Abstract
The Geospatial Stream Flow Model (GeoSFM) has been widely applied in data scarce regions for flood forecasting and stream flow simulation with remotely acquired data. GeoSFM was applied in the Upper Awash River basin (UARB) with observed input data set. GeoSFM sensitivity to observed input data quality, subbasin partition, and change in parameter were investigated. Results demonstrated that GeoSFM is sensitive to the size and number of subbasins. Among the eight model parameters, the basin loss and curve number are the most sensitive in UARB. GeoSFM evaluation using a split sample of 10 years observed daily discharge showed satisfactory performance, Nash-Sutcliff Efficiency 0.67 and 0.70, coefficient of determination, 0.60 and 0.65 for calibration and validation, respectively. The monthly average simulation captured 76 % of the observed variability over 10 years. Comparative analysis suggested increasing partitions improves performance in capturing flooding events and the single basin scenario can potentially be used for flood forecasting or resource assessment purposes. The 60 % coverage of vertisol in the basin and low quality of observed data affected model performance. Further evaluation of GeoSFM in heterogeneous soil type and land use/cover can help to identify the influence of dominant physical characteristics. In general, GeoSFM offers a competent platform for stream flow simulation and water resource assessment in data scarce regions.
Access provided by Autonomous University of Puebla. Download chapter PDF
Similar content being viewed by others
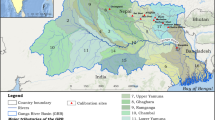
Keywords
- GeoSFM model
- FEWS-SFM model
- Flood forecasting
- Flow simulation
- Awash river
- Koka dam
- Rainfall–runoff
- Ethiopia
1 Introduction
Computer rainfall–runoff models are the go-to tools for understanding hydrological processes. These models offer fast and flexible platform to recreate historical scenarios and generate possible future ensembles. The trade-off in optimizing between capturing underlying hydrologic process and ease of application has been fading by advances in computing and data acquisition techniques. Expansion of hydrologic model domain offered variety to choose from but presented a growing challenge to identify suitable models for a particular purpose. The diversity of models can be exploited to user advantage once their strength and weaknesses are identified with respect to the intended modeling endeavor. For example, data scarcity is the major limitation to contemplate hydrological process modeling in the developing world. The growing availability of satellite-derived hydrometeorological data provides new opportunity to study watersheds of limited observed data. In response, models are being equipped with utilities to process and ingest these data.
Researchers have used different models for hydrological assessment, flow prediction, and other applications. The most commonly used model capable of predicting flows for ungauged watersheds is the Soil and Water Assessment Tool (SWAT). The application of SWAT in predicting stream flow and sediment as well as evaluation of the impact of land use and climate change on the hydrology of watersheds has been documented by various studies (Dessu and Melesse 2012, 2013a, b; Dessu et al. 2014; Wang et al. 2006a, b, 2008a, b, c; Wang and Melesse 2005; Behulu et al. 2013, 2014; Setegn et al. 2014; Mango et al. 2011a, b; Getachew and Melesse 2012; Assefa et al. 2014; Grey et al. 2013; Mohamed et al. 2015). However, SWAT has limited capability to dynamically ingest remote sensing data.
The Geospatial Stream Flow Model (GeoSFM) is developed to facilitate study of watersheds in the developing countries (Asante et al. 2008a). GeoSFM (also known as FEWS-SFM) is equipped with tools to access satellite-derived spatial and temporal input data. Despite the easy access and manipulation of remotely acquired data, verification with ground observed data remains to be critical (Dessu and Melesse 2013a; Funk et al. 2003). Neither simplicity to setup nor success histories of particular model suffices its use and implementation. Continuous testing and verification of models is essential to assist users in selection and implementation. Evaluation of GeoSFM with observed data can help to identify strength and weakness of the model, to interpret results and to improve efficiency in future applications. Exhaustive testing of a model requires sound reasoning, justifiable choices of methods, and criteria prior to practical application. Accordingly, detailed evaluation of the applicability of GeoSFM using ground observed input data set in the Upper Awash River Basin (UARB) is presented in this chapter.
GeoSFM is widely applied with satellite-derived data set in data scarce regions such as Greater Horn of Africa and Nepal. Asante et al. (2007) used the GeoSFM and TRMM (Tropical Rainfall Measuring Mission) rainfall data to develop a flood monitoring system for the Limpopo basin. They did not calibrate the model results against observed stream flow measurements, rather used the flow rates from model simulation to subjectively confirm forecasted extreme events from field sources. Mati et al. (2008) used the model to determine impact of land use/cover change in Mara River Basin. The model was also used to characterize Congo Basin stream flow using multisource satellite-derived data (Munzimi et al. 2010). Artan et al. (2007) applied GeoSFM and satellite rainfall estimates to compute runoff in the Nile River Basin and the Mekong River Basin, and reported a Nash-Sutcliff efficiency of 0.81. Shrestha (2011) used GeoSFM using observed rainfall in the Bagmati and Narayani River Basins, Nepal, and reported correlation values of 0.95 and 0.94 between observed and simulated flow rates. Shresta showed discharge estimated from satellite based rainfall follows the trend of observed values but recommended bias correction of satellite rainfall estimates. The above studies assessed hydrological variables indirectly from discharge outputs of GeoSFM based on satellite-derived spatial and temporal data inputs.
Awash River basin is currently the most developed river in Ethiopia (Fig. 18.1). The basin also represents international anthropological significance and cultural heritage (Johanson and Edey 1990; Haile-Selassie 2001; Asfaw et al. 2002). The Koka reservoir was commissioned in 1960 to store the peak summer flow from rainfall at the upstream highlands and ensure a stable discharge for power generation and irrigation in the dry period. The Koka reservoir is expected to provide sufficient head and discharge for hydroelectric power generation and irrigation and ample volume for flood storage during the summer. Hydrological challenges in the Awash basin include heavy sediment inflow to the Koka reservoir, extreme inflow variability, and lack of reliable operational and management tool. The Koka reservoir has been reported to have 35 % of its storage capacity filled with sediment from the upstream highlands (Paulos 1998). This reduction in capacity poses a challenge in the management of the reservoir and flood hazard to the residents and property downstream of the dam with unpredictable release and storage patterns. Shortage of water in the dry period and devastating flood in the summer are frequent events downstream. Dry period shortage has forced rationing of power and water for the large irrigation farms downstream. In August 1996, flooding damage was estimated at $9 million and affected 75,000 residents (DPPC 1997; Edossa et al. 2010; Rogner 2000).
According to Intergovernmental Panel on Climate Change report, Eastern Africa is expected to experience more floods in the wet season through the 21st century (Christensen et al. 2007; IPCC 2007). The current hydrologic challenge in the UARB may be exacerbated by lack of capacity and infrastructure to adapt to the changing climate (Collier et al. 2008; Yanda and Mubaya 2011; Dessu and Melesse 2013b). Hydrologic models are useful to predict extreme events of low or peak flow. GeoSFM can potentially assist in timely management and operation of Koka reservoir, avoiding flooding from excess releases as well as probable water shortage. Three to seven days rainfall forecast can be used to predict the runoff response of the Awash River and manage the reservoir accordingly. Reliable hydrological estimation and forecasting of the incoming flow may improve the operation of the Koka reservoir.
Hydrologic models have been used to understand the space–time relationship of hydrological processes. The uniqueness of watersheds can be captured by the use of distributed geospatial information from soil- and land-use databases. Planning and management tools customarily use outputs from hydrological simulations to facilitate management of water resources to meet growing demand while decreasing foreseeable hazards (Dessu et al. 2014 ) . There are numerous computer simulation models to capture rainfall–runoff process and estimate inflow discharge to a lake or reservoir.
Despite the increasing use and application of GeoSFM in data scarce regions, the performance of the model is barely scrutinized with observed input data. The purpose of this study was to assess the capability of GeoSFM to capture long-term rainfall–runoff process using observed data in the (UARB) . The specific objectives were: (1) to investigate sensitivity of GeoSFM to spatial partition scale, (2) to assess sensitivity of GeoSFM parameters in simulating the rainfall runoff process of UARB, and (3) to evaluate the performance of GeoSFM to capture observed hydrological pattern. Evaluation of GeoSFM helps to identify model strength and improve its performance efficiency for future applications. This study will help to establish baseline for further application of the model in the region using observed as well as regional remote sensing data.
2 Description of Study Area
The water resources of Awash River are key elements of development in Ethiopia . The location and hydrological properties of the basin enabled extensive development. The Awash River Basin covers 110,000 km2 area of the Great Rift Valley in Ethiopia (Fig. 18.1). The Awash River starts on the high plateau 3000 m above mean sea level (amsl) and flows along the East African rift valley into the Afar triangle and terminates in salty Lake Abbe over a stretch of 1200 km. The river drains major urban centers including Addis Ababa with population of more than 10.5 million (Taddese et al. 2003). The major economic activities in the basin are traditional mixed crop farming and livestock in the highlands, pastoralism in the lowlands, and commercial irrigation in the middle (Getahun 1978). Awash River Basin has been traditionally divided into four distinct physical and socioeconomic zones: Upper Basin, Upper Valley, Middle Valley, and Lower Valley (Taddese et al. 2003). The Upper Awash Basin (UARB) covers 11,500 km2 area stretching from the headwaters to the Koka reservoir at Koka dam .
The UARB rainfall is dictated by the Inter-Tropical Convergence Zone (ITCZ) . The rainfall distribution, especially in the highland areas, is bimodal with a short rainy season in March, April, and the main rains from June to September. The mean annual rainfall varies from 1600 mm at Ankober, in the highlands northeast of Addis Ababa to 160 mm at Asayita on the northern limit of the Basin. The mean annual runoff into Koka reservoir is 1660 million cubic meters (MCM). About 90 % of runoff occurs from July to October. Mean and maximum annual temperatures of at Koka dam are 20.8 and 23.8 °C, respectively.
3 Data and Methods
3.1 Description of Geospatial Stream Flow Model (GeoSFM)
GeoSFM (previously called FEWS-SFM) is a physically based, wide-area, continuous daily time-step, stream flow simulation model developed by the USGS/EROS Data Center (Artan et al. 2008). GeoSFM has been used to facilitate flood/drought early warning efforts by the Famine/Flood Early Warning System Network (FEWS-Net) (www.fews.net) and USAID. The model consists of a GIS-based module for data input, and a rainfall–runoff simulation model. The Graphic User Interface of the model runs in ArcGIS environment to prepare the necessary input data, perform simulation and display outputs (Asante et al. 2008a). Remote sensing or ground observed input data can be used to setup and run GeoSFM.
The rainfall–runoff simulation consists of three components: soil water budget, upland headwater basins routing, and river routing (Asante et al. 2008b). The runoff estimation conceptualizes the soil as composed of two main layers; an active soil layer where most of the soil–vegetation–atmosphere interactions take place and a groundwater layer. The active soil layer is further divided into an upper thin soil layer where evaporation, transpiration, and percolation take place and a lower soil layer where only transpiration and percolation occur. The runoff producing mechanisms is based on precipitation excess using the SCS curve number method (USDA-SCS 1972), rapid subsurface flow (interflow), and base flow. The surface upland routing is a physically based unit hydrograph method utilizing landscape attributes from digital elevation model (DEM) of the watershed. The interflow and baseflow components of the runoff are routed as a series of linear reservoirs. In the main river reaches, water is routed using a nonlinear formulation of the Muskingum-Cunge routing scheme (Cunge 1969; Kim and Lee 2010). GeoSFM provides the flow rate at the outlet of individual subbasins.
3.2 Input Data and Model Setup
GeoSFM uses spatial data to define basin physical characteristics and climate data to force simulation of rainfall–runoff process. Evaluation of GeoSFM was conducted in two steps: Input data collection and model testing. The general model setup and evaluation procedure is presented in Fig. 18.2. Required spatial inputs for model simulation are DEM of the basin, soil map, land use/cover map, and daily rainfall and potential evapotranspiration (PET) (Artan et al. 2008; Asante et al. 2008a), Fig. 18.3. Data preparation to fit GeoSFM input format was done after checking raw input data for continuity, reliability and consistency.
Topographic (scale 1:50,000 and 1:250,000) obtained from Ethiopian Mapping Agency were digitized and contours were interpolated to generate a 100 m DEM raster (Fig. 18.3a). Spot elevations and features such as rivers and streams, lakes, and roads were digitized for verification. Soil data was derived from 1:500,000 scale digitized soil unit map (Fig. 18.3b). Required soil attributes for GeoSFM were compiled from report of soil research stations in the basin (Bono and Seiler 1983), the FAO soil data base obtained from Global Soil Data Products (IGBP-DIS 2000), and guidelines presented in the GeoSFM technical manual (Asante et al. 2008b). Pellic Vertisol is the dominant soil type (>60 % of the basin). The land use/cover data was obtained from Woody Biomas Inventory project under the Ethiopian Ministry of Agriculture (Fig. 18.3c). The land use/cover attributes were reclassified to fit to the GeoSFM format. More than 75 % of the UARB is characterized by cropland with light stocks of woody plants. Land use/cover data was reclassified according to the GeoSFM format.
Observed rainfall and PET were obtained from Ethiopian Meteorological Services (Fig. 18.1). Thirty-five rain gage stations with daily rainfall record in and around the UARB were used. Daily rainfall and PET raster files were generated using inverse distance weight interpolation over the basin. Daily stream flow records derived from stage reading were obtained for the Melka Kunture and Melka Hombole stations from the Ethiopian Ministry of Water Resources Development (Fig. 18.1).
GeoSFM simulation was done for the UARB upstream of the Koka reservoir and the outlet is defined at Melka Hombole hydrologic station. Melka Hombole is at the entry of the Koka reservoir 40 km upstream of the Koka dam . To assess the models best performing module and the catchment property at different locations in the UARB, three scenarios were defined (Fig. 18.4): single basin (SB-1), 6 subbasins (SB-6), and 35 subbasins (SB-35). These three scenarios have the same basin outlet at Melka Hombole station.
For scenario SB-1, GeoSFM generates the parameters for the entire catchment and the river routing component was not dominant. Scenarios SB-6 and SB-35 activate river routing module to move the inflow from the upstream subbasin(s) to the inlet of the subbasin downstream. The single basin (SB-1) scenario is used for manual parameter sensitivity analysis and for Scenario SB-6, six set of parameter were generated and these parameters were calibrated based on the physical properties of the subbasins.
GeoSFM simulation was done over 10 years (1991–2000). The first five years (1991–1995) observed flow data segment was used for calibration and the second segment (1996–2000) was used for validation of the model. Melka Hombole stations at the inlet of Koka were used in the calibration and validation of GeoSFM (Fig. 18.1). Interpolated Rainfall and PET raster data from 1990–2000 were used for simulation and daily flow records from 1991 to 2000 at Melka Hombole station were used for calibration and validation of GeoSFM. Manual calibration was performed by changing parameter values. Due to larger set of parameters (i.e., one set for each subbasin), equivalent changes were applied for all parameters during the calibration of scenario SB-6 and SB-35.
3.3 Model Evaluation
GeoSFM model structure was evaluated based on relative comparison of the three scenarios (SB-1, SB-6, and SB-35) and observed daily discharge hydrograph. GeoSFM sensitivity to spatial scale was done by comparing 5 years daily simulated discharge outputs before calibration. Descriptive statistics of default simulation (mean, median, maximum, and quartiles) were used to assess consistency of model output. The frequencies of daily maximum discharge among the three scenarios were compared for entire discharge range and three classes of flow (<50 m3 s−1), medium (50–100 m3 s−1) and peak (>100 m3 s−1). The percentage of minimums and maximums was considered to represent the suitability of the respective partition scenario for a particular purpose. A partition scenario with higher percentage of peak flows may produce conservative flood simulation.
SB-1 scenario was used to assess sensitivity of the eight GeoSFM model parameters: soil depth, permeability, soil water holding capacity, Manning’s coefficient, curve number, top soil fraction, basin loss, and pan coefficient. The value of one parameter at a time was changed and plotted against the respective NSE (Nash-Sutcliff Efficiency). The relative rate of change of NSE was used as a measure of parameter sensitivity. A parameter displaying larger rate of change in NSE was considered more sensitive. Sensitivity analysis was done primarily on the assessment of the eight parameters used in GeoSFM . The eight parameters can be adjusted to capture the hydrologic process of a watershed (Asante et al. 2008b). Uncertainty associated with model input and model structure was evaluated based on relative performance of the three scenarios and observed daily discharge hydrograph.
The “performance,” “uncertainty,” and “realism” of GeoSFM simulations were assessed with respect to the observed flow data (Wagener 2003). Evaluation of GeoSFM was conducted based on statistical parameters and measures of “closeness” between the model output and the observed flow at Melka Hombole station. Mean relative error (MRE), Eq. 18.1, was used to evaluate unit independent expected deviation of the simulation from the observed flow. In flood simulation assessment, root mean square error (RMSE), Eq. 18.2, and NSE, Eq. 18.3, (Nash and Sutcliffe 1970) offer performance statistics sensitive to peak flow events. The RMSE value measures the models adequacy in matching the peak flows. The closer NSE values to one, the better the model efficiency; whereas, negative values generally indicate that the mean of the observed value is statistically better than the model result. Coefficient of determination (R 2), Eq. 18.4, evaluates the ability of GeoSFM in capturing the variability of observed flow.
where O is observed flow, S is simulated flow. Model performance was evaluated through objective functions that minimize the distance and optimize the variability between observed event and model result (Eq. 18.5). A second objective function that optimizes the NSE and R 2 to evaluate whether the simulations had optimally reproduced observed variability (White and Chaubey 2005; Dessu and Melesse 2012 ) of the natural hydrologic process while minimizing the overall deviation.
where k is the number of calibration sites included.
4 Results and Discussion
GeoSFM is developed for monitoring wide-area hydrologic events that require use of geospatial and time-series data in near-real time. The model was evaluated with respect to input data quality, spatial resolution, model parameters, and efficiency in capturing observed flow hydrograph. Three scenarios of singe (SB-1), six (SB-6), and thirty five (SB-35) subbasin partitions of UARB were setup and assessed based on their default, calibrated, and validated simulation outputs of daily and monthly average discharge. Ten years (1991–2000) of observed rainfall, evapotranspiration, and discharge data were used.
GeoSFM has been applied in Mara River Basin (13,750 km2) (Mati et al. 2008), Limpopo River Basin (6000 km2), (Asante et al. 2007), and Mekong River Basin (22,000 km2), (Asante et al. 2008b). The extent of spatial discretization that the model can optimally handle is useful to exchange and utilize modeling results of these studies. The three spatial partitioning scenarios are intended to provide GeoSFM flexibility with respect to size of watershed. Over the 5-years period of simulation (1991–1996), the average daily simulation outputs for the three scenarios were more than three times the 5-years observed average daily flow at Melka Hombole, 42.7 m3 s−1. For same input data and daily simulation for 5 years, the three scenarios (SB-1, SB-6, SB-35) produced a comparatively higher daily flow frequency of (12, 42, 46 %) of the time with respect to each other. To assess the effect of discretization on different flow regimes, the three scenarios were compared for daily flow brackets of >50 and >100 m3 s−1. Results showed comparative daily maximum frequency (10, 36, 54 %) and (9, 30, 61 %) for flow rates greater than 50 and 100 m3 s−1, respectively, for scenarios SB-1, SB-6 and SB-35. Low flows are barely captured in scenario SB-1 because GeoSFM handles minimum flow as zero for peripheral and 0.1 m3 s−1 for interior subbasins. This model structure may be responsible for the lower frequency of relative maximum for scenario SB-1 over the simulation period. Comparatively, scenario SB-1 produced less number of peaks than scenario SB-6 that may be attributed to averaging the hydrologic variables over the entire basin. The increasing frequency of peak flows with higher flow rate brackets for scenario SB-6 may suggests that further partitioning of UARB may improve the probability of capturing flooding events. On the other hand, scenario SB-1 is invariable for change of flow range. Scenario SB-6 has a better performance in capturing high local maximum for the whole range of flow but is less variable as the flow bracket increases. In general, results of scenario comparisons suggest that performance of GeoSFM is sensitive to the scale of basin partitioning.
Compared to the observed flow hydrograph at Melka Hombole, flood peaks prevail on a more gradually varying hydrograph. Individual subbasins were investigated to locate the beginning of peak flow at the outlet of UARB. Results from scenario SB-6 suggest that the baseflow is maintained by the upper reaches of the catchment, whereas the daily peak flows are more influenced by rainfall events in the mountainous area upstream of Melka Hombole station. Hence, further division of UARB in the scenario SB-6 has improvement in the capability of GeoSFM to separately capture local variability in the basin runoff response. The third scenario offered a better spatial variability but at the cost of larger set of parameters to tune in the calibration process.
Sensitivity analysis of eight GeoSFM parameters [Soil depth (SD), permeability (k), soil water holding capacity (SWHC), Manning’s coefficient (MC), curve number (CN), top soil fraction (TSF), basin loss (BL), and pan coefficient (k)] was conducted using SB-1 scenario (Fig. 18.5). Results have demonstrated that BL and CN are the most sensitive parameters followed by pan coefficient (k). The remaining five parameters did not show significant rate of change in NSE. Since only one parameter value was changed at a time, the result may not show combined sensitivity of the parameters. The sensitivity analysis can be used to refine the sensitive input data for the model as well as guiding the selection and adjustments of parameters in the calibration process. Identification of less sensitive parameters can facilitate the calibration process.
GeoSFM was calibrated and validated for the three scenarios (Fig. 18.4) over 10-years period (1991–2000). GeoSFM is developed for daily discharge simulation and short-term forecasting. Model performance evaluation was primarily based on the daily time-step simulation output. On the basis of the objective function, consistent performance efficiency was achieved for the three scenarios with average (NSE, R 2) results of (0.67, 0.70) for calibration and (0.60, 0.65) for validation of GeoSFM (Table 18.1). The RMS value obtained for the best run of scenario SB-35 is half of the other two scenarios suggesting further partitioning may help to capture the higher flow range. The maximum absolute error searches for the highest deviation of the historic record from the simulated flow. Very low bias from the mean value was observed suggesting an overall minimum variation of the mean of historical record and the simulated data.
The calibrated model overestimated the stream flow for the first rain season (April–July) while the peak flow during summer (July–September) is generally underestimated (Fig. 18.6a). The simulated flow hydrograph consistently crossed the observed flow hydrograph in mid of July and September in the ascending and recession limb. On the basis of cumulative annual flow volume and rainfall depth during the calibration period, the simulated flow mimics the rainfall pattern (Fig. 18.6b).
Observed rainfall and flow at Melka Hombole versus GeoSFM simulation results. a Average daily rainfall, daily observed flow, calibrated and validated simulation result (1991–2000), b mass curve for rainfall, observed flow and calibrated flow for Scenario SB-6, and c monthly observed and simulated flow hydrograph
GeoSFM was assessed based on how good the simulated runoff repeats the historical record. For instance, peak flow of 526.2 m3 s−1 was reported on 29 July 1993 but the respective rainfall value was 4.2 mm on same day and 8.8 mm for the previous day (Fig. 18.6a). Similarly, local peak flows were reported in 1992 from the simulated results unlike the small observed flow rate. The peak flow is not accompanied by a higher rainfall record. The mismatch in trend or amount of the simulated and observed flow rates may be due to the stage–discharge relationship of the rating curve used to determine discharge from a stage record. According to the observer assigned at Melka Hombole station (personal communication), the staff gage was submerged during peak floods of 1996.
On the basis of model evaluation results, GeoSFM has a potential for flood simulation and forecasting . The model performance may improve with use of better spatial resolution soil and land-use input data. However, considering annual water balance, the simulated flow has overestimated the total annual flow. Results of monthly average flow analysis over the simulation period (R 2 = 0.76) showed that GeoSFM has a potential to be used for water resource assessment (Fig. 18.6c).
The calibrated models for each scenario were validated with 5 years (1996–2000) daily data (Table 18.1). The calibrated GeoSFM model consistently overestimated stream flow for the short rain season (April–July) and underestimated the peak flow during summer (July–September). The simulated flow hydrograph crosses the observed flow hydrograph in the mid of the September overestimating the flow in the recession limb. Moreda and Bauwens (1998) reported that UARB rainfall variability is amplified on runoff response in the summer as compared to the low flow regime (November–February). This rainfall–runoff response variability may be attributed to the dominance (>60 %) of the black cotton soil (vertisol), in the UARB. Due to the high clay content, vertisol displays a range of runoff responses to rainfall depending on the available soil moisture and season of the year (Jutzi 1988). At the onset of rainy season, vertisols swell sell until all the cracks are filled and during the heavy summer the soil maintains a thin upper saturated soil layer increasing surface runoff generating higher stream flow. This could be one of the reasons that the model overestimates the stream flow in the first rainy season and underestimate the second. Therefore, the performance of the model in such soil types may need further investigation.
Annual performance of GeoSFM was assessed to pinpoint the best and worst years of simulation of the calibration and validation process (Fig. 18.7). Annual average of the daily deviation of simulation from observed flows remained below 6 m3 s−1 for the calibration period but showed a wide variability in the verification (Fig. 18.7a). Results of annual evaluation of the model performance demonstrated that GeoSFM performs best for wet years (1993, 1996, 2000) as compared to relatively dry years (1995, 1997) (Fig. 18.7a) . The correlation between the observed and simulated flow values are within the range of 0.64–0.71, indicating that the model has captured the pattern of the historical record. Comparing the maximum absolute deviation of daily simulation from the observed, the minimum in 2000 is three times the catastrophic maximum flooding year of 1996 (Fig. 18.7b). The mean annual simulated flow rate was greater than the observed through the simulation period (Fig. 18.7c). In the calibration process the model performs better for the wet season; in particular, during periods of uniform daily rainfall distribution. The weak model performance for the year 1997 may be explained by the quality of observed data (Fig. 18.7d). While Melka Kunture is upstream of Melka Hombole, and it takes a maximum of 2 days for the peak to travel between the stations, the peak was not captured at Melka Hombole.
5 Conclusion
Reliable simulation and forecasting tool may help in the management and operation of the Koka reservoir on the Awash River. GeoSM is one of such tools developed for monitoring wide-area hydrologic events that require use of geospatial and time-series data available in near-real time. This study assessed the performance of GeoSFM in stream flow and flood simulation of the UARB using ground observed data. The Awash River basin has been experiencing flooding downstream of the Koka dam. The location and hydrological properties of the basin were relatively suitable for extensive development, but catastrophic flooding has been claiming scores of human life and property damage.
Evaluation of GeoSFM was conducted on the basis of statistical measures, sensitivity to input data quality, space-time resolution of inputs and outputs, basin loss, and curve number were reported to be the most sensitive parameters. GeoSFM demonstrated satisfactory performance in daily rainfall–runoff simulation of the UARB. The model simulation also captured 76 % of the observed monthly average flow variability over 10 years. The nonlinear response of UARB to rainfall was fairly captured by the model. Summer runoff was fairly underestimated and the short rain period performance was poor. The over/underestimation may be attributed to the dominance of black cotton soil (Vertisol) in the basin, model structure, or quality of observed data. Statistical assessment of annual daily simulation indicated the potential of application of GeoSFM in data scarce regions.
The Geospatial Stream flow Model (GeoSFM) has been widely applied in flow simulation and flood forecasting with global/regional remote sensing input data in data scarce regions. Given reliable simulation performance with observed data, the application of GeoSFM for the UARB can be extended further with the increasing availability of remote sensing data. On the basis of simulation results from UARB, GeoSFM has demonstrated a fair platform for flow simulation and forecasting and hydrologic investigation in data scarce regions. Results of this study can be used as a baseline to improve the model as well as investigate its application and performance in similar hydrologic setting.
References
Artan G, Gadain H, Smith J, Asante K, Bandaragoda C, Verdin J (2007) Adequacy of satellite derived rainfall data for stream flow modeling. Nat Hazards 43(2):167–185
Artan GA, Asante K, Smith J, Pervez S, Entenmann D, Verdin JP, Rowland J (2008) Users manual for the geospatial stream flow model (GeoSFM). US Geological Survey
Asante KO, Artan GA, Pervez S, Ci Bandaragoda, Verdin JP (2008a) Technical manual for the geospatial stream flow model (GeoSFM). US geological survey, Reston
Asante KO, Arlan GA, Pervez S, Rowland J (2008b) A linear geospatial streamflow modeling system for data sparse environments. Int J River Basin Manage 6(3):233–241
Asante KO, Macuacua RD, Artan GA, Lietzow RW, Verdin JP (2007) Developing a flood monitoring system from remotely sensed data for the limpopo basin. IEEE Trans Geosci Remote Sens 45(6):1709–1714
Asfaw B, Gilbert WH, Beyene Y, Hart WK, Renne PR, WoldeGabriel G, Vrba ES, White TD (2002) Remains of Homo erectus from Bouri, Middle Awash. Ethiopia. Nature 416(6878):317–320
Assefa A, Melesse AM Admasu S (2014) Climate change in Upper Gilgel Abay river catchment, blue Nile basin Ethiopia. In: Melesse AM, Abtew W, Setegn S (Eds) Nile river basin: ecohydrological challenges, climate change and hydropolitics. pp 363–388
Behulu F, Setegn S, Melesse AM, Fiori A (2013) Hydrological analysis of the Upper Tiber Basin: a watershed modeling approach. Hydrol Process 27(16):2339–2351
Behulu F, Setegn S, Melesse AM, Romano E, Fiori A (2014) Impact of climate change on the hydrology of Upper Tiber River basin using bias corrected regional climate model. Water Res Manage 1–17
Bono R, Seiler W (1983) The soil of the Suke-Hararge Research Unit (Ethiopia) classification, morphology and ecology with soil scale of 1: 5000. Soil Conservation Research Project. University of Bern, University of Switzeralnd and The United Nations University, Tokyo
Christensen JH, Hewitson B, Busuioc A, Chen A, Gao X, Held I, Jones R, Kolli RK, Kwon WT, Laprise R, Magãna Rueda V, Mearns L, Meńendez CG, Räisänen J, Rinke A, Sarr A, Whetton P (2007) Regional climate projections. In: Solomon S, Qin D, Manning M, Chen Z, Marquis M, Averyt KB, Tignor M, Miller HL (eds) Climate change: the physical science basis, contribution of working group I to the fourth assessment report of the intergovernmental panel on climate change. Cambridge University Press, Cambridge
Collier P, Conway G, Venables T (2008) Climate change and Africa. Oxford Review of Econ Policy 24(2):337–353. doi:10.1093/oxrep/grn019
Cunge JA (1969) On the subject of a flood propagation computation method (Muskingum Method). J Hydraul Res 7(2):205–230
Dessu SB, Melesse AM (2012) Modelling the rainfall–runoff process of the Mara River basin using the soil and water assessment tool. Hydrol Process 26(26):4038–4049. doi:10.1002/hyp.9205
Dessu SB, Melesse AM (2013a) Evaluation and comparison of satellite and GCMs rainfall estimates for the mara river basin, kenya/tanzania. In: Younos T, Grady CA (eds) Climate change and water resources. The handbook of environmental chemistry. Springer, Berlin. doi:10.1007/698_2013_219
Dessu SB, Melesse AM (2013b) Impact and uncertainties of climate change on the hydrology of the Mara River basin. Kenya/Tanzania. Hydrol Process 27(20):2973–2986
Dessu SB, Melesse AM, Bhat MG, McClain ME (2014) Assessment of water resources availability and demand in the Mara River Basin. CATENA 115:104–114
DPPC (1997) Food vulnerability in Ethiopia and needs for preparedness. Disaster Prevention and Prepardness Commission, Addis Ababa
Edossa D, Babel M, Das Gupta A (2010) Drought analysis in the Awash River Basin, Ethiopia. Water Res Manage 24(7):1441–1460
Funk C, Michaelsen J, Verdin J, Artan G, Husak G, Senay G, Gadain H, Magadazire T (2003) The collaborative historical African rainfall model: description and evaluation. Int J Climatol 23(1):47–66
Getachew HE, Melesse AM (2012) Impact of land use/land cover change on the hydrology of Angereb watershed, Ethiopia. Int J Water Sci doi: 10.5772/56266, Vol. 1, 4:1-7
Getahun A (1978) Agricultural systems in Ethiopia. Agric Syst 3(4):281–293
Grey OP, Webber Dale G, Setegn SG, Melesse AM (2013) Application of the soil and water assessment tool (SWAT Model) on a small tropical Island state (great river watershed, Jamaica) as a tool in integrated watershed and coastal zone management. Int J Trop Biol Conserv 62(3):293–305
Haile-Selassie Y (2001) Late miocene hominids from the middle Awash, Ethiopia. Nature 412(6843):178–181
IGBP-DIS (2000) Global soil data products (compact disc). International geosphere-biosphere program, data and information services
IPCC (2007) Climate change 2007: the physical science basis. Contribution of working group i to the fourth assessment report of the intergovernmental panel on climate change. In: Solomon SD, Qin M, Manning Z, Chen M, Marquis KB, Averyt M, Tignor HL, Miller (ed) Cambridge University Press, Cambridge
Johanson D, Edey M (1990) Lucy: the beginnings of humankind. Touchstone Books
Jutzi S (1988) Deep black clay soils (Vertisols): management options for the Ethiopian highlands. Mountain research and development 153–156
Kim NW, Lee J (2010) Enhancement of the channel routing module in SWAT. Hydrol Process 24(1):96–107
Mango L, Melesse AM, McClain ME, Gann D, Setegn SG (2011a) Land use and climate change impacts on the hydrology of the Upper Mara River Basin, Kenya: results of a modeling study to support better resource management, special issue: climate, weather and hydrology of east African highlands. Hydrol Earth Syst Sci 15:2245–2258. doi:10.5194/hess-15-2245-2011
Mango L, Melesse AM, McClain ME, Gann D, Setegn SG (2011b) Hydro-meteorology and water budget of mara River basin, Kenya: a land use change scenarios analysis, In: Melesse A (ed) Nile river basin: hydrology, climate and water use (Chap. 2). Springer Science Publisher, pp 39-68, doi: 10.1007/978-94-007-0689-7_2
Mohammed H, Alamirew T, Assen M, Melesse AM (2015) Modeling of sediment yield in maybar gauged watershed using SWAT, northeast Ethiopia. CATENA 127:191–205
Mati BM, Mutie S, Gadain H, Home P, Mtalo F (2008) Impacts of land-use/cover change on the hydrology of the Transboundary mara river, Kenya/Tanzania. Lakes Reservoirs Res Manage 13:169–177
Moreda F, Bauwens W (1998) Influences of variability of rainfall on flow regimes in central Ethiopia. IAHS publication, pp 297–306
Munzimi Y, Hansen MC, Asante KO (2010) Congo basin streamflow characterization using multi-source satellite-derived data: preliminary results. In: AGU Fall Meeting Abstracts, p 1283
Nash JE, Sutcliffe JV (1970) River flow forecasting through conceptual models part I—a discussion of principles. J Hydrol 10(3):282–290
Paulos S (1998) Water release rules for Koka Reservoire for wet seasons. Addis Ababa University, Addis Ababa
Rogner M (2000) Benefit cost consideration for inter-basin water transfers between the middle Awash River basin and the Upper Rift Valley in Ethiopia. Darmstadt University of Technology, Darmstadt
Setegn SG, Melesse AM, Haiduk A, Webber D, Wang X, McClain M (2014) Spatiotemporal distribution of fresh water availability in the Rio Cobre Watershed. Jamaica CATENA 120:81–90
Shrestha MS (2011) Bias-adjustment of satellite-based rainfall estimates over the central Himalayas of Nepal for flood prediction. A Dissertation for the Degree of Doctor of Engineering Department of Civil and Earth Resources Engineering, Kyoto University, Japan
Taddese G, Sonder K, Peden D (2003) The water of the Awash River Basin: a future challenge to Ethiopia. ILRI, Addis Ababa
USDA-SCS (1972) National engineering handbook, hydrology section 4. USDA
Wagener T (2003) Evaluation of catchment models. Hydrol Process 17(16):3375–3378
Wang X, Shang S, Yang W, Melesse AM (2008a) Simulation of an agricultural watershed using an improved curve number method in SWAT. Tans Am Soc Agri Bio Engineers 51(4):1323–1339
Wang X, Yang W, Melesse AM (2008b) Using hydrologic equivalent wetland concept within SWAT to estimate streamflow in watersheds with numerous wetlands. Tans Am Soc Agri Bio Engineers 51(1):55–72
Wang X, Melesse AM, Yang W (2006) Influences of potential evapotranspiration estimation methods on SWAT’s hydrologic simulation in a Northwestern Minnesota Watershed. Trans ASAE 49(6):1755–1771
Wang X, Melesse AM (2006) Effects of STATSGO and SSURGO as inputs on SWAT model’s snowmelt simulation. J Am Water Res Assoc 42(5):1217–1236
Wang X, Melesse AM (2005) Evaluations of the SWAT model’s snowmelt hydrology in a Northwestern Minnesota Watershed. Trans ASAE 48(4):1359–1376
Wang X, Garza J, Whitney M, Melesse AM, Yang W (2008c) Prediction of sediment source areas within watersheds as affected by soil data resolution. In: Paul N (ed) Environmental modelling: new research (Findley; ISBN: 978-1-60692-034-3), Ch. 7, p 151–185, Nova science publishers, Inc., Hauppauge, NY 11788
White KL, Chaubey I (2005) Sensitivity analysis, calibration, and validation for a multisite and multivariable SWAT Model. JAWRA J Am Water Res Assoc 41(5):1077–1089
Yanda PZ, Mubaya CP (2011) Managing a changing climate in Africa, local level vulnerabilities and adaptation experiences. Mkuki Na Nyota, Dar-Es-Salaam
Acknowledgment
This work was supported by the Ethiopian Ministry of Water Resources, Addis Ababa University, the U.S. Agency for International Development and the U.S. Geological Survey.
Author information
Authors and Affiliations
Corresponding author
Editor information
Editors and Affiliations
Rights and permissions
Copyright information
© 2016 Springer International Publishing Switzerland
About this chapter
Cite this chapter
Dessu, S.B., Seid, A.H., Abiy, A.Z., Melesse, A.M. (2016). Flood Forecasting and Stream Flow Simulation of the Upper Awash River Basin, Ethiopia Using Geospatial Stream Flow Model (GeoSFM). In: Melesse, A., Abtew, W. (eds) Landscape Dynamics, Soils and Hydrological Processes in Varied Climates. Springer Geography. Springer, Cham. https://doi.org/10.1007/978-3-319-18787-7_18
Download citation
DOI: https://doi.org/10.1007/978-3-319-18787-7_18
Published:
Publisher Name: Springer, Cham
Print ISBN: 978-3-319-18786-0
Online ISBN: 978-3-319-18787-7
eBook Packages: Earth and Environmental ScienceEarth and Environmental Science (R0)