Abstract
In this paper, a simple, effective drought monitoring method is developed using two dimensional spectral space obtained from reflectance of near-infrared (NIR) and Red wavelengths. First, NIR–Red reflectance space is established using atmospheric and geometric corrected ETM+ data, which is manifested by a triangle shape and in which different surface targets possess certain spatial distribution rules. Second, perpendicular drought index (PDI) is developed on the basis of spatial characteristics of moisture distribution in NIR–Red space, as well as the relationships between PDI and soil moisture is examined. Validation work includes: comparison of PDI with in-situ drought index obtained from field measured data in the study area which includes bulk soil moisture content at different soil depths, field moisture capacity and wilting coefficient, etc.; and comparison of PDI with other recognized drought monitoring methods such as LST/NDVI and vegetation temperature condition index (VTCI). It is evident from the results that graph of PDI of field measured plots demonstrates very similar trends with ground truth drought data, LST/NDVI and VTCI. PDI is highly correlated with in-situ drought values calculated from 0 to 20 cm mean soil moisture with correlation coefficients of R 2 = 0.49 (r = 0.75). This paper concludes that PDI has a potential in remote estimation of drought phenomenon as a simple, effective drought monitoring index.
Similar content being viewed by others
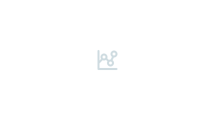
Avoid common mistakes on your manuscript.
Introduction
Drought may be described as a chronic, potential natural disaster characterized by prolonged and abnormal water shortage. During the last few decades, a good variety of drought monitoring models have been invented, for example, the Palmer drought severity index (PDSI) (Palmer 1965), the rainfall anomaly index (RAI) (van Rooy 1965), the crop moisture index (Palmer 1968), the Bhalme–Mooley index (BMDI) (Bhalme and Mooley 1980), the NOAA drought index (NDI) (Strommen et al. 1980), the surface water supply index (Shafer and Dezman 1982), the standardized anomaly index (Katz and Glantz 1986), the standardized precipitation index (SPI) (McKee et al. 1993), the normalized difference vegetation index (NDVI) based vegetation condition index (VCI) (Kogan 1995a) and temperature condition index (TCI) (Kogan 1995b). Su et al. (2003) summarized these methods into meteorological based indices (e.g., the standardized precipitation index), process based indices (e.g., evaporative fraction, EF), and satellite based indices (e.g., vegetation indices). Some of them are derived from climate factors and less relative to surface water characteristics and crop conditions while some only consider single surface factors like soil moisture content neglecting plant water demand, completely different results may be achieved from the same input parameters.
Drought could affect surface radiation, heat and water balance via changing surface bio-physical factors like NDVI, albedo and land surface temperature (LST). In general, with the development of drought, NDVI decreases, albedo and surface temperature increase, and soil moisture decreases provided that the other factors are stable. Combination of those parameters may provide useful methods for quantitative detection of spatial and temporal distribution of drought. LST and NDVI can provide the information on vegetation and surface moisture. Goward and Hope (1989) and Price (1990) developed LST–NDVI feature space. Since then, LST–NDVI spectral space has been widely used in land surface classification, soil moisture monitoring, estimation of live fuel moisture content and surface energy fluxes (Carlson and Sanchez-Azofeifa 1999; Carlson and Arthur 2000; Chuvieco et al. 2004, Gillies and Carlson 1995; Gillies et al. 1997; Goetz 1997; Han et al. 2005; Lambin and Rhrlich 1996; Nemani and Running 1989; Ridd 1995; Owen et al. 1998; Wan et al. 2004). Being calculated from whole shortwave reflectance data, surface broadband albedo is a more informative factor than any other indexes obtained by combination of limited band reflectance in the optical domain. Ghulam et al. (2004a) tried to substitute LST with albedo in LST–NDVI space, and explored the drought conditions using the slope of broadband albedo and NDVI scatter plot, and more recently developed vegetation condition albedo drought index (VCADI) (Ghulam 2006). However, as the location of a pixel in the LST–NDVI space is influenced by many factors, surface types may have different LST/NDVI slope and intercept for equal atmospheric and surface moisture conditions (Sandholt et al. 2002). In addition, different spatial resolution of visible, infrared and thermal band data of some sensors such as TM/ETM+, MODIS are not the same; more useful information is lost as a result of spectral sampling which should be carried out to construct spectral space using NDVI and LST products. Then, retrieval of surface albedo and LST contains uncertainties rooted from atmospheric correction of satellite data, decomposition of mixed pixel information, bi-directional reflectance distribution function (BRDF) modeling and spectral remedy by narrowband to broadband conversion (Liang 2003; Pokrovsky and Roujean 2002; Zhao et al. 2000). Therefore, the final error on extraction and quantifying of drought information would be magnified. Furthermore, NDVI, LST and albedo are after-effect indicators of drought and, out of interest when the focus must be the real time monitoring of drought conditions instead of hysteresis.
The main objective of this paper is to develop a new operational, drought monitoring method–perpendicular drought index (PDI), which is obtained from NIR–Red spectral reflectance space. PDI is validated with field obtained data and relationships between PDI and drought indexes such as LST–NDVI, albedo-NDVI, VTCI are explored.
Test site and data collection
Landsat Enhanced Thematic Mapper plus (ETM+) data registered on April 17, 2001 over Beijing, China is used in the paper. The Shun Yi remote sensing experiment field (E 116°26′–117°E, N 40°–40°21′) in Beijing, China was selected as the study area (Fig. 1). Four core test sites named as the central site (labeled C1–C5), the northwest site (labeled NW1–NW5), the northeast site (labeled NE1–NE5) and the southeast site (labeled SE1–SE5) were designed following a stratified sampling scheme covering different surface conditions. Each site was defined by five sampling fields corresponding to bare soil and croplands planted winter wheat with different drought and fertilization conditions, respectively. Soil moisture data from different soil depths of 5, 10, 20, 40, 60 and 100 cm were collected by both the CNC-503DR—intelligent water neutron meter and the weighing-after-drying method in over the fields NW1, NW2, NW3, NW4, NW5 the northwest test site, which corresponds with normal watered and fertilized bare soil, water stressed, over watered, fertilizer lacked and over fertilized croplands. A total of 25 ground measuring plots were deployed over those fields; 16 plots were in fields of NW1, NW2, NW3 and 9 in NW4, NW5 during the time the satellite made its overpass on April 17, 2001. In the weighing-after-drying method, soil moisture content (%) is calculated by dividing the water content of a 1,000 gm sample of earth by its dried earth counterpart and then multiplying by 100.
Method
Fundamental theory and spectral features of the NIR–Red spectral space can be found in the earlier work of Richardson and Wiegand (1977) and recent reports of Zhan et al. (2006) and Ghulam (2006). Vegetation lamina tissues strongly absorb incident radiances in blue, purple and red wavelengths and intensively reflect the near infrared (NIR) spectrum. The thicker the vegetation density, the smaller the reflectance in Red and the higher the reflectance in NIR bands become. Because the absorption of the Red range is saturated quickly, only the increase of reflectance in the NIR region could reflect the increase of vegetation. Then, from Red to NIR spectral region, the reflectance of bare soil is high but increases slowly. However, due to the strongest absorption by water, bare soil reflectance decreases distinctly with the increasing of soil moisture especially in the near infrared domain. Therefore, any mathematical operation which could strengthen the difference between NIR and Red could be used to describe the vegetation, surface drought status and discriminate the soil information from the vegetated pixel. Vegetation indices such as RVI, DVI, and NDVI, etc. are based on this theory.
Sub-image covering the Shun Yi district of Beijing, which has been the remote sensing experiment field of this project, was subset after geometric correction. Digital numbers (DNs) were converted into spectral radiance and top of the atmosphere (TOA) reflectance. Subsequently, atmospheric correction by the 6S code (Vermote et al. 1997) for visible and near infrared data and Modtran 4 for thermal infrared data were carried out to eliminate the atmospheric perturbation and obtain the reflectance and land surface temperature. Considering the spectral characteristics of surface targets and ETM+ spectral features, ETM+ band 3 (Red, 630–690 nm) and band 4 (NIR, 780–900 nm) were selected to construct the NIR–Red spectral space. The scatter plot of the atmospheric corrected NIR, Red reflectance spectrum demonstrated a typical triangle shape (Fig. 2), which was different from previously reported LST–NDVI spectral space. Different land cover types manifested certain regular distribution in the NIR–Red spectral space. Not only the vegetation coverage can be described, but also the surface drought severity can be characterized quantitatively in the space.
It can be seen from Fig. 2 that the distribution features of vegetation in the space is similar with what Richardson and Wiegand (1977) reported. Here, the AD line represents the change of surface vegetation from the full cover (A) and the partial cover (E) to bare soil (D) while BC refers soil moisture status for wet area (B), semi-arid surface to extremely drought surface (C). As can be seen, BC shows the direction of drought severity. There are close but complex relationships between the surface spectrum and land cover types and surface drought conditions. This encouraged the authors to build a NIR–Red spectral reflectance space based drought monitoring index which may be rather simple and effective compared to the LST–NDVI and albedo-NDVI space based methods in which retrieval of albedo and LST is quite expensive and problematic.
The soil line is made up of plots characterizing the spectral behavior of non-vegetated pixels and whose moisture varies obviously. It is not difficult to see from the Fig. 2 that the drought severity gradually rises from B to C, and reaches its climax at C. Here BC represents the soil line of the research area, supposing that the mathematical expression of the soil line can be expressed by the following equation.
Here, R s, red, R s, NIR refer to the atmospherically corrected reflectance of NIR band and red band, respectively, while M refers to the slope of the soil line, I is the interception on the vertical axis.
A line L, which disects the coordinate origin and is vertical to the soil line, can be delineated on Fig. 3. Therefore, as to the normal function of a line, L can be mathematically formulated from the soil line expression.
For bare soil, the distance from any points in the NIR–Red reflectance space to the line L represents the drought severity of the non-vegetated surface. With the increasing amount of vegetation, the plots shift upward along the direction vertical to the soil line while they do the same along the direction parallel to the soil line and orthogonal to normal line L with the increasing of the soil moisture. For a vegetated surface, the distance from L to any points in the NIR–Red spectral space may indicate the drought severity of a mixed pixel. That is, the farther the distance, the stronger the drought, and the less the soil moisture or vice versa. Thereby, it is possible to formulate the drought severity using the mathematical expression of the distance from point to line. Taking a random point, a pixel E (R red, R NIR ) in the NIR–Red reflectance space, the vertical distance from E (R red, R NIR ) to line L (PDI) can be written as the following.
With respect to a black body, the distance is the least amount which almost equals zero, and it is located just at the coordinate origin. In the case of other objects with some reflectance, the higher moisture content the target possesses, the nearer it is located to the coordinate origin. Generally speaking, objects placed near the line L are always bodies of water or are extremely wet regions and the drought value infinitely closes to 0, whereas in the most distant area from the line L in the space represents an extremely dry surface. In this case, drought value infinitely closes to 1.
M = 1.40426 and I = −0.0703 are determined from soil line equation which is extracted with atmospheric corrected NIR, Red reflectance of study area. Introducing M into formula (3), the final equation of PDI for this test site can be written as
Results and discussion
Validation of PDI
The comprehensive drought index (K) derived from in-situ measurements was used to validate PDI. K can be expressed as the following (Zhan et al. 1999).
Here, W (%) is effective soil moisture; W h (%) represents field moisture capacity; and W p refers wilting coefficient. When crops obtain enough water, no drought occurs, and here K = 0. If W = W p , effective soil moisture equals to zero, crops cannot absorb any water from the soil and tend to die away; this time K = 1. Thus, it can be seen that K represents well the field obtained drought data.
Then, what is the effective depth of satellite monitoring of soil moisture? Field measurement data from what depth should be taken into account for the calculation of K to compare with PDI? Ghulam et al. (2004b) believes that visible and near infrared spectral data have a close relationship with soil moisture at 10 cm soil depth. Using the in-situ data gathered over 25 control points, K 5, K 10, K 10, K 0–20 are obtained with soil moisture at 5, 10, 20 cm and mean values of 0–20 cm by Eq. 5. Thanks to high spatial resolution of ETM+ data, pixels representing ground control points can be easily identified via latitude and longitude of those plots. Therefore, PDI are calculated for every ground points with Eq. 4 using atmospheric corrected spectral reflectance. PDI, K were normalized between 0 and 1, because the value of K could be greater than 1 where there was some ground measured soil moisture greater than field moisture capacity. Comparison results as shown in Fig. 4 exhibit that PDI are highly accordant with K. Among them, correlations between PDI and K 0–20 are the strongest (R 2 = 0.49), next is K 10 and then the worst is K 5. As ground measurements of surface moisture content by intelligent water neutron meter (CNC-503DR), particularly at 0–5 cm soil depth, is affected by surface wind speed and other external conditions, it is normal to obtain relatively poor correlation between PDI and K 5 compared to those with K 10, K 20 and K 0–20.
Comparison of several drought indexes
With the occurrence of drought, surface vegetation coverage may be destroyed; in further effect, it will induce rising of surface albdeo, and heat flux. Ergo, drought process is not only denoted as deterioration of vegetation coverage and biomass but also is manifested as maladjustment of energy and water circulation as well as the variation of surface temperature and soil moisture. These variations can be directly reflected in LST–NDVI, albedo–NDVI spectral space. They are very often used in land cover classification, regional drought monitoring (Price 1990; Gillies and Carlson 1995; Su et al. 2003, Ghulam et al. 2004a; Wang et al. 2005). Here, a comparison between PDI and slope of LST–NDVI, albedo–NDVI and vegetation temperature condition index (VTCI) referenced from Wang et al. 2001 were conducted.
After geometric and atmospheric correction, LST is retrieved by ETM+ thermal band data using equation provided by Qin et al. (2001) and downscaled to 30 m resolution. Land surface broadband albedo is estimated using the method provided by Zhao et al. (2000) from visible and near infrared bands. Drought conditions in LST–NDVI, albedo–NDVI spectral space is quantified simply by implementation of LST/NDVI, albedo/NDVI operation; and values of four different indexes are normalized within 0–1 through statistical computation. It is evident from the results shown in Fig. 5 that trends of all graphs are quite similar. PDI stands neutral among those indexes. In other words, PDI values are below the VTCI, above the albedo–NDVI and just very close to LST–NDVI which is well-recognized and accepted as effective drought monitoring index by the science community. PDI is achieved from reflectance data while other indexes need to retrieve the LST, NDVI and surface albedo to be calculated. Retrieval of LST and albedo is very expensive and always resulted in greater uncertainties imposed from atmospheric correction and BRDF modeling. Therefore, it can be concluded that PDI is effective but easy to obtain compared to other drought indexes.
Summary
In this paper, an operational, effective method for drought monitoring—PDI is developed using spectral space derived from reflectance of near infrared and red wavelengths.
Perpendicular drought index was validated with field measured data and inter-comparison with other well recognized methods such as LST–NDVI, albedo–NDVI and VTCI. Results indicated that PDI is highly consistent with ground truth drought data reckoned from soil hydrologic factors such as soil moisture at different depths, field moisture capacity and wilting coefficient, graphs tendency of PDI, field measured and other commonly used drought indexes is quite identical (Fig. 5), correlation coefficient between PDI and field obtained drought values over 25 plots reaches 0.75 at the best case. Although the number of the field measurements were limited, they allowed the confirmation that the PDI provides, as stated in the theoretical design, correct information on surface drought conditions, which is robust over different surface types. It is recommended, however, that the PDI should be validated further with more field measurements in additional ecosystems.
Soil variability in reflectance is very important. Mathematical expression of PDI is established on the case of existing fixed soil line; however, the distribution of the soil line is highly dependent on soil type and fertilization, etc.; and bright-wet soils have higher reflectance in the red and near-infrared than do dark-dry soils. Therefore, the soil line does not monotonically increase with soil dryness in some cases, especially in large-scale drought monitoring for different soil types. The potential influence from soil colour variations on the Red, NIR bands need to be further studied.
In summary, taking the advantage of multidimensional information, PDI characterizes well the surface cover types, water and energy circulation and its dynamic changes, as well as has a clear biophysical connotation. PDI based on NIR-Red spectral space is rather simple, effective, and easy to obtain and operate. Future work of this paper will be focused to handle those problems and improve the performance of PDI via introducing the short wave infrared band, which is sensitive to water content in soil and vegetation, with the taking into account the effect of plant/soil multiple scattering on PDI.
References
Bhalme HN, Mooley DA (1980) Large-scale droughts/floods and monsoon circulation. Mon Weather Rev 108:1197–1211
Carlson TN Arthur ST (2000) The impact of land use-land cover changes due to urbanization on surface microclimate and hydrology: a satellite perspective. Glob Planet Change 25:49–65
Carlson TN, Sanchez-Azofeifa GA (1999) Satellite remote sensing of land use changes in and around San Jose, Costa Rica. Remote Sens Environ 70:247–256
Chuvieco E, Cocero D, Riano D, Martin P, Martınez-Vega J, Riva J, Perez F (2004) Combining NDVI and surface temperature for the estimation of live fuel moisture content in forest fire danger rating. Remote Sens Environ 92:322–331
Ghulam A (2006) Remote monitoring of farmland drought based n-dimensional spectral feature space. PhD Dissertation, Peking University, Beijing (in Chinese)
Ghulam A, Qin Q, Wang,L, Zhan Z, Wang D (2004a) Development of broadband Albedo based ecological safety monitoring index.In: 2004 IEEE International geoscience and remote sensing symposium (IGARSS), September 20–24, 2004, Anchorage, Alaska, Egan convention center, USA, VI:4115–4118
Ghulam A, Qin Q, Zhu L, Abdrahman P (2004b) Satellite remote sensing of groundwater: quantitative modelling and uncertainty reduction using 6S atmospheric simulations. Int J Remote Sens 25(23):5509–5524
Gillies RR, Carlson TN (1995) Thermal remote sensing of surface soil water content with partial vegetation cover for incorporation into climate models. J Appl Meteorol 34:745–756
Gillies RR, Carlson TN, Cui J, Kustas WP, Humes KS (1997) A verification of the ‘triangle method for obtaining surface soil water content and energy fluxes from remote measurements of the normalized difference vegetation index (NDVI) and surface radiant temperature. Int J Remote Sens 18(5):3145–3166
Goetz SJ (1997) Multisensor analysis of NDVI, surface temperature and biophysical variables at a mixed grassland site. Int J Remote Sens 18(1):71– 94
Goward SN, Hope AS (1989) Evaporation from combined reflected solar and emitted terrestrial radiation: Preliminary FIFE results from AVHRR data. Adv Space Res 9:239–249
Han L, Wang P, Wang J, Liu Z (2005) Study on NDVI-Ts space by combining LAI and evapotranspiration. Sci China Ser D 35(4):371–377
Katz RW, Glantz MH (1986) Anatomy of a rainfall index. Mon Weather Rev 114:764–771
Kogan FN (1995a) Droughts of the late 1980s in the United States as derived from NOAA polar-orbiting satellite data. Bull Am Meteor Soc 76(5):655–668
Kogan FN (1995b) Application of vegetation index and brightness temperature for drought detection. Adv Space Res 15:91–100
Lambin EF, Rhrlich D (1996) The surface temperature-vegetation index space for land cover and land-cover change analysis. International Journal of Remote Sensing 17(3):463–487
Liang S (2003)A direct algorithm for estimating land surface broadband albedos from MODIS imagery. IEEE Trans Geosci Remote Sens 41(1):136–145
McKee TB, Doesken NJ, Kleist J (1993) The relationship of drought frequency and duration to time scales. In: Proceedings of the 8th Conference on applied climatology. American Meteorological Society, Boston, pp 179–184
Nemani RR, Running SW (1989) Estimation of regional surface resistance to evapotranspiration from NDVI and thermal infrared AVHRR data. J Appl Meteorol 28:276–284
Owen TW, Carlson TN, Gillies RR (1998) An assessment of satellite remotely-sensed land cover parameters in quantitatively describing the climatic effect of urbanization. Int J Remote Sens 19(9):1663–1681
Palmer WC (1965) Meteorological drought. Research Paper No. 45, US Weather Bureau, Washington
Palmer WC (1968) Keeping track of crop moisture conditions, nationwide: the crop moisture index. Weatherwise 21:156–161
Pokrovsky O, Roujean J-L (2002) Land surface albedo retrieval via kernel-based BRDF modeling: 1. Statistical inversion method and model comparison. Auditori de Torrent, Spain, September 2002. Remote Sens Environ 84:100–119
Price JC (1990) Using spatial context in satellite data to infer regional scale evaportranspiration. IEEE Trans Geosci Remote Sens 28:940–948
Qin Z, Karnieli A, Berliner P (2001) A mono-window algorithm for retrieving land surface temperature from TM data and its application to the Israel-Egypt border region. Int J Remote Sens 22(18):3719–3746
Richardson AJ, Wiegand CL (1977) Distinguishing vegetation from soil background information. Photogrammetric Eng Remote Sens 43(12):1541–1552
Ridd MK (1995) Exploring a VIS (vegetation-impervious-surface-soil) model for urban ecosystem analysis through remote sensing: comparative anatomy for citied. Int J Remote Sens 16:2165–2185
Sandholt I, Rasmussen K, Andersen J (2002) A simple interpretation of the surface temperature/vegetation index space for assessment of surface moisture status. Remote sens Environ 79:213–224
Shafer BA, Dezman LE (1982) Development of a Surface Water Supply Index (SWSI) to assess the severity of drought conditions in snowpack runoff areas. In: Proceedings of the 50th annual western snow conference. Colorado State University, Fort Collins, pp 164–175
Strommen N, Krumpe P, Reid M, Steyaert L (1980) Early warning assessments of droughts used by the US agency for international development. In: Pocinki LS, Greeley RS, Slater L (eds) Climate and risk. The MITRE corporation, McLean, pp 8–37
Su Z, Abreham Yacob, Jun W (2003) Assessing relative soil moisture with remote sensing data: theory, experimental validation, and application to drought monitoring over the North China Plain. Phys Chem Earth 28:89–101
Van Rooy MP (1965) A rainfall anomaly index independent of time and space. Notos 14:43–48
Vermote FE, Tanre D, Deuze LJ, Herman M, Morcrette J-J (1997) Second Simulation of the Satellite Signal in the Solar Spectrum, 6s: An Overview. IEEE Trans Geosci Remote Sens 35(3):675–686
Wan Z, Wang P, Li X (2004) Using MODIS land surface temperature and normalized difference vegetation index products for monitoring drought in the southern Great Plains, USA. Int J Remote Sens 25(1):61–72
Wang P, Li X, Gong J, Song C (2001) Vegetaion temperature condition index and its application for drought monitoring. In: Proceedings of International geoscience and remote sensing symposium, 9–14 July, 2001, Sydney, Australia (Piscataway, NJ: IEEE), pp 141–143
Wang C, Luo C, Qi S, Niu Z (2005) A method of land cover classification for China based on NDVI-Ts space (in Chinese). J Remote Sens 9(1):93–99
Zhan Z, Peng Y, Wang K (1999) Drought condition analysis of Loess plateau. Areal Res Dev (in Chinese) 18(2):25–27
Zhan Z, Qin Q, Ghulam A, Wang D (2006) NIR–red spectral space based new method for soil moisture monitoring. Science in China (in press)
Zhao W, Tamura M, Takahashi H (2000) Atmospheric and spectral corrections for estimating surface albedo from satellite data using 6S code. Remote Sens Environ 76:202–212
Acknowledgments
The authors would like to extend their thanks to Professor Xu Xiru from the Institute of RS & GIS, Peking University for his kind suggestions and anonymous reviewers for their helpful comments. This work was supported by the Special Funds for Major State Basic Research (973) Project (Grant No.: G2000077900), the High-Tech Research and Development Program of China (Grant No.: 2001AA135110) and the New Technology Promotion Project from China Meteorological Administration (CMA)-monitoring and evaluation of meteorological disasters based on the retrieval of surface biophysical parameters with remote sensing data (Grant: CMATG2005M44).
Author information
Authors and Affiliations
Corresponding author
Rights and permissions
About this article
Cite this article
Ghulam, A., Qin, Q. & Zhan, Z. Designing of the perpendicular drought index. Environ Geol 52, 1045–1052 (2007). https://doi.org/10.1007/s00254-006-0544-2
Received:
Accepted:
Published:
Issue Date:
DOI: https://doi.org/10.1007/s00254-006-0544-2