337) and Doctoral Fund of Ministry of Education of China (20120092120068). None of the authors have a conflict of interest, financial or otherwise, directly or indirectly related to this work.
Author information
Authors and Affiliations
Corresponding authors
Additional information
F. Bai and W. Liao contributed equally to this work.
Rights and permissions
About this article
Cite this article
Bai, F., Liao, W., Yue, C. et al. Genetics pathway-based imaging approaches in Chinese Han population with Alzheimer’s disease risk. Brain Struct Funct 221, 433–446 (2016). https://doi.org/10.1007/s00429-014-0916-4
Received:
Accepted:
Published:
Issue Date:
DOI: https://doi.org/10.1007/s00429-014-0916-4