Abstract
Understanding the population structure and linkage disequilibrium (LD) is a prerequisite for association mapping of complex traits in a target population. In this study, we assessed the genetic diversity, population structure and the extent of LD in a panel of 192 inbred lines of Brassica napus from all over the world using 451 single-locus microsatellite markers. The inbred lines could be divided into P1 and P2 groups by a model-based population structure analysis. Out of the 142 inbred lines in the P1 group, 126 lines were from China and Japan, and the remaining 16 lines were from Europe, Canada and Australia. In the P2 group, 33 out of the 50 lines were from Europe, Canada, and Australia, and the remaining 17 lines were from China. Structure analysis further divided each group into two subgroups. AMOVA, pairwise F ST and neutrality analyses confirmed the differentiation between groups and subgroups. More than 80 % of the pairwise kinship estimates between inbred lines were <0.05, indicating that relative kinship is weak in our panel. Only 6 % linked marker pairs showed LD, suggesting the low level of LD in this association panel. The LD decayed within 0.5–1 cM at the genome level, and varied considerably across each group and subgroup, due to the population size, genetic background and genetic drift. The characterization of the population structure and LD patterns would be useful for performing association studies for complex agronomic traits in rapeseed.
Similar content being viewed by others
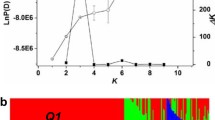
Avoid common mistakes on your manuscript.
Introduction
Most agronomic traits in crops are controlled by complex quantitative trait loci (QTLs) and their genetic bases are usually dissected using QTL mapping. In rapeseed (Brassica napus L.), studies identified QTLs for many quantitative traits such as oil content (Delourme et al. 2006), yield and yield components (Radoev et al. 2008), as well as flowering time (Long et al. 2007). In all these studies, the QTL mapping had been performed in segregating populations derived from biparental crosses. Although this approach had been proved to be efficient in detecting QTLs, it is difficult to identify closely linked markers for marker-assisted selection due to limited recombination events in only one cross. In addition, the ability to detect QTL in biparental populations is limited by the frequency of polymorphic loci between the two parents and the ability of the marker system to detect these loci.
Association analysis is an alternative for QTL mapping by using collections of varieties and breeding lines (Flint-Garcia et al. 2003). Distinct from the conventional QTL mapping, association mapping is based on linkage disequilibrium (LD) and utilizes the higher number of historical recombination events in natural population, thus a higher resolution of QTL mapping can be achieved than using the biparental segregating populations (Ersoz et al. 2007). Association mapping has been demonstrated to be a powerful tool for not only mapping QTLs in plants (Ersoz et al. 2007), but also identifying causal polymorphism within a gene that is responsible for the phenotypic variations (Yan et al. 2010). In addition, association mapping takes shorter research time, and investigates a greater number of alleles when compared with linkage analysis (Flint-Garcia et al. 2003).
The principle of association mapping is to detect correlations between phenotypes and linked markers on the basis of LD (Ersoz et al. 2007). Therefore, it is important to characterize LD levels and patterns in a population analyzed and to infer evolutionary forces including genetic drift, population structure, population admixture, levels of inbreeding, and selection that contribute to the emergence and maintenance of LD. Patterns of LD have been characterized in several crop species. The distance of LD varies significantly between outcrossing and inbreeding plants (Flint-Garcia et al. 2003). LD decays rapidly within 1–5 kb in maize diverse inbred lines (Yan et al. 2009), 1.1 kb in cultivated sunflower (Liu and Burke 2006), 300 bp in wild grapevine (Lijavetzky et al. 2007), whereas LD decays slowly within 250 kb in Arabidopsis (Nordborg et al. 2002), 100–200 kb in rice diverse lines (McNally et al. 2009; Huang et al. 2010) and 250 kb in cultivated soybean (Lam et al. 2010).
Oilseed rape is an important source of edible oil and protein-rich meal worldwide. Due to the complexity of its genome structure and the lack of high-quality molecular markers, understanding of the population structure and LD in rapeseed (Brassica napus L.) is limited so far and lagged behind the other crop species. Recently, several studies had been carried out in rapeseed. LD decays within 1–2 cM in 85 European rapeseed genotypes with canola quality (Ecke et al. 2010; Honsdorf et al. 2010), and more than 30 cM in both the resynthesized and traditional B. napus populations of 103 and 69 lines, respectively (Zou et al. 2010). The origins of the rapeseed cultivars and inbred lines used in these studies were limited and restricted to the European countries. Therefore, further characterization of the population structure and LD levels in a panel of genetically diverse genotypes collected from all over the world will be a benefit for association mapping of complex traits in rapeseed.
In this study, we genotyped a panel of 192 rapeseed inbred lines from all over the world using 451 single-locus SSR markers. The objectives of our research were to (1) assess the genetic diversity of our association mapping panel; (2) investigate the population structure among the inbred lines; (3) detect the patterns of LD in this panel.
Materials and methods
Sampling of inbred lines
A collection of 307 rapeseed (Brassica napus L.) inbred lines from the world major rapeseed growing countries were planted in the experimental field at Huazhong Agricultural University, Wuhan, China, in 2007 to evaluate their adaptability to local climate. Varieties with extremely early or late flowering and low seed setting were excluded from further analysis. The remaining lines were assayed using 13 unlinked microsatellite or simple sequence repeat (SSR) markers for discarding genetically similar genotypes. Finally, a panel of 192 inbred lines was selected, which included 139 lines from China, 24 lines from Europe, 17 lines from Canada, 8 lines from Australia, and 4 lines from Japan, for association mapping. These inbred lines have been self-pollinated for over five generations in Wuhan to decrease the residual heterozygosity in all accessions.
“Zhongyou 821 (ZY821)” and “No. 2127-17”, two parental lines that had been used to develop a double haploid (DH) population (BnaZNDH) for construction of a high-density genetic map (Cheng et al. 2009; Li et al. 2010; Xu et al. 2010), were also included in our panel. The detailed information of the 192 accessions was listed in Supplemental Table 1.
SSR genotyping
Genomic DNA was extracted from leaf tissues collected from a single plant of each accession. SSR markers from different resources were used for genotyping. Markers prefixed with Ra, Ol, Na, Ni, BN, MR, BRMS, and FITO were obtained at http://www.brassica.info/resource/markers.php, markers prefixed with BRAS and CB were obtained from literature (Radoev et al. 2008), markers prefixed with sN, sR, and sO were developed by Agriculture and Agri-Food Canada (http://brassica.agr.gc.ca/index_e.shtml). Markers prefixed with BnGMS, BnEMS, BrGMS, and BoGMS were developed by our laboratory (Cheng et al. 2009; Fan et al. 2010; Xu et al. 2010; Li et al. 2010).
Brassica napus (2n = 38, AACC) is an allotetraploid, arising from natural hybridization of the diploid species, B. rapa (2n = 20, AA) and B. oleracea (2n = 18, CC) (U 1935), probably during human cultivation (i.e., <10,000 years ago). Comparative studies revealed that the majority of the Arabidopsis genome could be aligned to six segments of the B. napus genome, indicative of triplication in the genomes of both progenitor species (Parkin et al. 2002). Due to the homeologous nature of the B. napus genome, SSR markers usually detect multiple loci, which make it difficult to assign alleles to specific loci. In this study, SSR markers were chosen only if they segregated in a single-locus model (Chen et al. 2008) to reduce ambiguous genotyping. Markers with more than 10 % missing data were not used for further analysis. PCR reaction followed the protocol described by Cheng et al. (2009) and the PCR products were visualized on 6 % polyacrylamide gel. For each SSR locus, alleles were scored in ascending order according to the amplified fragment size.
The positions of 191 SSR markers on the chromosomes were determined based on the genetic maps derived from the BnaZNDH population (Cheng et al. 2009; Li et al. 2010; Xu et al. 2010; Wang et al. 2011). For markers that were not on the genetic map, their chromosome information was inferred from their sequences assigned to the B. rapa physical map (http://www.brassica.info/resource/sequencing/status.php; Xu et al. 2010).
Statistical analysis
Genetic diversity
The number of alleles, gene diversity, and polymorphism information content (PIC) were estimated using the PowerMarker version 3.51 (Liu and Muse 2005). Large samples are expected to have more alleles than small samples. To compare the allele diversity in large samples with that in small samples, allele richness was estimated using the rare-fraction method in the HP-RARE package (Kalinowski 2005). The differences of gene diversity, PIC, and allele richness across loci were assessed using the Wilcoxon’s paired test implemented in SAS 8.02 (SAS Institute 1999).
Population structure and differentiation analyses
The model-based program STRUCTURE v2.2 (Pritchard et al. 2000) was used to infer population structure and to assign inbred lines to groups or subgroups using 451 SSR markers. Iterations were done for 10,000 times using a burn-in length of 10,000 MCMC (Markov Chain Monte Carlo) with the admixture and related frequency model. Five independent simulations were performed for each k (the number of populations), ranging from 1 to 10. The optimal k value was determined by the posterior probability [LnP(D)] and an ad hoc statistic Δk based on the rate of change in LnP(D) between successive k (Evanno et al. 2005). Inbred lines were assigned to corresponding groups based on their maximum membership probabilities, as done by Remington et al. (2001). The inferred groups were further subdivided into subgroups using a similar methodology. Because the pedigree information of many inbred lines was unknown, the classification of the lines was largely based on the STRUCTURE results.
Principal coordinate analysis (PCA) implemented in SAS 8.02 (SAS Institute 1999) and the unrooted neighbor-joining (N-J) tree based on the Nei’s distance using MEGA 4.0 (Tamura et al. 2007) were employed to depict genetic relationship between the 192 rapeseed inbred lines. Using inferred groups and subgroups, the hierarchical analysis of population differentiation was conducted using analysis of molecular variance (AMOVA) implemented in Arlequin V3.1 (Excoffier et al. 2005), with 1,000 permutations and sum of squared size differences as molecular distance. Genetic differentiation between pairs of groups or subgroups was calculated with pairwise F ST, a measure of heterozygosity within subpopulations relative to the total population (Weir and Cockerham 1984).
Neutrality test
The Ewens-Watterson’s neutrality test (Ewens 1972; Watterson 1978) was performed in each group and subgroup using the Manly’s algorithm (1985) implemented in the software PopGene version 1.31 (Yeh et al. 1999) to investigate the selective neutrality across loci. This is to test whether the observed homozygosity, calculated as the sum of squared allele frequency, is significantly higher or lower than the expected homozygosity by simulation under neutrality expectations and could suggest whether selection is in operation on a particular locus across populations (Ewens 1972; Watterson 1978).
Relative kinship
The relative kinship estimates identity by descent (IBD) by adjusting the probability of identity by state between two individuals with the average probability of identity by state between random individuals (Hardy and Vekemans 2002). The kinship matrix comparing all pairs of the 192 inbred lines was calculated on the basis of 451 SSRs using the software package SPAGeDi (Hardy and Vekemans 2002). All negative kinship values between individuals were set to zero (Yu et al. 2006).
Linkage disequilibrium
LD was estimated as the correlation coefficient r 2 between all pairs of SSRs using the package TASSEL version 2.1 (Bradbury et al. 2007). Only those SSRs with known chromosome information that published in previous studies were used for LD estimation (Cheng et al. 2009; Li et al. 2010; Xu et al. 2010; Wang et al. 2011). Rare alleles with allele frequency of <0.05 were treated as missing data (Wen et al. 2009). SSR markers on the same chromosome were considered as linked markers, and SSR markers from different chromosomes as unlinked markers. The LD was estimated for global, linked and unlined markers, respectively. The 99th percentile of r 2 distribution for unlinked markers was considered as the background level of LD, which determined whether LD is due to physical linkage (Mather et al. 2007).
The decay of LD with genetic distance was estimated as previously described (Mather et al. 2007). We combined SSR pairs into distance intervals, rather than considered them individually, to reduce the influence of outliers and to obtain a better visual description of the LD decay with distance. The genetic intervals of 0–0.5, 0.5–1, 1–2, 2–5, 5–10, 10–50, 50–100, and 100–200 cM were used in this study. The r 2 value for marker distance of 0 cM was assumed to be 1 as previously described (Yan et al. 2009), then a curve was drawn to describe the trend of LD decay using the nonlinear regression model.
Re-sampling strategy was performed to evaluate the impact of sample size on LD. Ten independent samples of 20, 40, 80, and 160 inbred lines were randomly selected from the total panel, respectively. For each sample size, LD was estimated using average r 2 across 10 random samples with global, unlinked, and linked markers, respectively.
Variance components of LD
The OHTA’s (1982) variance components of LD were analyzed across chromosomes using LinkDos (Black and Krafsur 1985) to better understand the patterns of LD in our panel. The total variance of LD was partitioned into variances within \( (D_{\text{IS}}^{2} {\text{ and }}D\prime_{\text{ST}}^{2} ) \) and between groups \( (D_{\text{ST}}^{ 2} {\text{ and }}D\prime_{\text{IS}}^{ 2} ) \). The variance components followed the equation: \( D\prime_{\text{ST}}^{ 2} + D\prime_{\text{IS}}^{ 2} = D_{\text{IT}}^{ 2} \). When \( D_{\text{IS}}^{ 2} < D_{\text{ST}}^{ 2} {\text{ and }}D\prime_{\text{ST}}^{ 2} < D\prime_{\text{IS}}^{ 2} , \) genetic drift plays a predominant role in shaping observed patterns of LD. Otherwise, epistatic selection plays a predominant role in determining the patterns of LD.
Results
Population structure and relative kinship in the panel of 192 inbred lines
The population structure in the panel of 192 inbred lines was analyzed using 451 single-locus SSR markers and a model-based software STRUCTURE (Fig. 1). The structure analysis was performed with the setting of possible clusters (k) ranging from 1 to 10 with five replications for each k. The LnP(D) value increased continuously with the increase of k from 1 to 10, and the most apparent change of LnP(D) appeared when k increased from 1 to 2. In addition, a sharp peak of ∆k appeared at k = 2 (Supplemental Fig. 1). Accordingly, the total panel could be divided into two main groups, designated as P1 and P2, respectively. The P1 group contained 142 inbred lines. Out of which, 126 lines were from China and Japan, and the remaining 16 lines were from Europe, Canada, and Australia. The P2 group contained 50 lines, 33 of which were from Europe, Canada, and Australia, and the remaining 17 lines were from China. The P1 and P2 groups were further subdivided into G1 and G2, and G3 and G4 subgroups, respectively, as suggested by the results of STRUCTURE analysis (Supplemental Table 1). The G1 subgroup contained 83 lines, 59 of which were inbred lines derived from a rapeseed recurrent selection population set up in Huazhong Agricultural University (HZAU) with 300 founder lines, and the remaining 24 lines were from Southeast and Southwest China, Europe, Canada, and Australia. The G2 subgroup contained 59 lines, 30 of which were cultivars from breeding institutions in Southeast and Southwest China, and the remaining 29 lines were from Yangtze Valley of China, Europe, Canada, and Australia. The G3 subgroup contained 42 lines, 32 of which were varieties from Europe, Canada, and Australia, and the remaining 10 lines were from China. The G4 subgroup contained 8 lines, 7 of which were inbred lines resynthesized recently or bred from wide hybridizations, and the remaining 1 line was from Canada. PCA and tree-based analyses gave very similar results as the STRUCTURE analysis (Supplemental Figs. 2, 3).
Population structure of 192 rapeseed inbred lines based on 451 SSR markers. When k (the number of subpopulations) is at 2, the 192 inbred lines were classified into two groups, P1 and P2. And when k = 2, the P1 group were further divided into two subgroups, G1 and G2, and the P2 group were divided into two subgroups, G3 and G4
Based on the 451 informative SSR markers, the average relative kinship between any two inbred lines was 0.0292. About 58 % kinship estimates between inbred lines were equal to 0, and 21 % kinship estimates ranged from 0 to 0.05 (Fig. 2). These results indicated that most lines in the panel have no or very weak kinship, which might be attributed to the broad range collection of genotypes and the exclusion of similar genotypes before analysis.
Population differentiation
AMOVA was performed and pairwise F ST was calculated to investigate population differentiation. AMOVA results revealed that 3.91 % (P < 0.01) of the total molecular variation in the panel was attributed to genetic differentiation between groups, 8.21 % (P < 0.01) was attributed to differentiation among subgroups (Table 1). Pairwise F ST of the two inferred groups was 0.09 (P < 0.001), suggesting that P1 is significantly divergent from P2. The levels of differentiation among subgroups were variable with F ST ranging from 0.07 (G1 with G2, P < 0.001) to 0.22 (G2 with G4, P < 0.001) (Supplemental Table 2). A similar pattern of differentiation among subgroups was also revealed using the Nei’s minimum distance. The correlation coefficient between F ST and Nei’s distance was 0.988 (P < 0.01; Supplemental Table 2).
Genetic diversity within groups and subgroups
The genetic diversity in the total panel, each inferred group and subgroup was assessed using allele number, allelic richness, gene diversity and PIC. In the total panel, the 451 single-locus SSR markers detected a total of 1,535 alleles, with 3.4 alleles per locus. The gene diversity, PIC, and allele richness were 0.43 (0.0364–0.8237), 0.37 (0.0357–0.8043), and 2.45 (1.20–5.89), respectively (Table 2). The P1 and P2 groups contained 1,472 and 1,349 alleles, with 3.3 and 3.0 alleles per locus, respectively. The number of alleles in P2 is similar to that in P1, although the population size of P2 was only one-third of P1. Additionally, P1 and P2 had a similar level of gene diversity (z = −0.9881, P = 0.1616), PIC (z = −0.6355, P = 0.2626) and allele richness (z = 0.3203, P = 0.3744). Within P1, G1, and G2 have a similar level of PIC (z = −0.914, P = 0.1804) and allele richness (z = 0.206, P = 0.4184). Within P2, G3, and G4 exhibited a similar level of allele richness (z = −0.947, P = 0.1718), but G4 showed a higher level of gene diversity (z = 3.0641, P = 0.0011) and PIC (z = 2.1368, P = 0.0163) than G3 (Table 2).
Neutrality within groups and subgroups
Although microsatellite loci are mostly neutral in the genome, they have been also reported to reflect selective effects (Vigouroux et al. 2002b; Zhang et al. 2009). Ewens-Watterson’s neutrality tests were performed across SSR loci (Table 3) to detect whether there is selective pressure within each group and subgroup. A portion of 5.99 and 8.43 % SSR loci deviated from the neutral model (P < 0.05) within P1 and P2, respectively, and 7.10, 8.65, 10.86, and 26.16 % deviated from the neutral model (P < 0.05) within G1, G2, G3, and G4, respectively. Among all the non-neutral loci, only 5 loci were shared between P1 and P2, a major part of the non-neutral loci were group-specific (21 specific to P1 and 32 specific to P2). Similar pattern was also observed in subgroups within each group. Within P1, 80.64 and 84.21 % of the non-neutral loci were specific to G1 and G2, respectively. Within P2, 81.25 and 92.31 % of the non-neutral loci were specific to G3 and G4, respectively, which might be attributed to adaptation-based selection.
Linkage disequilibrium
LD was investigated among SSRs in the total panel and in each group and subgroup. A total of 293 markers with known chromosome information were used for LD analysis. Out of 293 markers, 191 markers showed map position information. In the panel, the average r 2 of global markers was 0.0117, and only 6 % of the global marker pairs exhibited significant LD (P < 0.001), indicating that the LD level is very low in the panel of inbred lines (Table 4). Moreover, 17 % of the linked marker pairs and 5 % of the unlinked marker pairs showed significant LD (P < 0.001), and the average r 2 of linked and unlinked markers were 0.0247 and 0.0107, respectively, demonstrating that physical linkage is predominant in determining LD compared with random forces (Flint-Garcia et al. 2003). The average r 2 of global markers ranged from 0.0112 to 0.0328 in groups and 0.0173 to 0.0311 in subgroups (The G4 subgroup is omitted from the analysis of LD because the sample size is too small), respectively, suggesting that the extent of LD was elevated when the panel was partitioned into groups and subgroups (Table 4). In all groups and subgroups, both average r 2 and proportion of significant LD for linked markers were still higher than those for unlinked markers, which reinforced the view that physical linkage strongly influences LD in this panel of inbred lines.
The r 2 estimates were pooled across the 19 chromosomes, and the average r 2 of each genetic interval was plotted against genetic distance to estimate the decay of LD. The nonlinear regression curve exhibited a clear decay of LD with increase in genetic distance (Fig. 3). In this study, the 99th percentile of r 2 distribution for unlinked markers determined the background level of LD (r 2 < 0.067). LD decayed to the background level within 0.5–1 cM at the genome level (Fig. 3). A much slower decay of LD was observed within groups and subgroups (Supplemental Fig. 4), which might be attributed to the limited population size and narrow genetic background that inhibit LD decay (Ersoz et al. 2007).
LD decays (r 2) in the association panel consisting 192 inbred lines. The r 2 value for marker distance of 0 cM is defined as 1. The dots are mean r 2 values for marker intervals of 0, 0–0.5, 0.5–1, 1–2, 2–5, 5–10, 10–50, 50–100, and 100–200 cM, respectively. The curve was drawn across the dots using the nonlinear regression model
Re-sampling was performed in the total panel of inbred lines to evaluate the impact of sample size on LD. The re-sampling analysis revealed that the average r 2 of the global, linked, and unlinked markers decreased with the increase of sample size (Fig. 4a), indicating that LD is influenced by sample size. As mentioned above, the LD in P1 was lower than that in P2 (Table 4; Supplemental Fig. 4). To eliminate the influence of sample size on LD level, 50 inbred lines were re-sampled from P1 to match the sample size in P2. The average r 2 of 10 random samples of P1 was still lower than that of P2 across all genetic intervals except for the 0–0.5 cM interval (Fig. 4b), indicating that LD variation is related to genetic background in this panel of inbred lines.
Estimates of LD (r 2) in populations re-sampled from the total panel and from the P1 group. a LDs (r 2) for global, linked and unlinked markers were estimated in 10 independent samples with 20, 40, 80, and 160 lines re-sampled from the total panel. b LDs (r 2) for genetic intervals of 0–0.5, 0.5–1, 1–2, 2–5, 5–10, 10–50, 50–100, and 100–200 cM were estimated in 10 random subpopulations with 50 inbred lines re-sampled from the P1 group and compared with that in the P2 group. The bar indicates standard deviation
The OHTA’s variance components of LD were analyzed across chromosomes to examine whether genetic drift or epistatic selection plays a predominant role in determining LD. The variances of LD between groups are greater than that within groups across 19 chromosomes, i.e., \( D_{\text{ST}}^{ 2} > D_{\text{IS}}^{ 2} ,D\prime_{\text{IS}}^{ 2} > D\prime_{\text{ST}}^{ 2} \) (Supplemental Table 3). The variation of LD across chromosomes explained by genetic drift ranged from 62.39 % for chromosome C1 to 92.71 % for chromosome C3. This result implied that genetic drift plays a significant role in determining variation of LD in this panel of inbred lines.
Discussion
Genetic diversity in the rapeseed panel
Since SSR primers usually amplify several alleles from multiple homoeologous loci in B. napus, multiple-locus SSR markers are usually highly polymorphic. However, it is difficult to assign the multiple alleles to individual loci in B. napus (Hasan et al. 2008). In this study, 451 single-locus SSR markers randomly distributed in the B. napus genome were selected to evaluate the genetic diversity in the panel of 192 inbred lines. A total of 1,535 alleles, with an average of 3.4 alleles per locus, were detected in the rapeseed panel. The number of alleles per locus is slightly lower than that detected in 96 European rapeseed genotypes (Hasan et al. 2006), but higher than that in 72 rapeseed accessions (Chen et al. 2008). The difference of allelic richness between our panel and other germplasm collections may be caused by the differences of germplasm materials analyzed (Fukunaga et al. 2005) and only the single-locus SSR markers used (Vigouroux et al. 2002a). The utilization of the single-locus SSR markers for genotyping reduced the complexity of genotype scoring and analysis, however, the employment of only single-locus SSR markers may underestimate the genetic diversity of the panel.
Population structure and differentiation in the association panel
A collection of natural varieties usually generates high false positive rate in association mapping, therefore understanding of population structure of analyzed population is critically important for association mapping (Flint-Garcia et al. 2003). In this study, the 192 lines were clearly classified into two groups (P1 and P2). Inbred lines in P1 were mostly from China, and lines in P2 mostly from Europe, Canada, and Australia, which is consistent with the geographical distribution. Nevertheless, it was observed that a few lines in each group that are not in accordance with their geographical origins, which is consistent with the results in previous studies that demonstrated separation between Chinese and European or Australian cultivars assessed by agronomically important traits (Hu et al. 2007) or molecular markers (Chen et al. 2008, 2010). Previous studies have shown that Australian and European types clustered with some Chinese accessions (Cowling 2007), but also that certain Chinese accessions form a stand-alone group (Chen et al. 2008, 2010). Present results contrast with these findings, as Australian and European types were found in all groups and subgroups with Chinese types. B. napus is originated and originally cultivated in Europe (Liu 1984), and then spread to Australia, Canada and Japan. It was first introduced to China in the 1930–1940 directly from Europe or indirectly from Japan (Liu 1985). After introduced into China, B. napus cultivars have been crossed intensively to the Chinese B. rapa, the traditional Chinese oilseed crop that has been cultivated for more than 6,000 years for vegetables and edible oil, to introgress genes for adaption to local environments. At the end of 1970, a set of B. napus cultivars with low levels of either erucic acid or glucosinates were introduced from Europe, Canada, and Australia. Recently, the exchanges of germplasm between China and Europe, Australia and Canada are more frequent than ever before and used for intercross breeding and recurrent selection in these countries (Cowling 2007; Chen et al. 2008), which resulted in the introgression of exotic genomic fragments of varieties between these countries.
Population structure is an indicator of genetic differentiation among groups and subgroups. Several analyses were performed for evaluation of population differentiation to elucidate the population genetic basis of population structure in our association panel. The AMOVA results revealed that the genetic differentiation among groups and subgroups is significant, which is consistent with the results of pairwise F ST, suggesting the existence of population differentiation in this panel of 192 lines (Table 1; Supplemental Table 2). The PCA and N-J tree analyses provided a better visual description of genetic differentiation among subgroups (Supplemental Figs. 2, 3). Variable numbers of non-neutral loci were identified in each group and subgroup, reflecting that selection played an important role in shaping the population differentiation (Zhang et al. 2009). In addition, the majority of non-neutral loci were specific to group or subgroup, which further confirmed the existence of population differentiation in the panel.
Patterns of linkage disequilibrium in the rapeseed panel
LD reflects the evolutionary history of genome in a species (Ersoz et al. 2007). In this study, the 192 inbred lines collected from all over the world were used to estimate the extent of LD. The average r 2 in our association panel is 0.0117, which is lower than that detected in 85 European winter-type rapeseed accessions with canola quality (the average r 2 = 0.027) (Ecke et al. 2010). The LD decayed within 0.5–1 cM at the whole genome level (r 2 < 0.067) in our panel, and 1–2 cM at r 2 < 0.4 in the population of European winter-type rapeseed accessions (Ecke et al. 2010). If the threshold of LD decay is set to r 2 < 0.4 in this study, the distance of LD decay should be much shorter than 1–2 cM as described by Ecke et al. (2010) (Fig. 3). Lower r 2 and faster decay of LD in our panel are expected because the inbred lines have a diverse genetic background (Flint-Garcia et al. 2003; Supplemental Table 1; Table 2).
Physical linkage that determines LD between molecular marker and causative polymorphisms is the genetic basis for association mapping of genes or QTLs underlying traits of interest (Flint-Garcia et al. 2003). In this study, the extent of LD of linked markers is significantly higher than that of unlinked markers (Table 4), indicating that this rapeseed panel is suitable for association analysis and has the potential to identify QTL in a narrow interval equivalent to the distance of LD decay of 0.5–1 cM. This resolution is apparently higher than the conventional linkage mapping based on a biparental segregating population with the similar size. Based on the distance of LD decay in the population of European winter-type rapeseed accessions, it is estimated that 9,600 markers are required for genome wide association study (GWAS) with LD extending to 1–2 cM (Ecke et al. 2010). In our association panel, the LD decayed faster than that in the population of European winter-type rapeseed accessions, suggesting that more markers are probably needed for GWAS of complex traits. Therefore, the utilization of high-throughput genotyping by sequencing technology is necessary. To our present knowledge, no reference genome sequence of B. napus is available, however, genotyping of the association panel with such a large number of markers is still a very difficult task. On the other hand, due to the polyploidy nature of B. napus genome (Parkin et al. 2002) and the triplication of its two progenitor’s genomes of B. rapa and B. oleracea, bioinformatic analysis of the short reads generated with the next-generation sequencing technologies is also a big challenge in B. napus. However, Trick et al. (2009) recently developed a methodology and computational tools to exploit SNPs from short RNA reads generated using the Solexa sequencing system. By using a publicly available set of approximately 94,000 Brassica unigenes as a reference sequence, they discovered 23,330–41,593 putative single nucleotide polymorphisms (SNPs) between two cultivars, depending on the read depth stringency applied, suggesting that the discovery of SNPs in polyploidy B. napus is also feasible.
Various levels of LD in groups and subgroups were observed, indicating that population structure has significant impact on LD (Table 4; Supplemental Fig. 4). In the association panel, the impact of population structure on LD is at least partially attributed to the effect of population size (Fig. 4a). Resampling analysis indicated that P2 had higher level of LD than P1, although it had a much smaller sample size (Fig. 4b), suggesting that genetic background (allelic diversity and specific non-neutral loci, see in Tables 2, 3) also affects the level of LD as reflected by the impact of population structure on LD. The analysis of variance component of LD revealed that genetic drift predominantly causes LD variation between groups. Altogether, these results suggested that population size, genetic background and genetic drift together shaped the pattern of LD in this panel of inbred lines (Myles et al. 2009). However, due to the lack of more single-locus SSR markers, the LD patterns on different linkage groups were not evaluated in our study. Therefore, genotyping the association panel with high-throughput SNP markers should depict an LD map with a higher resolution, which will enhance our understanding of the population structure, LD decay and LD distribution.
References
Black WC, Krafsur ES (1985) A FORTRAN program for analysis of genotypic frequencies and description of the breeding structure of populations. Theor Appl Genet 70:484–490
Bradbury PJ, Zhang Z, Kroon DE, Casstevens TM, Ramdoss Y, Buckler ES (2007) TASSEL: software for association mapping of complex traits in diverse samples. Bioinformatics 23:2633–2635
Chen S, Nelson MN, Ghamkhar K, Fu T, Cowling WA (2008) Divergent patterns of allelic diversity from similar origins: the case of oilseed rape (Brassica napus L.) in China and Australia. Genome 51:1–10
Chen S, Zou J, Cowling WA, Meng J (2010) Allelic diversity in a novel gene pool of canola-quality Brassica napus enriched with alleles from B. rapa and B. carinata. Crop Pasture Sci 61:483–492
Cheng X, Xu J, Xia S, Gu J, Yang Y, Fu J, Qian X, Zhang S, Wu J, Liu K (2009) Development and genetic mapping of microsatellite markers from genome survey sequences in Brassica napus. Theor Appl Genet 118:1121–1131
Cowling W (2007) Genetic diversity in Australian canola and implications for crop breeding for changing future environments. Field Crops Res 104:103–111
Delourme R, Falentin C, Huteau V, Clouet V, Horvais R, Gandon B, Specel S, Hanneton L, Dheu JE, Deschamps M, Margale E, Vincourt P, Renard M (2006) Genetic control of oil content in oilseed rape (Brassica napus L.). Theor Appl Genet 113:1331–1345
Ecke W, Clemens R, Honsdorf N, Becker H (2010) Extent and structure of linkage disequilibrium in canola quality winter rapeseed (Brassica napus L.). Theor Appl Genet 120:921–931
Ersoz ES, Yu J, Buckler ES (2007) Applications of linkage disequilibrium and association mapping in crop plants. Genomics-assisted crop improvement Springer, Dordrecht, pp 97–120
Evanno G, Regnaut S, Goudet J (2005) Detecting the number of clusters of individuals using the software structure: a simulation study. Mol Ecol 14:2611–2620
Ewens WJ (1972) The sampling theory of selectively neutral alleles. Theor Popul Biol 3:87–112
Excoffier L, Laval G, Schneider S (2005) Arlequin version 3.0: an integrated software package for population genetics data analysis. Evol Bioinform Online 1:47–50
Fan C, Cai G, Qin J, Li Q, Yang M, Wu J, Fu T, Liu K, Zhou Y (2010) Mapping of quantitative trait loci and development of allele-specific markers for seed weight in Brassica napus. Theor Appl Genet 121:1289–1301
Flint-Garcia SA, Thornsberry JM, Iv B SE (2003) Structure of linkage disequilibrium in plants. Ann Rev Plant Biol 54:357–374
Fukunaga K, Hill J, Vigouroux Y, Matsuoka Y, Sanchez GJ, Liu K, Buckler ES, Doebley J (2005) Genetic diversity and population structure of teosinte. Genetics 169:2241–2254
Hardy OJ, Vekemans X (2002) SPAGeDi: a versatile computer program to analyse spatial genetic structure at the individual or population levels. Mol Ecol Notes 2:618–620
Hasan M, Seyis F, Badani AG, Pons-Kühnemann J, Friedt W, Lühs W, Snowdon RJ (2006) Analysis of genetic diversity in the Brassica napus L. gene pool using SSR markers. Genet Resour Crop Evol 53:793–802
Hasan M, Friedt W, Pons-Kühnemann J, Freitag NM, Link K, Snowdon RJ (2008) Association of gene-linked SSR markers to seed glucosinolate content in oilseed rape (Brassica napus ssp. napus). Theor Appl Genet 116:1035–1049
Honsdorf N, Becker HC, Ecke W (2010) Association mapping for phenological, morphological, and quality traits in canola quality winter rapeseed (Brassica napus L.). Genome 53:899–907
Hu S, Yu C, Zhao H, Sun G, Zhao S, Vyvadilova M, Kucera V (2007) Genetic diversity of Brassica napus L. Germplasm from China and Europe assessed by some agronomically important characters. Euphytica 154:9–16
Huang X, Wei X, Sang T, Zhao Q, Feng Q, Zhao Y, Li C, Zhu C, Lu T, Zhang Z, Li M, Fan D, Guo Y, Wang A, Wang L, Deng L, Li W, Lu Y, Weng Q, Liu K, Huang T, Zhou T, Jing Y, Li W, Lin Z, Buckler ES, Qian Q, Zhang Q-F, Li J, Han B (2010) Genome-wide association studies of 14 agronomic traits in rice landraces. Nat Genet 42:961–967
Kalinowski S (2005) HP-RARE 1.0: a computer program for performing rarefaction on measures of allelic richness. Mol Ecol Notes 5:187–189
Lam H, Xu X, Liu X, Chen W, Yang G, Wong F-L, Li M-W, He W, Qin N, Wang B, Li J, Jian M, Wang J, Shao G, Wang J, Sun SS-M, Zhang G (2010) Resequencing of 31 wild and cultivated soybean genomes identifies patterns of genetic diversity and selection. Nat Genet 42:1053–1059
Li H, Chen X, Yang Y, Xu J, Gu J, Fu J, Qian X, Zhang S, Wu J, Liu K (2010) Development and genetic mapping of microsatellite markers from whole genome shotgun sequences in Brassica oleracea. Mol Breed. doi:10.1007/s11032-010-9509-y
Lijavetzky D, Cabezas JA, Ibanez A, Rodriguez V, Martinez-Zapater JM (2007) High throughput SNP discovery and genotyping in grapevine (Vitis vinifera L.) by combining a re-sequencing approach and SNPlex technology. BMC Genomics 8:424
Liu H (1984) Origin and evolution of rapeseeds. Acta Agron Sin 10:9–18
Liu H (1985) Rapeseed genetics and breeding. Shanghai Science and Technology Press, Shanghai, pp 559–566
Liu A, Burke JM (2006) Patterns of nucleotide diversity in wild and cultivated sunflower. Genetics 173:321–330
Liu K, Muse SV (2005) PowerMarker: an integrated analysis environment for genetic marker analysis. Bioinformatics 21:2128–2129
Long Y, Shi J, Qiu D, Li R, Zhang C, Wang J, Hou J, Zhao J, Shi L, Park B-S, Choi SR, Lim YP, Meng J (2007) Flowering time quantitative trait loci analysis of oilseed Brassica in multiple environments and genome wide alignment with Arabidopsis. Genetics 177:2433–2444
Manly B (1985) The statistics of natural selection. Chapman and Hall, London
Mather KA, Caicedo AL, Polato NR, Olsen KM, McCouch S, Purugganan MD (2007) The extent of linkage disequilibrium in rice (Oryza sativa L.). Genetics 177:2223–2232
McNally KL, Childs KL, Bohnert R, Davidson RM, Zhao K, Ulat VJ, Zeller G, Clark RM, Hoen DR, Bureau TE, Stokowski R, Ballinger DG, Frazer KA, Cox DR, Padhukasahasram B, Bustamante CD, Weigel D, Mackill DJ, Bruskiewich RM, Rätsch G, Buell CR, Leung H, Leach JE (2009) Genomewide SNP variation reveals relationships among landraces and modern varieties of rice. Proc Natl Acad Sci USA 106:12273–12278
Myles S, Peiffer J, Brown PJ, Ersoz ES, Zhang Z, Costich DE, Buckler ES (2009) Association mapping: critical considerations shift from genotyping to experimental design. Plant Cell 21:2194–2202
Nordborg M, Borevitz JO, Bergelson J, Berry CC, Chory J, Hagenblad J, Kreitman M, Maloof JN, Noyes T, Oefner PJ, Stahl EA, Weigel D (2002) The extent of linkage disequilibrium in Arabidopsis thaliana. Nat Genet 30:190–193
Ohta T (1982) Linkage disequilibrium due to random genetic drift in finite subdivided populations. Proc Natl Acad Sci USA 79:1940–1944
Parkin IAP, Lydiate DJ, Trick M (2002) Assessing the level of collinearity between Arabidopsis thaliana and Brassica napus for A-thaliana chromosome 5. Genome 45:356–366
Pritchard J, Stephens M, Donnelly P (2000) Inference of population structure using multilocus genotype data. Genetics 155:945–959
Radoev M, Becker HC, Ecke W (2008) Genetic analysis of heterosis for yield and yield components in rapeseed (Brassica napus L.) by QTL mapping. Genetics. doi:108.089680
Remington DL, Thornsberry JM, Matsuoka Y, Wilson LM, Whitt SR, Doebley J, Kresovich S, Goodman MM, Buckler Iv ES (2001) Structure of linkage disequilibrium and phenotypic associations in the maize genome. Proc Natl Acad Sci USA 98:11479–11484
Tamura K, Dudley J, Nei M, Kumar S (2007) MEGA4: molecular evolutionary genetics analysis (MEGA) software version 4.0. Mol Biol Evol 24:1596–1599
The SAS Institute (1999) SAS/STAT User’s Guide, Version 8. SAS Institute, Cary
Trick M, Long Y, Meng JL, Bancroft I (2009) Single nucleotide polymorphism (SNP) discovery in the polyploid Brassica napus using Solexa transcriptome sequencing. Plant Biotechnol J 7:334–346
U (1935) Genome analysis in Brassica with special reference to the experimental formation of B. napus and peculiar mode of fertilization. Jpn J Bot 7:389–425
Vigouroux Y, Jaqueth JS, Matsuoka Y, Smith OS, Beavis WD, Smith JSC, Doebley J (2002a) Rate and pattern of mutation at microsatellite loci in maize. Mol Biol Evol 19:1251–1260
Vigouroux Y, McMullen M, Hittinger CT, Houchins K, Schulz L, Kresovich S, Matsuoka Y, Doebley J (2002b) Identifying genes of agronomic importance in maize by screening microsatellites for evidence of selection during domestication. Proc Natl Acad Sci USA 99:9650–9655
Wang F, Wang X, Chen X, Xiao Y, Li H, Zhang S, Xu J, Fu J, Huang L, Wu J, Liu K (2011) Abundance, marker development and genetic mapping of microsatellites from unigenes in Brassica napus. Mol Breed doi:10.1007/s11032-011-9658-7
Watterson GA (1978) The homozygosity test of neutrality. Genetics 88:405–417
Weir B, Cockerham C (1984) Estimating F-statistics for the analysis of population structure. Evolution 38:1358–1370
Wen W, Mei H, Feng F, Yu S, Huang Z, Wu J, Chen L, Xu X, Luo L (2009) Population structure and association mapping on chromosome 7 using a diverse panel of Chinese germplasm of rice (Oryza sativa L.). Theor Appl Genet 119:459–470
Xu J, Qian X, Wang X, Li R, Cheng X, Yang Y, Fu J, Zhang S, King GJ, Wu J, Liu K (2010) Construction of an integrated genetic linkage map for the A genome of Brassica napus using SSR markers derived from sequenced BACs in B. rapa. BMC Genomics 11:594
Yan J, Shah T, Warburton ML, Buckler ES, McMullen MD, Crouch J (2009) Genetic characterization and linkage disequilibrium estimation of a global maize collection using SNP markers. PLoS One 4:e8451
Yan J, Kandianis CB, Harjes CE, Bai L, Kim E-H, Yang X, Skinner DJ, Fu Z, Mitchell S, Li Q, Fernandez MGS, Zaharieva M, Babu R, Fu Y, Palacios N, Li J, DellaPenna D, Brutnell T, Buckler ES, Warburton ML, Rocheford T (2010) Rare genetic variation at Zea mays crtRB1 increases β-carotene in maize grain. Nat Genet 42:322–327
Yeh F, Yang R, Boyle T (1999) Popgene version 1.31, Microsoft window-based freeware for population genetic analysis. http://www.ualberta.ca/~fyeh/
Yu J, Pressoir G, Briggs WH, Bi IV, Yamasaki M, Doebley JF, McMullen MD, Gaut BS, Nielsen DM, Holland JB (2006) A unified mixed-model method for association mapping that accounts for multiple levels of relatedness. Nat Genet 38:203–208
Zhang D, Zhang H, Wang M, Sun J, Qi Y, Wang F, Wei X, Han L, Wang X, Li Z (2009) Genetic structure and differentiation of Oryza sativa L. in China revealed by microsatellites. Theor Appl Genet 119:1105–1117
Zou J, Jiang C, Cao Z, Li R, Long Y, Chen S, Meng J (2010) Association mapping of seed oil content in Brassica napus and comparison with quantitative trait loci identified from linkage mapping. Genome 53:908–916
Acknowledgments
We thank Prof. Jianbing Yan at Huazhong Agricultural University for his critical review of this manuscript, and sincerely thank Mayank Gautam for his critical reading of the manuscript. The research was supported by the National Natural Science Foundation of China (No. 31071452) and the Doctoral Fund of Ministry of Education of China (No. 20100146110019).
Author information
Authors and Affiliations
Corresponding author
Additional information
Communicated by H. Becker.
Electronic supplementary material
Below is the link to the electronic supplementary material.
122_2012_1843_MOESM1_ESM.ppt
Supplemental Fig. 1 Estimation of LnP(D) and Δk in the total panel and inferred groups. a) the total panel; b) the P1 group; c) the P2 group. The blue and red curves represent LnP(D) and Δk, respectively. The bar indicates standard deviation. Supplementary material 1 (PPT 161 kb)
122_2012_1843_MOESM2_ESM.ppt
Supplemental Fig. 2 The PCA analysis on the different populations. a) the total panel; b) the P1 group; c) the P2 group. Colored points represent inbred lines belonging to different groups and subgroups. Supplementary material 2 (PPT 172 kb)
122_2012_1843_MOESM3_ESM.ppt
Supplemental Fig. 3 Unrooted Neigbour-Joining tree of 192 inbred lines. Colored lines correspond to assignments to different model-based subgroups. Supplementary material 3 (PPT 219 kb)
122_2012_1843_MOESM4_ESM.ppt
Supplemental Fig. 4 LD decays (r 2) in the inferred groups and subgroups. The r 2 value for markers with genetic distance of 0 cM is defined as 1. The dots are mean r 2 values for marker genetic intervals of 0 cM, 0-0.5 cM, 0.5-1 cM, 1-2 cM, 2-5 cM, 5-10 cM, 10-50 cM, 50-100 cM and 100-200 cM, respectively. The curves were drawn using the nonlinear regression model. G4 subgroup was not included in the analysis due to its small population size. Supplementary material 4 (PPT 147 kb)
Rights and permissions
About this article
Cite this article
Xiao, Y., Cai, D., Yang, W. et al. Genetic structure and linkage disequilibrium pattern of a rapeseed (Brassica napus L.) association mapping panel revealed by microsatellites. Theor Appl Genet 125, 437–447 (2012). https://doi.org/10.1007/s00122-012-1843-5
Received:
Accepted:
Published:
Issue Date:
DOI: https://doi.org/10.1007/s00122-012-1843-5