Abstract
Based on numerical experiments using the NCAR CAM3-CLM3 models, this paper examines the impact of soil moisture, vegetation, and sea surface temperature (SST) on the inter-annual variability of climate over land. For each element, two experiments are carried out, with the inter-annual variability preserved in one experiment and eliminated in the other. Differences in the standard deviation of the precipitation and air temperature at the inter-annual time scale are used to quantify the impacts from soil moisture dynamics, vegetation dynamics, and oceanic forcing. The impact of oceanic forcing is mainly limited to the Tropics, with the strongest signal in the equatorial zone, and moisture convergence is the key linkage between SST forcing and tropical precipitation. Soil moisture plays a significant role in climate variability during the rainy seasons of all semi-arid regions (which is consistent with many previous studies), and during the dry seasons of the humid Amazon. Evapotranspiration is identified as the main mechanism linking precipitation variability to soil moisture. Amazon is the only region where vegetation dynamics has a significant influence on precipitation variability. However, the impact of vegetation dynamics on temperature is strong over the US Great Plains in all four seasons and in the Amazon region during the dry and dry-to-wet transition seasons.
Similar content being viewed by others
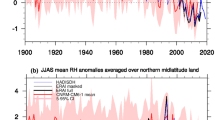
Avoid common mistakes on your manuscript.
1 Introduction
Predictions based on climate models indicate that the twenty-first century will experience an increase in the frequency and intensity of extreme events (e.g., Easterling et al. 2000; Meehl et al. 2000; Salinger 2005). Extreme events, including heat waves, heavy precipitation, and droughts, can have major devastating impacts on the human society. Studying the physical processes leading to these extreme events is therefore important both scientifically and in practice. Using a statistical model, Katz and Brown (1992) pointed out that the frequency of extreme events is more closely related to climate variability than the mean climate state.
Previous studies have pointed out that both terrestrial and oceanic forcings are important factors in regulating climate variability, based on both numerical experiments (e.g., Trenberth et al. 1998; Koster et al. 2000, 2006; Wang et al. 2007; Notar et al. 2011) and observation data analysis (e.g., Rasmusson and Carpenter 1982; Trenberth et al. 1998; Liu et al. 2006; Notaro et al. 2006; Alessandri and Navarra 2008). The oceanic impact is especially influential in the tropics (e.g., Koster et al. 2000). Meanwhile, terrestrial conditions, mostly represented by soil moisture and vegetation, influence the climate through modifying the radiative properties and fluxes of the land surface in both the tropics and extratropics (e.g., Notaro and Liu 2008; Notar et al. 2011; Wang et al. 2011). Both numerical experiments and statistical analysis have been used in studying land–atmosphere interactions. One advantage of statistical analysis lies in its wider applicability. That is, it is applicable to both model outputs and observational data. Several statistical methods, which are originally used in the study of ocean–atmosphere interaction, have recently been introduced into studies of land–atmosphere interaction. These methods include the Feedback Parameter method (Liu et al. 2006; Notaro et al. 2008; Notaro and Liu 2008; Zhang et al. 2008; Notaro 2008a, 2008b; Orlowsky and Seneviratne 2010), the Generalized Equilibrium Feedback Assessment (GEFA) method (Wang et al. 2012) and the Coupled Manifold method (Alessandri and Navarra 2008). In all these three methods, the impacts of vegetation/soil moisture in the climate system are primarily determined by the correlation between a terrestrial variable (e.g., soil moisture, vegetation) and an atmospheric variable (e.g., precipitation, air temperature). However, even a significant correlation does not guarantee a cause-and-effect relationship. Other factors, like the precipitation persistence, soil moisture memory and oceanic forcing, might synchronize the variations of both variables to arbitrarily increase their correlation (e.g., Wei et al. 2008; Zhang et al. 2008; Zeng et al. 2010; Sun and Wang 2012). Researchers who proposed new statistical methods to quantify land–atmosphere interactions are cautious about this possible overestimation. In order to alleviate this overestimation, further endeavors include deciphering the ‘black box’ of land–atmosphere interactions or categorizing different events according to a certain physics-based standard. The first type of studies focuses on breaking down the land–atmosphere interactive processes into two or more relatively independent steps (e.g., Dirmeyer 2006, 2011; Dirmeyer et al. 2009; Zeng et al. 2010; Santanello et al. 2011) and the second type of studies targets at grouping events based on the strength of the external oceanic forcing (e.g., Mei and Wang 2011; Mei and Wang 2012). Physical interpretations of results from statistical analysis are still challenging and can be better accomplished through a model framework, of which the physics are much better understood and the parameterization of physical processes can be manipulated to generate illustrative demonstrations of the interactive processes (e.g., Sun and Wang 2012).
Global Land–Atmosphere Coupling Experiment (GLACE) is an important community endeavor towards a better understanding of soil moisture-precipitation interactions (e.g., Koster et al. 2006; Guo et al. 2006; Seneviratne et al. 2006; Wang et al. 2007), and involves a large number of GCMs. For each GCM, the GLACE experiment includes two ensembles, one in which soil moisture interacts with precipitation and the other specifying a prescribed soil moisture. The strength of soil moisture-precipitation coupling is quantified using the inter-ensemble difference in intra-ensemble precipitation similarity (Koster et al. 2002, 2006). Wang et al. (2007) proposed an alternative index to quantify the land–atmosphere coupling strength, using the relative difference between the intra-ensemble precipitation variance of these two ensembles. The rationale is that the artificially reduced soil moisture variations in the ensemble with prescribed soil moisture are expected to decrease the precipitation variations in regions where soil moisture has strong control over precipitation. The changes of climate variability have been frequently used in climate studies as an index to quantify the impacts of different boundary conditions, for example, on precipitation and/or temperature (e.g., Koster et al. 2000; Dirmeyer 2001; Seneviratne et al. 2006).
Focusing on the inter-annual time scale, the current study examines how the terrestrial and the oceanic forcings influence the climate variability in all four seasons using the National Center for Atmospheric Research (NCAR) Community Atmosphere Model version 3 (CAM3) coupled with the Community Land Model version 3 (CLM3) (CAM3-CLM3). The idea is conceptually similar to GLACE index in that the inter-annual variability of one boundary condition for the atmosphere (soil moisture, vegetation, or sea surface temperature) is muted in one experiment compared with the control.
The rest of the paper is organized as follows. Section 2 presents the experimental design and methodology. Section 3 describes the climate impacts from soil moisture, sea surface temperatures (SSTs) and dynamic vegetation. Section 4 presents the conclusions and discussion.
2 Experimental design and methodology
The numerical model used in this study is the NCAR CAM3 coupled with CLM3 including the Dynamic Global Vegetation Model (DGVM) (CAM3-CLM3-DGVM). CAM3 simulates the atmospheric processes and provides atmospheric forcing (e.g., precipitation, radiation, specific humidity, wind) to the land model CLM3-DGVM; driven with the atmospheric forcing from CAM3, CLM3-DGVM simulates the land surface biogeophysical, physiological, biogeochemical processes and ecosystem dynamics, providing the surface fluxes (sensible heat flux, latent heat flux (or ET), and momentum flux) to CAM3 and updating the vegetation structure and distribution. For CAM3, the Eulerian spectral dynamical core is chosen with a T42 horizontal resolution (approximately 2.8° by 2.8°) and a total of 26 levels in the vertical direction. Details of the CAM3 model can be found in Collins et al. (2004). CAM3-CLM3 has been used extensively in studying the impacts of vegetation (e.g., Kim and Wang 2007b; Wang et al. 2011; Sun and Wang 2011), soil moisture (e.g., Koster et al. 2006; Kim and Wang 2007a; Wang et al. 2007; Notaro 2008a, b; Sun and Wang 2012) and SSTs (e.g., Fu et al. 2001) in the climate system.
Driven with the present day solar irradiance, the current study used the coupled land–atmosphere model CAM3-CLM3 in the five experiments, that is, DGVM_SST_Varying, NDGVM_SST_Varying, NDGVM_SST_Clim, Soilmr50P_SST_Varying and Soilmr50P_SST_Clim (Table 1). These five experiments allow three paired comparisons, each focusing on the contribution from interactive soil moisture (NDGVM_SST_Clim.vs. Soilmr50P_SST_Clim), dynamic vegetation (DGVM_SST_Varying.vs. NDGVM_SST_Varying) or oceanic forcing (Soilmr50P_SST_Varying.vs. Soilmr50P_SST_Clim).
The DGVM_SST_Varying experiment runs for 308 years, using the fully coupled model CAM3-CLM3 with dynamic vegetation model DGVM. The first 200 years of the simulation, with oceanic forcing cycling through the 1901-2000 observed SST (Hurrell et al. 2008), allows the global vegetation distribution to reach a quasi-equilibrium state. The last 108 years of the simulation, driven with the observed global SST during the period 1901–2008, models the soil moisture-precipitation interactions under the most realistic oceanic forcing. Branched from the 200th year of the DGMV_SST_Varying experiment, the NDGVM_SST_Varying experiment continues for another 108 years, with all the same experimental settings as the last 108 years of the DGVM_SST_Varying experiment except that vegetation distribution is kept static from year to year. These two experiments have been used to explore how vegetation dynamics enhances the multi-decadal variability of precipitation in the Amazon region (Wang et al. 2011). The focus here is on how the dynamic vegetation will influence the variations of the precipitation and air temperature with the SSTs acting as the external forcing.
The NDGVM_SST_Clim simulation is 108-year long, driven with climatological SST forcing and static vegetation, and vegetation is kept static at the quasi-equilibrium state derived from the first 200 years of the DGVM_SST_Varying simulation. Here the NDGVM_SST_Clim experiment is used to simulate soil moisture-precipitation interactions free of the low-frequency impacts from SST inter-annual variations and vegetation dynamics (Sun and Wang 2012).
Branched from the end of the NDGVM_SST_Clim experiment, the coupled CAM3-CLM3 models continue running for another 20 years, driven with monthly varying climatological SST forcing and sharing the same static vegetation distribution (which is also derived from the quasi-equilibrium state at the end of the 200-year DGVM_SST_Varying simulation). In each grid cell, soil moisture is prescribed to be at its 50th percentile derived from soil moisture simulated by the DGVM_SST_Varying experiment. Soil moisture is a slow-varying variable and is only prescribed at the beginning of each day instead of at every time step. At the end of this 20-year experiment, two 50-year runs (Soilmr50P_SST_Varying and Soilmr50P_SST_Clim) are branched separately, with soil moisture still being prescribed at its 50th percentile and vegetation cover being kept the same as in the NDGVM_SST_Clim experiments. The only difference between these two experiments is that climatological SST forcing is used in Soilmr50P_SST_Clim, and is replaced by the observed monthly SSTs (from 1951 to 2000) in the Soilmr50P_SST_Varying (Hurrell et al. 2008). The differences in the simulated climates are attributable to the impact of oceanic forcing. In consistency with referring to the SST forcing with climatological seasonal cycle as “climatological SST”, in the rest of this paper, “inter-annually varying SSTs” will be used to refer to the observed monthly SST forcing that include not only seasonal but also inter-annual variations.
The differences in the standard deviations of precipitation and 2-meter air temperature are used to represent the changes in climate variability. Climate variability differences between the Soilmr50P_SST_Varying and Soilmr50P_SST_Clim experiments demonstrate the influence from the inter-annually varying SST forcing free from the terrestrial influences, and differences in the climate variability between the NDGVM_SST_Clim and Soilmr50P_SST_Clim experiments represent the impacts of interactive soil moisture on the climate free from the low-frequency influences of the oceanic and vegetation impacts. Even though there are slight differences between the climatology of soil moisture from the NDGVM_SST_Clim experiment and the soil moisture specified in Soilmr50P_SST_Clim (at 50th percentile), these differences have negligible impact on the results of this study. Climate variability simulated by CAM3-CLM3 with prescribed soil moisture shows a very low sensitivity to the level of this prescribed soil moisture.
The difference between DGVM_SST_Varying and NDGVM_SST_Varying experiments shows the influence from the dynamic vegetation. Instead of prescribing soil moisture and using climatological SSTs forcing, both interactive soil moisture and inter-annually varying SSTs are kept in these two experiments. Prescribing soil moisture will reduce the vegetation dynamics, as the growth of vegetation is largely determined by the availability of soil moisture, especially in semi-arid regions/seasons where the vegetation growth is water-limited (e.g., Nemani et al. 2003; Anyamba and Tucker 2005; Barbosa et al. 2006). In the currently used CLM3-DGVM model, vegetation shows high sensitivity to water availability not only in the semi-arid regions but also in the humid tropics (Sun and Wang 2011). Prescribing soil moisture will greatly dampen the vegetation dynamics. Meanwhile, the land model is characteristic of high bare-ground evaporation and low sensitivity of evapotranspiration (ET) to vegetation cover changes. This causes the relatively low sensitivity of precipitation to vegetation cover changes when the climatological SSTs are used as its external forcing (Sun and Wang 2011). Here the observed monthly SST forcing (with both intra-annual and inter-annual variations) is applied in DGVM_SST_Varying and NDGVM_SST_Varying experiments.
In this study the difference in standard deviation between different experiments is used to quantify the impact of soil moisture feedback, vegetation feedback, and oceanic dynamics. Compared to using the ratio of standard deviation to quantify the impact of different processes following the approach of Koster et al. (2000), the results are similar in most regions. The difference in standard deviation is chosen over the ratio in this study to avoid the exaggeration of signals in places where the standard deviation being compared to is extremely small.
3 Terrestrial and oceanic impacts on climate variability
In this study, the standard deviation (SD) of precipitation and 2-m air temperature is used to represent the inter-annual climate variability. Here the inter-annual SDs are estimated based on seasonal averages of precipitation and temperature for each season respectively: JJA (June–July–August), SON (September–October-November), DJF (December–January–February) and MAM (March–April–May). It is important to note that due to the relatively short time scale of soil moisture dynamics, the impact of interactive soil moisture on climate variability at sub-seasonal time scale is likely to be stronger. In comparing the impact of three different factors (interactive soil moisture, vegetation dynamics, and SSTs), the focus in this paper is on the inter-annual time scale, a time scale that is relevant for all three factors.
3.1 The impacts of interactive soil moisture
From the Soilmr50P_SST_Clim experiment to the NDGVM_SST_Clim experiment, SD of precipitation increases, which is attributable to the soil moisture dynamics at the inter-annual time scale (Fig. 1). Values lower than the 90 % confidence levels based on the Fisher’s F-test are set to missing value. In JJA, the strongest increase occurs in North America (including Mesoamerica, southern Great Plains, Southeast and a large portion of the Northeast), South America (the northeastern border of the Amazon region), Africa (the Sahel and the northern part of the East Africa), and the northern part of Arabian Peninsula. During DJF season, regions of increased precipitation variations are located in the west and southern boundaries of the Amazon, the northern part of Patagonia and central area in Australia. For JJA and DJF seasons, regions of increased precipitation inter-annual variations correspond well with the GLACE-based hot spots of soil moisture-precipitation coupling in the same model identified by the ΔΦ index, which quantifies the precipitation variability caused by interactive soil moisture through comparing precipitation intraensemble relative variance (Wang et al. 2007). This does not come as a surprise, as both the ΔΦ index of Wang et al. (2007) and the increased SD here focus on the variations of precipitation time series. Discrepancies do exist, however, in the midlatitude Eurasia in JJA and a relatively large area in East Asia, where ΔΦ index demonstrates modest soil moisture-precipitation coupling at the synoptic time scale (Wang et al. 2007), while precipitation variability shows no detectable increase at the inter-annual time scale.
In the SON season, compared with the JJA, the strong increase of variability disappears in North America, Amazon north borders and the Sahel region. Instead, increase is found strong in the Amazon area which is in its dry-to-wet transition season. The least increase in precipitation variation occurs in MAM, with only scattered areas in the boundaries of the Amazon and areas close to the equator in the Africa demonstrating modest increase in precipitation variability.
In order to identify the linkage between soil moisture and precipitation variability, the differences in the variability of the ET (Fig. 2) and moisture convergence (MC, which is calculated as precipitation minus evapotranspiration since the changes of atmospheric storages are small at the monthly time scale) are compared between the NDGVM_SST_Clim experiment and the Soilmr50P_SST_Clim experiment. Compared with ET, MC shows negligible changes in SD. Regions of increased ET variability correspond well with those of precipitation variability, indicating that ET is the media linking the increased precipitation variability to soil moisture (e.g., Guo et al. 2006; Wang et al. 2007). Area of significant increase in ET variability is much larger than that of precipitation. That is because a successful passing of the ET perturbation to the precipitation still relies on some other critical physical processes which occur in both the boundary layer and the free atmosphere (e.g., Santanello et al. 2011).
Figure 3 shows the difference in the SD of the 2-m air temperature. Most regions showing significant increase are also regions of increased ET variability in Fig. 2, since ET influences the air temperature through its cooling effect. Discrepancies are found in the high latitudes, where the increase of temperature variability is not accompanied by significant increase in ET variability. Figure 4 shows how the ET and 2-m air temperature in four seasons are correlated at the inter-annual time scale in the NDGVM_SST_clim experiment. Compared with Figs. 2 and 3, regions showing increased variability in both ET and temperature are located in the areas where the ET and temperature are negatively correlated. Previous studies indicate that the negative correlation between ET and air temperature is attributable to the fact that soil moisture controls both ET and temperature, and ET cools the surface air (e.g., Notaro et al. 2006; Seneviratne et al. 2006). When soil moisture is prescribed in Soilmr50P_SST_Clim experiments, the negative correlation’s magnitude is mostly reduced and some even turns into positive (results not shown).
3.2 The impacts of dynamic vegetation
Figure 5 shows the increase in the inter-annual variability of precipitation from NDGVM_SST_Varying experiment to DGVM_SST_Varying experiment. Globally, Amazon region and some scattered areas in West Africa stands out as the only places where dynamic vegetation enhances inter-annual precipitation variability. The increase is mainly attributable to ET variation (Fig. 6), even though MC also shows an increase in variability in part of Amazon, especially during the SON and MAM season (not shown).
In addition to the Amazon region, areas of significant increase in temperature variations expand to the US Great Plains in four seasons (Fig. 7). In Amazon, the increase in temperature variability is attributable to the enhanced ET variations. In the US Great Plains, the enhanced temperature variability in SON is accompanied by a higher variability of net radiation. In the other three seasons, Bowen ratio, which represents the partition of radiation between the sensible and latent heat flux, demonstrates higher variability when the dynamic vegetation is included. The higher temperature variability could be caused by the increased Bowen ratio variability when the vegetation dynamics is included (e.g., Notar et al. 2011).
3.3 The impacts of the SSTs
Figure 8 shows the increase of precipitation variability in the Soilmr50P_SST_Varying experiment compared with the Soilmr50P_SST_Clim experiment, which is attributable to the oceanic impacts. When the inter-annual variations of SSTs are included, significant increase of precipitation variability are found only in several tropical regions well known for their climate sensitivity to oceanic forcings such as El Nino-Southern Oscillations (ENSO), including the northern and southeastern Amazon in DJF and MAM seasons (e.g., Fu et al. 2001), the Guinea Coast in JJA season (e.g., Goddard and Graham 1999; Giannini et al. 2003), the Indian Peninsula in JJA season (e.g., Cherchi et al. 2007), and in the Southeast Asia in all four seasons (e.g., Juneng and Tangang 2005).
In several extratropical regions known for climate sensitivity to ENSO (e.g., Pacific Northwest and U.S. Southeast), the signal for the increase of precipitation variability is weak and does not pass the significance test. However, the mean climate difference between the composites of El Nino years and La Nino years show very clear signal of ENSO impact consistent with the known pattern of ENSO impact in those regions. The lack of a strong signal for precipitation variability changes is partly related to the use of prescribed soil moisture here. Given the impact of soil moisture feedback on climate variability as shown in Sect. 3.1, interactive soil moisture is expected to further enhance the climate variability induced by oceanic forcing, an effect found in several previous studies (e.g., Schubert et al., 2004) and also supported by the comparison with the precipitation SD difference between the NDGVM_SST_Varying and NDGVM_SST_Clim experiments in this study (results not shown). What Fig. 8 presents is the impact of oceanic forcing in the absence of the amplification effect by soil moisture feedback.
Moisture convergence is the main contributor to the SST-induced increase in precipitation variability (Fig. 9). Globally, including the interannual variations of SSTs does not have large impacts on the variability of the ET and the 2-m air temperature (result not shown). In the deep tropics and outside of the dry seasons, ET is radiation-limited (e.g., Seneviratne et al. 2010; Sun and Wang 2012) and its variability is caused by the radiation variability (result not shown). Inter-annual variability of the oceanic forcing only causes small increase (lower than 0.5 K) in temperature variability in the Amazon, the Africa, the Indian Peninsula and the Southeast Asia.
4 Summary and discussion
This study targeted at quantifying the terrestrial and oceanic impacts on land precipitation and near-surface temperature at the inter-annual time scale in four seasons. In order to quantify the influence of each element, an experimental design similar to that of the GLACE studies was adopted here. For each element, two experiments are carried out, with the inter-annual variability preserved in one experiment and suppressed in the other. Focusing on climate variability at the inter-annual time scale, the differences in the SD of the precipitation and air temperature are used to demonstrate the impacts from soil moisture, vegetation cover, and SSTs.
Inter-annually, interactive soil moisture significantly increases the precipitation variability in the rainy seasons of the semi-arid regions and in the dry seasons of the humid Amazon, and ET is identified as the main linkage between soil moisture and precipitation variability. Inter-annually varying oceanic forcing causes the largest inter-annual precipitation variation in the deep tropics. Precipitation variability in extratropical regions, including those known for their climate sensitivity to ENSO, shows low sensitivity to oceanic forcing when soil moisture is prescribed, despite the response of model-simulated mean climate to ENSO in those regions. This indicates that interactive soil moisture plays a key role in enhancing the impact of oceanic forcing on climate variability in those regions. Moisture convergence is the linking factor between the SST forcing and the tropical precipitation. Amazon region is the only place where dynamic vegetation demonstrates significant influence on precipitation variability in four seasons.
In regions and seasons with strong increase of precipitation variability caused by interactive soil moisture, the variability of the 2-m air temperature is also enhanced by interactive soil moisture. In addition, in the semi-arid US Great Plains, US southeast and Patagonia, temperature in the transition seasons also demonstrates significantly increased variations attributable to soil moisture dynamics. Increased ET variability is identified as the cause of increased temperature variability. Dynamic vegetation is found to enhance temperature variations in the US Great Plains in all four seasons and in the Amazon region during the dry and dry-to-wet transition seasons. In the Amazon region, higher ET variability related to vegetation dynamics plays the main role. In the US Great Plains in SON, the increase in temperature variability is attributable to the enhanced variability of net radiation. The increased variations of Bowen ratio could be the cause of the increased temperature variability in JJA, DJF and MAM seasons.
Climate projections for the twenty-first century demonstrate increased climate variability attributable to the combined effects from global warming and oceanic influences (e.g., Salinger 2005), which will also trigger increased variations over land including extreme soil moisture conditions and vegetation fluctuations. Our results suggest that the resulting soil moisture and vegetation feedback could further reinforce the warming-triggered increase of climate variability, making it harder for crops and natural vegetation to survive. This has important implications on drought mechanisms, for example the 1930s “dust bowl” over the U.S. Great Plains. Another sensitive area is the Amazon, where warming- and drought-induced forest dieback (e.g., Cox et al. 2000) may increase the local climate variability thus further enhancing forest degradation.
The current study complements previous work of land–atmosphere coupling (e.g., Koster et al. 2006; Wang et al. 2007) by focusing on the impacts on inter-annual variability. Different than the GLACE experimental design, which focuses on sub-seasonal and seasonal time scales and needs a large number of ensemble experiments, only two continuous experiments are required here to study the impact of terrestrial or oceanic forcing. This approach can be conveniently applied to any model, and therefore may provide a useful tool for potential multi-model inter-comparison projects in the future.
References
Alessandri A, Navarra A (2008) On the coupling between vegetation and rainfall inter-annual anomalies: possible contributions to seasonal rainfall predictability over land areas. Geophys Res Lett 35:L02718. doi:10.1029/2007GL032415
Anyamba A, Tucker C (2005) Analysis of Sahelian vegetation dynamics using NOAA-AVHRR NDVI data from 1981–2003. J Arid Environ 63:596–614
Barbosa HA, Huete A, Baethgen W (2006) A 20-year study of NDVI variability over the Northeast Region of Brazil. J Arid Environ 67:288–307
Cherchi A, Gualdi S, Behera S et al (2007) The influence of Tropical Indian Ocean SST on the Indian summer monsoon. J Clim 20:3083–3105
Collins WD et al (2004) Description of the NCAR community atmosphere model 579 (CAM3.0). Tech Rep, NCAR/TN-464 + STR, Natl Cent for Atmos Res, Boulder, Colo
Cox PM, Betts RA, Jones CD, Spall SA, Totterdell IJ (2000) Acceleration of global warming due to carbon-cycle feedbacks in a coupled climate model. Nature 408:184–187
Dirmeyer PA (2001) An evaluation of the strength of land-atmosphere coupling. J Hydrometeorol 2:329–344
Dirmeyer PA (2006) The hydrologic feedback pathway for land-climate coupling. J Hydrometeorol 7:857–867
Dirmeyer PA (2011), The terrestrial segment of soil moisture-climate coupling. Geophys Res Lett 38. doi:10.1029/2011GL048268
Dirmeyer PA, Schlosser CA, Brubaker KL (2009) Precipitation, recycling, and land memory: an integrated analysis. J Hydrometeorol 10:278–288
Easterling DR, Meehl GA, Parmesan C, Changnon SA, Karl TA, Mearns LO (2000) Climate extremes: observations, modeling and impacts. Science 289:2068–2074
Fu R, Dickinson RE, Chen M, Wang H (2001) How do tropical sea surface temperatures influence the seasonal distribution of precipitation in the equatorial Amazon? J Clim 14:4003–4026
Giannini A, Saravanan R, Chang P (2003) Oceanic forcing of Sahel rainfall on interannual to interdecadal time scales. Science 302:1027–1030
Goddard L, Graham NE (1999) Importance of the Indian Ocean for simulating rainfall anomalies over eastern and southern Africa. J Geophys Res 104:19099–19116
Guo Z-C et al (2006) GLACE: the global land–atmosphere coupling experiment. Part II: analysis. J Hydrometeorol 7:611–625
Hurrell JW, Hack JJ, Shea D, Caron JM, Rosinski J (2008) A New Sea Surface Temperature and Sea Ice Boundary Dataset for the Community Atmosphere Model. J Clim 21:5145–5153
Juneng L, Tangang FT (2005) Evolution of ENSO-related rainfall anomalies in Southeast Asia region and its relationship with atmosphere–ocean variations in Indo-Pacific sector. Clim Dyn 25:337–350
Katz RW, Brown BG (1992) Extremee vents in a changing climate: variability is more important than averages. Clim Change 21:289–302
Kim YJ, Wang GL (2007a) Impact of initial soil moisture anomalies on subsequent precipitation over North America in the coupled land–atmosphere model CAM3-CLM3. J Hydrometeorol 8:534–550
Kim YJ, Wang GL (2007b) Impact of vegetation feedback on the response of precipitation to antecedent soil moisture anomalies over North America. J Hydrometeorol 8:534–550
Koster RD, Suarez MJ, Heiser M (2000) Variance and predictability of precipitation at seasonal to interannual timescales. J Hydrometeorol 1:26–46
Koster RD, Dirmeyer PA, Hahmann AN, Ijpelaar R, Tyahla L, Cox P, Suarez MJ (2002) Comparing the degree of land–atmosphere interaction in four atmospheric general circulation models. J Hydrometeorol 3:363–375
Koster RD et al (2006) GLACE: the global land–atmosphere coupling experiment. Part I: overview. J Hydrometeorol 7:590–610
Liu Z, Notaro M, Kutzbach J, Liu N (2006) Assessing global vegetation–climate feedbacks from observations, J. Climate 19:787–814
Meehl GA et al (2000) Trends in extreme weather and climate events: issues related to modeling extremes in projections of future climate change. Bull Am Meteorol Soc 81:427–436
Mei R, Wang G (2011) Impact of sea surface temperature and soil moisture on summer precipitation in the United States based on observational data. J Hydrometeorol 12:1086–1099. doi:10.1175/2011JHM1312.1
Mei R, Wang G (2012) Summer land-atmosphere coupling strength in the United States: comparison among observations, reanalysis data and numerical models. J Hydrometeorol in press
Nemani RR et al (2003) Climate-driven increases in global terrestrial net primary production from 1982 to 1999. Science 300:1560–1563
Notar M, Chen G, Liu Z (2011) Vegetation feedbacks to climate in the global monsoon regions. J Clim 24:5740–5756, doi: 10.1175/2011JCLI4237.1
Notaro M (2008a) Response of the mean global vegetation distribution to interannual climate variability. Clim Dynam 30:845–854
Notaro M (2008b) Statistical identification of global hot spots in soil moisture feedbacks among IPCC AR4 models. J Geophys Res 113:D09101. doi:10.1029/2007JD009199
Notaro M, Liu Z (2008) Statistical and dynamical assessment of vegetation feedbacks on climate over the boreal forest. Clim Dyn 31:691–712. doi:10.1007/s00382-00008-00368-00388
Notaro M, Liu Z, Williams JW (2006) Observed vegetation-climate feedbacks in the United States. J Clim 19:763–786. doi:10.1175/JCLI3657.1
Notaro M, Wang Y, Liu Z, Gallimore R, Levis S (2008) Combined statistical and dynamical assessment of simulated vegetation–rainfall interactions in North Africa during the mid-Holocene. Glob Chang Biol. doi:10.1111/j.1365-2486.2007.01495.x
Orlowsky B, Seneviratne SI (2010) Statistical analyses of land-atmosphere feedbacks and their possible pitfalls. J Clim 23:3918–3932. doi:10.1175/2010JCLI3366.1
Rasmusson EM, Carpenter TH (1982) Variations in tropical sea surface temperature and surface wind fields associated with the Southern Oscillation/El Niño. Mon Wea Rev 110:354–384
Salinger MJ (2005) Climate variability and change: past, present, and future—an overview. Clim Change 70:9–27
Santanello JA, Peters-Lidard CD, Kumar SV (2011) Diagnosing the sensitivity of local land-atmosphere coupling via the soil moisture-boundary layer interaction. J Hydrometeorol 12:766. doi:10.1175/JHM-D-10-05014.1
Schubert D et al (2004) Causes of long-term drought in the US Great Plains. J Clim 17(3):485–503
Seneviratne SI, Lüthi D, Litschi M, Schär C (2006) Land–atmosphere coupling and climate change in Europe. Nature 443:205–209
Seneviratne SI, Corti T, Davin EI et al (2010) Investigating soil moisture-climate interactions in a changing climate: a review. Earth Sci Rev 99:125–161
Sun S, Wang GL (2011) Diagnosing the equilibrium state of a coupled global biosphere-atmosphere model. J Geophys Res. doi:10.1029/2010JD015224
Sun S, Wang GL (2012) The complexity of using a feedback parameter to quantify the soil moisture-precipitation relationship. J Geophys Res. doi:10.1029/2011JD017173
Trenberth KE, Branstator Karoly D, Kumar A, Lau N-C, Ropelewski C (1998) Progress during TOGA in understanding and modeling global teleconnections associated with tropical sea surface temperatures. J Geophys Res 103:14291–14324
Wang GL, Kim YJ, Wang D (2007) Quantifying the strength of soil moisture-precipitation coupling and its sensitivity to changes in surface water budget. J Hydrometeorol 8:551–570. doi:10.1175/JHM573.1
Wang GL, Sun S, Mei R (2011) Vegetation dynamics contributes to the multi-decadal variability of precipitation in the Amazon region. Geophys Res Lett 38:L19703. doi:10.1029/2011GL049017
Wang F, Notaro M, Liu Z (2012), Influence of vegetation on the climate across North America—observational and modeling study. J Clim, in preparation
Wei J, Dickinson RE, Chen H (2008) A negative Soil moisture–precipitation relationship and its causes. J Hydrometeorol 9:1364–1376
Zeng XB, Barlage M, Castro C, Fling K (2010) Comparison of land–precipitation coupling strength using observations and models. J Hydrometeorol 11:979–994. doi:10.1175/2010JHM1226.1
Zhang J, Wang WC, Wei J (2008) Assessing land-atmosphere coupling using soil moisture from the Global Land Data Assimilation System and observational precipitation. J Geophys Res 113:D17119. doi:10.1029/2008JD009807
Acknowledgments
This work was supported from funding from NSF (ATM 0531845) and NOAA (NA080AR4310871). The authors thank the three anonymous reviewers for their constructive comments.
Author information
Authors and Affiliations
Corresponding author
Rights and permissions
About this article
Cite this article
Sun, S., Wang, G. Climate variability attributable to terrestrial and oceanic forcing in the NCAR CAM3-CLM3 Models. Clim Dyn 42, 2067–2078 (2014). https://doi.org/10.1007/s00382-013-1913-7
Received:
Accepted:
Published:
Issue Date:
DOI: https://doi.org/10.1007/s00382-013-1913-7