Abstract
This paper evaluated the precision and accuracy of two gridded monthly precipitation products (P-GPCC/V6 from the Global Precipitation Climatology Centre and P-UDEL/V3.01 from the University of Delaware—UDEL) and two monthly air temperature products (T-GHCN/V2 from the Global Historical Climatology Network and T-UDEL/V3.01 from UDEL) for the State of Acre, in southwestern Amazonia, northern Brazil. As reference, we used monthly climate series of these elements from January 1971 to December 2000, recorded at five conventional weather stations (CWS) of the Instituto Nacional de Meteorologia (INMET). Data were compared by linear regression; the coefficient of determination (r 2); Willmott’s index of agreement (d); Camargo’s performance index (c); the root-mean-square error (RMSE); mean systematic error (MSE); and unsystematic error (MSEu). Moreover, a trend analysis of the products at a seasonal and annual scale was performed. Regarding rainfall, it was found that GPCC as well as UDEL represents the mean and variability of rainfall throughout the series, but the performance of the product of GPCC was more homogenous and generally applicable for all stations. In relation to the air temperature standards, the precision of GHCN and UDEL was low, but the accuracy was moderate, according to statistical methods.
Similar content being viewed by others
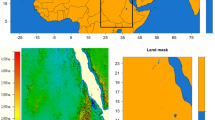
Avoid common mistakes on your manuscript.
Introduction
The Amazon is a key region for climate control on a local, regional and global scale. This is related to the high amount of solar energy in the region and water availability, resulting from convective processes and evapotranspiration (ET). Nevertheless, the rainfall regimes in the region are not homogeneous, but highly variable in spatial (regional and large scale) and temporal (intra-annual and interannual) terms, due to the influence of different meteorological systems at the local, meso- and large scale and climate variability modes (Rocha 2001; Duarte 2006; Reboita et al. 2010). Among the climate variability modes conditioning rainfall and air temperature patterns of the region of this study are the El Niño-Southern Oscillation (ENSO), the Atlantic dipole and the Pacific Decadal Oscillation (PDO) (Marengo 2005).
In Acre, a state in the southwest of the Brazilian Amazon, the performance of the meteorological systems, climate variability and associated mechanisms is not comprehensively understood, partly because scientific studies for this region focus only on static climate descriptions based on rainfall and air temperature (Duarte 2006). Due to the importance of this region in the regional and global climate, studies are needed to understand the intra-annual (monthly and seasonal) variation of rainfall and air temperature in the region, and how the interactions between meteorological systems and physiographic factors condition this variation (Almeida et al. 2015). In addition, it is important to understand how the climate variability modes (ENSO, dipole and PDO) influence the regional meteorological systems and consequently, the interannual, decadal and multidecadal rainfall and air temperature patterns (Almeida et al. 2016).
Studies addressing the high-frequency (monthly and seasonal) and low-frequency (interannual, decadal and multidecadal) climatic variability and on a regional scale require long (>30 years) continuous, high-quality climate series, regularly distributed in space and with high resolution (<0.5°). Anyway, due to the geographical and economic limitations in the region, spatially regularly distributed time series covering 30 years or more are scarce, hampering researches in different areas of the region. This is a recurring problem in many regions of the planet, mainly in most of South America, Africa and part of Asia (GPCC 2014; Prakash et al. 2015).
Although reanalysis data comprise long-term series (Reanalysis 1, from 1948 to the present) in grids, which consider the whole planet, the low spatial resolution of the grid (2.5° × 2.5°) restricts regional studies (Kalnay et al. 1996; Kanamitsu et al. 2002). An exception is the regional reanalysis for North America (NA), available with a spatial resolution of 0.3° × 0.3° (Mesinger et al. 2006). The numerical climate models are usually Atmospheric General Circulation Models (MCGA), which also hamper regional climate studies due to the low resolution of the simulations and the need for a robust computer structure for long-term simulations. In both cases, for regional climate studies downscaling techniques should be applied to obtain products with a higher resolution, which is time-consuming and requires computational power, mainly for dynamic downscaling. The rainfall products obtained by remote orbital sensors such as CMORPH, TRMM-3B42 and TRMM-3B43 have a high resolution (0.25° × 0.25° or 0.5° × 0.5°), provided by multiple estimators underlying the elaboration of the products of the Tropical Rainfall Measuring Mission (TRMM) satellite, and good accuracy and precision of these products for several relief conditions (Feidas 2010; Dinku et al. 2008). Nevertheless, they have no long-term data series (>30 years), which restricts studies on climatic variability and extreme events, e.g., on droughts, floods, desertification, and on the forest fires/arson common in the Amazon region (Almeida et al. 2016).
The grid data, obtained by techniques of spatial interpolation of rain and air temperature data, measured in networks of surface meteorological stations, include long-term data series. Some of these cover over 100 years, as in the case of grid data elaborated at the University of Delaware (Willmott and Matsuura 2001) and in various spatial resolutions, from 2.5° × 2.5° to high (0.5°) resolution (GPCC 2014; Willmott and Matsuura 2001). The temporal characteristics and high spatial resolution (0.5°) of these grid data indicate them for applications in regional climate studies, for example addressing dry-season climatic variability (GPCC 2014).
However, historical gridded data obtained with different products may contain several errors, and a comparison with data from in situ observations can reveal several errors, especially for remote areas, where the reliability of the observed data is particularly questionable. Therefore, the different methodologies applied in the spatial interpolation processes of gridded data, as well as terrain characteristics and the number of stations used to generate the grids, can generate interpolated products with disparate data, affecting the reliability of estimates (Sun et al. 2014).
Thus, given the need to obtain reliable climate data with spatial regularity, high resolution and long-term data series for regional climate studies (e.g., on drought, floods and desertification), the data precision and accuracy were evaluated of the two gridded monthly precipitation products (P-GPCC/V6 from the Global Precipitation Climatology Centre and P-UDEL/V3.01 from the University of Delaware {UDEL}) and the two monthly air temperature products (T-GHCN/V2 from the Global Historical Climatology Network and T-UDEL/V3.01 from UDEL).
Materials and methods
Description of gridded products
The GPCC product (Total Full V6) used in this study provides monthly precipitation data at the global level, in a grid with a spatial resolution of 0.5° × 0.5°, from 1948 to 2010. To generate this product, synoptic meteorological data were used, accessible by the Global Telecommunications System (GTS); monthly weather bulletins; weather station data from different countries; data collections of some international regional projects; as well as from the collection of global data of the Climatic Research Unit (UK) and the United Nations Food and Agriculture Organization (FAO) (Rudolf and Schneider 2005).
The quality of weather station data is rigorously controlled by preprocessing of the data source; time-series analyses that identify spurious changes in mean and variance; and spatial comparisons to check the accuracy of the climatological mean and the seasonal cycle in relation to neighboring points, to identify outliers. After these procedures, potential errors are corrected, if possible; otherwise, these records are discarded and included in the final composition (Schneider et al. 2014).
Thereafter, the rainfall data are interpolated by the method SphereMap (Willmot et al. 1985), and the errors associated with the interpolation process are estimated. The method SphereMap is a spherical adaptation of the Shepard weighting system (Shepard 1968), which considers: (a) the distances of the stations to the grid point (for a limited number of nearest stations), (b) the directional distribution of stations in relation to the grid point (to avoid excess weight of stations in clusters) and (c) the gradients of the data field at the grid point.
To generate the gridded product, the rainfall anomalies of the stations are interpolated and then superimposed on the total absolute precipitation (Beck et al. 2005). A detailed description of the methodology used to generate the GPCC precipitation product can be found in Rudolf and Schneider (2005), Becker et al. (2013) and Schneider et al. (2014).
The GHCN product (V2) used in this study provides monthly air temperature data at the global level, at a grid resolution of 0.5 × 0.5°, from 1948 to 2010. Data from 31 sources from across the world are compiled, and the quality is rigorously controlled, consisting of preprocessing and control of the source data. Homogeneity tests are also performed, aimed at minimizing the effect of non-climatic factors on the time series, such as changes of land use and soil cover (Lawrimore et al. 2011).
In this product, air temperature anomalies are used to normalize the data so that they can be compared and combined to represent the temperature patterns more accurately in relation to what is normal for different locations within a region, particularly in areas with varying altitude and/or sparse data (Fan and van Den Dool 2008).
For this calculation, the Climate Anomaly Method (CAM) (Ropelewski et al. 1984) or the First Difference Method (FDM) is used (Peterson et al. 1998). The CAM involves the calculation of temperature anomalies (from the 30-year climatological mean of 1961–1990 or 1971–2000) for each month and year of the registration period of a weather station. The FDM, on the other hand, is used to calculate anomalies of stations with a smaller data set, with at least two years of consecutive data. This method is based exclusively on the calculation of the air temperature difference between one year and the next.
After these calculations, the anomaly fields are combined with those of air temperature to provide final grid values by the method of Cressman (1958) for interpolation. A detailed description of the methodology used in the gridded air temperature product of GHCN was presented by Fan and Van den Dool (2008) and Lawrimore et al. (2011).
Apart from the products described above, two other databases generated by the University of Delaware were used for comparison, one of which is a product of precipitation (V3.01) and the other of air temperature (V3.01).
The precipitation grid of UDEL compiles data from various sources: the Global Historical Climatology Network (GHCN2), Canada’s Atmospheric and Environmental Service; the hydrometeorological Institute of Saint Petersburg—Russia; Greenland Climate Network; records from automatic weather stations of the Greenland Project, the National Center for Atmospheric Research (NCAR), daily data from India; rainfall data files of the African continent; monthly rainfall data of the South American continent; Global Surface Summary of the Day (GSOD) and of Legates and Willmott (1990). After a quality control and data blending, the absolute rainfall values were interpolated by the spherical method of Shepard (Shepard 1968) in a 0.5° x 0.5° grid (Matsuura and Willmott 2009).
The UDEL air temperature product is based on GHCN version 2 records of Legates and Willmott (Legates and Willmott 1990a, b). The gridded product is also available at a resolution of 0.5° × 0.5°, but this is generated by the method climatologically aided interpolation (CAI) (Willmott and Robeson 1995), adjusted by the digital elevation model (DEM).
The CAI uses climatological data of high spatial resolution to obtain a monthly air temperature difference at each station. These differences between stations are interpolated by the Shepard algorithm. Finally, each gridded monthly difference is added back to the corresponding field of monthly climate to obtain the final temperature value (Willmott and Robeson 1995).
It is noteworthy that the interpolation process generates a product based on absolute air temperature data instead of anomaly fields. After interpolating both products, errors are estimated by cross-validation.
Detailed descriptions of precipitation and air temperature products of UDEL were provided by Legates and Willmott (1990a, b), Willmott and Matsuura (1995), and Willmott and Robeson (1995).
Data validation
The precipitation and air temperature data of the products were validated for the period from January 1971 to December 2000, using climate series of five conventional weather stations (CWS) of INMET. Since there are only three stations in the State of Acre, data from two stations in the State of Amazonas were included.
A point grid with the GPCC data of precipitation, GHCN air temperature and UDEL precipitation and air temperature data was superimposed on the location of the stations (Fig. 1). For validation, data from the grid points closest to the stations were used (see Table 1).
The data were validated by comparing the monthly precipitation and air temperature values estimated by the gridded products with the observed CWS data of INMET. The trend of rainfall at a seasonal and interannual scale was also evaluated. Regarding air temperature, the trend of this variable was only analyzed on an annual scale, since the amplitude of mean temperature changes over the months is low in this study area near the Equator.
Aside from the analysis of seasonal and interannual trends, the data were also compared by the following methods or statistical indices: simple linear regression between the estimated data (Y) by the gridded data (GPCC, GHCN or UDEL) and observed data (X) at the weather stations; the coefficient of determination (r 2); the Willmott index of agreement (d); the Camargo performance index (c); root-mean-square error (RMSE); mean systematic error (MSEs); and the non-systematic error (MSEu). The r 2 quantifies the precision of the method, that is, the degree of dispersion of the points from the mean indicating the random error.
The Willmott index of agreement (d) determines the accuracy of the method, that is, it indicates the degree of deviation from the estimated in relation to the observed values (Willmott 1981). This index ranges from 0 for no agreement to 1 for perfect agreement. The agreement index is calculated by the following equation:
where N = number of observations; P i = values estimated by GPCC, GHCN or UDEL; O i = values observed at INMET stations; O = mean of observed values.
The index “c” developed by Camargo and Sentelhas (1997) evaluates the overall performance of the method, summarizing the results of the indices “d” and “r,” according to the equation:
where c = Camargo index; r = correlation coefficient; d = Willmott index.
The criterion by which the performance of estimated data according to the “c” index was interpreted is listed in Table 2.
The root-mean-square error (RMSE) provides information on data dispersion, i.e., the scattering degree obtained in comparison with the estimated values, where ideally, the RMSE would be equal to 0. It is estimated by the following equation:
Nevertheless, the RMSE does not indicate whether errors are systematic or non-systematic (random). According to Willmott (1981), it is therefore important to define each error type separately:
- (a)
systematic error (MSEs)—the pattern of an instrument or model to over- or underestimate data systematically, affecting the accuracy of measurement. This error represents the sum of sources of systematic errors from precipitation and air temperature estimates based on the regression equations (Y = a + b X):
$${\text{MSE}}_{\text{s}} = {\text{MSE}}_{\text{a}} + {\text{MSE}}_{\text{p}} + {\text{MSE}}_{\text{i}}$$(4)where
(MSEa)—additive systematic error—results from under- or overestimation compared to the values observed at stations in relation to the constant term (a) of estimates of GPCC, GHCN or UDEL, defined as:
$${\text{MSE}}_{\text{a}} = {\text{a}}^{ 2}$$(5)(MSEp) proportional systematic error—results from under- or overestimation compared to the observed values at weather stations in relation to the proportional term (b) of estimates of GPCC, GHCN or UDEL, defined as:
$${\text{MSE}}_{\text{p}} = \left( {b - 1} \right)^{2} \, \left[ {\frac{{\sum\nolimits_{i = 1}^{N} {O_{i}^{2} } }}{N}} \right]$$(6)where N = number of observations; O i = values observed at INMET stations.
(MSEi) interdependent systematic error—the degree of interdependence between the additive (a) and proportional error is given in terms of the covariance between the error due to the additive term (a) and the proportional error (b-1):
$${\text{MSE}}_{\text{i}} = 2a \, \left( {b - 1} \right)\bar{O}$$(7)where \(\bar{O}\) is the mean of the observed values.
- (b)
non-systematic error (MSEu)—the random error results from variations in measurements that do not follow a fixed trend, but that can be analyzed by their dispersion around the mean, which affects the measurement precision. The non-systematic (random) error is calculated by:
$${\text{MSE}}_{\text{u}} = {\text{MSE}} - {\text{MSE}}_{\text{s}}$$(8)where MSEs = systematic error; MSE = total mean error;
The MSE is given by:
where N = number of observations; P i = values estimated by GPCC, GHCN or UDEL; O i = values recorded at INMET stations.
According to Andrade-Júnior et al. (2003), MSE, MSEe and MSEu should be interpreted based on their square roots (RMSE, RMSEe and RMSEu), since these are defined in the same measurement units of O and P (mm or °C). Therefore, after calculating the error types, their roots were extracted, and only the former were used to interpret the results.
Results and discussion
Comparison of precipitation products
The interannual analysis of the climate data series (Fig. 2) indicated that the GPCC and UDEL products satisfactorily represented the rainfall pattern observed at the INMET stations in most years, since the Pearson’s correlation coefficient exceeded 0.72 for both products (Table 3).
Nevertheless, there was a clear discrepancy in relation to the in situ data, measured between 1979 and 1982 at all stations, with a relative error of overestimation between 15.96 and 435 mm (Table 4). Another data discrepancy, though with lower relative error, occurred between 1995 and 1997, reaching 46.94 mm of underestimation.
Silva et al. (2012) studied monthly precipitation estimates derived from the gridded product of UDEL and the algorithm 3B43_V6, for Rio Grande do Norte, northeastern Brazil, and observed that the interannual variation in data behavior coincided with El Niño periods, when the rainfall pattern in the state is altered.
Several studies described the influence of climate variability by ENSO on changes in the precipitation regime in the Amazon region between 1979 and 1982 (Souza et al. 2000; Liebmann and Marengo 2001; Marengo 2006; Andreoli et al. 2012; Almeida et al. 2016). However, the Multivariate ENSO-MEI index (2014) indicated a long period of neutrality, with the occurrence of positive anomalies in the sea surface temperature (SST) only in late 1982, when the monthly precipitation products of GPCC and UDEL converged with the INMET data again.
In 1995 and 1996, the phenomenon of ENSO was also considered neutral, but in 1997 anomalies exceeded 2 °C, classifying this El Niño as strong. The same anomaly continued in 1998, although in this year the data of the precipitation product coincided with the INMET data again (Fig. 2). In other words, the cause of the discrepancy between the data of the GPCC and UDEL products in the period analyzed was not related to the El Niño events.
In 1979, 1980, 1981 and 1997, when the dissimilarity between the observed and estimated data was greatest, an interhemispheric gradient between the northern and southern tropical Atlantic basins was observed, with positive anomalies in the northern basin (FUNCEME 2015). Several studies (Marengo et al. 2008, 2012 Zeng et al. 2008; Yoon and Zeng 2010) pointed out that this pattern was associated with negative rainfall anomalies in the Amazon, especially in the southern part.
This positive gradient was responsible for the low precipitation recorded at INMET stations. However, the data estimated by the gridded products did not follow this pattern, especially at the stations Eirunepé and Lábrea, where the relative errors were highest (Table 4) both for GPCC (177 mm for Eirunepé and 416 mm for Lábrea in 1980) and for UDEL (179 mm for Eirunepé and 436 mm for Lábrea in 1980).
Of the analyzed stations, Eirunepé is located farthest north and Lábrea farthest east (Fig. 1), which may explain the high relative error between the observed and estimated data for the products. This occurs because, due to the scarcity of data for the Amazon region, other stations located farther north and east of the region are considered in the composition of the data grid, which affects the result, since in these other areas the influence of positive anomalies of the North Atlantic SST pattern on the rainfall regime may be weaker than that of other phenomena.
Thus, the performance of other meteorological systems can help explain the discrepancy between the data for these specific periods. To this end, a more detailed study on the influence of mesoscale systems that can alter regional rain patterns is necessary. The reason is that, given the spatial resolution of the products reviewed here, they are unable to capture possible changes in regional rainfall patterns because the data underlying the grids are from a few scattered stations.
When observing the performance of products from a seasonal point of view (Fig. 3), observed and gridded precipitation had the same pattern, which again indicates the data agreement in the rainfall regime throughout the year. However, it was found that both products estimated rainfall rates best in the driest months, while in the rainiest months the discrepancy between the observed data by INMET and those estimated by grid products was greater. The exceptions were Rio Branco and Lábrea, where the performance of the UDEL product was unsatisfactory for both seasons.
Results similar to those of most stations were found by Sun et al. (2014) in a comparison with various gridded precipitation products for China (including those of GPCC and UDEL), who observed that these estimated rainfall better in the dry season.
The worse performance of estimates in the rainiest months can be explained by the higher occurrence of systematic measurement errors during rainfalls. The weather conditions (e.g., wind, air temperature and radiation) during a rainfall, as well as their intensity, affect the measurement quality due to evaporation (Schneider et al. 2014). In months with a lower number of rainfalls, errors of this nature are also minimized.
As the gridded products use data from several stations, which have systematic errors with different magnitudes, the dissimilarity of the estimates generated by these products tends to increase during the rainy season.
The agreement of GPCC (Fig. 4) and UDEL (Fig. 5) products for the data estimated throughout the climatological series was analyzed by regression analysis. This resulted in a model of the relationship between the observed rainfall values and those estimated by grid products.
Based on the regression coefficient r 2, it was found that the precipitation data of the station Cruzeiro do Sul were highly precise for the products of GPCC (0.82) and UDEL (0.89), whereas the precision of station Rio Branco was only high for the GPCC product (0.91). The precision at the other stations was satisfactory for both products.
According to Andrade-Júnior et al. (2003), the consideration of r2 as the only definition criterion of quality of a product is not appropriate, because this coefficient cannot establish the type and magnitude of the differences. Therefore, the types of errors associated with the products must be taken into consideration.
Table 5 shows the RMSE, as well as its ratio in relation to the mean of the observed data. The lowest RMSE values for GPCC were observed at the stations Cruzeiro do Sul (44.9 mm) and Rio Branco (35.6 mm), and the highest precision for the same stations. For UDEL, the RMSE of station Cruzeiro do Sul was the best (35.4 mm).
It is noteworthy that the error percentage of GPCC in relation to the precipitation mean over the time series was consistent with the ratio estimated by Rudolf et al. (1994) for four of the five stations. These authors analyzed data at a global level and estimated an error ratio between 7 and 40% in relation to the mean for regions with a low density of stations.
In general, it is understood that the error of good estimation methods is close to zero; however, the proportion of the error type is essential, i.e., whether it is random or systematic. For the precipitation products of GPCC and UDEL, more than 94% error (Table 6) with a non-systematic nature (MSEu) was observed at all stations, which affects the precision of the estimates, but not necessarily their accuracy. The latter is mainly associated with the systematic errors related to estimation errors inherent in the GPCC and UDEL interpolation method itself, which were low for both products.
With respect to the accuracy of the Willmott coefficient (Table 7), the coefficient d of all stations was found to be higher than 0.82, mainly for Cruzeiro do Sul (0.95 in GPCC and 0.97 in UDEL) and Tarauacá (0.92 in GPCC and 0.91 in UDEL).
These results indicate a high accuracy level for the GPCC and UDEL products, i.e., a high degree of similarity between the estimated precipitation values and those observed in situ. However, the analysis of the results of the products showed that while the accuracy of four of the five stations analyzed was relatively similar, the station Rio Branco had a distinct pattern with regard to the products of GPCC and UDEL (coefficient d of 0.97 and 0.82, respectively). In the latter case, this value was the lowest of the five studied stations.
Finally, in terms of both accuracy and precision, the overall performance of the results was expressed by coefficient c (Table 7). The performances of the products of GPCC and UDEL were found to be different for two stations. The performance of the station Rio Branco was classified as high for GPCC and poor for UDEL, while Eirunepé was rated good for GPCC and mean for UDEL.
Although both products proved accurate and moderately coincident with each other, the precipitation product of GPCC had a more homogenous overall performance for all stations.
To date, no studies comparing these products with each other were carried out for Amazonia. However, similar results were found by Sun et al. (2014) in a comparison of eight gridded products of precipitation and air temperature data from China, using weather station data as reference. The authors concluded that, although the products had a similar behavior as of 1950, the results of GPCC were better than those of UDEL for regions in China with low altitudinal gradient.
While UDEL interpolates the absolute precipitation data, the GPCC uses anomaly values to correct the sampling errors. According to Becker et al. (2013), this methodological difference may contribute to more accurate results in regions with low station density.
This result is consistent with findings of Chen et al. (2002), who compared four rainfall interpolation methods (Cressman, Barnes, Shepard, and the Optimal Interpolation—OI, of Gandin). It was found that estimates were more precise when the algorithms used anormalities instead of total rainfall values.
Comparison of products of monthly air temperature
An analysis of the interannual (Fig. 6) and monthly distribution of average temperature (°C) (Fig. 7) of the climatological series indicated that the products of GHCN and UDEL followed the tendency of air temperature observed at the INMET stations for most years, despite the discrepant absolute values, with median correlation coefficients (Table 8).
On the other hand, for the long-term variation of air temperature, the errors were reduced. Table 9 shows the RMSE as well as its ratio in relation to the mean of the observed data. A low RMSE value (≤1.6 °C) was observed for all stations and both products.
These values, although low, exceeded those of Willmott and Matsuura (1995), who obtained an error of only 0.38 °C when using the interpolator CAI with the DEM for air temperature data.
The difference in the uncertainty of estimates obtained by these authors and analyzed in our study can be explained, among other factors, by the fact that the number of weather stations in southwestern Amazonia is low.
The analysis of the error type (Table 10) highlighted that, in the GHCN product, the errors of data from all stations were mostly random (>98%), which affects the precision but not the accuracy of the estimates, as mentioned above. The random errors of data of UDEL were >82% for Cruzeiro do Sul, Eirunepé and Lábrea, while Rio Branco and Tarauacá had higher percentages of systematic errors (77 and 80%, respectively).
In the regression analysis between the observed values of mean air temperature and those estimated by the gridded products, it was found that the air temperature values for GHCN were moderately precise for Rio Branco (r 2 = 0.61) and little precise (r 2 < 0.35) for the other stations (Fig. 8).
The air temperature product of UDEL was more precise for all stations with low and/or medium precision, except for Rio Branco with r 2 = 0.78, indicating high precision for this product at this location (Fig. 9).
When considering the coefficient of Willmott (Table 11), similar accuracy values were found for the stations Eirunepé and Tarauacá. The other stations, however, had values that differed between GHCN and UDEL. These variations were mainly associated with the methodological differences used to generate these products.
The best agreement between UDEL (0.79) and GHCN (0.70) was obtained for the station Cruzeiro do Sul. This station is located at an altitude of 170 m asl and closest to the Andes of all analyzed stations. In an area with low density of meteorological observations, stations at higher altitudes can be used to interpolate air temperature values for the region of Cruzeiro do Sul. Since the interpolation method used by UDEL is the CAI adjusted by DEM, this product tends to generate more accurate results than GHCN for locations closer to high altitude areas.
The accuracy for the stations Lábrea and Rio Branco was higher for GHCN, with d values of 0.59 and 0.88, respectively, while the Willmott coefficient was 0.25 and 0.75, respectively, for UDEL for these stations. Located in the east of the study area (Fig. 1), these stations lie in the so-called arc of deforestation of the Brazilian Amazon.
Several studies (Foley et al. 2003; Rocha 2001; Saad et al. 2010) indicate that the increase in deforestation provokes air temperature increases, due, among other factors, to the higher solar incidence, wind penetration and reduced soil moisture, leading to reduced ET. Thus, when analyzing the trend in air temperature over the time series, it is worth remembering that the land-use changes in the surroundings of stations hamper interannual comparisons (Ducre-Robitaille et al. 2003).
In this respect, the GHCN product of air temperature obtained more accurate results, possibly because the quality control of this product uses homogenization techniques of input data to remove the influence of land-use change on data from stations that are used for interpolation.
When considering accuracy as well as precision (Table 11), the air temperature product had similar performance for almost all stations. An exception was the station Cruzeiro do Sul [with c index 0.39 (GHCN) and 0.57 (UDEL)], with very poor and poor performance, respectively. Only the performance for Rio Branco was classified as good for both products. The performance of the other stations was classified as poor and/or very poor for GHCN as well as UDEL.
A comparison between the statistical indices of the estimated values of precipitation and air temperature showed that the performance of the latter was lower than of the former, especially when considering the overall performance by the c index and its classification, as proposed by Camargo and Sentelhas (1997).
However, this performance should be carefully analyzed, firstly because the limits of this classification are arbitrary (which is inherent to any classification proposal), and secondly because this index provides the same weight for precision and accuracy values. As these (rainfall and air temperature) are meteorological variables, the variation around the mean is naturally high, due to the occurrence of anomalous years, interfering with the precision of the results, but not necessarily with their accuracy.
Moreover, the proper systematic errors of the measurements of observations of CWS located in remote areas cannot be ignored. As the observed data are used as standard parameters for the analyses, possible associated errors compromise the quality of the comparisons (Schneider et al. 2014). Thus, both issues can generate outliers, which influence the regression analysis and results of statistical indices and consequently the precision and accuracy of the estimates.
Therefore, in regions where no long-term weather data series with adequate spatial distribution are available, the existence of estimated air temperature values reasonably close to the observed can be considered satisfactory.
Conclusions
The different techniques of data quality control and interpolation methods indicated minor differences between precision and accuracy of the gridded products studied. With regard to precipitation, the results suggest that the products of GPCC as well as of UDEL adequately represent the precipitation variability over years, although for GPCC, the differences between the stations were smaller.
With respect to the air temperature behavior, the precision of GHCN and UDEL was low, while the accuracy was moderate, although the differences between products were significant for some stations.
In view of the lack of long-term data for the southwestern Amazon, depending on the study target, the gridded temperature products discussed here can be used in climate research, provided the uncertainty degree is taken into account in the analysis of results.
References
Almeida CT, Delgado RC, Oliveira Júnior JF, Gois G, Cavalcanti AS (2015) Avaliação das Estimativas de Precipitação do Produto 3B43-TRMM do Estado do Amazonas. Revista Floresta e Ambiente 22:279–286
Almeida CT, Oliveira-Júnior JF, Delgado RC, Cubo P, Ramos MC (2016) Spatiotemporal rainfall and temperature trends throughout the Brazilian Legal Amazon, 1973–2013. Int J Climatol. doi:10.1002/joc.4831
Andrade-Júnior AS, Bastos EA, Sentelhas PC, Silva AAG (2003) Métodos de estimativa da evapotranspiração de referência diária para Parnaíba e Teresina, Piauí. RBAgro 11:63–68
Andreoli RV, Souza RAF, Kayano MT, Candido LA (2012) Seasonal anomalous rainfall in the central and eastern Amazon and associated anomalous oceanic and atmospheric patterns. Int J Climatol 32:1193–1205
Beck C, Grieser J, Rudolf B (2005) A new monthly precipitation climatology for the global land areas for the period 1951 to 2000. Climate Status Report 2004, German Weather Service, Offenbach, Germany, pp 181–190
Becker A, Finger P, Meyer-Christoffer A, Rudolf B, Schamm K, Schneider U, Ziese M (2013) A description of the global landsurface precipitation data products of the Global Precipitation Climatology Centre with sample applications including centennial (trend) analysis from 1901–present. Earth Syst Sci Data Discuss 5:921–998
Camargo AP, Sentelhas PC (1997) Avaliação do desempenho de diferentes métodos de estimativa da evapotranspiração potencial no Estado de São Paulo. RBAgro 5:89–97
Chen M, Xie P, Janowiak JE, Arkin PA (2002) Global land precipitation: a 50-yr monthly analysis based on gauge observations. J Hydrometeorol 3(3):249–266
Cressman GF (1958) An operational objective analysis system. Mon Weather Rev 87:367–374
Dinku T, Chidzambwa S, Ceccato P, Connor SJ, Ropelewski CF (2008) Validation of high resolution satellite rainfall products over complex terrain. Int J Remote Sens 29(14):4097–4110
Duarte AF (2006) Aspectos da climatologia do Acre, Brasil, com base no intervalo 1971–2000. Rev Bras Meteorol 21:308–317
Ducre-Robitaille JF, Vincent LA, Boulet G (2003) Comparison of techniques for detection of discontinuities in temperature series. Int J Climatol 23:1087–1101
Fan Y, Van Den Dool H (2008) A global monthly land surface air temperature analysis for 1948–present. J Geophys Res 113:D01103
Feidas H (2010) Validation of satellite rainfall products over Greece. Theor Appl Climatol 99(1–2):193–216
Foley J, Costa JMH, Delire C, Ramankutty N (2003) Green surprise? How terrestrial ecosystems could affect earth’s climate. Front Ecol Environ 1:38–44
FUNCEME-Fundação Cearense de Meteorologia. http://www.funceme.br/produtos/manual/oceanografia/Campos_TSM/Dados/Dipolo/dipole_servain. Accessed 10 Jan 2015
GPCC 1.0 × 1.0 Monthly Precipitation Totals. http://www.esrl.noaa.gov/psd/data/gridded/data.gpcc.html. Accessed 25 Apr 2014
Kalnay et al (1996) The NCEP/NCAR 40-year reanalysis project. Bull Am Meteor Soc 77:437–470
Kanamitsu M, Ebisuzaki W, Woollen J, Yang SK, Hnilo JJ, Fiorino M, Potter GL (2002) NCEP-DOE AMIP-II reanalysis (R-2). Bull Am Meteorol Soc 83:1631–1643
Lawrimore JH, Menne MJ, Gleason BE, Williams CN, Wuertz DB, Vose RS, Rennie J (2011) An overview of the Global Historical Climatology Network monthly mean temperature data set, version 3. J Geophys Res 116:D19121
Legates DR, Willmott CJ (1990a) Mean seasonal and spatial variability in global surface air temperature. Theor Appl Climatol 41:11–21
Legates DR, Willmott CJ (1990b) Mean seasonal and spatial variability in gauge corrected, global precipitation. Int J Climatol 10:111–127
Liebmann B, Marengo J (2001) Interannual variability of the rainy season and rainfall in the Brazilian Amazon Basin. J Clim 14:4308–4318
Marengo JA (2005) The characteristics and variability of the atmospheric water balance in the Amazon basin: spatial and temporal variability. Clim Dyn 24:11–22
Marengo JA (2006) On the hydrological cycle of the Amazon Basin: a historical review and current state-of-the-art. Rev Bras Meteorol 21:119
Marengo JA, Nobre CA, Tomasella J, Cardoso MF, Oyama MD (2008) Hydro-climatic and ecological behaviour of the drought of Amazonia in 2005. Phil Trans R Soc B 3(63):1773–1778
Marengo JA, Chou SC, Kay G, Alves LM, Pesquero JF, Soares WR, Santos DC, Lyra AA, Sueiro G, Betts R, Chagas DJ, Gomes JL, Bustamante JF, Tavares P (2012) Development of regional future climate change scenarios in South America using the Eta CPTEC/HadCM3 climate change projections: climatology and regional analyses for the Amazon, São Francisco and the Paraná River basins. Clim Dyn 38:1829–1848
Matsuura K, Willmott CJ (2009) Terrestrial precipitation gridded monthly time series (Version 2.01). Newark: Center for Climatic Research, Department of Geography, University of Delaware, 2009. http://climate.geog.udel.edu/∼climate/. Accessed 21 Nov 2014
Mesinger F, DiMego G, Kalnay E, Mitchell K (2006) North American regional reanalysis. Bull Am Meteorol Soc 87(3):343
Multivariate Enos Index (MEI). http://www.esrl.noaa.gov/psd/data/climateindices/mei/. Accessed 23 Nov 2014
NOAA/NCEP GHCN CAMS Monthly Temperature. http://iridl.ldeo.columbia.edu/SOURCES/.NOAA/.NCEP/.CPC/.GHCN_CAMS/.gridded/.deg0p5/. Accessed 25 Apr 2014
Peterson TC, Karl TR, Jamason PF, Knight R, Easterling DR (1998) First difference method: maximizing station density for the calculation of long-term global temperature change. J Geophys Res 103:967–974
Prakash S, Mitra AK, Momin IM, Rajagopal EN, Basu S, Collins M, Turner AG, Achuta RK, Ashok K (2015) Seasonal intercomparison of observational rainfall datasets over India during the southwest monsoon season. Int J Climatol 35:2326–2338
Reboita MS, Gan MA, Rocha RP, Ambrizzi T (2010) Regimes de precipitação na América do Sul: uma revisão bibliográfica. Rev Bras Meteorol 25:185–204
Rocha EJP (2001) Balanço de umidade e influência de condições de umidade e influência de condições de contorno superficiais sobre a precipitação da Amazônia. São José dos Campos: INPE, p 210 (INPE-10243-T.DI/904)
Ropelewski CF, Janowiak JE, Halpert MS (1984) The Climate Anomaly Monitoring System (CAMS), report. Climate Analysis Center, NWS, NOAA, Washington, DC
Rudolf B, Schneider U (2005) Calculation of gridded precipitation data for the global land-surface using in situ gauge observations. In: Proceedings of the 2nd workshop of the international precipitation working group IPWG, Monterey, p 231–247
Rudolf B, Hauschild H, Rueth W, Schneider U (1994) Terrestrial precipitation analysis: operational method and required density of point measurements. In Desbois M, Desalmond F (eds) Global precipitations and climate change. NATO ASI Series I, vol 26. Springer, Berlin, pp 173–186
Saad SI, Rocha HR, Silva Dias MAF (2010) Can the deforestation breeze change the rainfall in Amazonia? A case study for the BR-163 highway region. Interact Terra 14:1–25
Schneider U, Becker A, Finger P, Meyer-Christoffer A, Ziese M, Rudolf B (2014) GPCC’s new land surface precipitation climatology based on quality-controlled in situ data and its role in quantifying the global water cycle. Theor Appl Climatol 115:15–40
Shepard D (1968) A two-dimensional interpolation function for irregularly-spaced data. In: Proceedings of the 1968 23rd ACM National Conference, ACM Press, New York, pp 517–524
Silva CMS, Lúcio PS, Spyrides MHC (2012) Distribuição espacial da precipitação sobre o Rio Grande do Norte: estimativas via satélites e medidas por pluviômetros. Revista Brasileira de Meteorologia 27(3):337–346
Souza EB, Kayano MT, Tota J, Pezzi L, Fischi G, Nobre C (2000) On the influences of the El Niño, La Niña and Atlantic Dipole pattern on the Amazonian rainfall during 1960–1998. Acta Amaz 30(2):305–318
Sun Q, Miao C, Qingyun D, Dongxian K, Aizhong Y, Zhenhua D, Wei G (2014) Would the ‘real’ observed dataset stand up? A critical examination of eight observed gridded climate datasets for China. Environ Res Lett 9:015001
Willmott CJ (1981) On the validation of models. Phys Geogr 2:184–194
Willmott CJ, Matsuura K (1995) Smart interpolation of annually averaged air temperature in the United States. J Appl Meteorol 34:2577–2586
Willmott CJ, Robeson SM (1995) Climatologically aided interpolation (CAI) of terrestrial air temperature. Int J Climatol 15:221–229
Willmott CJ, Matsuura K (2001) Terrestrial air temperature and precipitation monthly and annual time series (1950–1999) (version 1.02). Centre for Climate Research, University of Delaware, Newark, NJ, USA
Willmott CJ, Legates DR, Rowe CM, Philpot WD (1985) Small-scale climate maps: a sensitivity analysis of some common assumptions associated with grid-point interpolation and contouring. Amer Cartogr 12:5–16
Yoon JH, Zeng N (2010) An Atlantic influence on Amazon rainfall. Clim Dyn 34:249–264
Zeng N, Yoon JH, Marengo JA, Subramaniam A, Nobre CA, Mariotti A, Neelin JD (2008) Causes and impacts of the 2005 Amazon drought. Environ Res Lett 3:014002
Acknowledgements
The authors thank the Instituto Nacional de Meteorologia—INMET, the Global Precipitation Climatology Centre—GPCC and the University of Delaware—UDEL for the rainfall and air temperature data sets.
Author information
Authors and Affiliations
Corresponding author
Rights and permissions
About this article
Cite this article
Tostes, J.O., Lyra, G.B., Oliveira- Júnior, J.F. et al. Assessment of gridded precipitation and air temperature products for the State of Acre, southwestern Amazonia, Brazil. Environ Earth Sci 76, 153 (2017). https://doi.org/10.1007/s12665-017-6467-2
Received:
Accepted:
Published:
DOI: https://doi.org/10.1007/s12665-017-6467-2