Abstract
Emerging as an important issue in the disciplines of landscape ecology and landscape hydrology which inspired it, defining the concept of landscape metrics in a hydrological context has become a challenge to both landscape planners and engineers. Accordingly, the present study addresses the relationships existing between flooding phenomena and landscape metrics (shape index, fractal dimension index, perimeter-area ratio, related circumscribing circle, and contiguity index) of land use/land cover, hydrological soil groups and geological permeability classes. A regionalization approach was adopted employing 39 select catchments (33—4800 km2 in area, 0.47—21 m3 s−1 in mean discharge), located within the southern basin of the Caspian Sea. These catchments were predominantly covered by forest (57.4%), while rangeland, farmland and urban areas accounted for 25.9%, 11.7%, and 1.6%, respectively. Class-level landscape structural metrics of land use/land cover, hydrological soil groups and geological permeability classes have then been served as inputs to stepwise multiple linear regression analysis in an attempt to explain the flood magnitudes. The regression models (0.69 ≤ r2 ≤ 0.84) suggested that the catchments’ flood magnitude could explicitly be predicted using average measure of the shape and related circumscribing circle indices for the land use/land cover classes and those of hydrologic soil groups and geological permeability classes of the catchments. This indicated that regularity (vs. irregularity) of the landscape, pedoscape, and lithoscape, as represented by the shape index as well as the circumscribing circle index (for elongation and convolution), explained 69–84% of the variation in the flood magnitudes in the catchment.
Similar content being viewed by others
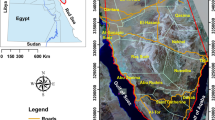
Avoid common mistakes on your manuscript.
1 Introduction
Over the past three decades statistical measures of landscapes’ structure, composition and configuration-related attributes have proliferated under extensive efforts to provide landscape metrics for land and water resource management applications (McGarigal et al. 2002; Jaeger 2000; He et al. 2000; Gustafson 1998; McGarigal and Marks 1995; Li and Reynolds 1995; Baker and Cai 1992; Turner and Gardner 1991; Turner 1990; O’Neill et al. 1988). However, establishing such metrics’ meanings in an ecological context in general or more specifically in a hydrological context remains in its infancy. Given the well-documented relationship between pattern and process, investigators have hypothesized that a given landscape’s attributes contribute to the hydrological processes occurring within it, and more specifically to the magnitude of floods at the catchment scale. Such floods arise through a complex interaction between exogenous factors (e.g., meteorological events) and indigenous factors (Nied et al. 2013). The indigenous factors are related to catchment geometrics including but not limited to size, shape, drainage density, length of the main stream and. The ecological attributes of the catchment such as land use/land cover (LULC), soil characteristics and geology. A number of studies have sought to determine whether any significant relationship exists between catchment geometrics and flood discharge (e.g. see Pfaundler 2001). Most of these have investigated regression models developed to predict different flood discharge recurrence periods based on ecological attributes such as soil and geology (e.g. see Merz and Blöschl 2005; Uhlenbrook et al. 2002; Pfaundler 2001).
Structure (shape), composition and configuration of a landscape are accounted as three key features (Amiri et al. 2016; Forman and Godron 1986), which can be analyzed and described using the appropriate landscape metrics (Rutledge 2003). Accordingly, a plenty of the landscape metrics has already been developed, by which structure (shape), composition and configuration of a given landscape can quantitatively be described. Of these, landscape structure has the greatest influence on water and nutrient flow in catchments (Amiri and Nakane 2009; Uuemmaa et al. 2007; Wickham et al. 2002; Turner and Rabalais 2003). The study of landscape structure examines the spatial relationships between different shapes of land use and land cover patches within a catchment, focusing on the distribution of energy and material flows according to the shape of those patches. Given that the geometrical and morphometric features of a patch can affect the landscape functions in terms of energy and material flow (e.g., water and nutrients) within a catchment (Amiri and Nakane 2009; Kim 2005), it has been suggested that landscape metrics could provide scientists and practitioners with reliable information for the management of stream water quality (Amiri and Nakane 2009; Uuemmaa et al. 2007). There is an increasing demand for the development of appropriate indicators and methods to evaluate the influence of landscape on fresh water quality and its management (Kearns et al. 2005; Griffith 2002). In fact, Kearns et al. (2005) proposed a screening method for applying landscape metrics to fresh water research and management.
While the application of catchment geometric features to the regionalization of flood discharge patterns originated several decades ago, using landscape ecology-based metrics to explain the relationship between change in land cover and in-stream water quality and quantity (Amiri and Nakane 2009; Moreno et al. 2007; Uuemaa et al. 2005) only began around 2010. Still, in its infancy, this approach has yet to provide hard evidence to link landscape metrics with catchment hydrological responses such as flood discharge. While hundreds of landscape metrics have been developed, these can generally be categorized into three types: structure-, composition- and configuration-related metrics (Turner et al. 2001; Rutledge 2003).
Although LULC had been focusing by landscape ecology since its emergence, extending the fundamental concepts of landscape ecology into soil and geology, which are situated beneath the LULC, the terms of pedoscape and lithoscape are introduced by which spatial variations in the soil and geology can be addressed through borrowing the metrics from landscape ecology. It is hypothesized that alongside the exogenous factors, the spatial variations in attributes of soil and geology might effect on flood generation at catchment scale. As a considerable number of these metrics have not been explicitly defined in an ecological context, defining their ecological meaning and interpretation has progressed little.
Apart from the dominance of percentage-based landscape compositional metrics and applying catchment geometrics including but not limited to catchment area, slope, drainage density, slope of the land, initial moisture content, rainfall patters, which can be accounted as prevalent describers of flood magnitudes in regionalization work (e.g. see Merz and Blöschl 2005; Uhlenbrook et al. 2002; Pfaundler 2001), few, if any studies have sought to address the critical question of whether catchments’ structural landscape attributes contribute to flood generation in absence of the afore-mentioned customary variables. Hence, the current study’s main objectives were to: (i) explore whether there exists a significant association between flood discharges of different recurrence periods and patch level landscape structural metrics associated with the catchment’s ecological attributes [e.g., LULCs, hydrologic soil groups (HSGs), and geological permeability classes (GPCs)] (ii) develop models by which changes in the recurrence period of flood discharges could be explained by changes in the landscape structural metrics in in absence of the traditional variables.
2 Material and Methods
2.1 Study Area
Situated in the southern basin of the Caspian Sea, the study area (Fig. 1) covers 38,467 km2 (lat: 35°36′-37°19′ E, long: 49°48′-54°41’ N) across a wide range of elevations (−16 m—4782 m AMSL). The basin’s diverse landscape encompasses the administrative boundaries of two provinces, housing 5,554,817 inhabitants (ISC 2012). While 59 rivers drain precipitation from within the basin to the Caspian Sea, limitations in data access and the need to achieve a homogeneous data set led to only 39 catchments being investigated in the present study. Varying in the area (32 to 2325 km2) and in 40-year (1971–2010) mean discharge (0.47–21 m3 s−1), these catchments are dominated by forest cover (57.4%), while rangeland, farmland and urban areas account for 25.9%, 11.7%, and 1.6%, respectively. Bodies of water (e.g., wetlands) represent less than 1% of the total area. The underlying bedrock is predominantly made up of granite and andesite. A moderately permeable soil (C group) (USDA 1986), covers 53.9% of the catchment and is, therefore, the dominant soil type in terms of its hydrological behaviors (Fig. 1 and Table 1).
2.2 Data Sets
Digital elevation models (30 m × 30 m) downloaded from the USGS served to delineate upstream catchment boundaries. A digital LULC map (2002; scale 1:250, 000) was obtained from the Forest, Range, and Catchment Management Organization of Iran (http://frw.org.ir). Land suitability and geological maps (scale 1:250,000) were obtained from the Iranian Soil and Water Research Institute (http://www.swri.ir) and Geological Survey of Iran (http://www.gsi.ir), respectively. River discharge data (1971–2010) was provided by the Water Resources Management Company of Iran (http://www.wrm.ir). The present study is mainly based on secondary data sets (Amiri and Nakane 2008), where the data have already been handled by the relevant institutions in terms of sampling, analyzing, storing and publishing.
2.3 Methods
To conduct the present study, a secondary database (Sliva and Williams 2001; Amiri and Nakane 2008) was employed. Accordingly, all the information layers were first transformed into a common digital format, then co-registered with the WGS84 source (zone 39n). The upper catchment boundaries were then delineated by applying a digital elevation model (USGS) for each river gauging station.
To conduct the present study with more homogeneous catchments, only those with 40 years of continuous hydrological data (i.e., 1971–2010) and areas within the range μ ± 3δ (where μ is the mean catchment area, and δ the standard deviation of the mean) were included. Accordingly, 39 out of 56 rivers were selected (Fig. 1). Sampling processes and devices were in conformity with WRMC Guidelines for Surface Water Quality Monitoring (2009) and EPA-841-B-97-003 standards (Dohner et al. 1997).
Based on the previous studies (Mahdavi et al. 2010; Gholami et al. 2016; Yazdani and Sheikh 2017), Pearson Type III Distribution was applied to estimate flood magnitudes for 2-, 5-, 25-, 50-, 100-, and 200-year recurrence periods for each of the catchments. Accordingly, the 2-, 5-, 25-, 50-, 100-, and 200-year recurrence period flooding discharges were estimated (USGS 1982; Burn and Goel 2001) as q2, q5, q25, q50, q100, and q200, respectively (Table 1).
The LULC map (http://frw.org.ir, 2002) was aggregated into ten classes, including:
-
Forest [low density (0–25%), moderate density (25.1–50%), high density (501.-75%)] (Sangani et al. 2015); abbreviated as F1, F2, F3) (Table 2),
-
Rangeland [low density (0–25%), moderate density (25.1–50%), high density (501.-75%)] (Sangani et al. 2015); abbreviated as R1, R2, R3) (Table 2),
-
Urban (abbreviated U) (Table 2), along with,
-
Agricultural lands (abbreviated as A) (Table 2),
-
Water bodies, and
-
Barren.
Reclassification of the land suitability map (http://www.swri.ir) was also conducted to generate a map HSGs, with four soil group classes: S1, S2, S3 and S4 (USDA 1986; Sangani et al. 2015) (Table 2), whose infiltration rate decrease, respectively and representing as low infilterable soil (S1), moderate infilterable soil (S2), high infilterable soil (S3) and very high infilterable soil (S4).
Digital geological maps (http://www.gsi.ir, scale 1:250,000), documenting 19 geological classes (Sangani et al. 2015) were reclassified into three GPCs based on effective porosity, type, size and connectivity of cavities, rock density, pressure gradient and features of the fluid (i.e., viscosity; Fatehi et al. 2015) using Spatial tools in ArcGIS 10.9. The re-classified maps of GPCs illustrate spatial variations of geological permeability across the study area, represented by 3 geological permeability classes, which are named as low permeable rock (G1), moderate permeable rock (G2), high permeable rock (G3) (Table 2).
Maps of the LULC, HSGs, and GPCs were then overlaid on a catchment boundary map, in order to calculate the true extent (%) of each ecological attribute (LULC, HSG and GPC classes) within the catchments. Thereafter, the landscape structural metrics of shape index (shp), fractal dimension index (frac), perimeter-area ratio (para), related circumscribing circle (rcc), and contiguity index (ci) were calculated at the class level for each of the catchment’s ecological attributes (Table 2). Accordingly, this approach can generate 15 independent variables, out of which 7 variables are from land use/land cover classes, 4 variables from hydrological soil groups, and 3 variables from geological permeability classes, for each of the landscape structural metrics of shape index (shp), fractal dimension index (frac), perimeter-area ratio (para), related circumscribing circle (rcc), and contiguity index (ci). Normality of the dataset was tested using Kolmogorov-Smirnov for different recurrence periods (Table 3).
To be more specific, for the case of shape index (shp), this approach has generated 15 independent variables for a given catchment as following:
-
F1 shp , F2 shp , F3 shp , R1 shp , R2 shp , R3 shp , U shp , A shp from LULC map,
-
S1 shp , S2 shp , S3 shp , S4 shp from HSGs map, and
-
G1 shp , G2 shp , G3 shp , from GPCs map.
It is noteworthy that 15 independent variables have been generated for fractal dimension index (frac), perimeter-area ratio (para), related circumscribing circle (rcc), and contiguity index (ci), as well.
2.3.1 Landscape Metrics
Shape Index
This index measures the complexity of patch shape in comparison to a standard shape (square or almost square) of the same size (Forman and Godron 1986). It can be calculated by the following formula:
where shp is shape index, N i is the number of patches of category i (here i = F1, F2, F3, R1, R2, R3, U, A, S1, S2, S3, S4, G1, G2, and G3), L i is the perimeter and A i is the area of each patch in a given category. Farina (2000) noted that the farther shp is from one, the more the patch deviates from an iso-diametric shape. Moreover, shp is also applied to assess the shape of a patch using the spatial connectedness of cells or patches at the patch or landscape level, respectively (Turner et al. 2001). The value of the index ranges between 0 (for a perfectly square shape) up to ∞ for a highly irregular shape (Rutledge 2003).
Fractal Dimension Index
This index represents the degree of shape complexity for patches of a given landscape category. The index varies (1 ≤ frac ≤ 2), and measures shape complexity of a patch or a set of patches (of a given class) (Rutledge 2003; Turner et al. 2001). If the index approaches one, the patch is regular (square) in shape; as it approaches its higher limit it represents a more irregular (convoluted) patch (Rutledge 2003). The frac can be calculated as:
where, frac is fractal dimension index, while P ij is perimeter (m) of patch ij and a ij stands for area (m2) of patch ij (McGarigal and Marks 1995). The frac is sensitive to the resolution of the study, since finer resolutions often result in finer details, thereby affecting the perimeter-area ratio (Rutledge 2003).
Perimeter-Area Ratio
The para is a simple measure of shape complexity, but without standardization to a simple Euclidean shape. It represents the ratio of patch perimeter (m) to the area (m2) for a given landscape category. Therefore, for a given shape, it depends on the patch area (McGarigal and Marks 1995; Rutledge 2003). The para can be calculated as (McGarigal and Marks 1995):
where, P ij is perimeter (m) of patch ij, and A ij is the perimeter (m) of patch ij, and i and j are the identifying column and row numbers of a given grid for the patch in question. The value of the index varies in the range 0 < para < +∞.
Related Circumscribing Circle
The RCC measures to what extent the shape of a given patch deviates from a convoluted shape and approaches a narrow and elongated one (Turner et al. 2001). Varying between 0 for convoluted patches to 1 for narrow elongated ones (Rutledge 2003), the RCC is calculated as:
where, a ij is the area (m2) of patch ij, and \( {a}_{ij}^s \) is the area (m2) of the smallest circumscribing circle around patch ij, and i and j are the identifying column and row numbers of a given grid for the patch in question. (McGarigal and Marks 1995).
Contiguity Index
Spatial connectedness of cells within a grid-format patch can be assessed by CI. This metric can provide a measure of patch boundary configuration and patch shape, as well (McGarigal and Marks 1995). CI is calculated as:
where, C ijr is the contiguity value for pixel r in patch ij, v is sum of the values in a 3-by-3 cell template, and a ij is the area of patch ij in terms of number of cells. For a single pixel patch the CI = 0, and approaches an upper limit of 1.0 as patch contiguity, or connectedness, increases (McGarigal and Marks 1995).
-
To determine the linkage between landscape metrics of a given catchment’s ecological attributes and flood magnitudes, multiple regression modeling methods (linear, logarithmic, power and exponential) were applied using a stepwise regression analysis (entry criterion P ≤ 0.05, exclusion criterion P ≥ 0.100) to develop multiple linear regression models through which the magnitude of floods (dependent variable; m3 s−1 km−2) could be explained by an individual pairing of landscape metrics (rcc, ci, frac, para, and shp) and LULCs, HSGs or GPCs category [e.g., Q = f (F1 shp , F2 shp , F3 shp , R1 shp , R2 shp , R3 shp , U shp , A shp , S1 shp , S2 shp , S3 shp , S4 shp , G1 shp , G2 shp , G3 shp ) as independent variables]. This would yield the general equation:
where
- y i :
-
is the ith flood magnitude by area of the catchment (m3 s−1 km−2),
- x1 … xn:
-
are the catchment landscape metrics (rcc, ci, frac, para, and shp)
- β1 … βn:
-
are the coefficients of the catchment landscape metrics retained, with P ≤ 0.05
- β0:
-
is a constant, with P ≤ 0.05, and
- εi:
-
is the error for the ith flooding attributes.
Inter-variable collinearity of the developed models was assessed using the variation inflation factor (VIF), where a VIF < 10 for all model parameters indicated a lack of collinearity (Neter et al. 1996; Chatterjee et al. 2000). The models’ goodness of fit was evaluated using scatter plots of observed vs. predicted values (Fig. 2) (Ahearn et al. 2005). The last critical criteria for choosing a candidate model was that it made sense from the standpoint of landscape ecology. All statistical analyses were conducted by IBM SPSS for Windows, Release 19. Fragstat was used to calculate landscape metrics of catchment ecological attributes (LULCS, HSGs, and GPCs).
3 Results
Significant stepwise regression procedure-based models were derived for the 2-, 5-, 10-, 25-, 50-, 100- and 200-year flood magnitude applying five landscape structural metrics, namely Shape Index (shp), Fractal Dimension Index (frac), Perimeter-Area Ratio (para), Related Circumscribing Circle (rcc), Contiguity Index (ci) for LULC, soil and geology (Eqs. 7, 8, 9, 10, 11, 12 and 13). Further statistics for these models can be found in Table 4. The following are multiple linear regression models by which variations in the annual peak discharge have been explained by changes in landscape structural metrics of catchments:
Where, logq 2 … logq 200 is the base 10 logarithm of the 2-, …, 200-year recurrence period flood’s magnitude (m3 s−1 km−2) for the given catchment. Other variables have been described in the earlier section.
It should be noted that (ir) regularity of a given landscape’s patches, regardless of their prevalent functions, might provide a different insight as to their function in the new arena of landscape hydrology. These contradictory functions can obviously be inferred from the role of the shape index of the high density forest patches (Eq. 8). Although the alleviating impacts of forested lands on peak flow of streams and rivers is well documented (Jones and Grant 1996; Alila et al. 2009; Amatya et al. 2011), the model suggests that the level of overall complexity of the forest patches might be of considerable importance in explaining variations in the magnitude of logq5. Accordingly, if the shape index of the high density forest patches (F1 shp ) increases in the catchments, it might contribute to an increase in the magnitude of logq5 due to the greater irregularity in the shape of the forest patches. Moreover, one might expect the flood-alleviating function of forest patches to be enhanced, if they are laid out in a more regular pattern or shape in a given landscape.
About 84% of the total variations in the magnitude of logq10 (Eq. 9) were explained by the values of the landscape-related metrics (F1 shp , R1 shp , R3 shp and A shp ) and the lithoscape-related metrics (G1 shp and G2 shp ), in absence of any pedoscape-related metrics. The distinct difference between Eq. 9 (logq10) and Eq. 8 (logq5) is that the shape index of agricultural patches has an influence on the magnitude of logq10, but not on that of logq5. The logq10 shows an inverse association with A shp , the shape index of agricultural patches, indicating that the lower the shape index of agricultural patches (i.e., the more regularly-shaped the agricultural patches are), the greater the magnitude of logq10. Therefore, only the addition or alteration of agricultural lands into regularly-shaped patches (i.e., square or close to square) would likely reduce the magnitude of 10-year recurrence period floods in the catchments.
Of the total variation in the magnitude of the catchments’ logq25 (Eq. 10), about 79% was explained by the values of landscape-related metrics (F1 cir , R1 cir and R3 cir ), a pedoscape-related metric (S4 cir ) and the lithoscape-related metrics (G2 cir and G3 cir ). Moreover, the mean related circumscribing circle index (RCC) for the R1 cir and R3 cir (high and low density rangeland patches, respectively) along with those of G2 cir and G3 cir (moderately and highly permeable geological patches, respectively) were inversely related to the magnitude of logq25 in the catchments. An increase in the mean RCC values of these landscape and lithoscape-related metrics would therefore result in a decrease in the magnitude of logq25 in the catchments. Based on the definition of the RCC, the narrower and more elongated high density forest (F1 rcc ) and low permeable soil (S4 rcc ) patches are, the greater the magnitude of logq25 would be in the catchments. This indicates that compared to those of relatively convoluted shape, high density forest patches of relatively narrow and elongated shape would not contribute significantly to the infiltration process. The model also suggests that the narrower and more elongated the high and low density rangeland patches (R1 rcc and R3 rcc ) are, the lower the magnitude of logq25 would be in the catchment.
Of the total variation in the magnitude of logq50 about 81% was significantly explained by a combination of landscape-related metrics (A shp , R2 shp and R3 shp ), pedoscape-related metrics (S4 shp ) and lithoscape-related metrics (G2 shp and G3 shp ) (Table 4). In this case, all landscape, pedoscape and lithoscape-related metrics were inversely related to the magnitude of logq50 in the catchments. As for agricultural patches, an increase in their irregularity would result in a decrease in the magnitude of logq50 in the catchments. This implies that only those agricultural patches of more regular shape might contribute to the magnitude of 50-year recurrence period floods.
The equations predicting the magnitude of logq 100 (Eq. 12, r2 = 0.79, p-value<0.005) and logq 200 (Eq. 13, r2 = 0.76, p-value<0.005) are quite similar in terms of model structure, i.e., number and type of variables, and nature of relationship (direct or inverse) with dependent variables.
Only the rcc index of the low permeable soil patches (S4 rcc ) was positively related to the magnitudes of logq 100 or logq 200 in the catchments. Accordingly, it implies that only an elongated low permeable soil patch would contribute runoff generation, while a convoluted low permeable soil patch would have a contributing role on infiltration process. It has indicted that low permeable soil might represent two distinct functions on the basis of their shapes in the catchments.
Findings (Eq. 12, r2 = 0.79, p-value<0.005) also suggest that there is a direct relationship between F1rcc and logq 100 . It means that decreasing the measure of F1rcc, which would result in a more convoluted high density forest patches in the catchments, could contribute infiltration process. In contrast, runoff generation might be contributed by increasing in the measure of the measure of F1rcc, which would result in a more elongated high density forest patches. Accordingly, based on to what extent the high density forest patches are of an elongated or a convoluted shape, two different hydrological functions might be expected in the catchments.
4 Discussion
In summary, only two of five landscape ecology-related metrics (including shape and related circumscribing circle indices) employed in the present study yielded reliable models for determining flood magnitudes in the study area at different recurrence periods. With the exception of flood recurrence periods of 50 or more years, the shape index for different landscape, pedoscape and lithoscape categories could be applied in developing predictive models for low magnitude floods of 2-, 5- or 10-year recurrence periods. However, for medium magnitude floods (2-, 5- or 10-year recurrence period) and high magnitude floods (including 100- and 200-year recurrence periods), the shape index was not determinative of flood magnitude, whereas the RCC was.
For the low-magnitude floods, the present study showed that agricultural and rangeland patches’ degree of deviation from an iso-diametric shape (square) affected the magnitude of 2-, 5- and 10-year recurrence period floods. More specifically, the closer these within-catchment patches approached an iso-diametric shape, the greater the magnitude of floods of 2-, 5- and 10-year recurrence. This contrasts with the findings and privileged views of previous studies (e.g., see O’Connell et al. 2007), which suggested that rangeland patches contributed to infiltration during the rainfall-runoff process much more so than agricultural patches.
However, (ir) regularity in the shape of forest patches, in particular, the degree by which forest patches deviate from an iso-diametric shape as represented by differences in shape index, were found to be closely tied to the extent of low magnitude floods in the catchments. The present results indicate that an increasing degree of irregularity in forest patches could cause their role to deviate from their privileged function of alleviating floods and lowering their magnitudes. Otherwise stated, the flood-alleviating function of the forest patches in catchments could be somewhat compromised if these patches were of a more regular shape. Nevertheless, it should be noted that shape-dependent functions of high density forest, while significant variables for low and medium magnitude flood-predicting models are not so for high magnitude flood-predicting models. This concurs with the work of Bathurst et al. (2011) who showed that the extent of forest cover might not have a significant effect on peak discharges, which have a recurrence period of over 10 years.
For medium and high magnitude floods, the present findings indicate that the RCC for some of landscape, pedoscape and lithoscape categories could provide predictive variables for reliable models. The more convoluted the shape of the rangeland patches, the greater the number of medium and high magnitude floods occur in the catchment. However, established theory and research suggests that a greater expanse of rangelands contributes to decreasing peak flows (e.g., see Sikka et al. 2003). Therefore, expanded rangelands might only help in reducing peak flows for medium and high magnitude floods in the catchment of interest if they were of a narrower and more elongated shape.
Though additional forest patches would be expected to alleviate the risk of medium and high magnitude floods in the catchment, land cover changes, in particular reforestation initiatives, should ensure that these changes do not result in narrow and elongated forest patches, as these would not contribute to the alleviation of medium and high magnitude floods, if anything, the contrary. Although there are studies (e.g., see Bathurst et al. 2011) that show forest cover might have a negligible effect on the peak flow discharge for recurrence periods exceeding 10 years, the findings of the present study indicate that besides percent forest cover, the shape of the forest patches should be considered as well.
Another point that must be noted regarding the developed models is that among the four hydrologic soil groups (S1, S2, S3 and S4), the low permeable soil patches (S4) can be considered as a significant variable for explaining the total variations in the magnitude of 25-, 100- to 200- year recurrence period floods, but not for the 50- year recurrence period floods, in the catchments under study. Specifically, a more elongated shape for the low permeable soil patches would enhance the magnitude of 25-, 100- and 200-year recurrence period flood in the catchments under study.
5 Conclusions
Findings of the present study showed that out of five landscape ecology-inspired metrics the landscape structural metrics of shape and related circumscribing circle are significant factors in explaining hydrological responses in general and extreme responses in particular. Therefore they should be considered in determining the magnitude of floods of different recurrence periods. Moreover, it suggests that not only percent area of land use/land cover, soil type, and geology can explain total variations in the flood magnitudes, but also shape, and related circumscribing circle indices of the land use/land covers, hydrologic soil groups, and geological permeability classes. It also indicates that (ir) regularity in shape of a given patch (shape index), and its elongation and convolution (related circumscribing circle index), for different landscape, pedoscape and lithoscape categories, can influence the categories’ functions (flood alleviation/amplification) at a landscape (catchment) scale.
To gain a greater insight into the applicability of landscape metrics, further studies are recommended, aiming at to find out whether landscape configuration-representing metrics and landscape composition-representing metrics might any potential to be applied in explaining hydrological process in catchments.
References
Ahearn DS, Sheibley RW, Dahlgren RA, Anderson M, Johnson J, Tate KW (2005) Land use and land cover influence on water quality in the last free-flowing river draining the western Sierra Nevada, California. J Hydrol 313(3-4):234–247
Alila Y, Kuraś PK, Schnorbus M, Hudson R (2009) Forests and floods: A new paradigm sheds light on age-old controversies. Water Resour Res 45(8):W08416
Amatya DM, Douglas-Mankin KR, Williams TM, Skaggs RW, Nettles JE (2011) Advances in forest hydrology: Challenges and opportunities. Trans ASABE 54(6):2049–2056
Amiri BJ, Fohrer N, Cullmann J, Hörmann G, Müller F, Adamowski J (2016) Regionalization of Tank Model Using Landscape Metrics of Catchments. Water Resour Manag 30(14):5065–5085
Amiri BJ, Nakane K (2008) Entire catchment and buffer zone approach to modeling linkage between river water quality and land cover—a case study of Yamaguchi Prefecture, Japan. Chin Geogr Sci 18(1):85–92
Amiri BJ, Nakane K (2009) Modeling the linkage between river water quality and landscape metrics in the Chugoku district of Japan. J Water Resour Manag 23:931–956
Baker WL, Cai Y (1992) The programs for multiscale analysis of landscape structure using the GRASS geographical information system. Landsc Ecol 7:291–302
Bathurst JC, Iroumé A, Cisneros F, Fallas J, Iturraspe R, Novillo MG, Urciuolo A et al (2011) Forest impact on floods due to extreme rainfall and snowmelt in four Latin American environments 1: Field data analysis. J Hydrol 400(3):281–291
Burn DH, Goel NK (2001) Flood Frequency Analysis for the Red River at Winnipeg, Canadian J. Engineering 28:355–362
Chatterjee S, Hadi AS, Price B (2000) The Use of Regression Analysis by Example. John Wiley and Sons, New York
Dohner E, Markowitz A, Barbour M, Simpson J, Byrne J, Dates G (1997) Volunteer stream monitoring: a methods manual environmental protection agency: office of water (EPA 841-B-97-003). Environmental Protection Agency: Office of Water and Washington, DC
Farina A (2000) Principles and Methods in Landscape Ecology, Kluwer Academic Publisher, The Netherlands, pp 154–155
Fatehi I, Amiri BJ, Alizadeh A, Adamowski J (2015) Modeling the Relationship between Catchment Attributes and In-stream Water Quality. Water Resour Manag 29(14):5055–5072
Forman RTT, Godron M (1986) Landscape Ecology. Wiley, New York
Gholami V, Asghari A, Taghvaye Salimi E (2016) Flood hazard zoning using geographic information system (GIS) and HEC-RAS model (Case study: Rasht City). Caspian Journal of Environmental Sciences 14(3):263–272
Griffith J (2002) Geographic techniques and recent applications of remote sensing to landscape-water quality studies. Water Air Soil Pollut 138:181–197
Gustafson EJ (1998) Quantifying landscape spatial pattern: what is the state of the art?. Ecosystems 1(2):143–156
He HS, DeZonia BE, Mladenoff DJ (2000) An aggregation index (AI) to quantify spatial patterns of landscapes. Landsc Ecol 15:591–601
ISC (2012) Iran statistical yearbook. Iran Statistical Center, Tehran
Jaeger JAG (2000) Landscape division, splitting index, and effective mesh size: new measures of landscape fragmentation. Landsc Ecol 15:115–130
Jones JA, Grant GE (1996) Peak flow responses to clear-cutting and roads in small and large basins, western Cascades, Oregon. Water Resour Res 32(4):959–974
Kearns FR, Maggi KN, Carter JL, Resh VH (2005) A method for the use of landscape metrics in freshwater research and management. Landsc Ecol 20:113–125
Kim JK (2005) Exploring the effects of local development regulations on ecological landscape structure. Ph.D. thesis, Texas A&M University, p 184, Seen 27 February 2013 at http://repository.tamu.edu//handle/1969.1/2403
Li H, Reynolds JF (1995) On definition and quantification of heterogeneity. Oikos 73:280–284
Mahdavi M, Osati K, Sadeghi SAN, Karimi B, Mobaraki J (2010) Determining suitable probability distribution models for annual precipitation data (a case study of Mazandaran and Golestan provinces). Journal of Sustainable Development 3(1):159
McGarigal K, Cushman SA, Neel MC, Ene E (2002) FRAGSTATS: spatial pattern analysis program for categorical maps. Computer software program produced by the authors at the University of Massachusetts, Amherst, available at the following web site: http://www.umass.edu/landeco/research/fragstats/fragstats.html
McGarigal K, Marks BJ (1995) FRAGSTATS: Spatial Analysis Program for Quantifying Landscape Structure. USDA Forest Service General Technical Report PNW-GTR-351, Gustafson, 1998
Merz R, Blöschl G (2005) Flood frequency regionalisation—spatial proximity vs. catchment attributes. J Hydrol 302(1):283–306
Moreno D, Pedrocchi C, Comin FA, Cabezas A (2007) Creating wetlands for the improvement of water quality and landscape restoration in semi-arid zones degraded by intensive agricultural use. Ecol Eng 30(2):103–111
Neter J, Kutner HM, Nachtsheim CJ, Wasserman W (1996) Applied Linear Statistical Models. Irwin, Chicago
Nied M, Hundecha Y, Merz B (2013) Flood-initiating catchment conditions: a spatio-temporal analysis of large-scale soil moisture patterns in the Elbe River basin. Hydrol Earth Syst Sci 17(4):1401–1414
O’Connell PE, Ewen J, O’Donnell G, Quinn P (2007) Is there a link between agricultural land-use management and flooding? Hydrol Earth Syst Sci 11(1):96–107
O’Neill RV, Krummel JR, Gardner RH, Sugihara G, Jackson B, DeAngelis DL, Milne BT, Turner MG, Zygmunt B, Christensen SW, Dale VH, Graham RL (1988) Indices of landscape pattern. Landsc Ecol 1:153–162
Pfaundler M (2001) Adapting, analysing and evaluating a flexible index flood regionalisation approach for heterogeneous geographical environments. Diss., Technische Wissenschaften ETH Zürich, Nr. 14253, https://doi.org/10.3929/ethz-a-004176052
Rutledge DT (2003) Landscape indices as measures of the effects of fragmentation: can pattern reflect process? Department of Conservation, Wellington
Sangani MH, Amiri BJ, Shabani AA, Sakieh Y, Ashrafi S (2015) Modeling relationships between catchment attributes and river water quality in southern catchments of the Caspian Sea. Environ Sci Pollut Res 22(7):4985–5002
Sikka AK, Samra JS, Sharda VN, Samraj P, Lakshmanan V (2003) Low flow and high flow responses to converting natural grassland into bluegum (Eucalyptus globulus) in Nilgiris catchments of South India. J Hydrol 270(1):12–26
Sliva L, Williams DD (2001) Buffer zone versus the whole catchment approaches to studying land use impact on river water quality. Water Res 35(14):3462–3472
Turner MG (1990) Spatial and temporal analysis of landscape patterns. Landsc Ecol 4:21–30
Turner MG, Gardner RH (1991) Quantitative Methods in Landscape Ecology. Springer-Verlag, New York
Turner MG, Gardner RH, O'neill RV (2001) Landscape ecology in theory and practice, vol 401. Springer, New York
Turner RE, Rabalais NN (2003) Linking Landscape and Water Quality in the Mississippi River Basin for 200 Years. Bioscience 53(6):563–572
Uhlenbrook S, Steinbrich A, Tetzlaff D, Leibundgut C (2002) Regional analysis of the generation of extreme floods. International Association of Hydrological Sciences, Cape Town, Publication 274:243–249
USDA (1986) Urban hydrology for small catchments, Technical Release 55 (TR-55) (Second ed.). Natural Resources Conservation Service, Conservation Engineering Division, Washington, DC
USGS (1982) Guidelines for determining flood flow frequency. Office of Water Data Coordination, Bulletin B, 17B, Virginia
Uuemaa E, Roosaare J, Mander U (2005) Scale dependence of landscape metrics and their indicatory value for nutrient and organic matter losses from catchments. Ecol Indic 5:350–369
Uuemmaa E, Roosaare J, Mander U (2007) Landscape metrics as indicators of river water quality at the catchment scale. Nord Hydrol 38(2):125–138
Wickham JD, Wade TG, Riitters KH, O’Neill RV, Smith JH, Smith ER, Jones KB, Uhlenbrook S, Steinbrich A, Tetzlaff D, Leibundgut C (2002) Regional analysis of the generation of extreme floods. International Association of Hydrological Sciences, Publication 274:243–249
Yazdani MR, Sheikh Z (2017) Applying geostatistical methods for analyzing regional flood frequency in North of Iran (Case Study: Mazandaran Catchments). J Agric Sci Technol 19:861–875
Acknowledgements
The first author (B.J.A.) acknowledges that the present study has financially been supported through visiting professor fellowship which has been awarded by Chinese Academy of Sciences (CAS) in Nanjing Institute of Geography and Limnology.
Author information
Authors and Affiliations
Corresponding author
Rights and permissions
About this article
Cite this article
Amiri, B.J., Junfeng, G., Fohrer, N. et al. Regionalizing Flood Magnitudes using Landscape Structural Patterns of Catchments. Water Resour Manage 32, 2385–2403 (2018). https://doi.org/10.1007/s11269-018-1935-3
Received:
Accepted:
Published:
Issue Date:
DOI: https://doi.org/10.1007/s11269-018-1935-3