Abstract
The development of artificial biocrust using cyanobacterium Phormidium tenue has been suggested as an effective strategy to prevent soil degradation. Here, a combination of in silico approaches with growth rate, photosynthetic pigment, morphology, and transcript analysis was used to identify specific genes and their protein products in response to 500 mM NaCl in P. tenue. The results show that 500 mM NaCl induces the expression of genes encoding glycerol-3-phosphate dehydrogenase (glpD) as a Flavoprotein, ribosomal protein S12 methylthiotransferase (rimO), and a hypothetical protein (sll0939). The constructed co-expression network revealed a group of abiotic stress-responsive genes. Using the Basic Local Alignment Search Tool (BLAST), the homologous proteins of rimO, glpD, and sll0939 were identified in the P. tenue genome. Encoded proteins of glpD, rimO, and DUF1622 genes, respectively, contain (DAO and DAO C), (UPF0004, Radical SAM and TRAM 2), and (DUF1622) domains. The predicted ligand included 22B and MG for DUF1622, FS5 for rimO, and FAD for glpD protein. There was no direct disruption in ligand-binding sites of these proteins by Na+, Cl−, or NaCl. The growth rate, photosynthetic pigment, and morphology of P. tenue were investigated, and the result showed an acceptable tolerance rate of this microorganism under salt stress. The quantitative real-time polymerase chain reaction (qRT-PCR) results revealed the up-regulation of glpD, rimO, and DUF1622 genes under salt stress. This is the first report on computational and experimental analyses of the glpD, rimO, and DUF1622 genes in P. tenue under salt stress to the best of our knowledge.
Similar content being viewed by others
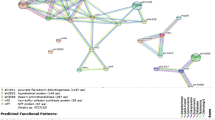
Avoid common mistakes on your manuscript.
Introduction
Abiotic stresses are one of the main concerns of the agricultural industry and one of the most important reasons for the decline in the annual production of agricultural products (Yamazaki et al. 2020). These stresses, including drought, salinity, excessive concentrations of heavy metal, heat, and cold, affect crops and soil quality, leading to reduced agricultural yields (Goyal et al. 2020; Nagarajan and Nagarajan 2009; Parmar et al. 2017; Yamazaki et al. 2020). Investigation on the effect of high concentrations of NaCl on plant growth/development and soil quality is a long-standing research topic (Khator and Shekhawat 2020; Yadav et al. 2020). NaCl leads to ionic, osmotic, and oxidative stresses within the plant cell (Borsani et al. 2001; Lan et al. 2020). Overproduction of reactive oxygen species (ROS) and loss of photosynthetic potential at different stages of plant growth due to salinity stress can lead to a sharp decrease in biomass production and an increase in cellular senescence, and also prevent normal cell growth (Allu et al. 2014; Liang et al. 2020; Nawaz et al. 2010; Yang and Guo 2018). On the other hand, from a molecular point of view, previous studies reveal the detrimental effects of high concentrations of NaCl on protein functionality and stability (Büdel et al. 2016; Coleman and Lee 2004; Cramer et al. 2011; de Souza Silva and Fay 2012; Doi 2019; Gião and Keevil 2014; Ishida et al. 2009; Maestre et al. 2017; Mugnai et al. 2018). It has been demonstrated that high concentrations of NaCl lead to reduced protein activity (Khalid et al. 2003; Namwong et al. 2006). Soil quality control is one of the main pillars of sustainable agriculture (Doran and Parkin 1994; Lynn et al., 2021; Mukherjee et al. 2021). The main factors reducing soil quality are soil erosion, acidification, salinization, each of which will be addressed. These stresses lead to fundamental changes in soil mineral components, soil structure, soil texture, water holding capacity, and soil pH (Fageria and Baligar 2008; Obalum et al. 2017; Smith et al. 2016). Among the aforesaid cases, soil salinization is one of the main problems facing agriculture, which leads to a sharp decline in soil quality. The salinized soils are categorized into three main parts: saline (do not contain excess exchangeable Na+), sodic and saline-sodic soils (both soluble salts and exchangeable Na+ are high) (Osman 2018; Qadir et al. 2001). Reducing soil salinization through salt leaching or improved agricultural practices are well known; however, most of these techniques are cost-intensive (Rocha et al. 2020). Putting together, choosing a strategy that leads to improved soil quality, improved plant growth/development, and reduced cost of cultivation is an interesting subject in agricultural management. Thus, it is crucial to develop efficient strategies to dampen the salt stress effects on cell normal metabolism (Khodadadi et al. 2020; Langridge and Fleury 2011; Rezaei Qusheh Bolagh et al. 2020). The development of artificial biocrusts is an efficient and innovative biotechnological tool for improving soil quality and reducing salt stress effects (Kakeh et al. 2020). This can be effective in soil stabilization, nutrient and carbon cycling, wind and water erosion prevention, and soil fertility improvement. Among the aforesaid abnormal situations which eventually influence soil quality, soil salinity could be prevented by developing biocrust by minimizing water evaporation (Kakeh et al. 2021). The biocrust layer of the soil contains lichens, mosses, and microorganisms such as cyanobacteria (Lázaro et al. 2008; West 1990; Wu et al. 2013). As the first dryland microorganisms, cyanobacteria play a vital role in primary soil stabilization (Belnap et al. 2016). The main challenge in developing biocrusts is to characterize species that can survive in the long term under stressful conditions. Extremely and moderately halophilic bacteria dominate in saline environments (0.5% to saturated NaCl). They have developed an efficient metabolism for the utilization of proteins and amino acids, an interesting field of research for further biotechnological applications (Lanyi 1974; Reed et al. 2013). P. tenue is a filamentous cyanobacterium that belongs to the Oscillatoriales order, living in the first top millimeters of the soil. It has shown tolerance under salinity stress and is a potential microorganism in producing artificial biocrusts (Bowker et al. 2018; Hagemann 2011; Klähn and Hagemann 2011; Tonk et al. 2007).
Previous studies on artificial biocrust production mainly focus on the soil quality and features during the different stages of biocrust formation (Cruz de Carvalho et al. 2018; Roncero-Ramos et al. 2019). This study aimed to characterize the salt tolerance mechanism of P. tenue from a molecular point of view by focusing on transcript pattern and structural bioinformatics to facilitate the genome editing of this microorganism. Although there is a lack of transcriptome data for non-model microorganisms, in silico data analysis can fill this gap (Sahoo et al. 2020).
Considering the numerous components of cell metabolism, boosting the potential ability of the cell might be performed from different points of view, such as balancing the energy metabolism, bio-synthesizing functional proteins, enzymes, etc. (Fasani et al. 2018; Hug et al. 2020; Liang et al. 2021). Characterizing the expression pattern, amino acid sequences, ligand-binding poses, and molecular docking with different stressors will reduce the complexity of the molecular and biochemical activity of the target proteins for further investigation (Basak et al. 2020; Jugder et al. 2016; Kharwar et al. 2021; Lu and Cheng 2021; Mathpal et al. 2018; Oshone et al. 2017; Punjabi et al. 2018; Wan et al. 2004). To achieve this goal, with minimal costly experimental procedures, introducing strategies in combining genomic, transcriptomic, and structural proteomic data shed light on genome editing of organisms and microorganisms for further industrial applications (Arora et al. 2021; Bhardwaj et al 2018, 2017, 2021; Hindré et al. 2012; Mallet 2012; Naraian 2019; Phatak et al. 2009). In this regard, the present study used a combination of in silico approaches to further characterize some of the main stress-responsive genes in P. tenue, which pave the way for future genome editing of this microorganism.
Herein, investigating transcriptome patterns along with structural proteomics, qRT-PCR, growth pattern, and cellular morphological characteristics demonstrated the up-regulation of DUF1622, glpD, and rimO under salt stress. The genes mentioned above were involved in a stress-responsive gene co-expression network. Structural proteomics approaches demonstrated no competition between stressors and native protein ligands. Finally, a low rate of cell depigmentation reveals the balanced metabolism of P. tenue to survive under salt stress.
Materials and methods
Computational methods
The microarray dataset, with GSE37482 ID, previously was created to investigate the effect of 500 mM NaCl on cyanobacterium Synechocystis sp. PCC 6803 (Dickson et al. 2012). This dataset was retrieved from the NCBI GEO database, pre-processed, normalized, and analyzed using the R programming language by the LIMMA package (Ritchie et al. 2015). Among the upregulated genes, glpD (SGL_RS11080), rimO (SGL_RS15315), and sll0939 (SGL_RS03405) were selected for the rest of the study due to their involvement in some of the major biochemical pathways in response to NaCl stress. As there is no transcriptome data for P. tenue, the co-expression network related to glpD, rimO, and sll0939 genes was constructed by the CyanoEXpress database specific to transcriptome data of Synechocystis sp. PCC 6803 (Hernandez-Prieto and Futschik 2012) Although evolutionary distances between two species greatly influence the results, some conserved sub-co-expression networks may be considered. The amino-acid sequence of glpD (WP_010872971.1), rimO (WP_010873783.1), and sll0939 (WP_010871504.1) proteins from cyanobacterium Synechocystis sp. PCC 6803 was aligned against cyanobacteria P. tenue proteins with BLOSUM62 matrix using the UniProt database. (Consortium 2019). After alignment, the most similar sequences were selected for further studies. The conserved domain of homologous proteins in P. tenue with WP_139296916.1 (homologous to rimO), WP_073607160.1 (homologous to glpD), WP_073608523.1 (homologous to sll0939) accession numbers was predicted by NCBI, conserved domain database (CDD) server v3.18, with an expected threshold value of 0.01. (Lu et al. 2020). Protein modelling and docking were performed for the predicted glpD, rimO, and sll0939 homologous in P. tenue. Protein modelling was performed using trRosetta. (Yang et al. 2020). Structural reliability was calculated using the Ramachandran plot, ERRAT, ModEval, QMEAN, and trRosetta web servers (Colovos and Yeates 1993; Eramian et al. 2008; Laskowski et al. 2005; Mahdavi et al. 2020; Shen and Sali 2006). Since the protein structure of rimO (WP 139296916.1) from P. tenue already existed in the Swiss model database (A0A1U7J8T6 9CYAN), the overall and residue-wise Root-Mean-Squared Deviation (RMSD) values were calculated with reference to the above-mentioned protein structure of rimO, using the Visualization Molecular Dynamics (VMD) software package on the backbone atoms of the selected amino acids. The protein model of rimO (WP 139296916.1) in the Swiss model database (A0A1U7J8T6 9CYAN) was built using homology modeling and the template used for this modeling was protein structure with 4jc0.1.A PDB ID. It is noteworthy that the structure of the rimO protein was more regressively obtained here, using various servers, to calculate the reliability of the modeled structure, and then it was compared with the existing model in the Swiss model data base.
The prediction of potential ligand-binding sites, plausible ligands, and docking of Na+, Cl−, and NaCl to the modelled structures were performed by COACH-D and Hex (Macindoe et al. 2010; Yang et al 2012, 2013). The structures were visualized using Python-Based Molecular Visualization System (PyMOL) and VMD (Humphrey et al. 1996; Schrödinger 2018).
Experimental methods
Cell culture and stress treatment
Cyanobacterium P. tenue was obtained from the Iranian Biological Resource Center. The cells were grown for 15 days in a 250 ml culture shaker flask at 100 rpm at 30 °C, in a BG-11 liquid medium under continuous illumination of 50 μmol of photons m2s−1. The exponentially growing cells were harvested by centrifugation at 5000 g for 5 min and washed twice with sterile distilled water. As the stress condition, pellets were resuspended in fresh BG-11 medium containing 500 mM NaCl for 24 h with three biological replicates. Cells resuspended in the NaCl free BG-11 medium with three biological replicates as the control condition (Allakhverdiev and Murata 2008; Allakhverdiev et al. 2000; Dickson et al. 2012; Murata et al. 2007; Ozturk and Aslim 2010; Vonshak et al. 1988). As P. tenue is a filamentous, photosynthetic cyanobacterium, its growth is directly related to the photosynthetic pigment chlorophyll a. Based on the aforesaid notion, the growth rate of P. tenue was determined with two particular methods, including mass dry weight and chlorophyll-a measurement. To quantify the dry weight, 2 ml of the homogenized culture was centrifuged and rinsed with distilled water. The pellet was dried at 60 °C and weighted for biomass calculation. To determine the chlorophyll a, 1 mL of homogenized culture was centrifuged and, after discarding the supernatant, 1.5 mL of methanol (99%) was added to the pellet, mixed, and immersed in the dark for 24 h at 45 °C. The chlorophyll extract was prepared by centrifugation at 4000 rpm for 10 min. After measuring the optical density (absorbance at wave-length of 665.2 and 652.4, A665.2, and A652.4) of the extract, chl a concentration was determined. The experiment was done every 24 h for 7 days for control cells (0 mM NaCl) and salt-treated cells (500 mM NaCl) with three biological and technical replicates (Jiang et al. 2015; Pei et al. 2017). To analyze the effect of 500 mM NaCl stress on the morphology of P. tenue, 7-day 500 mM NaCl-treated and control sample cells were visualized using the Nikon TS100 Inverted Phase Contrast Microscope.
Primer design and quantitative real-time PCR
The nucleotide sequences of WP_139296916.1 (homologous to rimO), WP_073607160.1 (homologous to glpD), and WP_073608523.1 (homologous to sll0939) were retrieved from NCBI using the tblastn tool. Melting temperature, primer homo-dimer, and hetero-dimer parameters were analyzed by Oligo 7 software (Rychlik 2007). Sequences of primers are shown in Table 1. Total RNA of Cyanobacterium P. tenue was isolated using Trizol (TRI reagent Sigma-Aldrich, USA) besides glass beads for cell wall disruption according to the manufacturer’s protocol. QuantiTect Reverse Transcription Kit (Qiagen, Germany) was used for cDNA synthesis in the total volume of 20 μl reaction according to the manufacturer’s protocol. The qRT-PCR reaction was performed in the total volume of 20 μl containing 10 μl of qRT-PCR GreenMaster (Bio-Rad), 120 ng of cDNA, and 10 pm of each primer in a Rotor-Gene® 6000 (Qiagen, Germany). The qRT-PCR temperature cycling started by 5 min at 95 °C for initial denaturation followed by 40 cycles of 30 s at 95 °C, 30 s at 62 °C, and 15 s at 72 °C. Relative gene expression was calculated using the relative expression software tool (REST). Logarithmic fold changes (LogFCs) were calculated using the comparative 2−ΔΔCt method.
Statistical analysis
One-way analysis of variance (ANOVA) was used in R version 3.6.3. for statistical analysis. The means of treatments were compared with Tukey’s test at P < 0.05. Each data point represented means ± SE (n = 3).
Results
Transcriptome and functional site analysis
Transcriptome data along with GSE37482 ID analysis indicated the up-regulation of glpD (SGL_RS11080), rimO (SGL_RS15315), and sll0939 (SGL_RS03405) genes under 500 mM NaCl stress for 24 h with LogFCs 5.270922, 2.53, 5.27 (Table 2) in Synechocystis sp. PCC 6803. Here, we characterized the main co-expressed genes with glpD (SGL_RS11080), rimO (SGL_RS15315), and sll0939 (SGL_RS03405) genes. The accuracy of the constructed network required further validation. The co-expressed genes with glpD (SGL_RS11080) are involved in glycerol metabolism, stress response, and hypothetical proteins. The co-expressed genes with sll0939 (SGL_RS03405) are hypothetical proteins, stress-responsive proteins, and transcriptional regulators. The co-expressed genes with rimO (SGL_RS15315) are ATP- and GTP-binding proteins, transcriptional regulators, and hypothetical proteins (Table 3). Analyzing the conserved domain of homologues genes in P. tenue reveals that glpD (WP_073607160.1) protein contains conserved (DAO and DAO_C) domains is involved in the metabolic pathway of glycerophosphate, such as oxidoreductase activity. In this pathway, a quinone with sn-glycerol 3-phosphate is converted to a quinol and dihydroxyacetone phosphate by the glpD enzyme (Fig. 1B) (Eungrasamee et al. 2020). The hypothetical protein (WP_073607160.1) contains the DUF1622 domain, which is highly conserved among bacteria and archaea. This domain belongs to the category of DUFs (Mironov et al. 2019; Uchiyama et al. 2012). The rimO (WP_139296916.1) protein possesses the following domains: UPF0004, Radical-SAM, and TRAM-2. As a transferase protein, rimO is involved in the methylation of residue Asp-89 in ribosomal protein S12 (Fig. 1A) (Georg et al. 2019; Mironov et al. 2019).
schematic representation of biochemical pathways related to glpD and rimO proteins. A [sulfur carrier] − SH + AH2 + l-aspartate89- [ribosomal protein uS12]-hydrogen + 2 S-adenosyl-l-methionine = 3-methylsulfanyl-l-aspartate89- [ribosomal protein uS12]-hydrogen + 5′-deoxyadenosine + [sulfur carrier]-H + A + 2 H + + l-methionine + S-adenosyl-l-homocysteine. B A quinone + sn-glycerol 3-phosphate = a quinol + dihydroxyacetone phosphate
Structural modeling and validation:
Structural modelling was conducted using trRosetta and Phyre2. Structural accuracy was validated using the Ramachandran plot, ERRAT, ModEval, and trRosetta web servers. Predicted models and Ramachandran plots for each glpD (WP_073607160.1), rimO (WP_139296916.1), and DUF1622 (WP_073608523.1) protein are shown in (Fig. 2A–C). The confidence interval of all predicted structures in the trRosetta database was very high. Based on the Ramachandran plot, 96%, 91%, 92% of DUF1622 (WP_073608523.1), glpD (WP_073607160.1), and rimO (WP_139296916.1) amino acids were in the most favored regions (Fig. 2D–F). The ERRAT server predicted the overall scores of 93.784, 95.8621, and 100 for glpD (WP_073607160.1), rimO (WP_139296916.1), and DUF1622 (WP_073608523.1), respectively. The analysis of the Chi-plot reveals a good predicted structure for the proteins, where the server described that the predicted structure passed the analyses. The QMEAN score was calculated to be 0.69 ± 0.05, 0.71 ± 0.05, and 0.68 ± 0.07 for glpD (WP_073607160.1), rimO (WP_139296916.1), and DUF1622 (WP_073608523.1) proteins, respectively. Modeller Scoring results are demonstrated in Table 4. The overall RMSD for rimO (WP_139296916.1) proteins was calculated to be 2.3, and the RMSD values for selected amino acids are listed in Table 5. The analysis of the above-mentioned parameters reveals that the predicted structures are reliable for further studies.
The predicted structures for glpD, rimO and sll0939. A, D Predicted structure for glpD with trRosetta database with very high confidence and Ramachandran plot with 96% of amino acids in most favoured region. B, E Predicted structure for rimO with trRosetta database with very high confidence and Ramachandran plot with 92% of amino acids in most favoured region. C, F Predicted structure for sll0939 with trRosetta database with very high confidence and Ramachandran plot with 91% of amino acids in most favoured region
Molecular docking
Native ligands were predicted using COACH-D, which utilizes TM-SITE, S-SITE, COFACTOR, FINDSITE, and ConCavity to predict the ligand-binding pockets and AutoDock Vina as an efficient algorithm for ligand—protein docking. Stressor-proteins docking was carried out using the Hex software, which uses Fourier-transform (FFT) correlation techniques for docking. The predicted ligands include flavin adenine dinucleotide (FAD) for glpD (WP_073607160.1), which participates in redox reactions and the transfer of either one or two electrons, hydrogen atoms, or hydronium ions (Lu et al. 2015). Iron/sulfur pentasulfide-connected clusters (FS5) molecules predicted for rimO (WP_139296916.1) protein that participates in catalyzing sulfur insertion into ribosomal protein S12, which belongs to a subgroup of Radical-S-adenosylmethionine (radical-SAM) enzymes (Forouhar et al. 2013). Finally, Bacterioruberin (22B) and Magnesium (Mg) ligands were predicted for DUF1622 (WP_073608523.1) (Fig. 3). Docking of Na+, Cl−, and NaCl to the DUF1622 (WP_073608523.1), glpD (WP_073607160.1), and rimO (WP_139296916.1) proteins were performed in two steps. The NaCl molecule is soluble in water and breaks up into Na+ and Cl−. Thus, in the first step, the stressors (Na+, Cl−, NaCl) were simultaneously docked into the proteins in the presence of native ligands (FAD, FS5, 22B, and MG) (Fig. 4). Na+, Cl−, and NaCl were then docked separately to identify their binding poses on the DUF1622 (WP_073608523.1), glpD (WP_073607160.1), and rimO (WP_139296916.1) proteins (Fig. 5). The most favourable binding free energies were observed in rimO-FS5 (− 5.0 kcal mol−1), DUF1622-22B (− 3.6 kcal mol−1), and DUF1622-MG (− 1 kcal mol−1), respectively (Fig. 6). However, glpD-FAD-binding presents the least favourable binding free energy (+ 0.3 kcal mol−1). The Na+, Cl−, and NaCl-binding energies to the proteins varied from − 0.8 to − 1.9 kcal mol−1. The binding energies for each protein were different in the presence of Na+, Cl−, and NaCl. The results demonstrate that the FAD-glpD-binding energy increases from + 0.3 to − 1.1 kcal mol−1 when Na+ is added to the complex. On the other hand, a decrease in the FAD-glpD-binding energy was observed from + 0.3 to + 0.6 and + 2.6 kcal mol−1 upon the addition of Cl− and NaCl, respectively. Na+, Cl−, and NaCl do not alter the rimO-FS5-binding energy (− 5 to − 4.8 kcal mol−1). The DUF1622-22B binding energy decreased from − 3.6 to − 2.7, − 2.5, and − 3.4 kcal mol−1 upon the addition of Na+, Cl− and NaCl, respectively. No differences were observed in the MG-DUF1622 binding energy in the presence of Na+ or Cl− and NaCl (Fig. 6). Docking of Na+, Cl− and NaCl to glpD, rimO, and DUF1622 shows no direct overlap in the ligand-binding sites of these proteins. The results demonstrate two Na+ or Cl−-binding poses, with − 46 kcal mol−1 for Na+ and 34 kcal mol−1 for Cl− for the first binding pose and− 48 kcal mol−1 for Na+ and − 41 kcal mol−1 for Cl− for the second binding pose, for the DUF1622 protein. Four binding poses of NaCl were predicted for DUF1622 with an average binding energy of − 83, − 76, − 76.5, and − 90.5 kcal mol−1 for each pose. One Na+-binding pose, one Cl−-binding pose, and three NaCl-binding poses were predicted for the glpD and rimO proteins. The binding energies of Na+ and Cl− to glpD were predicted to be − 88 and − 58 kcal mol−1, respectively. The NaCl-glpD binding energies were − 115, − 111.5, and − 112 kcal mol−1. The binding energies of Na+ and Cl− to rimO were − 63 and − 34 kcal mol−1 for Na+ and Cl−, respectively. The NaCl-rimO-binding energies were − 79, − 77, and − 74.6 kcal mol−1 (Fig. 7). This is a valuable observation as these proteins are upregulated upon salt stress treatment. Showing different ligand-binding epitopes of Na+, Cl−, and NaCl that differs from the native ligand-binding sites is supportive of their proper activity upon salt stress treatment, which ensures the survival of the organism. Investigating the detailed structural interactions of NaCl with all the three proteins shows that the Cl− forms electrostatic interactions with positively charged residues (Fig. 7). Atomistic interactions of all "binding poses" for each native ligand Na+, Cl−and NaCl are presented in Table 6.
A The complex of DUF1622 and MG in presence of Na+, B The complex of DUF1622 and 22B in presence of Na+, C The complex of DUF1622 and 22B in presence of Cl−, D The complex of DUF1622 and MG in presence of Cl−, E The complex of rimO and FS5 in presence of Na+, F The complex of rimO and FS5 in presence of Cl−, G The complex of glpD and FAD in presence of Cl−, H The complex of glpD and FAD in presence of Na+
structural representation of Na+, Cl−, and NaCl molecules binding poses on the glpD, rimO and DUF1622 A Predicted Cl− binding pose in glpD, B Predicted Na+ binding pose in glpD, C Predicted NaCl binding poses in glpD, D Predicted Cl− binding pose in rimO, E Predicted Na+ binding pose in rimO, F Predicted NaCl binding poses in rimO. G Predicted Cl− binding pose in DUF1622, H Predicted Na+ binding pose in DUF1622, I Predicted NaCl binding poses in DUF1622
The binding free energies of all proteins in complex with their predicted native ligands. Energyt is the total binding energy of native ligand and the protein. Energyu Na+ is the Na+ binding energy to the binding site. Energyt Na+ is the binding energy of native ligand in presence of Na+. Energyu Cl− is the Cl− binding energy to the binding site. Energyt Cl− is the binding energy of native ligand in presence of Cl−
Growth rate, chlorophyll (a), and morphological analysis of P. tenue under salt stress
The growth rate was compared among the cells cultured under control or stress condition. Measuring the dry mass weight and chlorophyll-a content demonstrated the potential ability of this bacterium to grow under salinity conditions (Fig. 8). Studying the morphology of P. tenue gives clues about the cellular mechanism of this microorganism as they are photosynthetic microorganisms, and the cell morphology directly affects the photosynthetic rate. P. tenue bears a Gram-negative cell envelope containing an outer membrane external to the cytoplasmic membrane, which is thicker than in most other well-characterized Gram-negative bacteria. After 7 days of treatment with NaCl, no extensive variability was observed between the stress-treated cells and the control. After 7 days, cells started depigmentation under 500 mM NaCl at a low rate (Fig. 9). The defence mechanism against these effects includes biosynthesis of osmoprotectant regulators, ROS–scavenging antioxidant enzymes, as well as the resynthesize of photosynthetic pigments and photosystem proteins, which may be due to balanced energy-linked assimilation and ATP production under such conditions. The most important output of morphological analysis in our study was the low rate of cell depigmentation under NaCl stress.
Transcript analysis
One specific goal was to investigate gene expression concerning the physiological changes under salt stress, particularly the expression profiles of transcripts encoding components of salt-responsive genes, including glpD, DUF1622, and rimO. Changes in transcript abundance of each glpD, rimO, and DUF1622 under NaCl conditions were analyzed using qRT-PCR. For an accurate transcript-level analysis, 16S rRNA, a housekeeping gene, was selected as a reference gene. The specificity of PCR products was determined using melting curve analysis. There was no amplification in no-template control (NTC). A relative gene expression of glpD, DUF1622, and rimO was compared to the internal 16S rRNA gene. The up-regulation of selected genes was observed after 24 h of treatment of cells with 500 mM NaCl. The qRT-PCR results indicate the up-regulation of glpD, DUF1622, and rimO under salt stress conditions with 2.9, 2.6, and 3.2-fold change for glpD, rimO, and sll0939 (Fig. 10).

taken from three independent biological replicates. Different lower case letters above columns indicate significant differences at the (P < 0.05) and show significant differences compared with control
Relative gene expression analysis of glpD, rimO and DUF1622 genes under 500 mM NaCl for 24 h condition. Error bars represent the means ± SD
Discussion
The development of artificial biocrusts is an efficient strategy to reduce the harmful effects of abiotic stresses, such as soil salinity and plant growth/development (Cramer et al. 2011; Wu, et al. 2013; Belnap et al. 2016). P. tenue is a halophilic microorganism that can be used to produce artificial biocrusts (Antoninka et al. 2016; Bowker et al. 2018; Bu et al. 2017; Büdel et al. 2016; Chamizo et al. 2018; Sommer et al. 2020; Steele et al. 2014; Xin et al. 2015). Biological soil crusts not only directly impact the soil surface, but also alters soil characteristics. Biological soil crusts prevents water loss leads to lower water evaporation and consequently, decreased soil salinity, which could increase the soil quality and better plants growth/development (Kakeh et al. 2021). In this study, a combination of in silico approaches, gene expression, growth rate, and morphological analysis was implemented to identify the NaCl-responsive genes and their protein products in P. tenue. Transcriptome data showed an increase in the expression level of the sll0939, glpD, and rimO genes upon salt stress treatment in Synechocystis sp. PCC 6803. Up-regulation of the sll0939 gene as a defence mechanism against salt, acidic pH, and osmotic stress was reported in previous studies (Uchiyama et al. 2012, 2014). The glpD enzyme is involved in the biosynthesis of glycerol phosphate in the glycerolipid pathway (Uchiyama et al. 2012). Up-regulation of the glpD gene under hyperosmotic and salt stress is reported in previous studies (Eungrasamee et al. 2020; Kamonchanock et al. 2020; Kirsch et al. 2019). An increase in the expression level of the rimO gene also shows an increased tolerance against cold, osmotic, and salinity stresses (Kanesaki et al. 2002; Los et al. 2008). A co-expression network is one of the most valuable outputs of transcriptome data that link genes based on their similarity in function or regulation. There are different gene regulatory mechanisms in prokaryotes, such as uber-operons, super operons, persistent genes, clusters of pathway-related operons, and clusters of statistically correlated genes. A co-expression network is valuable, especially in characterizing genes with no identified function, which needs a large amount of transcriptome data under different conditions (Allocco et al. 2004; Eisen et al. 1998). In the case of organisms or microorganisms, which have not been studied for their whole transcriptome, such as P. tenue, the model organisms- or microorganisms-related data could be employed and give us clues about conserved co-expression networks and sub-networks. These conserved sub-networks reveal the importance of the biological process within the cell. The results of a study mainly focused on this concept reveal similarities in the patterns of co-expressed genes in the mentioned microorganisms despite a high evolutionary distance between E. coli and B. subtilis (Carpentier et al. 2005). In the present study, the constructed networks included genes in stress sensing and response alongside hypothetical proteins. Two main steps in structural bioinformatics include homology modelling and refinement and molecular docking of native ligands and stressors. Primary homology modelling was conducted by Phyre2 and trRosetta web servers. The predicted models were then analyzed for structural validations using Ramachandran plot, ERRAT, ModEval, trRosetta, and QMEAN. In brief, the trRosetta server outputs were more reliable for the proteins presented in our study. This server employs a direct energy minimization approach along with interresidue distance and orientation distributions, predicted by a deep residual neural network. In molecular docking, the binding poses of the native ligands and stressors with the proteins were modelled. COACH-D uses the AutoDock Vina docking algorithm for the accurate identification of ligand-binding pockets. The structural analysis of glpD demonstrated the FAD-binding domain, consistent with previous studies for similar proteins (Yeh et al. 2008; Zhao et al. 2018). While the predicted FS5 ligand (a crucial ligand for C-S bond formation) for rimO is also consistent with previous studies (Forouhar et al. 2013; Jarrett 2015), no experimental structural analysis has yet been conducted for sll0939. Increasing NaCl concentrations in the environment is strong evidence for the plausible binding of NaCl molecules to the proteins. The structure and ligand-binding sites of glpD, rimO, and sll0939 in the presence of Na+, Cl−, and NaCl have been studied. The ligand-binding mechanism was studied at two levels: (1) the prediction of ligand-binding sites and plausible native ligands for each protein and (2) the mechanism of Na+, Cl− and NaCl binding to each protein. One of the main reasons for the decline in protein normal functionality under stress is related to competition between the native ligand and stressors in binding to the protein (Arakawa and Timasheff 1991; Singh and Shrivastava 2017). Interference in the ligand-binding sites reduces the activity of the proteins. Despite the strong binding energy between Na+, Cl−, and NaCl with the proteins, none of these molecules interferes with the ligand-binding sites. On the other hand, the docking results reveal that in all NaCl-protein docking poses, the binding energy decreases except for the FAD-glpD docking that showed an increase in the binding energy when the protein is subjected to Na+. It has been demonstrated that 500 mM NaCl greatly influences the cellular metabolism of cyanobacteria, especially photosystem I and II, which dampen normal cell growth (Allakhverdiev and Murata 2008). After 7 days of salt treatment, morphological analysis reveals a low rate of cell depigmentation. Transcript analysis was carried out for the DUF1622, glpD, rimO genes under 500 mM NaCl after 24 h using qRT-PCR. Even though the transcript level is strongly affected by the batch effect and the transcriptional regulatory mechanism of the aforementioned genes, the results supported the transcriptome data.
Conclusions
In this study, the effect of high concentrations of NaCl on P. tenue was studied from transcriptomic and structural proteomics perspectives. Microarray data analysis reveals the up-regulation of the glpD, rimO, and sll0939 genes whose product proteins contain (DAO and DAO_C, DUF1672), (UPF0004, Radical_SAM and TRAM_2), and (DUF1622) domains. Protein structure modelling and molecular docking with Na+, Cl−, and NaCl demonstrated that the predicted target sites of stressors do not overlap with native ligand-binding sites. The growth rate, chlorophyll measurement, and morphological analysis also reveal that a small number of cells were depigmented under salinity stress after 7 days. The qRT-PCR results indicate the up-regulation of glpD, rimO, and DUF1622 under 500 mM NaCl. The results of this study facilitate the genetic manipulation and protein engineering of P. tenue for further biotechnological and industrial applications, such as producing artificial biocrust for preventing soil degradation.
References
Allakhverdiev SI, Murata N (2008) Salt stress inhibits photosystems II and I in cyanobacteria. Photosynth Res 98:529–539
Allakhverdiev SI, Sakamoto A, Nishiyama Y, Inaba M, Murata N (2000) Ionic and osmotic effects of NaCl-induced inactivation of photosystems I and II in Synechococcus sp. Plant Physiol 123:1047–1056
Allocco DJ, Kohane IS, Butte AJ (2004) Quantifying the relationship between co-expression, co-regulation and gene function. BMC Bioinform 5:1–10
Allu AD, Soja AM, Wu A, Szymanski J, Balazadeh S (2014) Salt stress and senescence: identification of cross-talk regulatory components. J Exp Bot 65:3993–4008
Antoninka A, Bowker MA, Reed SC, Doherty K (2016) Production of greenhouse-grown biocrust mosses and associated cyanobacteria to rehabilitate dryland soil function. Restor Ecol 24:324–335
Arakawa T, Timasheff S (1991) The interactions of proteins with salts, amino acids, and sugars at high concentration. In: Gilles R, Hoffmann EK, Bolis L (eds) Advances in Comparative and Environmental Physiology. Springer, Berlin, pp 226–245
Arora K, Kumar P, Bose D, Li X, Kulshrestha S (2021) Potential applications of algae in biochemical and bioenergy sector. 3 Biotech 11:1–24
Basak N, Krishnan V, Pandey V, Punjabi M, Hada A, Marathe A, Jolly M, Palaka BK, Ampasala DR, Sachdev A (2020) Expression profiling and in silico homology modeling of Inositol penta kis phosphate 2-kinase, a potential candidate gene for low phytate trait in soybean. 3 Biotech 10:1–21
Belnap J, Weber B, Büdel B (2016) Biological soil crusts as an organizing principle in drylands. In: Weber B, Büdel B, Belnap J (eds) Biological soil crusts: an organizing principle in drylands. Springer, Cham, pp 3–13
Bhardwaj AK, Shukla A, Mishra RK, Singh S, Mishra V, Uttam K, Singh MP, Sharma S, Gopal R (2017) Power and time dependent microwave assisted fabrication of silver nanoparticles decorated cotton (SNDC) fibers for bacterial decontamination. Front Microbiol 8:330
Bhardwaj AK, Shukla A, Maurya S, Singh SC, Uttam KN, Sundaram S, Singh MP, Gopal R (2018) Direct sunlight enabled photo-biochemical synthesis of silver nanoparticles and their Bactericidal Efficacy: photon energy as key for size and distribution control. J Photochem Photobiol B 188:42–49
Bhardwaj AK, Sundaram S, Yadav KK, Srivastav AL (2021) An overview of silver nano-particles as promising materials for water disinfection. Environ Technol Innov. https://doi.org/10.1016/j.eti.2021.101721
Borsani O, Valpuesta V, Botella MA (2001) Evidence for a role of salicylic acid in the oxidative damage generated by NaCl and osmotic stress in Arabidopsis seedlings. Plant Physiol 126:1024–1030
Bowker MA, Reed SC, Maestre FT, Eldridge DJ (2018) Biocrusts: the living skin of the earth. Springer
Bu C, Wang C, Yang Y, Zhang L, Bowker MA (2017) Physiological responses of artificial moss biocrusts to dehydration-rehydration process and heat stress on the Loess Plateau, China. J Arid Land 9:419–431
Büdel B, Dulić T, Darienko T, Rybalka N, Friedl T (2016) Cyanobacteria and algae of biological soil crusts. In: Weber B, Büdel B, Belnap J (eds) Biological soil crusts: an organizing principle in drylands. Springer, Cham, pp 55–80
Carpentier A-S, Torrésani B, Grossmann A, Hénaut A (2005) Decoding the nucleoid organisation of Bacillus subtilis and Escherichia coli through gene expression data. BMC Genomics 6:1–11
Chamizo S, Mugnai G, Rossi F, Certini G, De Philippis R (2018) Cyanobacteria inoculation improves soil stability and fertility on different textured soils: gaining insights for applicability in soil restoration. Front Environ Sci 6:49
Coleman RA, Lee DP (2004) Enzymes of triacylglycerol synthesis and their regulation. Prog Lipid Res 43:134–176
Colovos C, Yeates TO (1993) Verification of protein structures: patterns of nonbonded atomic interactions. Protein Sci 2:1511–1519
Consortium U (2019) UniProt: a worldwide hub of protein knowledge. Nucleic Acids Res 47:D506–D515
Cramer GR, Urano K, Delrot S, Pezzotti M, Shinozaki K (2011) Effects of abiotic stress on plants: a systems biology perspective. BMC Plant Biol 11:1–14
Cruz de Carvalho R, dos Santos P, Branquinho C (2018) Production of moss-dominated biocrusts to enhance the stability and function of the margins of artificial water bodies. Restor Ecol 26:419–421
de Souza Silva CMM, Fay EF (2012) Effect of salinity on soil microorganisms. Soil Health Land Use Manage 10:177–198
Dickson DJ, Luterra MD, Ely RL (2012) Transcriptomic responses of Synechocystis sp. PCC 6803 encapsulated in silica gel. Appl Microbiol Biotechnol 96:183–196
Doi Y (2019) Glycerol metabolism and its regulation in lactic acid bacteria. Appl Microbiol Biotechnol 103:5079–5093
Doran JW, Parkin TB (1994) Defining and assessing soil quality. Defin Soil Qual Sustain Environ 35:1–21
Eisen MB, Spellman PT, Brown PO, Botstein D (1998) Cluster analysis and display of genome-wide expression patterns. Proc Natl Acad Sci 95:14863–14868
Eramian D, Eswar N, Shen MY, Sali A (2008) How well can the accuracy of comparative protein structure models be predicted? Protein Sci 17:1881–1893
Eungrasamee K, Incharoensakdi A, Lindblad P, Jantaro S (2020) Synechocystis sp. PCC 6803 overexpressing genes involved in CBB cycle and free fatty acid cycling enhances the significant levels of intracellular lipids and secreted free fatty acids. Sci Rep 10:1–13
Fageria N, Baligar V (2008) Ameliorating soil acidity of tropical Oxisols by liming for sustainable crop production. Adv Agron 99:345–399
Fasani E, Manara A, Martini F, Furini A, DalCorso G (2018) The potential of genetic engineering of plants for the remediation of soils contaminated with heavy metals. Plant Cell Environ 41:1201–1232
Forouhar F, Arragain S, Atta M, Gambarelli S, Mouesca J-M, Hussain M, Xiao R, Kieffer-Jaquinod S, Seetharaman J, Acton TB (2013) Two Fe-S clusters catalyze sulfur insertion by radical-SAM methylthiotransferases. Nat Chem Biol 9:333
Georg J, Rosana ARR, Chamot D, Migur A, Hess WR, Owttrim GW (2019) Inactivation of the RNA helicase CrhR impacts a specific subset of the transcriptome in the cyanobacterium Synechocystis sp. PCC 6803. RNA Biol 16:1205–1214
Gião MS, Keevil CW (2014) Listeria monocytogenes can form biofilms in tap water and enter into the viable but non-cultivable state. Microb Ecol 67:603–611
Goyal D, Yadav A, Prasad M, Singh TB, Shrivastav P, Ali A, Dantu PK, Mishra S (2020) Effect of heavy metals on plant growth: an overview. In: Naeem M, Ansari AA, Gill SS (eds) Contaminants in Agriculture. Springer, Cham, pp 79–101
Hagemann M (2011) Molecular biology of cyanobacterial salt acclimation. FEMS Microbiol Rev 35:87–123
Hernandez-Prieto MA, Futschik ME (2012) CyanoEXpress: a web database for exploration and visualisation of the integrated transcriptome of cyanobacterium Synechocystis sp PCC6803. Bioinformation 8:634
Hindré T, Knibbe C, Beslon G, Schneider D (2012) New insights into bacterial adaptation through in vivo and in silico experimental evolution. Nat Rev Microbiol 10:352–365
Hug JJ, Krug D, Müller R (2020) Bacteria as genetically programmable producers of bioactive natural products. Nat Rev Chem 4:172–193
Humphrey W, Dalke A, Schulten K (1996) VMD: visual molecular dynamics. J Mol Graph 14:33–38
Ishida TA, Nara K, Ma S, Takano T, Liu S (2009) Ectomycorrhizal fungal community in alkaline-saline soil in northeastern China. Mycorrhiza 19:329–335
Jarrett JT (2015) The biosynthesis of thiol-and thioether-containing cofactors and secondary metabolites catalyzed by radical S-adenosylmethionine enzymes. J Biol Chem 290:3972–3979
Jiang L, Pei H, Hu W, Ji Y, Han L, Ma G (2015) The feasibility of using complex wastewater from a monosodium glutamate factory to cultivate Spirulina subsalsa and accumulate biochemical composition. Biores Technol 180:304–310
Jugder BE, Ertan H, Wong YK, Braidy N, Manefield M, Marquis CP, Lee M (2016) Genomic, transcriptomic and proteomic analyses of Dehalobacter UNSWDHB in response to chloroform. Environ Microbiol Rep 8:814–824
Kakeh J, Gorji M, Mohammadi MH, Asadi H, Khormali F, Sohrabi M, Cerdà A (2020) Biological soil crusts determine soil properties and salt dynamics under arid climatic condition in Qara Qir. Science of The Total Environment, Iran, p 139168
Kakeh J, Gorji M, Mohammadi MH, Asadi H, Khormali F, Sohrabi M (2021) Effect of biocrusts on profile distribution of soil water content and salinity at different stages of evaporation. J Arid Environ 191:104514
Kamonchanock E, Aran I, Lindblad P, Saowarath J (2020) Synechocystis sp. PCC 6803 overexpressing genes involved in CBB cycle and free fatty acid cycling enhances the significant levels of intracellular lipids and secreted free fatty acids. Sci Rep. https://doi.org/10.1038/s41598-020-61100-4
Kanesaki Y, Suzuki I, Allakhverdiev SI, Mikami K, Murata N (2002) Salt stress and hyperosmotic stress regulate the expression of different sets of genes in Synechocystis sp. PCC 6803. Biochem Biophys Res Commun 290:339–348
Khalid E, Babiker E, Tinay AE (2003) Solubility and functional properties of sesame seed proteins as influenced by pH and/or salt concentration. Food Chem 82:361–366
Kharwar S, Bhattacharjee S, Mishra AK (2021) Bioinformatics analysis of enzymes involved in cysteine biosynthesis: first evidence for the formation of cysteine synthase complex in cyanobacteria. 3 Biotech 11:1–15
Khator K, Shekhawat G (2020) Nitric oxide mitigates salt-induced oxidative stress in Brassica juncea seedlings by regulating ROS metabolism and antioxidant defense system. 3 Biotech 10:1–12
Khodadadi F, Tohidfar M, Vahdati K, Dandekar AM, Leslie CA (2020) Functional analysis of walnut polyphenol oxidase gene (JrPPO1) in transgenic tobacco plants and PPO induction in response to walnut bacterial blight. Plant Pathol 69:756–764
Kirsch F, Klähn S, Hagemann M (2019) Salt-regulated accumulation of the compatible solutes sucrose and glucosylglycerol in cyanobacteria and its biotechnological potential. Front Microbiol 10:2139
Klähn S, Hagemann M (2011) Compatible solute biosynthesis in cyanobacteria. Environ Microbiol 13:551–562
Lan C-Y, Lin K-H, Chen C-L, Huang W-D, Chen C-C (2020) Comparisons of chlorophyll fluorescence and physiological characteristics of wheat seedlings influenced by iso-osmotic stresses from polyethylene glycol and sodium chloride. Agronomy 10:325
Langridge P, Fleury D (2011) Making the most of ‘omics’ for crop breeding. Trends Biotechnol 29:33–40
Lanyi JK (1974) Salt-dependent properties of proteins from extremely halophilic bacteria. Bacteriol Rev 38:272
Laskowski RA, Watson JD, Thornton JM (2005) ProFunc: a server for predicting protein function from 3D structure. Nucleic Acids Res 33:W89–W93
Lázaro R, Cantón Y, Solé-Benet A, Bevan J, Alexander R, Sancho L, Puigdefábregas J (2008) The influence of competition between lichen colonization and erosion on the evolution of soil surfaces in the Tabernas badlands (SE Spain) and its landscape effects. Geomorphology 102:252–266
Liang Y, Li D, Chen Y, Cheng J, Zhao G, Fahima T, Yan J (2020) Selenium mitigates salt-induced oxidative stress in durum wheat (Triticum durum Desf.) seedlings by modulating chlorophyll fluorescence, osmolyte accumulation, and antioxidant system. 3 Biotech 10:1–14
Liang Z, Zhi H, Fang Z, Zhang P (2021) Genetic engineering of yeast, filamentous fungi and bacteria for terpene production and applications in food industry. Food Res Int 147:110487
Los DA, Suzuki I, Zinchenko VV, Murata N (2008) Stress responses in Synechocystis: regulated genes and regulatory systems. Caister Academic Press, Norfolk, pp 117–157
Lu Y, Cheng L (2021) Computational analysis of LexA regulons in Proteus species. 3 Biotech 11:1–15
Lu CH, Yu CS, Lin YF, Chen JY. Predicting flavin and nicotinamide adenine dinucleotide-binding sites in proteins using the fragment transformation method. Biomed Res Int. 2015; 2015:402536
Lu S, Wang J, Chitsaz F, Derbyshire MK, Geer RC, Gonzales NR, Gwadz M, Hurwitz DI, Marchler GH, Song JS (2020) CDD/SPARCLE: the conserved domain database in 2020. Nucleic Acids Res 48:D265–D268
Lynn TM, Zhran M, Wang LF, Ge T, Yu SS, Kyaw EP, Latt ZK, Htwe TM (2021) Effect of land use on soil properties, microbial abundance and diversity of four different crop lands in central Myanmar. 3 Biotech 11:1–15
Macindoe G, Mavridis L, Venkatraman V, Devignes M-D, Ritchie DW (2010) HexServer: an FFT-based protein docking server powered by graphics processors. Nucleic Acids Res 38:W445–W449
Maestre FT, Sole R, Singh BK (2017) Microbial biotechnology as a tool to restore degraded drylands. Microb Biotechnol 10:1250–1253
Mahdavi S, Razeghi J, Pazhouhandeh M, Movafeghi A, Kosari-Nasab M, Kianianmomeni A (2020) Characterization of two predicted DASH-related proteins from the green alga Volvox carteri provides new insights into their light-mediated transcript regulation and DNA repair activity. Algal Res 52:102116
Trisilowati, Mallet DG. In silico experimental modeling of cancer treatment. ISRN Oncol. 2012;2012:828701
Mathpal P, Kumar U, Kumar A, Kumar S, Malik S, Kumar N, Dhaliwal H, Kumar S (2018) Identification, expression analysis, and molecular modeling of Iron-deficiency-specific clone 3 (Ids3)-like gene in hexaploid wheat. 3 Biotech 8:1–11
Mironov KS, Sinetova MA, Shumskaya M, Los DA (2019) Universal molecular triggers of stress responses in Cyanobacterium Synechocystis. Life 9:67
Mugnai G, Rossi F, Felde VJMNL, Colesie C, Büdel B, Peth S, Kaplan A, De Philippis R (2018) Development of the polysaccharidic matrix in biocrusts induced by a cyanobacterium inoculated in sand microcosms. Biol Fertil Soils 54:27–40
Mukherjee A, Bhowmick S, Yadav S, Rashid MM, Chouhan GK, Vaishya JK, Verma JP (2021) Re-vitalizing of endophytic microbes for soil health management and plant protection. 3 Biotech 11:1–17
Murata N, Takahashi S, Nishiyama Y, Allakhverdiev SI (2007) Photoinhibition of photosystem II under environmental stress. Biochimica et Biophysica (BBA) Acta Bioenergetics 1767:414–421
Nagarajan S, Nagarajan S (2009) Abiotic tolerance and crop improvement. In: Pareek A, Sopory SK, Bohnert HJ (eds) Abiotic stress adaptation in plants. Springer, Dordrecht, pp 1–11
Namwong S, Hiraga K, Takada K, Tsunemi M, Tanasupawat S, Oda K (2006) A halophilic serine proteinase from Halobacillus sp. SR5-3 isolated from fish sauce: purification and characterization. Biosci Biotechnol Biochem 70:1395–1401
Naraian R (2019) Mycodegradation of Lignocelluloses. Springer
Nawaz K, Hussain K, Majeed A, Khan F, Afghan S, Ali K (2010) Fatality of salt stress to plants: morphological, physiological and biochemical aspects. Afr J Biotechnol 9:34
Obalum S, Chibuike G, Peth S, Ouyang Y (2017) Soil organic matter as sole indicator of soil degradation. Environ Monit Assess 189:176
Oshone R, Ngom M, Chu F, Mansour S, Sy MO, Champion A, Tisa LS (2017) Genomic, transcriptomic, and proteomic approaches towards understanding the molecular mechanisms of salt tolerance in Frankia strains isolated from Casuarina trees. BMC Genomics 18:1–21
Osman KT (2018) Saline and sodic soils. In: Osman KT (ed) Management of soil problems. Springer, Cham, pp 255–298
Ozturk S, Aslim B (2010) Modification of exopolysaccharide composition and production by three cyanobacterial isolates under salt stress. Environ Sci Pollut Res 17:595–602
Parmar N, Singh KH, Sharma D, Singh L, Kumar P, Nanjundan J, Khan YJ, Chauhan DK, Thakur AK (2017) Genetic engineering strategies for biotic and abiotic stress tolerance and quality enhancement in horticultural crops: a comprehensive review. 3 Biotech 7:1–35
Pei H, Jiang L, Hou Q, Yu Z (2017) Toward facilitating microalgae cope with effluent from anaerobic digestion of kitchen waste: the art of agricultural phytohormones. Biotechnol Biofuels 10:1–18
Phatak SS, Stephan CC, Cavasotto CN (2009) High-throughput and in silico screenings in drug discovery. Expert Opin Drug Discov 4:947–959
Punjabi M, Bharadvaja N, Sachdev A, Krishnan V (2018) Molecular characterization, modeling, and docking analysis of late phytic acid biosynthesis pathway gene, inositol polyphosphate 6-/3-/5-kinase, a potential candidate for developing low phytate crops. 3 Biotech 8:1–20
Qadir M, Ghafoor A, Murtaza G (2001) Use of saline–sodic waters through phytoremediation of calcareous saline–sodic soils. Agric Water Manag 50:197–210
Reed CJ, Lewis H, Trejo E, Winston V, Evilia C (2013) Protein adaptations in archaeal extremophiles. Archaea. https://doi.org/10.1155/2013/373275
Rezaei QBF, Solouki A, Tohidfar M, Zare Mehrjerdi M, Izadi-Darbandi A, Vahdati K (2020) Agrobacterium-mediated transformation of Persian walnut using BADH gene for salt and drought tolerance. J Horticult Sci Biotechnol. https://doi.org/10.1080/14620316.2020.1812446
Ritchie ME, Phipson B, Wu D, Hu Y, Law CW, Shi W, Smyth GK (2015) limma powers differential expression analyses for RNA-sequencing and microarray studies. Nucleic Acids Res 43:e47–e47
Rocha F, Esteban Lucas-Borja M, Pereira P, Muñoz-Rojas M (2020) Cyanobacteria as a nature-based biotechnological tool for restoring salt-affected soils. Agronomy 10:1321
Roncero-Ramos B, Román J, Gómez-Serrano C, Cantón Y, Acién F (2019) Production of a biocrust-cyanobacteria strain (Nostoc commune) for large-scale restoration of dryland soils. J Appl Phycol 31:2217–2230
Rychlik W (2007) OLIGO 7 primer analysis software. PCR Prim Des. https://doi.org/10.1007/978-1-59745-528-2_2
Sahoo S, Mahapatra SR, Das N, Parida BK, Rath S, Misra N, Suar M (2020) Functional elucidation of hypothetical proteins associated with lipid accumulation: Prioritizing genetic engineering targets for improved algal biofuel production. Algal Res 47:101887
Schrödinger L, DeLano W (2020) PyMOL. Retrieved from http://www.pymol.org/pymol
Shen MY, Sali A (2006) Statistical potential for assessment and prediction of protein structures. Protein Sci 15:2507–2524
Singh S, Shrivastava AK (2017) In silico characterization and transcriptomic analysis of nif family genes from Anabaena sp. PCC7120. Cell Biol Toxicol 33:467–482
Smith P, House JI, Bustamante M, Sobocká J, Harper R, Pan G, West PC, Clark JM, Adhya T, Rumpel C (2016) Global change pressures on soils from land use and management. Glob Change Biol 22:1008–1028
Sommer V, Karsten U, Glaser K (2020) Halophilic algal communities in biological soil crusts isolated from potash tailings pile areas. Front Ecol Evol 8:46
Steele DJ, Franklin DJ, Underwood GJ (2014) Protection of cells from salinity stress by extracellular polymeric substances in diatom biofilms. Biofouling 30:987–998
Tonk L, Bosch K, Visser PM, Huisman J (2007) Salt tolerance of the harmful cyanobacterium Microcystis aeruginosa. Aquat Microb Ecol 46:117–123
Uchiyama J, Asakura R, Kimura M, Moriyama A, Tahara H, Kobayashi Y, Kubo Y, Yoshihara T, Ohta H (2012) Slr0967 and Sll0939 induced by the SphR response regulator in Synechocystis sp PCC 6803 are essential for growth under acid stress conditions. Biochimica et Biophysica Acta (BBA) Bioenergetics 1817:1270–1276
Uchiyama J, Asakura R, Moriyama A, Kubo Y, Shibata Y, Yoshino Y, Tahara H, Matsuhashi A, Sato S, Nakamura Y (2014) Sll0939 is induced by Slr0967 in the cyanobacterium Synechocystis sp. PCC6803 and is essential for growth under various stress conditions. Plant Physiol Biochem 81:36–43
Vonshak A, Guy R, Guy M (1988) The response of the filamentous cyanobacterium Spirulina platensis to salt stress. Arch Microbiol 150:417–420
Wan X-F, VerBerkmoes NC, McCue LA, Stanek D, Connelly H, Hauser LJ, Wu L, Liu X, Yan T, Leaphart A (2004) Transcriptomic and proteomic characterization of the Fur modulon in the metal-reducing bacterium Shewanella oneidensis. J Bacteriol 186:8385–8400
West NE (1990) Structure and function of microphytic soil crusts in wildland ecosystems of arid to semi-arid regions. Advances in ecological research. Elsevier, pp 179–223
Wu Y, Rao B, Wu P, Liu Y, Li G, Li D (2013) Development of artificially induced biological soil crusts in fields and their effects on top soil. Plant Soil 370 (1–2):115–124
Xin Z, Yong S, Yang L, Rong-Liang J, Xin-Rong L (2015) Osmotic adjustment of soil biocrust mosses in response to desiccation stress. Pedosphere 25:459–467
Yadav S, Modi P, Dave A, Vijapura A, Patel D, Patel M (2020) Effect of Abiotic Stress on Crops, Sustainable Crop Production. IntechOpen
Yamazaki K, Ishimori M, Kajiya-Kanegae H, Takanashi H, Fujimoto M, Yoneda J-I, Yano K, Koshiba T, Tanaka R, Iwata H (2020) Effect of salt tolerance on biomass production in a large population of sorghum accessions. Breed Sci 70:167–175
Yang Y, Guo Y (2018) Unraveling salt stress signaling in plants. J Integr Plant Biol 60:796–804
Yang J, Roy A, Zhang Y (2012) BioLiP: a semi-manually curated database for biologically relevant ligand–protein interactions. Nucleic Acids Res 41:D1096–D1103
Yang J, Roy A, Zhang Y (2013) Protein–ligand binding site recognition using complementary binding-specific substructure comparison and sequence profile alignment. Bioinformatics 29:2588–2595
Yang J, Anishchenko I, Park H, Peng Z, Ovchinnikov S, Baker D (2020) Improved protein structure prediction using predicted interresidue orientations. Proc Natl Acad Sci 117:1496–1503
Yeh JI, Chinte U, Du S (2008) Structure of glycerol-3-phosphate dehydrogenase, an essential monotopic membrane enzyme involved in respiration and metabolism. Proc Natl Acad Sci 105:3280–3285
Zhao Y, Li X, Wang F, Zhao X, Gao Y, Zhao C, He L, Li Z, Xu J (2018) Glycerol-3-phosphate dehydrogenase (GPDH) gene family in Zea mays L: Identification, subcellular localization, and transcriptional responses to abiotic stresses. PloS one 13:e0200357
Funding
No funding to declare.
Author information
Authors and Affiliations
Corresponding author
Supplementary Information
Below is the link to the electronic supplementary material.
Rights and permissions
About this article
Cite this article
Shahbazi, M., Tohidfar, M. & Azimzadeh Irani, M. Identification of the key functional genes in salt-stress tolerance of Cyanobacterium Phormidium tenue using in silico analysis. 3 Biotech 11, 503 (2021). https://doi.org/10.1007/s13205-021-03050-w
Received:
Accepted:
Published:
DOI: https://doi.org/10.1007/s13205-021-03050-w