Abstract
Mono-centric regions have experienced a progressive transformation towards polycentric structures. Transition to discontinuous and dispersed spatial configurations was particularly evident in formerly compact and dense European cities, as a result of increasingly complex commuting patterns. Under the hypothesis that central locations have a balanced rate of resident population to internal workers and attract workers from neighbouring places, a multivariate analysis of commuting patterns may reveal latent transformations of compact, mono-centric cities towards polycentrism and settlement scattering. A more comprehensive understanding of the intimate nature and implications of urban transitions taking place in the last decades in Athens (Greece), a representative metropolitan region in southern Europe, was carried out using census data analyzed through a framework integrating descriptive statistics and mapping, multivariate exploratory data analysis, non-parametric inference and regression models. The analytical framework was proposed with the aim to assess commuting patterns vis à vis urban hierarchy in a context of (more or less) de-centralized growth. Empirical results for the study area indicate the persistence of a bi-centric spatial structure gravitating on the central cities of Athens and Piraeus, with early formation of employment sub-centres and local-scale alterations of the mono-centric scheme as a result of discontinuous urban expansion. Exurban development was associated with the formation of self-contained sub-centres with an increasing proportion of workers in total resident population.
Similar content being viewed by others
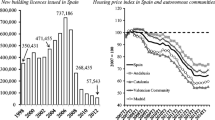
Avoid common mistakes on your manuscript.
1 Introduction
Experiencing a long transition toward more dispersed territorial configurations, contemporary cities in Europe have underwent intense socioeconomic transformations altering spatial distribution and density of economic functions (Leontidou et al. 2007; Páez 2007; Angel et al. 2011; Salvati and Gargiulo Morelli 2014). Abandoning compact and dense urban growth and mono-centric spatial organizations, European regions have becoming increasingly polycentric, thanks to exurban development, depolarization of central cities, businesses delocalization, planning deregulation, infrastructural development and increased access to private mobility (Beriatos and Gospodini 2004; Burgel 2004; Catalàn et al. 2008; Arapoglou and Sayas 2009; Ceccarelli et al. 2014). In this regard, settlement scattering and discontinuous city expansion led to multiple challenges for metropolitan regions and urban societies (Haase et al. 2010; Hayek et al. 2011; Pojani and Stead 2015; Cuadrado-Ciuraneta et al. 2017). Especially dense and mono-centric Mediterranean cities—likely the most compact and land-saving urban agglomerations in Europe—have underwent a (more or less) evident transition towards urban polycentrism and discontinuous low-density morphologies up to the early 1980s (Nuissl and Rink 2005; Paul and Tonts 2005; Balta and Eke 2011; Colantoni et al. 2016; Duvernoy et al. 2018).
Given the intimate relationship between urban form and socioeconomic functions, understanding causes and consequences of exurban development on metropolitan transformations is a priority of regional planning and decision-making (Giannakourou 2005; Schneider and Woodcock 2008; Terando et al. 2014; Zambon et al. 2018). Controlling discontinuous urban expansion in the light of sustainable development of metropolitan regions—intended as a challenging priority in the political agenda at various governance scale—requires a comprehensive understanding of spatial dynamics in morphology and functions of rapidly-evolving metropolitan regions (Catalàn et al. 2008; Pérez 2010; Chorianopoulos et al. 2014; Colantoni et al. 2015).
As a complex and multifaceted phenomenon, exurban development requires a place-specific analysis based on the integrated assessment of multiple dimensions of urban growth and change (Angel et al. 2011; Salvati et al. 2013; Barrington-Leigh and Millard-Ball 2015; Carlucci et al. 2017). Analysis of commuting patterns is a key dimension in urban studies, and may produce valuable indicators identifying sequential urbanization-suburbanization cycles (Lee 2010). Under the hypothesis that discontinuous and low-density urban forms hint at a greater private vehicle use, decreased accessibility and longer average transport distances (Newman and Kenworthy 1989; Anderson et al. 1996; Newman and Kenworthy 1999; Horner 2002; Low et al. 2005; Mattingly and Morrissey 2014), commuting has been object of several studies concerning North American and European cities (e.g. Stead 2001; Giuliano and Narayan 2003; Garcia Palomares 2010). Low-density settlements and discontinuous spatial distribution of resident population in metropolitan areas have contributed to the increasing vehicle miles travelled per head (Stead 2001; Travisi et al. 2010; Zhao 2010), influencing travel distances and provision of public transport (Zagorianakos 2004; Garcia Palomares 2010; Haghshenas and Vaziri 2012). Besides, private cars became a prerequisite for living among dispersed residential areas and commercial and office places (Bhatta 2010), causing private vehicle dependency and consequent pollution, health hazards and fossil fuel consumption (Frumkin 2002).
Recent studies have addressed the relationship between spatial systems and commuting patterns (Aguilera 2005; Cervero and Day 2008; Kim and Choe 2011). Examining the travelling characteristics along recently developed areas allows identification of specific patterns and processes of urban growth (Sultana and Weber 2014). In these regards, earlier studies have evidenced how commuting patterns depend on key variables related to (1) urban form (i.e. settlement size, degree of mixed land-use, building density); and (2) factors indirectly connected with the structure of urban systems, such as availability and quality of transport infrastructure, disposable income, density of economic activities and dominant lifestyles (Stead 2001; Nicolas et al. 2003; Pacione 2005; Milakis et al. 2008; Travisi et al. 2010). The latter variables are intrinsically bound within exurban development and, for this reason, the analysis of mobility’s evolution in metropolitan regions provides relevant suggestions on the nature of urban transformations (Newman and Kenworthy 1999; Giuliano and Narayan 2003; Nicolas et al. 2003; Cervero and Day 2008; Kim and Choe 2011).
While consensus exists on the importance of measuring and understanding urban sprawl dynamics, methodologies and indicators needed to accomplish these goals have been (and still are) debated extensively (e.g. Pili et al. 2017; Di Feliciantonio et al. 2018). In fact, urban sprawl is a complex phenomenon that requires multivariate assessment of relevant socioeconomic dimensions rather than looking at individual variables, such as demographic density and population redistribution over space. This phenomenon requires a place-specific analysis (Cho et al. 2015; Rontos et al. 2016; Duvernoy et al. 2018). Analysis of commuting patterns is a valuable base of knowledge for assessment of sequential cycles of urbanization-suburbanization, mainly because vehicle miles travelled per capita are strongly influenced by economic structures and the spatial distribution of population (Di Feliciantonio and Salvati 2015). More specifically, the distance of residence from the urban core, together with settlement size, land-use mix and building density are relevant variables influencing commuting patterns (Stead 2001). Because these variables are inherently bound within the concept of urban sprawl, analyzing the evolution of transportation schemes provides useful indications on the nature of the urban transition taking place (Giuliano and Narayan 2003; Nicolas et al. 2003; Cervero and Day 2008; Kim and Choe 2011). Investigating exurban development in light of changing commuting patterns is also particularly convenient for analyzing the impact of low-density development on the sustainability of urban systems (Lee 2010). In this view, sustainable transportation is a fundamental pillar of sustainable cities, mainly because the transport sector accounts for more than 20% of global CO2 emissions and nearly 60% of global oil consumption (International Energy Agency 2012).
In addition to the intrinsic relationship with urban (and exurban) development, changes in commuting patterns over time reveal other key issues for metropolitan transformations. Level and quality of urban mobility (congestion, average travel-time, vehicle miles per-capita) and the availability of public transport additionally impact the socioeconomic sphere (Mayeres et al. 1996; Stead 2001; Mattingly and Morrissey 2014). A polycentric distribution of employment and people may shorten travelling distances as the latter locate within or close to employment sub-centres (Aguilera 2005). A comparative analysis of urban sustainability for compact and dispersed forms allows identification of (apparent and latent) linkages between land-use patterns and individual mobility (Giuliano and Narayan 2003). Higher vehicle miles travelled per-capita are mainly related to urban contexts characterized by medium–low settlement densities, dispersed population and spatially-polarized distribution of jobs (Stead 2001; Travisi et al. 2010; Zhao 2010).
Based on these premises, this paper proposes a novel approach grounded on indicators and exploratory statistics with the aim to investigate (apparent and latent) spatial patterns characterizing the local-scale commuting regime in the Athens’ metropolitan region. Athens was taken as a representative example of southern European cities experiencing a progressive shift from compact and spatially-continuous urbanization to more dispersed patterns scattered over a larger region (Chorianopoulos et al. 2010; Polyzos and Minetos 2013; Salvati 2013, 2016). Our study illustrates an original framework integrating multivariate statistical techniques (principal component analysis, multidimensional scaling, principal coordinate analysis, hierarchical clustering) and producing a comprehensive knowledge of urban spatial structures based on commuting patterns.
2 Methodology
2.1 The study area
The relationship between urban growth and local-scale commuting patterns was investigated in the Athens’ metropolitan area, a region belonging to the administrative unit of Attica, one of the most relevant historical districts of Greece, extending more than 3000 km2 of land (Fig. 1). The area overlaps the boundaries of the Athens’ Urban Atlas region identified by Eurostat and European Environment Agency for statistical reporting of city statistics (Zambon et al. 2017). At national level, Attica was subdivided in more than 100 municipalities classified as “core” (the inner cities of Athens and Piraeus), “agglomeration” and “ring” sub-districts, according to population density (Gargiulo Morelli et al. 2014). The study area includes three coastal plains (Messoghia, Marathon, Thriasio) outside the greater Athens’ area, and relevant mainland reliefs including Parnitha, Pendeli, Imitos and Egaleo mountains (Zitti et al. 2015).
The recent Athens’ development was the outcome of a series of political circumstances, as influenced by refugees’ migration from Turkey, World War II and the following civil war (Leontidou 1990). These events have significantly transformed the Athens spatial configuration resulting in a unique urban system with Piraeus since the 1940s (Milakis et al. 2008). Since the 1950s, a rapid development occurred in Athens, transforming from an industrial-based productive organization to a tertiary-centred economy, including finance, banking, insurance and real estate (Couch et al. 2007). Until the 1980s, urbanization processes involved the greater Athens’ area and more scattered urban nodes along the sea coast. Since the early 1990s, peripheral areas located North-East and North-West of Athens, have undergone extensive exurban development and real estate speculation (Delladetsima 2006; Chorianopoulos et al. 2010; Colantoni et al. 2016). The 2004 Olympic Games have attracted internal and international investments (Salvati and Serra 2016), stimulating development of new infrastructures, despite a negative increase of the Greek public debt (Chorianopoulos et al. 2014). An intense development interested some municipalities North East of Athens (Maroussi, Kifissià, Pendeli) where some Olympic installations (including the Olympic stadium) were located, stimulating a more dispersed urban growth (Arapoglou and Sayas 2009). More recently, the 2007 recession reduced building activities by more than 50% over 5 years (Salvati 2016), shaping urban development towards dispersed settlements, enhancing the role of discontinuous settlements in the Athens’ metropolitan landscape (Rontos et al. 2016).
2.2 Data and variables
This study makes use of census data released by Greek National Statistical Service (ELSTAT) for 1991, 2001 and 2011, quantifying commuting flows for specific partitions of the study area. Elementary data on commuting flows aggregated at the spatial scale of municipalities were derived from the 2001 population and household census; this kind of data was not available in the last population census (2011). Data include the number of people working (1) in the same municipality where they live and (2) in another municipality, indicating separately the name of the municipalities where they live and work. Flows from/to Greek municipalities outside the Athens’ metropolitan region were aggregated in a single class labelled as ‘external flows’ and considered a separate spatial unit in the subsequent analysis. Based on these information, a square matrix indicating the amount of residents in a given i-th municipality working in the same municipality or commuting to another municipality (in the total number of residents in a given i-th municipality) was finally prepared and analysed as follows.
2.3 An exploratory analysis of commuting patterns
The approach proposed in this paper integrates parametric and non-parametric statistical techniques (e.g. principal component analysis, principal coordinate analysis, multidimensional scaling, hierarchical clustering) in a multi-step analysis’ procedure mixing linear and non-linear frameworks, with the final aims (1) to characterize spatial patterns of commuting in the study area, (2) to identify latent distributional patterns of economic functions in inner cities, central business districts and sub-centres and, finally, (3) to infer changes in the metropolitan hierarchy of urban centres in the study area.
2.3.1 Principal component analysis
A principal component analysis (PCA) was run on commuting flows’ matrix with the aim to identify urban centres and employment sub-centres based on spatial convergence between residence’s location and commuting patterns. The number of relevant components was selected based on a screen-plot; components with eigenvalues > |2| were extracted and analyzed. A biplot representing component loadings and scores on the same factorial plane was used to identify spatial patterns of convergence (or divergence) between residence and commuting flows. Under the assumption that central place locations are characterized by a high proportion of internal workers and a high attractiveness of workers residing in neighbouring districts, this plot may discriminate central places along components, defined as municipalities with a similar value and sign of both loadings and scores on the same component. Municipalities with a divergent position of loadings and scores in the factorial plane were considered locations with a lower rank in the Attica’s metropolitan hierarchy.
The number of components extracted by the PCA according with a parsimony criterion is generally low compared with the high number of input variables (corresponding to all municipalities in the study area plus a unique spatial unit aggregating the commuting flows from/to other Greek municipalities outside the study area, labelled as ‘external flows’ in Sect. 2.2). As a consequence of the high number of input variables, the selected components may explain a relatively low variance in the data matrix, identifying linear relationships among variables and highlighting relevant (but possibly partial) spatial patterns in the data matrix. Additional analysis’ steps included statistical techniques exploring non-linear relationships among variables, as described in the following sections.
2.3.2 Principal coordinates analysis
Taken as an alternative approach to factor analysis, Principal Coordinates Analysis (PCoA) is a metric multidimensional scaling procedure analyzing an input data matrix quantifying dissimilarities between pairs of units and producing a coordinate matrix as output whose configuration minimizes a loss function. Classical scaling procedures assume that units’ coordinates are derived by eigenvalue decomposition of a proximity matrix derived adopting Euclidean distances among units. A summary plot of PCoA illustrating the position of each spatial unit along the first two axes (coordinate 1 and coordinate 2) was used to identify spatial polarities in commuting patterns, distinguishing central place locations (with a considerable rate of internal workers and attracting working from—more or less close—locations) from peripheral locations characterized by a moderate-low rate of internal workers.
2.3.3 Multidimensional scaling
Considered an alternative approach to factor analysis, a non-metric Multi-Dimensional Scaling (MDS) was run on the commuting flows’ matrix with the aim to detect meaningful spatial dimensions explaining multivariate similarities or dissimilarities (distances) between the investigated spatial units (i.e. municipalities). Contrary to a metric MDS, such as the PCoA, non-metric MDS identifies (1) a non-parametric monotonic relationship between dissimilarities in the data matrix and the Euclidean distances between spatial units, and the location of each unit in the resulting geometrical space; coordinates of each spatial unit were found minimizing a representative quantity (the so called ‘stress’), taken as a quality measure of data representation in the multivariate plane.
In this study, MDS was used to order municipalities in a two-dimensional space with the final aim to reproduce the observed (geometrical) distances based on a measure of similarity in commuting flows among spatial units. By producing a multivariate configuration that best approximates the observed distances, convergent spatial units in the MDS plane are considered urban nodes with similar demographic characteristics and socioeconomic functions, representing central places or employment sub-centres. As a result, the identification of unique or multiple sub-centres contributes to define mono-centric or polycentric metropolitan spatial structures.
2.3.4 Hierarchical clustering
A one-way Hierarchical Clustering (HC) was run on the same data matrix by use of Euclidean distances and Ward’s agglomeration rule. Cluster analysis organizes spatial units in a hierarchical sequence through a dendrogram, relating variables with a similar spatial pattern in homogeneous groups. In this study, HC was used to define and profile areas of influence of the urban centres and sub-centres identified by PCA, PCoA and MDS (see Sects. 2.3.1–2.3.3), i.e. outlining municipalities that show coherent commuting patterns gravitating on the same central place.
2.4 A summary analysis of commuting patterns in Athens
A specific set of thematic indicators mixing original variables and results derived from previous analysis was prepared with the aim at providing a comprehensive overview of commuting patterns in the study area, inferring latent spatial structures and relevant information to discriminate mono-centric from polycentric expansion. A total of 8 indicators was calculated quantifying the concentration of total workers and the density of internal workers in any municipality (being representative of central business districts and employment sub-centres), spatial diversification of commuting flows as a measure of urban centrality, and convergence between geometrical and geographic metrics computing distances among municipalities based on commuting flows’, all intended as proxies of urban hierarchy. Indicators were calculated at the municipal scale as follows: (a) the ratio of total workers in a given i-th municipality (residents + commuters) to the number of residents declaring to work (irrespective of the place of work), hereafter ‘Work-to-Res’ (b) the ratio of internal workers (i.e. working in the same municipality where they live) in the total number of residents declaring to work, irrespective of the place of work (‘self-containment’); (c) Shannon diversity (‘DiverH’) and (d) Pielou evenness (‘EquitJ’) in commuting flows (both indexes were calculated on the proportion of residents working in the same municipality or commuting to another municipality according to the data matrix described above); (e)-(h) four concordance indexes calculating, for each municipality, a Pearson linear correlation coefficient between a given geometric representation of distance among municipalities (based on the analysis of commuting flows) and linear distance over space (four distance metrics were considered here: ‘Euclidean’, ‘Manhattan’, ‘Geographical’ and a non-parametric ‘Rho’ measure of similarity/dissimilarity in commuting flows). Physical distances among municipalities were calculated using the spatial coordinates of the municipal ‘centroid’ determined with ArcGIS 10 package (ESRI Inc., Redwoods, USA) according to the relative function that computes the centre of gravity of each municipality. The Shannon H’ index was calculated for each municipality as follows:
where pi is the relative proportion of people working in each i-th municipality and residing in a j-th municipality (with both i = j and i ≠ j). For each municipality, the J index was derived from the Shannon index applied to the proportion of people working in a given i-th municipality and residing in a j-th municipality (with both i = j and i ≠ j) according to the formula:
where Hmax is the natural logarithm of the number of considered classes. Shannon index ranges from 0 (low diversity) to infinity (high diversity in working place destinations), while Pielou’s index ranges between 0 (low evenness) and 1 (high evenness in working place destinations). High H’ values characterize employment centres and sub-centres attracting workers from a large number of neighbouring locations. High J values indicate municipalities with a spatially-balanced gravitation structure, i.e. with an equal distribution of workers residing in neighbouring places. Maps were used to illustrate the distribution of the 8 indicators of commuting structure in the study area.
A summary PCA was finally run on the data matrix constituted of the 8 indicators at each municipal location with the objective to provide a summary evaluation of mono-centric versus polycentric urban structures based on commuting patterns in Attica.
3 Results
3.1 Descriptive and inferential statistics
Population living and working in the same municipality as a proportion of total resident population was rather variable in the study area between 1991 and 2011, with a peak observed in 2001 (49.5%). Athens’ prefecture concentrated a slightly lower proportion of self-contained population (Table 1), being higher in peripheral districts of Eastern Attica, Western Attica and Piraeus (including Salamina island). The highest and the lowest self-containment rates were respectively observed in Western Attica in 2001 (64.3%) and in Athens in 2011 (35.2%). The spatial organization of the Athens’ metropolitan region was inferred from a linear analysis regressing population density and distance from downtown Athens over a relatively long time period using municipal scale data. Results of this analysis (Table 2) indicate a progressive strengthening of a mono-centric model up to the early 1980s with the highest slopes—indicating a pronounced urban gradient – being associated with the highest intercepts—indicating population concentration in central municipalities of Athens and Piraeus. In the following decades, the mono-centric model was less effective to explain the spatial structure of Athens, evidencing a moderate decline in both urban slope and Athens’ (demographic and economic) centrality in the Greek metropolitan system (Fig. 2).
3.2 A preliminary analysis of commuting patterns in Athens
Under the hypothesis that a progressive convergence between loadings (place of residence) and scores (place of work) indicates a high rate of commuters’ self-containment, a PCA biplot (Fig. 3) was used to identify spatial alignment between places of residence and work in the study area. The analysis contributes to identify employment sub-centres with business districts attracting workers from neighbouring locations. The quadrants of the factorial plane (PC1 vs. PC2) classify municipalities that show convergence between residence and working places in four gravitational fields respectively centred on: (1) the municipality of Athens (code A101, upper right quadrant; see “Appendix” for code’s list), (2) the municipality of Piraeus (code A401, upper left quadrant), (3) the municipalities of Kifissia, Maroussi, Chalandri and Aghia Paraskevì, an emerging business district forming the core of the Olympic ‘cluster’ (codes A108, A123, A143 and A103, lower right quadrant) and, finally, (4) the municipalities of Elefsina, Aspropyrgos and Mandra, situated in the Thriasio plain, west of Athens, and hosting a consolidated industrial centre (codes A301 and A307, lower left quadrant). The remaining municipalities in the study area were ordered according with gravitation intensity to each of the four urban centres illustrated above. Municipalities with compact and dense settlements situated in the Greater Athens’ area were gravitating on downtown Athens. Commuting flows of residents in coastal and island municipalities of south-western Attica were gravitating on Piraeus, because of high accessibility by sea. Population residing in western Attica municipalities and other fringe areas north and east of Athens gravitated on Thriasio municipalities, thanks to the infrastructural development promoted by 2004 Olympics—e.g. the Attiki highway and the new national motorways, connecting western Attica with both northern and eastern Attica. Municipalities in the northern side of Attica and those placed in the rapidly-expanding Messoghia district—surrounding the new Athens’ International Airport—gravitated on the Olympic district. Infrastructural development, and especially a new underground line and the improved suburban train network have consolidated these commuting patterns.
Biplot of a principal component analysis analyzing commuting patterns in Attica (codes indicate individual municipalities in the study area, see list in “Appendix”); gravitation fields are outlined with the related central location (Piraeus, Athens, Thriasio and the Olympic district)
3.3 Identifying spatial polarities and urban poles
A plot based on a metric Multidimensional Scaling (PCoA) identified urban centres with the highest attractiveness for workers residing in neighbouring municipalities along axis 1 (Fig. 4). Axis 2 identified a spatial gradient distinguishing central municipalities (mainly placed in the Athens’ conurbation and gravitating on Athens, Piraeus or Thriasio employment centres) from rural municipalities in peripheral locations, e.g. Lavrio (A214), Aghios Kostantinos (A261), Koropi (A213) and Keratea (A212), all situated in south-eastern Attica 30-40 km far away from Athens.
3.4 A non-metric analysis of gravitational fields
Non-metric MDS was used to classify central and peripheral municipalities in the Attica region based on the four gravitational areas (Athens, Piraeus, Maroussi Olympic districts, Thriasio industrial district) identified in Sects. 3.1 and 3.2 (Fig. 5). Municipalities clustering close to 0 along both MDS axes are considered urban centres with a moderate gravitation on one of the four central locations. Municipalities clustered far away from the centre of the MDS axes are considered peripheral locations gravitating on one of the four central places, according to the quadrant they are located in. Peripheral locations include island municipalities with workers commuting mainly with Athens and Piraeus, coastal municipalities situated 30-40 km away from Athens with workers commuting preferentially with the new Olympic Central Business District and inland municipalities west of Athens with workers commuting primarily with Thriasio industrial locations.
3.5 Profiling latent spatial structures of commuting patterns
A cluster analysis was run on the data matrix reporting commuting flows within- and between-municipalities in the study area (Fig. 6). Attica municipalities were discriminated in two groups, evidencing municipalities with working population gravitating on Athens and the new Olympic business district on the one side, and on Piraeus and Thriasio industrial district on the other side. Results based on similarities in commuting patterns across the study area, outline that two distinct functional areas can be detected in the region (respectively centred on Athens and Piraeus), with formation (or consolidation) of two related employment sub-centres (Olympic district and Thriasio district). These results confirm the prevalent di-centric spatial structure of the study area, based on (1) the traditional Athens-Piraeus di-pole and (2) emergent sub-centres attracting commuting flows from neighbouring municipalities.
3.6 An indicator-based analysis of Athens’ metropolitan configuration
Selected indicators summarizing results from earlier analysis of commuting flows in the study area were calculated and illustrated through maps (Fig. 7). Indicators provide a multidimensional overview on spatial patterns of workers commuting within and between municipalities in Attica, outlining structural characteristics of the metropolitan region. The ratio of total workers in a given i-th municipality (including resident workers and commuters) to the number of residents declaring to work (irrespective of the place of work) shows a rather fragmented spatial distribution with higher values observed in (1) central municipalities of Athens, Piraeus and the neighbouring urban municipalities, (2) municipalities east of Athens (Messoghia district close to the Athens’ International Airport) and (3) municipalities east of Athens in Thriasio, Megara and Salamina districts. The ratio of internal workers (i.e. working in the same municipality they live) in the total number of residents declaring to work (irrespective of the place of work) outlines a gradient from urban central locations and rural, peripheral municipalities (both characterized by high values of the indicators) to peri-urban and economically-dynamic municipalities in the north-eastern fringe of Athens. Diversification in the spatial profile of commuting flows at the municipal scale was evaluated using H’ diversity and J evenness indexes. These variables identify a consolidated urban–rural gradient with the highest values associated to urban municipalities and the lowest values recorded in peripheral locations. Indexes of spatial concordance in commuting flow patterns in Attica evidence the urban footprint of greater Athens, attributing the highest values to central municipalities around Athens and the new business district. Central locations of Piraeus and Thriasio district showed less intense commuting patterns compared with Athens’ municipality.
Spatial distribution of selected commuting indicators in the study area (see Sect. 2.4). (a) Total workers to residential workers (i), (b) Self-containing rate of commuting workers, (c) Spatial diversity (H’) of commuting flows, (d) Spatial evenness (J) of commuting flows, (e) Manhattan distances in commuting flows, (f) Euclidean distances in commuting flows, (g) Geographical distances in commuting flows, (h) Rho distances in commuting flows
The PCA run on the spatial distribution of the abovementioned indicators outlines spatial similarities in commuting flows over the study area (Fig. 8). Component 1 extracted 48.7% of the total variance and was associated with distance metrics (‘Euclidean’, ‘Manhattan’ and ‘Geographical’, positive loadings; ‘Rho’, negative loadings); component 2 extracted 25.8% of the total variance and was associated to the ratio of total workers in a given i-th municipality (including resident workers and commuters) to the number of residents declaring to work irrespective of the place of work (‘Work-to-Res’). Diversity metrics received high loadings on both components. The PCA biplot is a meaningful tool to discriminate municipalities in central locations and peripheral places, highlighting the functional hierarchy of urban centres in Attica.
4 Discussion
The most recent urban transitions observed in Mediterranean Europe have altered the consolidated and historical bonds between mono-centric forms and spatially-polarized economic functions (Andreotti et al. 2001; Allen et al. 2004; Páez 2007; Arbaci 2008; Salvati and Gargiulo Morelli 2014). Although compact growth was the most traditional spatial trend in such contexts, several Mediterranean cities experienced urban sprawl in the last decades (Salvati and Carlucci 2016) with relevant socioeconomic and environmental consequences (King et al. 1997; Salvati 2013; Artmann 2014). Mitigating the negative effects of dispersed urban expansion requires implementation of a sustainable development strategy, focusing on a better comprehension of the nature and consequences of urban transitions (Burchell et al. 2005; Hall and Pain 2006; Couch et al. 2007; Bhatta 2010; Kazemzadeh-Zow et al. 2017).
An integrated approach is essential to identify the multifaceted spatial relationships among land-use in metropolitan areas (Guillain and Le Gallo 2010; Díaz-Pacheco and García-Palomares 2014; De Rosa and Salvati 2016). Considering both morphological and socioeconomic indicators, a consistent exploration of similarities and divergences concerning the spatial development of contemporary cities can be achieved (Salvati et al. 2016). Based on this statement, the present work focused on the Athens’ region, an emblematic urban case owing to the intrinsic nature and implications of its urban transition on structural and historical traits (Leontidou 1990; King et al. 1997; Burgel 2004). By exploring the main characteristics of the Athens’ metropolitan structure from commuting data, our analysis contributes to the debate on sustainability of compact versus dispersed forms. Being grounded on compact urban settlements, characterized by a high degree of social mixité and land-use heterogeneity, the city of Athens presents a mono-centric structure, with a growing distance from the inner city, producing an increase in private mobility, especially in suburban areas (Milakis et al. 2008). Commuting rate in rural areas resulted to be low since local labour force was employed locally in agriculture and the tourism sector (e.g. Gakenheimer 1999). However, low- and intermediate-density areas in North-Eastern Attica were characterized by intense commuting. In this area, infrastructure-driven sprawl intensified in recent years (e.g. Stead 2001). Employment sub-centres (resident population > 10,000) emerged as a response to the infrastructural development driven by the 2004 Olympic Games, featuring a particularly high concentration of workers commuting from the surrounding municipalities.
Integrating multiple statistical techniques allowed a comprehensive knowledge of urban spatial structures based on commuting patterns. The latter can describe the progressive shift in local urbanization patterns in AMR, from compact and spatially-continuous forms to more dispersed settlements scattered over a larger region. Spatial convergence between places of residence and work can be identified in the AMR. A high rate of commuters’ self-containment identifies employment centres with business districts that attract workers from adjacent places.
Different municipal profiles emerged considering the convergence between residence and workplaces based on their gravitation intensity. First, the municipality of Athens attracted working population from the surrounding compact and dense settlements (Delladetsima 2006). Second, the municipality of Piraeus attracted commuting flows from coastal and island municipalities in south-western Attica due to the high accessibility by sea (Rontos et al. 2016). Third, the municipalities of Kifissia, Maroussi, Chalandri and Aghia Paraskevì (forming a new business district in Attica and the core of the Olympic ‘cluster’), attracted working population residing in the northern side of the Athens’ urban agglomeration and those living in the rapidly-expanding Messoghia district, e.g. surrounding the new Athens’ International Airport. The self-containment rate of municipalities located around the main Olympic pole was generally low (Arapoglou and Sayas 2009). Fourth, industrial municipalities of Elefsina and Mandra involved western municipalities which are peri-urban and economically-dynamic north-eastern fringe areas gravitated on Thriasio municipalities (Chorianopoulos et al. 2010). This background is the result from the infrastructural development driven by 2004 Olympics, mainly the Attica highway and the new national motorways, connecting western Attica with northern and eastern Attica.
In brief, urban centres attracted workers residing in neighbouring municipalities. According to distinctive characteristics of commuting flows (spatial direction and intensity), our analysis allows identification of central districts and rural municipalities in peripheral locations. Two functional areas are detected in the region (Athens and Piraeus), with formation of two related employment sub-centres (Olympic district and Thriasio district). A prevalent di-centric spatial structure, grounded in the traditional Athens-Piraeus di-pole and in emerging sub-centres, attracted commuting flows from neighbouring municipalities. Municipalities in central locations and peripheral places highlighted the functional hierarchy of urban centres in Attica (Rontos et al. 2016).
The evolution of commuting patterns contributed to shed light on urban sprawl and recent population dynamics in the AMR. Even though the self-containment rate of municipalities has generally consolidated throughout the AMR, systematically higher commuting rates were observed in the urban ring and in the prefecture of Eastern Attica. In fact, the presence of economic activities linked with agriculture and tourism sectors offered job employment to the working residents living in these areas (Giannopoulos 1977; Zagorianakos 2004; Milakis et al. 2008).
The recent urban expansion in the form of low-density development was a substantial obstacle to the achievement of sustainable transportation (Lee 2010; Gargiulo Morelli et al. 2014; Pojani and Stead 2015). Contrary to planned urbanization processes, exurban development in the Mediterranean region often appeared in informal ways (Zambon et al. 2017). Urban planning can play a crucial role in the attempt to mitigate the environmental, social and economic impacts of increasing commuting (Nicolas et al. 2003). Land-use policies should prevent out-of-plan low-density settlements, while promoting the consolidation of polycentric sub-centres (Zambon et al. 2018). These sub-centres can reproduce the traditional compact mono-centric model, resulting in self-contained, mixed service-residential settlements (Zhao 2010). Moreover, an accessible and well-connected public transport network at the regional level would limit private transportation, which is considered less environmentally efficient and socially equitable (Kim and Choe 2011). Spatial inequality depends on both the geographical scale and mobility, which results in social segregation (Rothwell and Massey 2015; Wei 2015; Ewing et al. 2016). Considering together residential mobility and commuting, patterns of social segregation in metropolitan regions can be examined more comprehensively (Maloutas 2004, 2007). For instance, low-income residents settling in urban municipalities have restricted transportation mobility (Chapple 2001; Ong and Miller 2005; Grengs 2010). In these regards, planners and policy makers may adopt a comprehensive strategy aimed at improving social mobility and increasing housing and work accessibility at affordable costs (Ewing et al. 2016).
Projects improving transport networks were implemented in Attica (e.g. new underground lines, suburban railways and the Athens’ ring road), shaping a multi-nucleated territorial asset, particularly evident in the case of eastern Attica (Colantoni et al. 2016). However, development of many suburban centres has been still negatively influenced by infrastructural lacks (Pili et al. 2017). Empirical results of our study revealed that an unsustainable commuting profile was found primarily in low-density settlements, especially in North-Eastern Attica, where major infrastructure development took place only for the 2004 Olympic Games (Delladetsima 2006; Milakis et al. 2008; Chorianopoulos et al. 2010).
5 Conclusions
Considering together urban growth and commuting spatial patterns constitutes a knowledge base to implement appropriate land-use policies as tools for sustainable urban development. In the study area factors including (1) the concentration of industrial activities in few urban poles, (2) the development of infrastructural networks including the Olympic venues, (3) the consolidation of mixed population/employment sub-centres and, finally, (4) the relatively low cost of land suggest that recently transformed areas are candidate targets for integrated sustainable commuting strategies. Such a strategy contributes to design more efficient policies promoting public transportation, together with the conservation of traditional rural villages characterized by self-contained settlements and moderately high quality of life.
References
Aguilera, A.: Growth in commuting distances in French polycentric metropolitan areas: Paris, Lyon and Marseille. Urban Stud. 42(9), 1537–1547 (2005)
Allen, J., Barlow, J., Leal, J., Maloutas, T., Padovani, L.: Housing and Welfare in Southern Europe. Wiley, London (2004)
Anderson, W.P., Kanaroglou, P.S., Miller, E.J.: Urban form, energy and the environment: a review of issues, evidence and policy. Urban Stud. 33, 7–35 (1996)
Andreotti, A., Garcia, S.M., Gomez, A., Hespanha, P., Kazepov, Y., Mingione, E.: Does a Southern European model exist? J. Eur. Area Stud. 9(1), 43–62 (2001)
Angel, S., Parent, J., Civco, D.L., Blei, A., Potere, D.: The dimensions of global urban expansion: estimates and projections for all countries, 2000–2050. Prog. Plan. 75, 53–107 (2011)
Arapoglou, V.P., Sayas, J.: New facets of urban segregation in southern Europe—gender, migration and social class change in Athens. Eur. Urban Reg. Stud. 16(4), 345–362 (2009)
Arbaci, S.: (Re)Viewing ethnic residential segregation in southern European cities: housing and urban regimes as mechanisms of marginalisation. Hous. Stud. 23(4), 589–613 (2008)
Artmann, M.: Assessment of soil sealing management responses, strategies, and targets toward ecologically sustainable urban land use management. Ambio 43, 530–541 (2014)
Balta, M.O., Eke, F.: Spatial reflection of urban planning in metropolitan areas and urban rent; a case study of Cayyolu, Ankara. Eur. Plann. Stud. 19(10), 1817–1838 (2011)
Barrington-Leigh, C., Millard-Ball, A.: A century of sprawl in the United States. Proc. Natl. Acad. Sci. 112(27), 8244–8249 (2015)
Beriatos, E., Gospodini, A.: “Glocalising” urban landscapes: Athens and the 2004 olympics. Cities 21, 187–202 (2004)
Bhatta, B.: Analysis of Urban Growth and Sprawl from Remote Sensing Data. Springer, Berlin (2010)
Burchell, R.W., Downs, A., McCann, B., Mukherji, S.: Sprawl Costs—Economic Impacts of Unchecked Development. Island Press, Washington (2005)
Burgel, G.: Athènes, de la balkanisation à la mondialisation. Méditerranée 103(3–4), 59–63 (2004)
Carlucci, M., Grigoriadis, E., Rontos, K., Salvati, L.: Revisiting an hegemonic concept: long-term ‘Mediterranean Urbanization’ in between city re-polarization and metropolitan decline. Appl. Spat. Anal. Policy 10(3), 347–362 (2017)
Catalàn, B., Saurì, D., Serra, P.: Urban sprawl in the Mediterranean? Patterns of growth and change in the Barcelona Metropolitan Region 1993–2000. Landsc. Urban Plan. 85, 174–184 (2008)
Ceccarelli, T., Bajocco, S., Perini, L., Salvati, L.: Urbanisation and land take of high quality agricultural soils—exploring long-term land use changes and land capability in Northern Italy. Int. J. Environ. Res. 8(1), 181–192 (2014)
Cervero, D., Day, J.: Suburbanization and transit-oriented development in China. Transp. Policy 15(5), 315–323 (2008)
Chapple, K.: Time to work: job search strategies and commute time for women on Welfare in San Francisco. J. Urban Aff. 23, 155–173 (2001)
Cho, S.H., Kim, S.G., Roberts, R.K., Lambert, D.M., Kim, T.: Effects of land-related policies on land development during a real estate boom and a recession. Growth Change 46(2), 218–232 (2015)
Chorianopoulos, I., Pagonis, T., Koukoulas, S., Drymoniti, S.: Planning, competitiveness and sprawl in the Mediterranean city: the case of Athens. Cities 27, 249–259 (2010)
Chorianopoulos, I., Tsilimigkas, G., Koukoulas, S., Balatsos, T.: The shift to competitiveness and a new phase of sprawl in the Mediterranean city: enterprises guiding growth in Messoghia—Athens. Cities 39, 133–143 (2014)
Colantoni, A., Grigoriadis, E., Sateriano, A., Venanzoni, G., Salvati, L.: Cities as selective land predators? A lesson on urban growth, (un)effective planning and sprawl containment. Sci. Total Environ. 545–546, 329–339 (2016)
Colantoni, A., Mavrakis, A., Sorgi, T., Salvati, L.: Towards a ‘polycentric’ landscape? Reconnecting fragments into an integrated network of coastal forests in Rome. Rendiconti Accademia Nazionale dei Lincei 26(3), 615–624 (2015)
Couch, C., Petschel-held, G., Leontidou, L.: Urban Sprawl in Europe: Landscapes, Land-Use Change and Policy. Blackwell, London (2007)
Cuadrado-Ciuraneta, S., Durà-Guimerà, A., Salvati, L.: Not only tourism: unravelling suburbanization, second-home expansion and “rural” sprawl in Catalonia, Spain. Urban Geogr. 38(1), 66–89 (2017)
De Rosa, S., Salvati, L.: Beyond a ‘side street story’? Naples from spontaneous centrality to entropic polycentricism, towards a ‘crisis city’. Cities 51, 74–83 (2016)
Delladetsima, P.: The emerging property development pattern in Greece and its impact on spatial development. Eur. Urban Reg. Stud. 13(3), 245–278 (2006)
Di Feliciantonio, C., Salvati, L.: ‘Southern’ alternatives of urban diffusion: investigating settlement characteristics and socio-economic patterns in three Mediterranean regions. Tijdschrift voor Economische en Sociale Geografie 106(4), 453–470 (2015)
Di Feliciantonio, C., Salvati, L., Sarantakou, E., Rontos, K.: Urban sprawl, labour market and class diversification: evidences from a pre-crisis Mediterranean City. Qual. Quant. 52(4), 1501–1522 (2018)
Díaz-Pacheco, J., García-Palomares, J.C.: Urban sprawl in the Mediterranean urban regions in Europe and the crisis effect on the urban land development: Madrid as study case. Urban Studies Research, Article ID 807381 (2014)
Duvernoy, I., Zambon, I., Sateriano, A., Salvati, L.: Pictures from the other side of the fringe: urban growth and peri-urban agriculture in a post-industrial city (Toulouse, France). J. Rural Stud. 57, 25–35 (2018)
Ewing, R., Hamidi, S., Grace, J.B., Wei, Y.D.: Does urban sprawl hold down upward mobility? Landsc. Urban Plan. 148, 80–88 (2016)
Frumkin, H.: Urban sprawl and public health. Public Health Rep. 117(3), 201 (2002)
Gakenheimer, R.: Urban mobility in the developing world. Transp. Res. A 33(7), 671–689 (1999)
Garcia Palomares, J.C.: Urban sprawl and travel to work: the case of the metropolitan area of Madrid. J. Transp. Geogr. 18, 197–213 (2010)
Gargiulo Morelli, V., Rontos, K., Salvati, L.: Between suburbanisation and re-urbanisation: revisiting the urban life cycle in a Mediterranean compact city. Urban Res. Pract. 7(1), 74–88 (2014)
Giannakourou, G.: Transforming spatial planning policy in Mediterranean countries: europeanization and domestic change. Eur. Plan. Stud. 13, 319–331 (2005)
Giannopoulos, G.A.: An analysis of trip length and land use patterns in the greater Athens area. Transportation 6, 379–392 (1977)
Giuliano, G., Narayan, D.: Another look at travel patterns and urban form: the US and Great Britain. Urban Stud. 40(11), 2295–2312 (2003)
Grengs, J.: Job accessibility and the modal mismatch in Detroit. J. Transp. Geogr. 18, 42–54 (2010)
Guillain, R., Le Gallo, J.: Agglomeration and dispersion of economic activities in and around Paris: an exploratory spatial data analysis. Environ. Plan. B Plan. Des. 37, 961–981 (2010)
Haase, A., Kabisch, S., Steinführer, A., Bouzarovski, S., Hall, R., Ogden, P.: Spaces of reurbanisation: exploring the demographic dimension of inner-city residential change in a European setting. Popul. Space Place 16(5), 443–463 (2010)
Haghshenas, H., Vaziri, M.: Urban sustainable transportation indicators for global comparison. Ecol. Ind. 15, 115–121 (2012)
Hall, P., Pain, K.: The Polycentric Metropolis. Learning from Mega-City Regions in Europe. Earthscan, London (2006)
Hayek, U.W., Jaeger, J.A., Schwick, C., Jarne, A., Schuler, M.: Measuring and assessing urban sprawl: what are the remaining options for future settlement development in Switzerland for 2030? Appl. Spat. Anal. Policy 4(4), 249–279 (2011)
Horner, M.W.: Extensions to the concept of excess commuting. Environ. Plan. A 34, 543–566 (2002)
International Energy Agency: CO2 Emissions from Fuel Combustion. International Energy Agency, Paris (2012)
Kazemzadeh-Zow, A., Zanganeh Shahraki, S., Salvati, L., Neisani Samani, N.: A spatial zoning approach to calibrate and validate urban growth models. Int. J. Geogr. Inf. Sci. 31(4), 763–782 (2017)
Kim, K.J., Choe, S.C.: In search of sustainable urban form for Seoul. Libr. Sustain. Urban Regen. 10, 43–65 (2011)
King, R., Proudfoot, L., Smith, B.: The Mediterranean: Environment and Society. Arnold, London (1997)
Lee, S.: Transport and the recession: an opportunity to promote sustainable transport. Int. Plan. Stud. 15(3), 213–226 (2010)
Leontidou, L.: The Mediterranean city in transition. Cambridge University Press, Cambridge (1990)
Leontidou, L., Afouxenidis, A., Kourliouros, E., Marmaras, E.: Infrastructure-related urban sprawl: mega-events and hybrid peri-urban landscapes in southern Europe. In: Couch, C., Petschel-Held, G., Leontidou, L. (eds.) Urban sprawl in Europe: landscapes, land-use change and policy, pp. 71–101. Blackwell, Oxford (2007)
Low, N., Gleeson, B., Green, R., Radovic, D.: The Green City: Sustainable Homes, Sustainable Suburbs. University of New South Wales, Sydney (2005)
Maloutas, T.: Segregation and residential mobility: spatially entrapped social mobility and its impact on segregation in Athens. Eur. Urban Reg. Stud. 11(3), 195–211 (2004)
Maloutas, T.: Middle class education strategies and residential segregation in Athens. J. Educ. Policy 22(1), 49–68 (2007)
Mattingly, K., Morrissey, J.: Housing and transport expenditure: socio-spatial indicators of affordability in Auckland. Cities 38, 69–83 (2014)
Mayeres, I., Ochelen, S., Proost, S.: The marginal external costs of urban transport. Transp. Res. Part D Transp. Environ. 1(2), 111–130 (1996)
Milakis, D., Vlastos, T., Barbopoulos, N.: Relationships between urban form and travel behaviour in Athens, Greece. A comparison with western European and north American results. Eur. J. Transp. Res. 8(3), 201–215 (2008)
Newman, P., Kenworthy, J.: Sustainability and Cities: Overcoming Automobile Dependence. Island Press, Washington DC (1999)
Newman, P.W.G., Kenworthy, J.R.: Gasoline consumption and cities. J. Am. Plan. Assoc. 55, 24–37 (1989)
Nicolas, J.P., Pochet, P., Poimboeuf, H.: Towards sustainable mobility indicators: application to the Lyons conurbation. Transp. Policy 10(3), 197–208 (2003)
Nuissl, H., Rink, D.: The ‘production’ of urban sprawl in eastern Germany as a phenomenon of post-socialist transformation. Cities 22(2), 123–134 (2005)
Ong, P.M., Miller, D.: Spatial and transportation mismatch in Los Angeles. J. Plan. Educ. Res. 25, 43–56 (2005)
Pacione, M.: Urban Geography: A Global Perspective. Routledge, London (2005)
Páez, A.: Spatial perspectives on urban systems: developments and directions. J. Geogr. Syst. 9(1), 1–6 (2007)
Paul, V., Tonts, M.: Containing urban sprawl: trends in land-use and spatial planning in the Metropolitan Region of Barcelona. J. Environ. Plan. Manag. 48(1), 7–35 (2005)
Pérez, J.M.G.: The real estate and economic crisis: an opportunity for urban return and rehabilitation policies in Spain. Sustainability 2(6), 1571–1601 (2010)
Pili, S., Grigoriadis, E., Carlucci, M., Clemente, M., Salvati, L.: Towards sustainable growth? A multi-criteria assessment of (changing) urban forms. Ecol. Ind. 76, 71–80 (2017)
Pojani, D., Stead, D.: Sustainable urban transport in the developing world: beyond megacities. Sustainability 7(6), 7784–7805 (2015)
Polyzos, S., Minetos, D.: Informal housing in Greece: a multinomial logistic regression analysis at the regional level. Eur. Spat. Res. Policy 20(2), 57–85 (2013)
Rontos, K., Grigoriadis, S., Sateriano, A., Syrmali, M., Vavouras, I., Salvati, L.: Lost in protest, found in segregation: divided cities in the light of the 2015 ‘Oki’ referendum in Greece. City Cult. Soc. 7(3), 139–148 (2016)
Rothwell, J.T., Massey, D.S.: Geographical effects of intergenerational income mobility. Econ. Geogr. 91(1), 83–106 (2015)
Salvati, L.: Urban expansion and high-quality soil consumption—an inevitable spiral? Cities 31, 349–356 (2013)
Salvati, L.: The dark side of the crisis: disparities in per capita income (2000–12) and the urban-rural gradient in Greece. Tijdschrift voor Economische en Sociale Geografie 107(5), 628–641 (2016)
Salvati, L., Carlucci, M.: In-between stability and subtle changes: urban growth, population structure, and the city life cycle in Rome. Popul. Space Place 22(3), 216–227 (2016)
Salvati, L., Gargiulo Morelli, V.: Unveiling urban sprawl in the Mediterranean region: towards a latent urban transformation? Int. J. Urban Reg. Res. 38(6), 1935–1953 (2014)
Salvati, L., Morelli, V.G., Rontos, K., Sabbi, A.: Latent exurban development: city expansion along the rural-to-urban gradient in growing and declining regions of Southern Europe. Urban Geogr. 34(3), 376–394 (2013)
Salvati, L., Sateriano, A., Grigoriadis, S.: Crisis and the city: profiling urban growth under economic expansion and stagnation. Lett. Spat. Resour. Sci. 9(3), 329–342 (2016)
Salvati, L., Serra, P.: Estimating rapidity of change in complex urban systems: a multidimensional, local-scale approach. Geogr. Anal. 48(2), 132–156 (2016)
Schneider, A., Woodcock, C.E.: Compact, dispersed, fragmented, extensive? A comparison of urban growth in twenty-five global cities using remotely sensed data, pattern metrics and census information. Urban Stud. 45(3), 659–692 (2008)
Stead, D.: Relationships between land use, socio-economic factors and travel patterns in Britain. Environ. Plan. B 28(4), 499–528 (2001)
Sultana, S., Weber, J.: The nature of urban growth and the commuting transition: endless sprawl or a growth wave? Urban Stud. 51(3), 544–576 (2014)
Terando, A.J., Costanza, J., Belyea, C., Dunn, R.R., McKerrow, A., Collazo, J.A.: The southern megalopolis: using the past to predict the future of urban sprawl in the Southeast US. PLoS ONE 9(7), e102261 (2014)
Travisi, C.M., Camagni, R., Nijkamp, P.: Impacts of urban sprawl and commuting: a modeling study for Italy. J. Transp. Geogr. 18, 382–392 (2010)
Wei, Y.H.D.: Spatiality of regional inequality. Appl. Geogr. 61, 1–10 (2015)
Zagorianakos, E.: Athens 2004 Olympic Games’ Transportation Plan: a missed opportunity for Strategic Environmental Assessment (SEA) integration. J. Transp. Geogr. 12, 115–125 (2004)
Zambon, I., Serra, P., Sauri, D., Carlucci, M., Salvati, L.: Beyond the ‘Mediterranean city’: socioeconomic disparities and urban sprawl in three Southern European cities. Geografiska Annaler Ser. B Human Geogr. 99(3), 319–337 (2017)
Zambon, I., Benedetti, A., Ferrara, C., Salvati, L.: Soil matters? A multivariate analysis of socioeconomic constraints to urban expansion in Mediterranean Europe. Ecol. Econ. 146, 173–183 (2018)
Zhao, P.: Sustainable urban expansion and transportation in a growing megacity: consequences of urban sprawl for mobility on the urban fringe of Beijing. Habitat Int. 34, 236–243 (2010)
Zitti, M., Ferrara, C., Perini, L., Carlucci, M., Salvati, L.: Long-term urban growth and land-use efficiency in Southern Europe: implications for sustainable land management. Sustainability 7(3), 3359–3385 (2015)
Author information
Authors and Affiliations
Corresponding author
Appendix
Appendix
See Table 3.
Rights and permissions
About this article
Cite this article
Ciommi, M., Chelli, F.M. & Salvati, L. Integrating parametric and non-parametric multivariate analysis of urban growth and commuting patterns in a European metropolitan area. Qual Quant 53, 957–979 (2019). https://doi.org/10.1007/s11135-018-0798-2
Published:
Issue Date:
DOI: https://doi.org/10.1007/s11135-018-0798-2