Abstract
The study of hydrological response to long-term climate changes is a particularly significant problem and an important task for the applied hydrology. This topic is also a challenge for geography. There are many different methods and criteria for the determination of this hydro-climatic relationship. The aim of present work is to explore one of these approaches: climate elasticity of streamflow (\( \varepsilon_{p} \)). As a case region, the territory of seven catchment areas situated in Northwest Bulgaria was selected. Results obtained show relatively large variations of \( \varepsilon_{p} \)—it ranges from 0.526 to 1.404, thus a ten percent change in mean annual precipitation would be reflected within 5.26–14.04% change in mean annual flow. The calculations in this paper establish strong inverse correlations between \( \varepsilon_{p} \), runoff coefficient and mean annual streamflow (a coefficient of determination: R2 > 0.80), explained by the nonlinear “rainfall–runoff” relationships. There are also spatial variations of the \( \varepsilon_{p} \) value—it is lower in the upper streams and increases toward the mouths of the rivers. The assessment of climate elasticity of streamflow is an informative approach for an estimate of climate change impacts to hydrological systems and provides an opportunity for effective water resources management.
Access provided by Autonomous University of Puebla. Download chapter PDF
Similar content being viewed by others
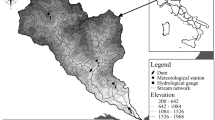
Keywords
Introduction
Climate changes represent one of the most important challenges for the functioning of environmental processes. The hydrological cycle is no exception because it is closely related to the climate system. Any change in climatic parameters has direct or indirect hydrological effects (Zaharia et al. 2018). Estimates of the sensitivity of streamflow to long-term climate changes are required for different water planning and water management activities. This study is prompted by the need to extend these assessments in the context of already established global and regional rainfall changes and increasing air temperature trends. Furthermore, numerous research of the hydrological response to long-term climate changes, including those describing the hydro-climatic relationships, are theoretical (model-based): Schaake and Němec (1992) used the Sacramento model; Yates et al. (1998), (1999) worked by the linear regression coefficients; Chiew and McMahon (2002), Chiew (2006) used the SIMHYD and AWBM models, Gelfan et al. (2015) worked by the ECOMAG and SWAP models; Guo et al. (2016)—SWAT model, etc. Excluding the hydrological models, sometimes showing uncertain results, an alternative approach to estimate the runoff sensitivity is directly from the hydro-climatic data set. A similar empirical (data-based) method is a climate (precipitation) elasticity of streamflow (Sankarasubramanian and Vogel 2001; Chiew 2006; Chiew et al. 2006; Fu et al. 2007, 2011; Sun et al. 2013; Allaire et al. 2015; Tsai 2017; Andréassian et al. 2017; Xing et al. 2018; Hristova et al. 2018). The primary goal of present study is to explore this approach for seven catchments in Northwest Bulgaria, using a nonparametric estimator which calculates the elasticity values directly from the rainfall and runoff time series data.
Study Area
Study area includes the drainage basins of seven right tributaries of the Danube River, situated in Northwest Bulgaria. This region is located between 43° 24′–44° 04′ N latitude and 22° 21′–23° 32′ E longitude, it covers an area of 3790.5 km2 (3.4% of the country’s territory) (Fig. 1, Table 1).
Relief, hydrographic, and hydrometric features in study area (Hristova et al. 2017)
The catchment areas drain in three geomorphological units: the Balkan Mountains, the Fore-Balkans, and the Danube Plain. Study area belongs to the temperate-continental climate type (DFA according to Köppen classification). Mean annual air temperature, measured during the 1961–2015 period, varies from 4.2 °C (in the Chuprene Reserve area) to 11.6 °C (in the town of Lom). Average precipitation sums for the 1960–2017 period, vary from 538 mm (Lom) to 773 mm (Stakevtsi) and reach up to 1200 mm in the Balkan Mountains. Study area is an important agricultural region (cereal and technical crops: wheat, barley, corn, and sunflower production; perennial vineyards cultivation). There are over 50 small reservoirs and a hydropower cascade, located in the upstream of the Lom River. Listed activities reflect the favorable soil-climatic conditions and the environmental transformations due to human impact in this area.
Data and Methods
Annual discharge data provided by the National Institute of Meteorology and Hydrology are used. There are seven hydrometric stations, covering areas from 53 to 1087 km2, at the mean elevation 193–1411 m above sea level (Hydrological Reference…, 1981; Hristova et al. 2017). Precipitation output data from seven meteorological stations within the same catchments or up to 10 km near them for correlations with streamflow are used (Table 3). The length of time series used critically influences the estimation of the climate elasticity. It is recommended to use as long a record as possible to estimate elasticity in order to capture the full range of observed variability. Hydro-climatic output data for 58 years, recorded during the 1960–2017 period are selected.
Climate elasticity of streamflow, also called “precipitation elasticity” or “streamflow sensitivity factor”, is an indicator for the sensitivity of hydrological systems to the long-term climate fluctuations. It is based on a conceptual catchment model and represents a proportional change of annual streamflow (Q) to precipitation variability (P) (Sankarasubramanian and Vogel 2001; Chiew 2006; Chiew et al. 2006; Fu et al. 2007, 2011; Allaire et al. 2015; Gao et al. 2016; Andréassian et al. 2017). This relation is calculated by the formula
transformed into the nonparametric estimator (Sankarasubramanian and Vogel 2001):
where \( \varepsilon_{p} \) is a climate elasticity, \( \bar{P} \) and \( \bar{Q} \) are mean annual precipitation and streamflow for the whole period, \( P_{i} \) and \( Q_{i } \) are annual precipitation and streamflow for the ith year.
To compute climate elasticity estimates, the output hydro-climatic data was calculated for each pair of annual time series (Pi, Qi). As the nonparametric estimate of \( \varepsilon_{p} \), the median value of annual data set obtained was defined. The statistical results established were mapped in the software program ArcGIS 10.4, using the KRIGING interpolation technique (already applied and recommended as a reliable approach in similar studies) (Khanal et al. 2014; Gao et al. 2016). They are grouped in equal classes by a color legend, according to their values.
Results and Discussions
To achieve more objective researches on the hydrological response to rainfall changes, the streamflow and precipitation time series were computed for every possible 12-month annual periods. Table 2 presents the coefficient of determination (R2) of the “Qi (mm) − Pi” relationship (for each pair of annual data), it also shows the corresponding \( \varepsilon_{p} \) values. To define the most reliable \( \varepsilon_{p} \) for three catchments in the United States, China, and Australia this coefficient was used, because of “the time-series having the strongest precipitation-streamflow relationship, i.e., the highest coefficient of determination, is inferred as producing a more appropriate elasticity value” (Fu et al. 2011). The results surprisingly show: the highest R2 value coincides with the calendar year. Exceptions are the catchments of the Salashka River, Stakevska River, and Lom River (at Gorni Lom), where it approximates the water year accepted in Bulgaria and many countries across the world, respectively (Table 2). These fragments contradict the diverse results obtained from Fu et al. (2011), but listed numbers confirm their statement that the \( \varepsilon_{p} \) values are higher in annual cycles, starting from the dry season. This fact allows implement of a seasonal approach in the climate elasticity of streamflow studies and needs more research. Henceforth, only the annual time series having the largest coefficient of determination will be shown here.
Results obtained show significant variations of \( \varepsilon_{p} \) value—it ranges within 0.526–1.404, therefore indicating that an eventually ten percent change in mean annual precipitation would be reflected as increase/decrease from 5.26 to 14.04% in mean annual streamflow (Table 3). Some of the abovementioned values are slightly higher than the climate elasticity results established from Hristova et al. (2018) for the entire Bulgarian territory and are partly similar or slightly higher/lower to the calculated numbers for 1337 drainage basins in the United States, for above 200 catchments in the middle and high latitudes of the Northern Hemisphere (chiefly in the territories of Europe and Northern America), for 519 drainage basins in France and for 79 catchments in the Poyang Lake basin in China (Sankarasubramanian and Vogel 2001; Chiew et al. 2006; Sun et al. 2013, Andréassian et al. 2017). Furthermore, the long-term climate forecasts, the optimistic (RCP 4.5) and the pessimistic (RCP 8.5) scenarios predict a change of annual streamflow from −8.0 to 9.0% in study area for 2071–2100, compared to the 1976–2005 period, according to the “National Plan for river basins… (2016–2021)” at the Danube River basin Directorate. The computed \( \varepsilon_{p} \) values nearly approximated these numbers.
The results in this paper confirm the following characteristics: relatively strong inverse correlations: “\( \varepsilon_{p} \)—runoff coefficient” and “\( \varepsilon_{p} \)—mean annual streamflow (mm)” (Fig. 2).
This specificity is explained by the fact that “rainfall–runoff” processes are nonlinear, suggesting: “the absolute streamflow change for a given absolute change in rainfall would be reflected as a higher \( \varepsilon_{p} \) in catchments with a lower runoff coefficient” (Chiew 2006). Results obtained contradict the especially clear inverse relationship “\( \varepsilon_{p} \)—mean annual precipitation” (R2 = 0.6711; the graphic is not shown here), even proven as a stronger than the correlation between \( \varepsilon_{p} \) and mean annual streamflow (mm) for 219 catchments in Australia (Chiew 2006). There is a limited number of available rainfall stations—it could be a possible reason explaining the weaker correlation. Despite the abovementioned contradictions, considering all analyzed “precipitation–streamflow” relationships, it could be summarized that they are so powerful because of the direct response of runoff to rainfall. Some multivariate regression relationships, arising from the hydro-climatic correlations, e.g., “\( \varepsilon_{p} \) vs. mean annual temperature, rainfall, and streamflow (mm)”, show the following: “the climate elasticity value increases with the temperature and streamflow if the precipitation decreases, but it decreases with the temperature and streamflow if the precipitation increases” (Fu et al. 2007). Although there are not used annual temperature data, the hydro-climatic conditions in the investigated area indirectly confirm this statement.
The calculations in present work establish spatial variations of the climate elasticity between the mountainous and flat part of studied catchments (an inverse relationship “\( \varepsilon_{p} \) vs. the altitude of catchments”; R2 = 0.7754). The geographical analysis shows the elasticity is lower in the upper streams and increases toward the mouths of the rivers (Fig. 3). Similar peculiarities were described for drainage basins in the United States and Australia, where the sources of the rivers belong to humid climate type with long snow cover duration, while the downstream sections flows through semiarid desert landscapes (Sankarasubramanian and Vogel 2001; Chiew et al. 2006). Although the hydrological processes in Northwest Bulgaria occur at different climate, geological, geomorphological, and biogeographical conditions, the results establish analogous spatial characteristics. Further researches explaining this phenomenon are needed.
Conclusion
The main findings of present work are the following: (1) the climate elasticity (\( \varepsilon_{p} \)) values are related to the coefficient of determination (R2) of the “rainfall–runoff” annual time series. The nonparametric estimator is computed for calendar or water years, according to the highest R2 value; (2) a ten percent change in mean annual precipitation would be reflected within 5–14% change in mean annual flow; (3) there are strong inverse correlations between the \( \varepsilon_{p} \), runoff coefficient and annual streamflow, due to the nonlinear hydro-climatic processes; (4) there are spatial differences of the \( \varepsilon_{p} \) value—it is lower in the upper streams and increasing toward the mouths of the rivers. This paper presents the climate elasticity of streamflow (\( \varepsilon_{p} \)) as an informative and easy to use approach for an estimate of hydrological response to long-term rainfall changes. In future, this research could be extended with other climatic parameters for more in-depth results, e.g., mean annual air temperature, potential evapotranspiration (PET), humidity index, etc., and their impacts on runoff.
References
Allaire M, Vogel R, Kroll C (2015) The hydromorphology of an urbanizing watershed using multivariate elasticity. Adv. Water Resour 86(Part A):147–154
Andréassian V, Coron L, Lerat J, Moine N (2017) Climate elasticity of streamflow revisited—an elasticity index based on long-term hydrometeorological records. Hydrol Earth Sci 4503–4524
Chiew F (2006) Estimation of rainfall elasticity of streamflow in Australia. Hydrol Sci J des Sci Hydrol 51, Book 4, 613–625
Chiew F, McMahon T (2002) Modelling the impacts of climate change on Australian streamflow. Hydrol Process (16):1235–1245
Chiew F, Peel M, McMahon T, Siriwardena L (2006) Precipitation elasticity of streamflow in catchments across the world. In: Climate variability and changes—hydrological impacts. IAHS, Publ. vol 308, 256–262 pp
Fu G, Charles S, Chiew F (2007) A two-parameter climate elasticity of streamflow index to assess climate change effects on annual streamflow. Water Resour 43(11)
Fu G, Chiew F, Charles S, Mpelasoka F (2011) Assessing precipitation elasticity of streamflow based on the strength of the precipitation—streamflow relationship. In: Book of 19th international congress on modelling and simulation, Perth, Australia, 3567–3572 pp, 12–16 Dec 2011
Gao G, Fu B, Wang S, Liang W, Jiang X (2016) Determining the hydrological response to climate variability and land use/cover change in the Loess Plateau with the Budyko framework. Sci Total Environ (557–558):331–342
Gelfan A, Semenov V, Gusev E, Motovilov Y, Nasonova O, Krylenko I, Kovalev E (2015) Large basin hydrological response to climate model outputs: uncertainty caused by internal atmospheric variability. Hydrol Earth Syst Sci 19:2737–2754
Guo J, Su X, Singh V, Jin J (2016) Impacts of climate and land use/cover change on streamflow using SWAT and a separation method for the Xinyang river basin. Water 8:1–14
Hristova N (2012) Hydrology of Bulgaria. Tip-Top Press, 832 pp
Hristova N, Ivanova E, Seymenov K (2017) Geographical aspects of floods in Northwest Bulgaria. Int Knowl J IF: 1.023 16.2:907–915
Hristova N, Penkov I, Seymenov K (2018) Fluctuations and climate elasticity of annual streamflow in Bulgaria. Geography. Annual Book of Sofia University, vol 111, Book 2, 63–73 pp
Khanal S, Anex RP, Anderson CJ, Herzmann DE (2014) Streamflow impacts of biofuel policy-driven landscape change. Clim Chang Mitig 9(10):209–230
Sankarasubramanian A, Vogel R (2001) Climate elasticity of streamflow in the United States. Water Resour Res 37(6):1771–1781
Schaake J, Němec J (1992) Sensitivity of water resource systems to climate variation. Hydrol Sci J des Sci Hydrol 27(3):327–343
Sun S, Chen H, Ju W, Song J, Zhang H, Sun J, Fang Y (2013) Effects of climate change on annual streamflow using climate elasticity in Poyang Lake Basin, China. Theor Appl Climatol 122(1–2):169–183
Tsai Y (2017) Multivariate climate and anthropogenic elasticity of streamflow in the Eastern United States. J Hydrol: Reg Stud 9:199–215
Vogel R, Wilson I, Daly C (1999) Regional regression models of annual streamflow for the United States. J Irrig Drain Eng 125:148–157
Yates D, Strzepek K (1998) Modelling the Nile River basin under climatic change. J Hydrol Eng (3):98–108
Xing W, Wang W, Zou S, Deng C (2018) Projection of future runoff change using climate elasticity method derived from Budyko framework in major basins across China. Glob Planet Chang 162:120–135
Zaharia L, Perju R, Toroimac G (2018) Climate changes and effects on river flow in the Romanian Carpathians. In: Book of tenth international scientific conference “air and water components of the environment”, Cluj-Napoca, Romania, 211–218 pp
xxx Hydrological Reference Book of the rivers in the Republic of Bulgaria (1981) GUHM–BAS
xxx National Plan for river basin management (2016) Danube directorate. www.bd-dunav.org
Author information
Authors and Affiliations
Corresponding author
Editor information
Editors and Affiliations
Rights and permissions
Copyright information
© 2020 Springer Nature Switzerland AG
About this chapter
Cite this chapter
Seymenov, K. (2020). Climate Elasticity of Annual Streamflow in Northwest Bulgaria. In: Nedkov, S., et al. Smart Geography. Key Challenges in Geography. Springer, Cham. https://doi.org/10.1007/978-3-030-28191-5_9
Download citation
DOI: https://doi.org/10.1007/978-3-030-28191-5_9
Published:
Publisher Name: Springer, Cham
Print ISBN: 978-3-030-28190-8
Online ISBN: 978-3-030-28191-5
eBook Packages: Earth and Environmental ScienceEarth and Environmental Science (R0)